- Plant Breeding and Biotechnology Laboratory, Department of Botany, University of Dhaka, Dhaka, Bangladesh
In a world where climate change is real and its consequences are unprecedented, understanding of the plant adaptive capacity and native stress-responsive machinery is crucial. In recent years, universal stress proteins (USPs) have received much attention in the field of plant science due to their stress-specific transcriptional regulation. This study focuses on the extensive characterization of the USP gene family members in the monocot crop rice (Oryza sativa L. var. japonica). Here, we report a total of 44 USP genes in the rice genome. In silico characterization of these genes showed that domain architecture played a major role in the functional diversification of the USP gene family which holds for all plant USPs. On top of that, a higher conservation of OsUSP members has been exhibited with a monocot genome (Zea mays L.) as compared to a dicot genome (Arabidopsis thaliana L.). Expression profiling of the identified genes led to the discovery of multiple OsUSP genes that showed pronounced transcript alteration under various abiotic stress conditions, indicating their potential role as multi-functional stress-specific modules. Furthermore, expression validation of OsUSP genes using qRT-PCR provided a strong evidence for the utility OsUSP genes in building multi-stress tolerant plants. Altogether, this study provides leads to suitable USP candidates that could be targeted for plant breeding and genetic engineering experiments to develop stress resilient crop species.
Introduction
Alteration of the growth environment from optimum to adverse conditions is a common occurrence in a plant’s life cycle. Being sessile, plants must exhibit a dynamic response to cope with subtle to drastic changes in their nearby regimes. Such changes can range from diverse ecological parameters such as temperature, water and nutrient availability, salt content, etc. to intrinsic factors such as accumulation of reactive oxygen species (ROS). Under such unfavorable conditions, plants can suffer numerous repercussions, e.g., arrested growth and development, lower photosynthetic capacity, abnormalities in flowering time, reduced fertility and germination rate, abated total yield, etc. (Munns, 2002; Barnabás et al., 2008). To overcome this, plants have evolved highly complex yet coordinated responses through temporal and spatial regulation of genes that can mitigate unwanted effects resulting from various abiotic stressors. Generally, perception of stressors triggers many downstream signaling cascades within plant cells, such as protein kinases, phosphatases, transcription factors, molecular chaperones and defense-related proteins that mediate a suitable response to ensure plant survival (Jung et al., 2015). However, in a world where climate change is experiencing a rapid spike, plants native stress-responsive machinery is not able to protect the cellular system from stress inflicted damages (Lesk et al., 2016). These circumstances have accelerated the search for stress-induced genetic components that hold the capacity to endow plants with enhanced stress resilience through modern biotechnological tools. In this regard, the most suitable candidates include those genes that can mediate protection against multiple stressors simultaneously. One such group of proteins that have recently come into the spotlight for their stress-specific roles in plants, are the universal stress protein (USP) family (Chi et al., 2019).
The first USP was reported in E. coli where the candidate protein responded to a multitude of environmental perturbations including nutrient starvation, temperature shock, oxidative and osmotic stress, heavy metal toxicity, antibiotics, etc. (Nyström and Neidhardt, 1992; Zarembinski et al., 1998; Sousa and McKay, 2001). Later, USPs have been reported in many other organisms including other bacteria, archaea, fungi, and even plants (Vollmer and Bark, 2018). Although E. coli USPs by structure only contain the USP-domain, plant USPs have largely diversified in function by accumulating additional accessory domains that allow them to participate in numerous varying functions (Chi et al., 2019). Consequently, the multi-functional nature of USPs is derived from their structural diversity. Information of the evolution and function of plant USPs is very limited to date. A few genes have been studied in rice, Arabidopsis, cotton, etc. which were found to play a key role in stress regulation (Sauter, 2002; Merkouropoulos and Tsaftaris, 2013; Jung et al., 2015). Nevertheless, these studies strongly hint to the importance of