- 1Plant Breeding, Genetics, and Biotechnology Division, International Rice Research Institute, Metro Manila, Philippines
- 2Department of Phytomedicine, Leibniz Universität Hannover, Hannover, Germany
- 3Crop and Environmental Sciences Division, International Rice Research Institute, Metro Manila, Philippines
Attempting to achieve long-lasting and stable resistance using uniformly deployed rice varieties is not a sustainable approach. The real situation appears to be much more complex and dynamic, one in which pathogens quickly adapt to resistant varieties. To prevent disease epidemics, deployment should be customized and this decision will require interdisciplinary actions. This perspective article aims to highlight the current progress on disease resistance deployment to control bacterial blight in rice. Although the model system rice-Xanthomonas oryzae pv. oryzae has distinctive features that underpin the need for a case-by-case analysis, strategies to integrate those elements into a unique decision tool could be easily extended to other crops.
Why Customize the Deployment of Bacterial Blight Resistance Genes?
Very often, elite rice varieties carrying effective resistance genes are distributed across broad geographic areas to maximize their socioeconomic impact. Eventually, the resistance gene will overlap with virulent pathogen populations that persist in low frequency. Prolonged exposure will increase selected population and leads to an outbreak. In this case, deployment is perhaps the most influential event compromising durability. Preventing disease epidemics requires a deeper understanding of the biological systems and interdisciplinary approaches to interconnect the factors that account for conducive environments, locally effective genes, and pathogen dynamics. A systematic monitoring of the pathogen population, which incorporates current understanding of effector biology, emerges as a key aspect to drive pathogen-informed deployment. However, it is essential that such information is readily transferred to breeding pipelines to guarantee the right variety profiles. We also believe that geographic information systems can be used to couple disease forecasting models with in-field surveys and other on-the-ground work to map epidemics in real time and therefore be integrated into a unique decision tool.
Bacterial blight (BB), caused by Xanthomonas oryzae pv. oryzae (Xoo), is the most important bacterial disease of rice. At least 39 resistance genes (Xa) have been identified from wild and cultivated accessions (Khan et al., 2014; Zhang et al., 2014a); among which Xa4, xa5, xa13, and Xa21 appear to be widely used in breeding programs across Asia (Khan et al., 2014). Disease resistance deployment to control Xoo emerges as a perfect case for analysis because (i) the disease is widely distributed across rice-growing regions worldwide, (ii) resistance has a strong race-specific component, and (iii) many Xa genes have been incorporated into released varieties. A game-changing feature of the pathosystem is that Xoo uses transcription activator-like (TAL) effectors to promote colonization and ensure nutrient uptake. In contrast to other rice pathosystems, Xa genes can be classified into distinct functional categories and only a small number appears to encode for NBS-LRR proteins (Boch et al., 2014). In this paper, we describe the key elements that need to be considered if we are to implement a strategy to customize the deployment of Xa genes in rice agroecosystems.
Breeding Fast, Breeding Precisely
Conventional breeding in rice uses pedigree breeding and selection, which employs a forward breeding approach based on traits of interest. The selection of advanced pedigree lines and recombinant inbred lines requires a long process that can take 8-9 years to generate elite lines for varietal release. With the recent advances in technologies, breeding for target traits can be fast-tracked by the application of marker-assisted selection (MAS) focusing on improving tolerance to abiotic stresses—drought, submergence, salinity, and soil problems—for unfavorable environments, along with increased yield, biotic stress resistance, and improved grain quality, traits that are also required for a favorable environment. Recently, the duration for developing improved varieties through forward breeding was decreased to 5-6 years through implementing rapid generation advance and MAS techniques. Further enhancement uses marker-assisted backcrossing (MABC) retaining the good traits of the recipient parents, combined with precision in incorporating specific traits of interest into high-yielding mega-varieties with superior grain quality, thus further reducing the time of producing elite lines to 3-4 years. Recently, a further transformation in breeding has been revolutionizing precision breeding while doubling the rate of genetic gains. The availability of 3,000 sequenced rice genomes provides an unprecedented wealth of data to mine alleles for novel genes (Li et al., 2014). This is coupled with advances in high-throughput SNP genotyping across large breeding populations in various platforms (Fluidigm’s Dynamic ArraysTM, Douglas Scientific Array TapeTM, and LGC’s automated systems for running KASPTM markers) to accelerate rice improvement (Thomson, 2014). Likewise, genotyping by sequencing (GBS) is currently becoming a choice for low-cost high-density genome-wide scans using multiplexed sequencing.