plant USPs for further studies. OsUSP1 from rice was the first USP gene identified in the plant kingdom that has been involved in the activation of signaling cascade in response to ethylene during hypoxia (Sauter, 2002). It was reported that stomatal closure is enhanced by SpUSP during drought stress to limit the detriment in tomatoes (Loukehaich et al., 2012). Two Arabidopsis thaliana USP isoforms (At3g62550 and At3g53990) were reported to show enhanced expression under drought (Shinozaki and Yamaguchi-Shinozaki, 2007). Recent studies have also highlighted the role of USPs in conferring benefits during other abiotic stresses (Udawat et al., 2016). For instance, the AtUSP (At3g53390) gene is significantly induced by salt, osmotic stress, and wounding (Isokpehi et al., 2011). Overexpressing AtUSP (At3g53990) conferred tolerance against oxidative and heat stresses whereas sensitivity to these stressors was observed in atusp (SALK_146059) mutant lines (Jung et al., 2015). AtUSP was also found to be involved in cold stress (Melencion et al., 2017). Apart from that, USP regulates ethylene-mediated signaling and thereby modulates fruit ripening (Isokpehi et al., 2011). A tomato USP protein, SlRd2 interacts with calcineurin B-like interacting protein kinase (SlCipk6) and is involved in the regulation of ROS production (Gutiérrez-Beltrán et al., 2017). In cotton, two closely related genes GhUSP1 and GhUSP2, within the USP family were reported to be drought-responsive (Maqbool et al., 2009). Moreover, AtUSP promoter has shown to upregulate GUS expression in response to abscisic acid (ABA), 1-aminocyclopropane-1-carboxylic acid (ACC), dehydration, heat, cold, salt, and osmotic stress (Bhuria et al., 2016). This is a clear indication of the functional capacity of plant USPs under multi-stress conditions. In line with such importance, forty-one USP genes were identified in the model plant Arabidopsis thaliana (Bhuria et al., 2019). Out of these, AtUSP9 and AtUSP12 were identified as multiple stress-responsive.
Rice is an important crop and an ideal model for the comparative study of gene functions owing to high efficiency of genetic transformation and ease of cultivation and propagation (Basso et al., 2020). With the release of the whole genome sequence of rice (Song et al., 2019), it is very convenient to comprehensively analyze an entire gene family. Recently, 38 USP genes were reported in rice (Japonica variety) (Chi et al., 2019). However, a detailed analysis of the functional annotation and mode of regulation of these genes is still lacking. Due to the multi-stress responsive nature of plant USPs, it is imperative to look for the suitable candidates that could be targeted for improving crop resilience under multiple environmental adversities. Here, considering the important role of USP proteins in plant stress regulation and the lack of information about this gene family in a crop species, our study aimed to screen the USP gene family in rice. This study provides an in-depth bioinformatics characterization of the identified USP genes along with their developmental and stress-specific transcriptional regulation. Overall, this study provides suitable USP genes for future biotechnological applications aiming to generate of multi-stress tolerant agricultural crops and serves as a framework for investigating the USP gene family in other important plant species.
Materials and Methods
Identification, Gene Nomenclature and in silico Analysis of USP Genes in Rice
To identify the USP genes in rice, blastp and Hidden Markov Model (HMM) search had been done. Rice [Oryza sativa Japonica Group (Japanese rice) cv. Nipponbare] USP protein sequences were retrieved from the publicly available genome database in the Rice Annotation Project Database (RAP-DB1) (Sakai et al., 2013) using Arabidopsis USP (At1g68300) as a query sequence. Subsequently, each of the newly identified sequences were used as a query sequence to find the other new members. To confirm the result from HMM and blastp search, the putative USP sequences were also analyzed against the Pfam database2 (Mistry et al., 2020). Nomenclature of rice USPs was given according to the chromosomal location in descending order (Hasan et al., 2021). Information on the gene locus ID, protein size and full-length cDNA, the molecular weight, theoretical pI, the number of amino acids were obtained from the RAP-DB database. The subcellular localization of the proteins was predicted using CELLO v.2.5: subCELlular LOcalization predictor3 (Yu et al., 2006) and WoLF pSORT4 software (Horton et al., 2007). ChloroP5 (Emanuelsson et al., 1999) was used to verify chloroplast localization.