At IRRI, in-house genotyping services for various breeding programs contribute to fast-tracking the breeding cycle from hybridization to population advancement in 2-3 years to generate elite lines. Structurally, IRRI breeding hubs facilitate multi-environment testing with strategically selected locations in South Asia (India), Southeast Asia (Myanmar and the Philippines), and East and Southern Africa (Burundi), in partnership with other partners from both the public and private sector. Through this transformed breeding process, we envision generating various combinations of effective Xa genes in similar or different elite backgrounds for market segmentation. The strategy will likely promote shuffling of resistance mechanisms displayed on the field to prevent rapid pathogen adaptation to single-gene virulence, deployed in a customized manner (Figure 1). Real-time deployment of resistant varieties with various combinations of effective Xa genes can be customized through gene rotation or mixture in a single genetic background targeted for evolving or dynamic pathogen populations, especially in a BB-endemic rice-growing environment. In a broader context, breeding programs are allowed to prioritize genes that are effective across multiple locations, but also genes that combine different mechanisms and show low turnover rate.
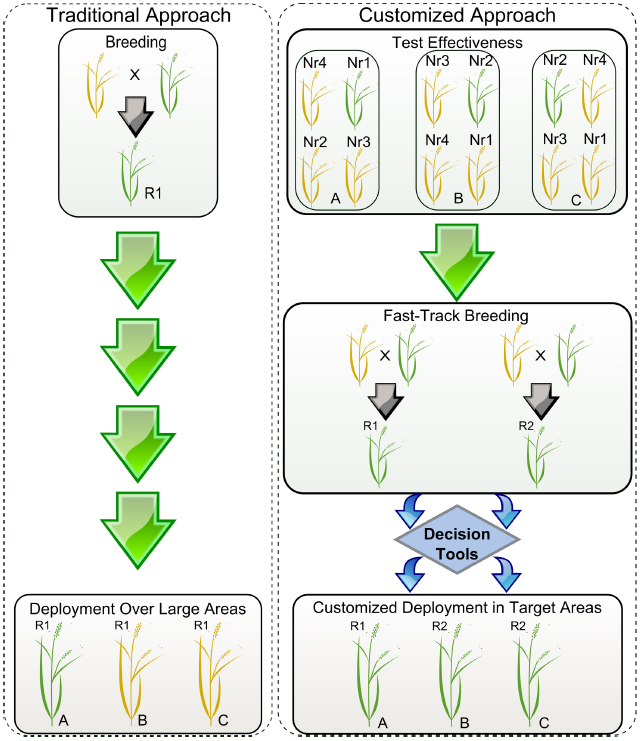
Figure 1. Simplified scheme showing the deployment of resistant varieties using traditional versus customized approaches. R1 and R2 represent resistant elite varieties carrying hypothetical genes 1 and 2. Yellow and green plants represent susceptible and resistant phenotypes, respectively. Locations A, B, and C represent cropping regions that do not share boundaries. Nr1, Nr2, Nr3, and Nr4 are near-isogenic lines (NILs) for each of the available resistance genes 1, 2, 3, and 4. During traditional deployment, variety R1 is bred and released in large areas but is effective only in particular locations. During customized deployment, the effectiveness of the resistance genes and pathogen population structures are monitored using disease hotspots, seasonal collections, and pathogenicity tests done in a confined setting. Using a decision tool, breeding programs can rapidly customize the elite varieties to be deployed in targeted locations based on variety profiles.