Exon–Intron Distribution of OsUSP Genes, Identification of Conserved Motifs and Amino Acid Content in OsUSP Proteins
The GFF3 annotation file for the rice genome was downloaded from the Ensembl Plants6 (Howe et al., 2020). Information regarding the position of exon–introns and UTR regions of rice USP genes were extracted from this file. For the identification of conserved motifs, MEME SUITE software (v.5.3.3) was used (Bailey et al., 2009) with all parameters were kept in default settings except the number of motifs to find was set to 10. The “Gene Structure View” option of TBtools (v.1.092) (Chen et al., 2020) was used to visualize the exon–intron distribution and conserved motifs of rice USP members. Both the modified GFF3 file and MEME SUITE XML output file was used for this task. Amino acid content was calculated using the Biopython (v.1.78) package.
Chromosomal Localization of OsUSP Genes and Their Synteny Analysis With Arabidopsis and Maize Genome
Chromosomal distribution of each OsUSP gene was retrieved from the Oryzabase database for rice7. For the duplication study within the rice genome, data including the synonymous rate (Ks) and non-synonymous rate (Ka) values were retrieved from the plant genome duplication database8 (Lee et al., 2012). Duplication type and selection pressure was calculated according to Hasan et al. (2021). The estimated date (Mya, million years ago) of each duplication event was calculated by using T = Ks/2λ where T is divergence time, Ks is the number of synonymous substitutions per site, and λ is the fixed substitution rate of 6.5 × 10–9 mutations per synonymous site per year in grasses (Gaut et al., 1996). Syntenic relationships between rice, Arabidopsis and maize USP genes were constructed and visualized using MCScanX (Wang et al., 2012) and TBtools (v.1.092) (Chen et al., 2020), respectively. In TBtools, the “Advanced Circos” option was used for visualizing the syntenic blocks.
Phylogenetic Relationship Between Rice, Arabidopsis, and E. coli USP Proteins Along With Their Domain Architecture
To get an idea about the evolutionary history of the rice USP gene family, we performed a phylogenetic analysis using the USP protein sequences of rice, Arabidopsis, and E. coli. Arabidopsis and E. coli USP protein sequences were retrieved from TAIR (Berardini et al., 2015) and UniProt (UniProt Consortium, 2021) databases, respectively. The protein sequences were aligned using the online version of MAFFT tool v.7 (Katoh et al., 2019) with default parameters. Next, gaps in the aligned regions were trimmed using the Phyutility tool (v.2.7.1) (Smith and Dunn, 2008) to retain 75% of the consensus alignment. With the trimmed multiple sequence alignment file, a phylogenetic tree was constructed using IQ-Tree v.2.1.2 (Minh et al., 2020) with 1000 ultrafast bootstrap replicates. Finally, the tree was visualized and edited using iTOL (v.6) (Letunic and Bork, 2019). The domain features were uploaded as an additional dataset in the required format (details on the iTOL help page). Domain information of each USP protein was downloaded from Pfam (Finn et al., 2014).
Identification of Microsatellite Markers, Glycosylation and Phosphorylation Sites in OsUSPs
For the identification of SSR markers in OsUSP members, a microsatellite identification tool (MISA9) (Beier et al., 2017) was used. The minimum repeat unit was defined as 10 units for mononucleotide repeats and 5 units for dinucleotide, trinucleotide, tetranucleotide, pentanucleotide, and hexanucleotide repeats. The maximum length of sequence between two markers was set to 100 units. The number of glycosylation sites in OsUSP proteins was predicted using NetNGlyc 1.0 server10 (Gupta and Brunak, 2002) with a threshold of 0.5. The predicted phosphorylation sites of all OsUSP members were identified using NetPhos3.111 (Blom et al., 1999, 2004) with the threshold of 0.75. Predictions were performed with all three residues (Tyr, Thr, Ser) for both generic and kinase-specific phosphorylation.