Monitoring of Effective Genes
In order to customize deployment, we need to assess the effectiveness of R-genes in specific target areas and understand the evolutionary potential of the local Xoo population. Seasonal monitoring, when timely executed and concerted in a cost-effective manner, could be very useful for breeders to direct breeding efforts (Figure 2). The first aspect of monitoring entails a collection of field strains to understand population genetic structure and local distribution. So far, all markers based on restriction fragment length polymorphism profiling or repeated sequences (Choi et al., 1998; Chen et al., 2012; Mishra et al., 2013) have not succeeded in describing functional groups or simply are not currently available in high-throughput platforms. However, many large-scale shotgun sequencing projects involving short-reads are underway and would potentially allow us to identify a set of SNP markers for standard use in field genotyping across different regions. Ultimately, the hope is that current advances in long-reads sequencing chemistry will resolve TAL effector sequences within complex samples, thus opening the door for more informative monitoring initiatives. The second aspect may involve the use of near-isogenic lines (NILs) carrying updated sets of Xa genes. Traditionally, this material has been used as a tool to identify phenotypic groups under controlled conditions (Ogawa et al., 1988). NILs are also suitable for deployment in disease-endemic areas or “hotspots” to capture low-prevalence genotypes that might escape from seasonal collection or to assess the effectiveness of Xa genes at a local scale (Figure 1). Ideally, both aspects could provide regional breeders with real-time decision support for small-scale interventions. Since 2013, IRRI has been deploying NILs in target areas of Asia and Africa and coordinating efforts to collect Xoo samples with local partners. Lessons learned from engaging rice research programs suggest that monitoring must be a participatory exercise and information exchange between national partners is more likely to occur under a common platform.
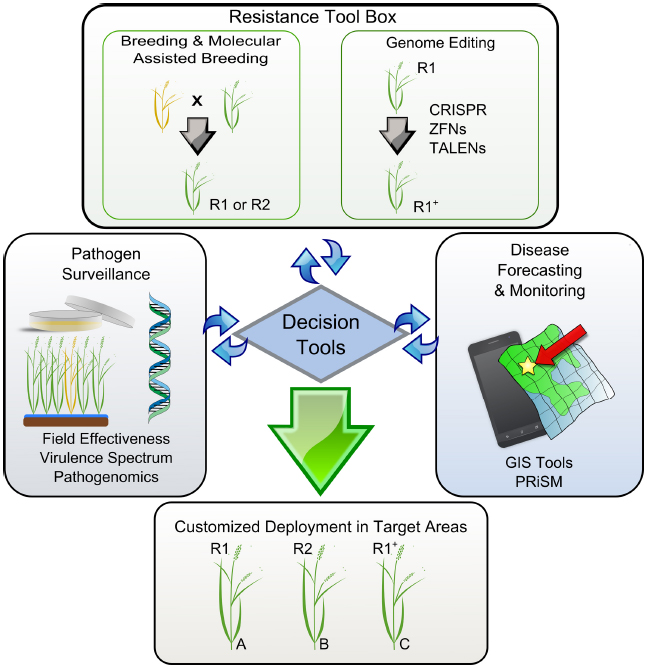
Figure 2. A model representing key elements supporting the decision tools for customized deployment of resistance genes in rice. Disease-prone areas are predicted and geo-referenced with other environmental constraints using GIS. Pathogen surveillance and the effectiveness of R-genes can be adapted to a seasonal base and used by breeding programs to timely direct breeding efforts. R1 and R2 represent resistant elite varieties carrying hypothetical genes 1 and 2. Yellow and green plants represent susceptible and resistant phenotypes, respectively. Locations A, B, and C represent cropping regions that do not share boundaries. A resistance tool kit provides adequate technologies that allow fast-tracking the response to particular needs. For instance, R1+ represents an elite variety with an artificially expanded spectrum of recognition that can be deployed in additional areas. All elements are gathered and interconnected through a unique platform (decision tool) for customized deployment in targeted areas.
Exploiting Effectors to Drive Deployment
Scientists defined effectors as molecular instruments that facilitate a parasitic life style (Hogenhout et al., 2009). Translational research done in a number of crops has tailored effector biology into a useful tool for disease resistance breeding (Gawehns et al., 2013; Vleeshouwers and Oliver, 2014). In oomycetes and fungal pathosystems, effectors have been used to facilitate cloning R-genes, discovering novel specificities, or avoiding unnecessary breeding effort (Vleeshouwers et al., 2011; Oliver et al., 2012; Rietman et al., 2012). Recent advances in the biochemical function of bacteria TAL effectors suggest that their activity has a major impact on virulence (Kay and Bonas, 2009). TAL effectors activate the expression of specific host susceptibility genes (S) in order to create a favorable environment. For instance, increasing sucrose availability or reducing copper-mediated toxicity within the xylem vessels appears to be a clear output of its virulence function during rice-Xoo interaction (Chen et al., 2010; Yuan et al., 2010). With the TAL-DNA recognition decoded (Boch et al., 2009; Moscou and Bogdanove, 2009), it is now possible to have a catalog of validated TAL targets in the rice genome (Noël et al., 2013). The increasing evidence that TAL repertoires can determine host specificity in a gene-for-gene fashion (Yang and White, 2004; Gu et al., 2005; Yang et al., 2006; Antony et al., 2010; Tian et al., 2014; Wang et al., 2014) highlights their potential use in translational research. For instance, Xoo strains carrying TAL effectors Avrxa10, AvrXa23, and AvrXa27 are unable to colonize rice accession containing Xa10, Xa23 and Xa27, respectively (Gu et al., 2009; Tian et al., 2014; Wang et al., 2014).