Expression Analysis of OsUSP Genes at Different Tissues, Developmental Stages and Under Stress Conditions Using Publicly Available mRNA-Seq Data
Temporal and spatial expression profiles of OsUSP genes were retrieved from Genevestigator12 (Hruz et al., 2008) for different developmental stages, anatomical tissues and in response to stress conditions (Supplementary Tables 1–3). Heatmaps were generated for each type of expression data using the “Heatmap Illustrator” option in TBtools v.1.092 (Chen et al., 2020). Heatmaps of both tissue and developmental expression patterns were created using the log10 transformed mean expression values. As for the expression under stress conditions, the log2 fold change in expression data was used to generate the Heatmap.
Analysis of Cis-Regulatory Elements and GO Enrichment of OsUSP Genes
To identify the cis-regulatory elements present in the putative promoter region, 1 kb upstream sequence of each OsUSP gene was used. Subsequently, the sequences were uploaded to the PlantCARE database13 (Lescot, 2002) to detect the presence of various cis-elements. Later, the presence of these cis-regulatory elements was visualized using a stacked bar plot. Gene Ontology enrichment analysis of OsUSP genes was performed with the help of agriGO database v.2 (Tian et al., 2017). The results were visualized in the R programming platform.
Plant Growth and Stress Treatments
For expression profiling, a uniformly developed rice seeds of BRRI-53 variety were placed in a greenhouse (16 h photoperiod, 28 ± 2°C temperature) (Islam et al., 2015). The 15 days old seedlings were then taken for various experimental treatment such as salt (200 mM), drought (mannitol; 150 mM), oxidative (30% H2O2), cold (4°C), heat (42°C), and dehydration (air-dried), ABA (10 mM), gibberellic acid (GA3, 1 mM), salicylic acid (SA, 2 mM) (Islam et al., 2015). The untreated seedlings were considered as a control to the treated seedlings. After 16 h treatment, leaves were collected (three biological replicates), immediately frozen in liquid nitrogen, and stored at −80°C.
RNA Isolation and RT-PCR Analysis
Rice seeds of BRRI-53 variety were grown in the controlled environmental condition of 28 ± 2°C temperature and 16 h photoperiod in a growth chamber. Fifteen days’ old seedlings were then used for various abiotic stress treatment such as salt (200 mM NaCl), drought (150 mM Mannitol), oxidative (30% H2O2), cold (4°C), heat (42°C), dehydration (air-dried) and hormonal treatment such as, ABA, GA3, and SA (Islam et al., 2015), for 16 h. The untreated seedlings were considered as a control to all these stresses. The tissues were harvested, and total plant RNA was isolated from rice shoots using TRIzol reagent (Invitrogen, United States) as per the manufacturer’s protocol (Supplementary Figure 1).
First-strand cDNA was synthesized with reverse transcriptase (Thermo Fisher Scientific, United States) by following the manufacturer’s protocol. A reaction mix (12 μl) was prepared in a PCR tube containing 10 μl of DNase treated RNA, 1 μl of DEPC-H2O and 1 μl oligo dT primer. The reaction mixture was incubated at 65°C for 5 min and chilled down on ice immediately for 5 min. Then, a master mix of 4 μl containing 5X reaction buffer, 1 μl of Riboblock RNAse inhibitor, 1 μl of Revertaid and 2 μl of 10 mM dNTPs mix was added in the tubes. The mixture was incubated at 42°C for 60 min. After the first strand synthesis, the reaction was terminated by heat inactivation at 70°C for 5 min. The expression of individual genes was measured with gene-specific primers by real-time PCR analysis with a cycler Applied biosystem 7500 and SYBR Green mixture (Bio-Rad, United States) (Supplementary Table 4). The relative expression of specific genes was quantitated with the 2-ΔΔCt calculation method (Livak and Schmittgen, 2001), where ΔΔCt was the difference in the threshold cycles and the reference gene, which was rice eEF1 for the expression analyses (Islam et al., 2015). The sequences of gene-specific primers are provided in Supplementary Table 5.
Statistical Analysis
Statistical analysis was performed for the relative normalized expression data from three biological replicates under each treatment (n = 3). The paired student’s t-test was performed for each treatment against the respective controls to determine the significance level that were marked with ∗, ∗∗, ∗∗∗ in case of P-value < 0.05, <0.01, and <0.001; respectively.