Theoretically, the allelic diversity of TAL effectors in a region can be used to help deployment interventions, but in practice we need to overcome some technical issues: (i) What is the best way to efficiently capture TAL repertoires (TALome)? Probably, a combination of TAL enrichment methods and high-throughput sequencing using long-reads will be enough to map the TALome, although cost-efficiency remains a major limitation. (ii) How do we improve current algorithms to efficiently catalog TAL targets? While many candidate S-genes have been validated experimentally, we expect an enhanced accuracy of prediction tools (Doyle et al., 2012; Grau et al., 2013; Pérez-Quintero et al., 2013) as more Xoo genomes become available and the algorithms can be trained on natural alleles of candidate targets using enlarged rice data sets (Li et al., 2014). (iii) Is it worthwhile assessing epiallelic variation of TAL effectors among field isolates? So far, no evidence suggests that Xoo actively uses this pathway to adapt to the selection pressure imposed by agricultural deployment. For now, it does not seem likely that a diagnostic test will be included in regular surveys because detecting gene expression in field samples can be technically challenging and economically not feasible (Gijzen et al., 2014). In summary, we cannot afford to exclude effectors-based information from a modern rice breeding and deployment program, but addressing these questions is essential for fine tuning the overall strategy. Beside effectors biology, the study of cell to cell signaling pathways or virulence regulation in response to abiotic factors are some of the emerging areas of research that will need some attention in the near future.
Mapping Disease in Real Time
The technologies currently exist to merge all of these concepts into a working system for targeted deployment. For example, the Philippine Rice Information System (PRiSM1) gathers data from farmers’ fields throughout the Philippines. Trained individuals use a standardized survey portfolio based on the IRRI publication “A Survey Portfolio to Characterize Yield-Reducing Factors in Rice” (Savary and Castilla, 2009), collecting data on yield-reducing (biotic stresses) and also yield-limiting (abiotic stresses) factors, current yields, and farmers’ agronomic practices using smartphones. The data are submitted via a wireless connection (Wi-Fi or cellular) to a centralized database in near real time. These data allow us to create a profile of a local targeted environment for breeders to reference by linking with other sources of information. The profile could include the most common pathogen population structure (collected in the fields being surveyed), farmers’ market preferences, farmers’ agronomic practices, and biotic and abiotic stresses in the target area. For example, using this technology, breeders working to develop a variety for an area where flooding is common could see where BB and flooding are most likely to occur together. Then, these data could be coupled with the local Xoo population structure and local market preferences to develop a variety that farmers would be likely to adopt and that is tailored to that specific environment’s stresses (Figure 2).
Broadening the Spectrum of Recognition, Only if Necessary
Genome editing techniques enable targeting specific DNA sequences and introducing a broad range of precise genetic modifications (Fichtner et al., 2014). Beside the current direction of the regulatory debate, the outcome of this technology is not a GMO product because it does not contain any foreign DNA (Waltz, 2012). While most of the available genome editing tools (ZFNs, TALENs, CRISPR-Cas9) have been successfully tested in rice (Li et al., 2012; Miao et al., 2013; Zhang et al., 2014b; Zhou et al., 2014), current progress on virulence mechanisms promoted by TAL effectors has inspired new ways to immunize crops using such an approach. For instance, resistance can be acquired by disrupting the TAL effector binding site of major S-gene promoters, such as members of the SWEET sucrose-efflux transporter family (Li et al., 2012). Eventually, broad-spectrum resistance can be created if several family members are targeted at the same time, thus limiting the access of the bacteria to alternative nutrient resources. Resistance can also be engineered using multiple decoy TAL-binding sites fused upstream of a single executor R-gene (Römer et al., 2009). Among all the rice R-genes that have been reported (Gu et al., 2005; Tian et al., 2014; Wang et al., 2014), few have potential applications as executors because they trigger strong localized cell-death and are induced only in the presence of the pathogen. We predict that genome editing tools will be integrated into the next-generation resistance tool kit, but might be considered only when no other alternative is available (Figure 2). For instance, elite varieties with an artificially expanded spectrum of recognition (either R or S) may become a solution in regions where management practices or pyramiding of existing Xa genes are no longer options. Swarna-Sub1 is a high-yielding mega-variety that shows a yield advantage in flood-prone areas of Asia (Ismail et al., 2013) but it is quite susceptible to Xoo infection in some of these unfavorable environments. Current attempts to precisely fast-track effective combinations of Xa genes into Swarna-Sub1 are under way, but the number of effective Xa genes available is limited and alternative strategies to increase the diversity of mechanisms are key for sustainable deployment-based management (Figure 2). Whether we are planning to exploit R- or S-genes to broaden the spectrum of resistance, it is clear that genome editing tools will be important assets for next-generation resistance breeding.