Homology Based Modeling and Molecular Docking Study
Three-dimensional structures of nine USPs were created using the MODELLER tool of MPI bioinformatics toolkit14 (Zimmermann et al., 2018; Gabler et al., 2020) based on the templates identified using the HHpred tool. Crystal Structure of the Usp protein of Mycobacteria (PDB-5AHW_F), hypothetical protein MJ0577 (PDB-1MJH_B), UspA from Lactobacillus plantarum (PDB-3S3F_A), USP from A. thaliana (PDB-3GM3_E), USP from Nitrosomonas (PDB-3TNJ_A), USP from Burkholderia pseudomallei (PDB-4WNY_A), Human Cdkl5 Kinase Domain (PDB-4BGQ_A), Pseudokinase MLKL from Mus musculus (PDB-4BTF_A) and hypothetical protein PH0823 (PDB-2DUM_B) were used as templates for OsUSP2, OsUSP3, OsUSP6, OsUSP14, OsUSP22, OsUSP32, OsUSP33 and OsUSP36, respectively. All these structures were visualized using BIOVIA Discovery Studio v.21.1.0.20298 (BIOVIA, 2021) (Supplementary Figure 2).
3D structure of the above nine OsUSP proteins was used as a receptor to find out the binding affinity with three well-established inhibitors of E. coli UspA – ZINC000104153710, ZINC000004268284, ZINC000000217308 (Bandyopadhyay et al., 2021). Four kinase inhibitors – fisetin, luteolin, myricetin, quercetin (Cassidy and Setzer, 2010) were used to check their binding affinity toward the kinase domain-containing OsUSPs. The 3-D chemical structure of these ligands was downloaded from the PubChem compound database15 (Kim et al., 2021) as an SDF file. The overall docking process was done using PyRx Virtual Screening software (Dallakyan and Olson, 2015) which uses Open Babel (O’Boyle et al., 2011) for importing SDF files, removing salts and energy minimization, uses AutoDock tools (Morris et al., 2009) for the preparation of protein and to generate input files and AutoDock Vina (Trott and Olson, 2010) as docking wizard. Grid box parameters were set to perform a blind docking (Hetényi and van der Spoel, 2002) of the inhibitors on the targeted proteins. The 2-D and 3-D interaction of the protein–ligands complex has been observed by using the BIOVIA Discovery Studio v.21.1.0.20298 (BIOVIA, 2021). Overall, a strategic framework of the present study has been summarized in Figure 1.
Results
Identification of USP Genes in Rice
Based on the blastp and HMM search, a total of 44 candidate USP genes were identified in rice (Oryza sativa L.). Relevant details about these genes are presented in Table 1. These identified USP genes were named from OsUSP1 to OsUSP44 in order of their chromosomal position. Among them, two splice variants were observed for genes OsUSP1, OsUSP2, OsUSP11, OsUSP16, OsUSP20, OsUSP22, OsUSP25, OsUSP27, OsUSP30, OsUSP31, and OsUSP35; whereas, OsUSP12 had three splice variants. The length of deduced complementary DNA sequence (CDS) of OsUSPs ranged from 222 bp (OsUSP31) to 2817 bp (OsUSP20) long. Altogether, the forty-four OsUSP genes encoded for a total of 57 proteins. The protein length ranged from 74 aa (OsUSP31) to 939 aa (OsUSP20). Meanwhile, the protein weight varied greatly from the lowest of 17.22 kDa (OsUSP13) to the highest of 120.81 kDa (OsUSP41), and the predicted isoelectric point range from 4.74 (OsUSP30) to 10.39 (OsUSP37). In silico subcellular localization prediction indicated that OsUSPs were localized in the cytoplasm, mitochondria, nucleus, and chloroplast (Table 1).