One Tool to Rule Them All
It is only a matter of time before information and communication technologies (ICTs) lead the research revolution on the agricultural landscape. Currently, very precise information can be retrieved and/or delivered to and from farmers’ hands in real time. Platforms that incorporate crop health status under well-characterized environments are coming and will soon become tools for informed interventions. Therefore, it becomes very important that pathologists, breeders, and epidemiologists endeavor to integrate diagnostics, disease models, and breeding efforts into a unique platform for customized deployment (Figure 2). This vision does not exclude other fields of research that also contribute to increased variety adoption and are important in the rice value chain. These are exciting times, like never before, rice scientists have the possibility to adapt their breeding programs and decide which variety will be promoted next season to reduce the chance of future epidemics.
Conflict of Interest Statement
The authors declare that the research was conducted in the absence of any commercial or financial relationships that could be construed as a potential conflict of interest.
Acknowledgments
Scientists at the International Rice Research Institute (IRRI) are partially funded by the Global Rice Science Partnership (GRiSP), and projects under the Stress-tolerant rice for poor farmers in Africa and South Asia (STRASA) supported by the Bill & Melinda Gates Foundation (BMGF). The Philippine Rice Information System (PRiSM) is a collaborative project between Department of Agriculture (DA)-Philippine Rice Research Institute (PhilRice), DA-Bureau of Plant Industry (BPI), DA-Regional Field Offices (RFOs), and IRRI, in support to DA’s Food Staples Sufficiency Program, with funding support from the DA-National Rice Program through the DA-Bureau of Agricultural Research. We want to thank Hei Leung and Michael Thomson for reviewing the manuscript.
Footnotes
References
Antony, G., Zhou, J., Huang, S., Li, T., Liu, B., White, F. F., et al. (2010). Rice xa13 recessive resistance to bacterial blight is defeated by induction of the disease susceptibility gene Os-11N3. Plant Cell 22, 3864–3876. doi: 10.1105/tpc.110.078964
PubMed Abstract | Full Text | CrossRef Full Text | Google Scholar
Boch, J., Bonas, U., and Lahaye, T. (2014). TAL effectors-pathogen strategies and plant resistance engineering. New Phytol. 204, 823–832. doi: 10.1111/nph.13015
PubMed Abstract | Full Text | CrossRef Full Text | Google Scholar
Boch, J., Scholze, H., Schornack, S., Landgraf, A., Hahn, S., Kay, S., et al. (2009). Breaking the code of DNA binding specificity of TAL-type III effectors. Science 326, 1509–1512. doi: 10.1126/science.1178811
PubMed Abstract | Full Text | CrossRef Full Text | Google Scholar
Chen, L. Q., Hou, B. H., Lalonde, S., Takanaga, H., Hartung, M. L., Qu, X. Q., et al. (2010). Sugar transporters for intercellular exchange and nutrition of pathogens. Nature 468, 527–532. doi: 10.1038/nature09606
PubMed Abstract | Full Text | CrossRef Full Text | Google Scholar
Chen, X. L., Yu, L., Gao, L. L., Jiang, T., Li, Q. Y., and Huang, Q. (2012). Elevational variation in diversity of Xanthomonas oryzae pv. oryzae in South-West China. J. Phytopathol. 160, 261–268. doi: 10.1111/j.1439-0434.2012.01892.x
Choi, S. H., Vera Cruz, C. M., and Leach, J. E. (1998). Distribution of Xanthomonas oryzae pv. oryzae DNA modification systems in Asia. Appl. Environ. Microbiol. 64, 1663–1668.
Doyle, E. L., Booher, N. J., Standage, D. S., Voytas, D. F., Brendel, V. P., Van Dyk, J. K., et al. (2012). TAL Effector-Nucleotide Targeter (TALE-NT) 2.0: tools for TAL effector design and target prediction. Nucleic Acids Res. 40, 117–122. doi: 10.1093/nar/gks608
PubMed Abstract | Full Text | CrossRef Full Text | Google Scholar
Fichtner, F., Castellanos, R. U., and Ülker, B. (2014). Precision genetic modifications: a new era in molecular biology and crop improvement. Planta 239, 921–939. doi: 10.1007/s00425-014-2029-y
PubMed Abstract | Full Text | CrossRef Full Text | Google Scholar
Gawehns, F., Cornelissen, B. J. C., and Takken, F. L. W. (2013). The potential of effector-target genes in breeding for plant innate immunity. Microb. Biotechnol. 6, 223–229. doi: 10.1111/1751-7915.12023
PubMed Abstract | Full Text | CrossRef Full Text | Google Scholar
Gijzen, M., Ishmael, C., and Shrestha, S. D. (2014). Epigenetic control of effectors in plant pathogens. Front. Plant Sci. 5:638. doi: 10.3389/fpls.2014.00638
PubMed Abstract | Full Text | CrossRef Full Text | Google Scholar
Grau, J., Wolf, A., Reschke, M., Bonas, U., Posch, S., and Boch, J. (2013). Computational predictions provide insights into the biology of TAL effector target sites. PLoS Comput. Biol. 9:e1002962. doi: 10.1371/journal.pcbi.1002962
PubMed Abstract | Full Text | CrossRef Full Text | Google Scholar
Gu, K. Y., Yang, B., Tian, D. S., Wu, L. F., Wang, D. J., Sreekala, C., et al. (2005). R gene expression induced by a type-III effector triggers disease resistance in rice. Nature 435, 1122–1125. doi: 10.1038/nature03630
PubMed Abstract | Full Text | CrossRef Full Text | Google Scholar
Gu, K., Tian, D., Qiu, C., and Yin, Z. (2009). Transcription activator-like type III effector AvrXa27 depends on OsTFIIAgamma5 for the activation of Xa27 transcription in rice that triggers disease resistance to Xanthomonas oryzae pv.oryzae. Mol. Plant Pathol. 10, 829–835. doi: 10.1111/j.1364-3703.2009.00567.x
PubMed Abstract | Full Text | CrossRef Full Text | Google Scholar
Hogenhout, S. A., van der Hoorn, R. A. L., Terauchi, R., and Kamoun, S. (2009). Emerging concepts in effector biology of plant-associated organisms. Mol. Plant Microbe Interact. 22, 115–122. doi: 10.1094/MPMI-22-2-0115
PubMed Abstract | Full Text | CrossRef Full Text | Google Scholar
Ismail, A. M., Singh, U. S., Singh, S., Dar, M. H., and Mackill, D. J. (2013). The contribution of submergence tolerant (Sub1) rice variety to food security in flood-prone rainfed areas in Asia. Field Crops Res. 152, 83–93. doi: 10.1016/j.fcr.2013.01.007
Kay, S., and Bonas, U. (2009). How Xanthomonas type III effectors manipulate the host plant. Curr. Opin. Microbiol. 12, 37–43. doi: 10.1016/j.mib.2008.12.006
PubMed Abstract | Full Text | CrossRef Full Text | Google Scholar
Khan, M. A., Naeem, M., and Iqbal, M. (2014). Breeding approaches for bacterial leaf blight resistance in rice (Oryza sativa L.), current status and future directions. Eur. J. Plant Pathol. 139, 27–37. doi: 10.1007/s10658-014-0377-x