Analysis of OsUSP Gene Structure, Conserved Motifs and Amino Acid Content
To better understand the development of the OsUSP gene family, the exon–intron distribution of OsUSP genes was analyzed. In general, the number of exons and introns in OsUSP genes showed moderate variation (Figure 2A). In the case of introns, the numbers ranged from zero to ten, with OsUSP32 having the highest and OsUSP23 having the lowest number of introns (10 and 0, respectively). On average, OsUSP genes that contained both USP and kinase domains had a higher number of introns compared to those with only a USP domain. This indicates a similarity in the exon–intron architecture between genes that were more similar on a protein level. Similarly, the number of exons varied from one to eleven, with OsUSP32 having the highest number of exons. Only a single exon was found in four genes, namely – OsUSP19, OsUSP23, OsUSP26, and OsUSP30. Several OsUSP genes such as OsUSP1, OsUSP12, OsUSP25, OsUSP31 contain multiple 3′-UTR regions. This likely reflects the possibility of alternative splicing in these genes.
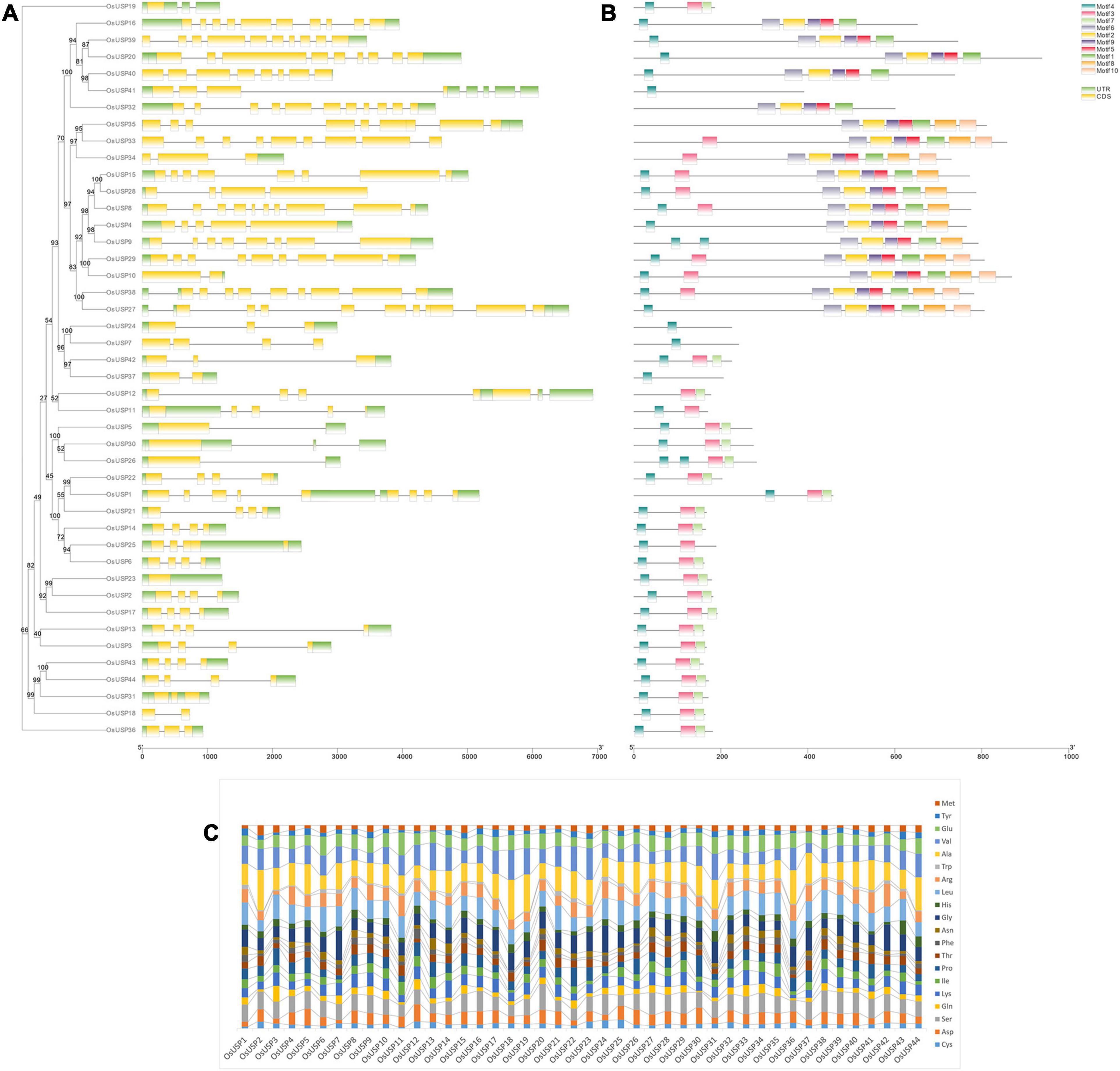
Figure 2. Overview of gene structure, conserved motifs, and amino acid composition of OsUSP members. (A) Exon–intron distribution of all the identified OsUSP genes. Yellow and green boxed indicate exon and UTR regions, respectively, whereas black lines indicate the position of introns. (B) The position of conserved motifs in OsUSPs. Each colored box indicates a single motif (legends are provided in the figure). The two scales at the bottom indicate gene (left) and protein (right) length. A maximum-likelihood tree with 1000 ultrafast bootstrap replicates was constructed using IQ-Tree. The bootstrap values are given as numbers on the internal nodes. (C) Amino acid (aa) composition of OsUSPs shown in a stacked bar plot. The percent content of each amino acid is indicated with a different color (see figure for details).
Elucidation of conserved motifs using MEME software revealed a total of 10 conserved motifs among rice USP proteins (Figure 2B). As expected, there was quite some variation in terms of motif number in each protein. In general, most proteins either contained three, nine or all 10 of the conserved motifs. This observation perfectly coincided with the presence of all three domains – USP, Kinase, and U-Box; two domains – USP and Kinase; or just a single USP domain in the protein. In the former case, 10 motifs were found in the proteins, whereas for those with only the USP domain, the number of conserved motifs was between one to three. From this observation, it could be deduced that motifs 3, 4, and 7 are associated with the USP domain; motifs 1, 2, 5, 6, 8, and 9 are linked to the kinase domain and motif 10 corresponds to the U-box domain. In addition to this, the amino acid (aa) composition of OsUSPs (Figure 2C) showed that OsUSPs with the same domain(s) show more similarity in their aa pattern compared to those with slightly different domain attributes. This can be exemplified by looking at the aa composition of USP 32-39 (Figure 2C). Here, USP 32-35 and 38-39 have both a USP and a kinase domain and are relatively alike in their aa composition. However, USP36 and USP35 lack a kinase domain and it is discernible that their aa composition is more diverse from USP 32-35 and 38-39. There appears to be a consistency in gene structure, motif conservation, and AA composition with the type of domain a given OsUSP contains.
Chromosomal Distribution and Synteny Analysis
The identified OsUSP genes were distributed over 11 out of 12 rice chromosomes (Figure 3A). The exact number of OsUSP genes per chromosome varied widely. Chromosome 2 contained the highest number of eight USP genes, followed by chromosome 1 with seven genes. A relatively moderate number of USP genes were found in chromosomes 5 and 12, where the number reached six and five, respectively. Both chromosomes 3 and 10 contained four members. As for other chromosomes, the number of USP gene family members ranged from one to three, with chromosome 4 being the only exception, which did not contain any USP genes.