Li, J. Y., Wang, J., and Zeigler, R. S. (2014). The 3,000 rice genomes project: new opportunities and challenges for future rice research. GigaScience 3:8. doi: 10.1186/2047-217X-3-8
PubMed Abstract | Full Text | CrossRef Full Text | Google Scholar
Li, T., Liu, B., Spalding, M. H., Weeks, D. P., and Yang, B. (2012). High-efficiency TALEN-based gene editing produces disease-resistant rice. Nat. Biotechnol. 30, 390–392. doi: 10.1038/nbt.2199
PubMed Abstract | Full Text | CrossRef Full Text | Google Scholar
Miao, J., Guo, D., Zhang, J., Huang, Q., Qin, G., Zhang, X., et al. (2013). Targeted mutagenesis in rice using CRISPR-Cas system. Cell Res. 23, 1233–1236. doi: 10.1038/cr.2013.123
PubMed Abstract | Full Text | CrossRef Full Text | Google Scholar
Mishra, D., Vishnupriya, M. R., Anil, M. G., Konda, K., Raj, Y., and Sonti, R. V. (2013). Pathotype and genetic diversity amongst Indian isolates of Xanthomonas oryzae pv. oryzae. PLoS ONE 8:e81996. doi: 10.1371/journal.pone.0081996
PubMed Abstract | Full Text | CrossRef Full Text | Google Scholar
Moscou, M. J., and Bogdanove, A. J. (2009). A simple cipher governs DNA recognition by TAL effectors. Science 326, 1501. doi: 10.1126/science.1178817
Noël, L. D., Denancé, N., and Szurek, B. (2013). Predicting promoters targeted by TAL effectors in plant genomes: from dream to reality. Front. Plant Sci. 4:333. doi: 10.3389/fpls.2013.00333
PubMed Abstract | Full Text | CrossRef Full Text | Google Scholar
Ogawa, T., Yamamoto, T., Khush, G. S., Mew, T. W., and Kaku, H. (1988). Near-isogenic lines as international differentials for resistance to bacterial blight of rice. RGN 5, 106–107.
Oliver, R. P., Friesen, T. L., Faris, J. D., and Solomon, P. S. (2012). Stagonospora nodorum: from pathology to genomics and host resistance. Annu. Rev. Phytopathol. 50, 23–43. doi: 10.1146/annurev-phyto-081211-173019
PubMed Abstract | Full Text | CrossRef Full Text | Google Scholar
Pérez-Quintero, A., Rodriguez, R. L., Dereeper, A., Lopez, C., Koebnik, R., Szurek, B., et al. (2013). An improved method for TAL effectors DNA-binding sites prediction reveals functional convergence in TAL repertoires of Xanthomonas oryzae strains. PLoS ONE 8:e68464. doi: 10.1371/journal.pone.0068464
PubMed Abstract | Full Text | CrossRef Full Text | Google Scholar
Rietman, H., Bijsterbosch, G., Cano, L. M., Lee, H. R., Vossen, J. H., Jacobsen, E., et al. (2012). Qualitative and quantitative late blight resistance in the potato cultivar Sarpo Mira is determined by the perception of five distinct RXLR effectors. Mol. Plant Microbe Interact. 25, 910–919. doi: 10.1094/MPMI-01-12-0010-R
PubMed Abstract | Full Text | CrossRef Full Text | Google Scholar
Römer, P., Recht, S., and Lahaye, T. (2009). A single plant resistance gene promoter engineered to recognize multiple TAL effectors from disparate pathogens. Proc. Natl. Acad. Sci. U.S.A. 106, 20526–20531. doi: 10.1073/pnas.0908812106
PubMed Abstract | Full Text | CrossRef Full Text | Google Scholar
Savary, S., and Castilla, N. P. (2009). A survey portfolio for the characterization of rice pest constraints. IRRI Discuss. Paper 18, 32.
Thomson, J. M. (2014). High-throughput SNP genotyping to accelerate crop improvement. Plant Breed. Biotechnol. 2, 195–212. doi: 10.9787/PBB.2014.2.3.195
Tian, D., Wang, J., Zeng, X., Gu, K., Qiu, C., Yang, X., et al. (2014). The rice TAL effector-dependent resistance protein Xa10 triggers cell death and calcium depletion in the endoplasmic reticulum. Plant Cell 26, 497–515. doi: 10.1105/tpc.113.119255
PubMed Abstract | Full Text | CrossRef Full Text | Google Scholar
Vleeshouwers, V. G., and Oliver, R. P. (2014). Effectors as tools in disease resistance breeding against biotrophic, hemibiotrophic, and necrotrophic plant pathogens. Mol. Plant Microbe Interact. 27, 196–206. doi: 10.1094/MPMI-10-13-0313-IA
PubMed Abstract | Full Text | CrossRef Full Text | Google Scholar
Vleeshouwers, V. G., Raffaele, S., Vossen, J., Champouret, N., Oliva, R., Segretin, M. E., et al. (2011). Understanding and exploiting late blight resistance in the age of effectors. Annu. Rev. Phytopathol. 49, 507–531. doi: 10.1146/annurev-phyto-072910-095326
PubMed Abstract | Full Text | CrossRef Full Text | Google Scholar
Waltz, E. (2012). Tiptoeing around transgenics. Nat. Biotechnol. 30, 215–217. doi: 10.1038/nbt.2143
PubMed Abstract | Full Text | CrossRef Full Text | Google Scholar
Wang, C., Zhang, X., Fan, Y., Gao, Y., Zhu, Q., Zheng, C., et al. (2014). Xa23 is an executor R protein and confers broad-spectrum disease resistance in rice. Mol. Plant 8, 290–302. doi: 10.1016/j.molp.2014.10.010
PubMed Abstract | Full Text | CrossRef Full Text | Google Scholar
Yang, B., Sugio, A., and White, F. F. (2006). Os8N3 is a host disease susceptibility gene for bacterial blight of rice. Proc. Natl. Acad. Sci. U.S.A. 103, 10503–10508. doi: 10.1073/pnas.0604088103
PubMed Abstract | Full Text | CrossRef Full Text | Google Scholar
Yang, B., and White, F. F. (2004). Diverse members of the AvrBs3/ PthA family of type III effectors are major virulence determinants in bacterial blight disease of rice. Mol. Plant Microbe Interact. 17, 1192–1200. doi: 10.1094/MPMI.2004.17.11.1192
PubMed Abstract | Full Text | CrossRef Full Text | Google Scholar
Yuan, M., Chu, Z., Li, X., Xu, C., and Wang, S. (2010). The bacterial pathogen Xanthomonas oryzae overcomes rice defenses by regulating host copper redistribution. Plant Cell 22, 3164–3176. doi: 10.1105/tpc.110.078022
PubMed Abstract | Full Text | CrossRef Full Text | Google Scholar
Zhang, F., Zhuo, D. L., Zhang, F., Huang, L. Y., Wang, W. S., Xu, J. L., et al. (2014a). Xa39, a novel dominant gene conferring broad-spectrum resistance to Xanthomonas oryzae pv. oryzae in rice. Plant Pathol. doi: 10.1111/ppa.12283 [Epub ahead of print].
Zhang, H., Zhang, J., Wei, P., Zhang, B., Gou, F., Feng, Z., et al. (2014b). The CRISPR/Cas9 system produces specific and homozygous targeted gene editing in rice in one generation. Plant Biotechnol. J. 12, 797–807. doi: 10.1111/pbi.12200
PubMed Abstract | Full Text | CrossRef Full Text | Google Scholar
Zhou, H., Liu, B., Weeks, D. P., Spalding, M. H., and Yang, B. (2014). Large chromosomal deletions and heritable small genetic changes induced by CRISPR/Cas9 in rice. Nucleic Acids Res. 42, 10903–10914. doi: 10.1093/nar/gku806
PubMed Abstract | Full Text | CrossRef Full Text | Google Scholar
Keywords: customized deployment, forward breeding, TAL effectors, R-genes, genome editing
Citation: Dossa GS, Sparks A, Vera Cruz C and Oliva R (2015) Decision tools for bacterial blight resistance gene deployment in rice-based agricultural ecosystems. Front. Plant Sci. 6:305. doi: 10.3389/fpls.2015.00305
Received: 26 February 2015; Accepted: 16 April 2015;
Published: 05 May 2015.
Edited by:
Laurent D. Noël, Centre National de la Recherche Scientifique, FranceReviewed by:
Tina B. Jordan, Eberhard Karls University Tübingen, GermanyWei Qian, Chinese Academy of Sciences, China
Copyright © 2015 Dossa, Sparks, Vera Cruz and Oliva. This is an open-access article distributed under the terms of the Creative Commons Attribution License (CC BY). The use, distribution or reproduction in other forums is permitted, provided the original author(s) or licensor are credited and that the original publication in this journal is cited, in accordance with accepted academic practice. No use, distribution or reproduction is permitted which does not comply with these terms.
*Correspondence: Ricardo Oliva, Plant Breeding, Genetics, and Biotechnology Division, International Rice Research Institute, DAPO Box 7777, Metro Manila 1301, Philippines, r.oliva@irri.org