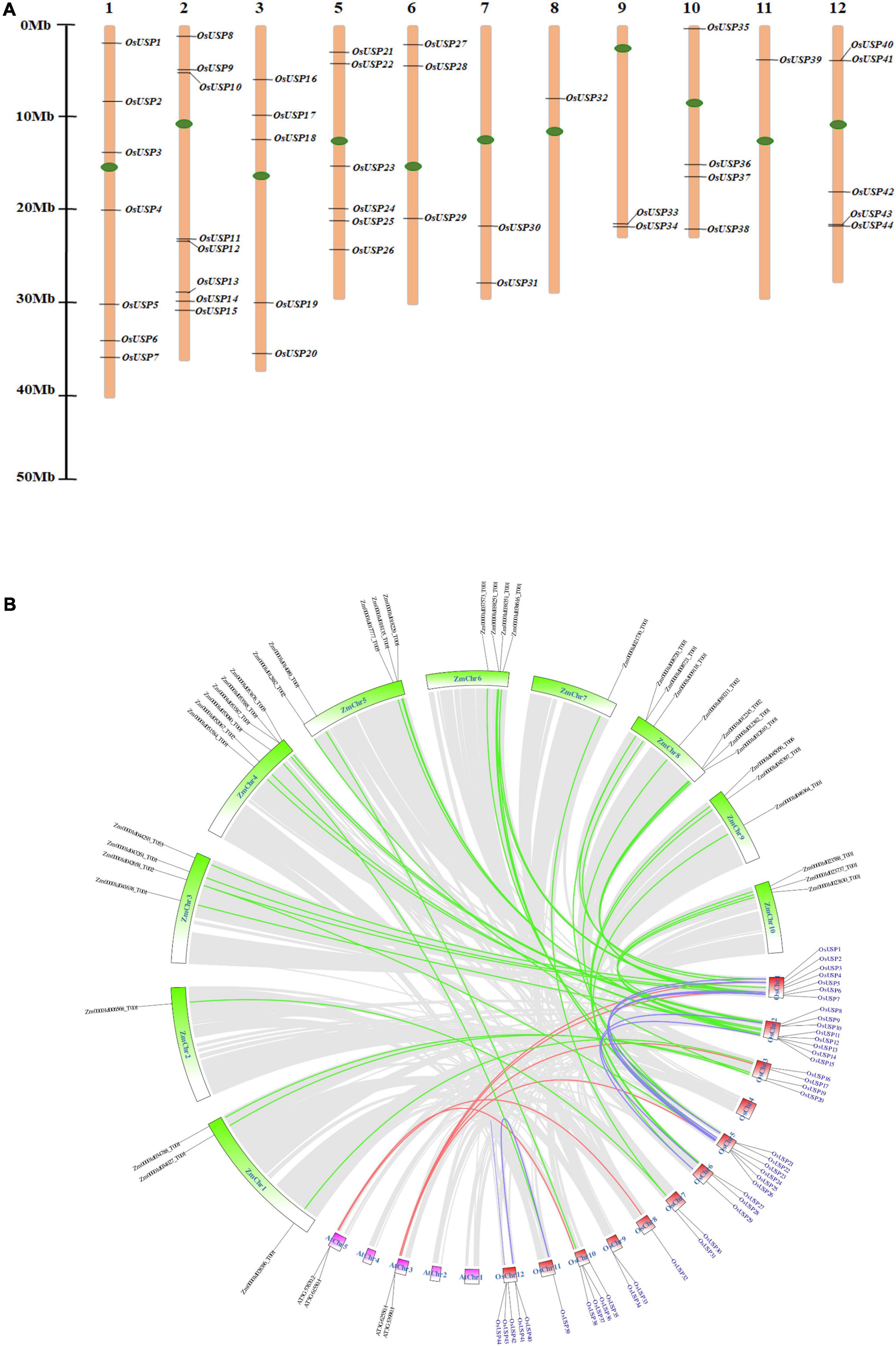
Figure 3. Chromosomal localization and syntenic relationship of rice USP genes. (A) Distribution of 44 OsUSP genes over 11 rice chromosomes. Each OsUSP gene was pointed to the exact position of a particular chromosome that could be calculated by using the scale provided on the left. Each chromosome number is indicated on the top of each chromosome bar. Maximum eight genes clustered in chromosome 2, while minimum one gene was present on chromosome no 8 and 11. The left bar represents scale in Megabase (Mb). (B) Synteny analysis of the rice genome with itself, with Arabidopsis thaliana and with maize genomes. Synteny blocks corresponding to OsUSP genes were indicated by different colors. Blue lines indicate synteny between OsUSP genes, the red line indicates synteny between OsUSP genes and their orthologs in Arabidopsis thaliana, and green lines indicate synteny between OsUSP genes and their orthologs in maize. Chromosomes of the three species were shown in different colors – red, pink, and green represent rice, Arabidopsis thaliana, and maize chromosomes, respectively. Positions of all OsUSP genes (blue) and their counterparts in two other species (black) were indicated as well.
The gene duplication events among OsUSP genes were investigated to evaluate the extension of this gene family members. A total of 11 OsUSP gene pairs were found to be duplicated- OsUSP1 and OsUSP22, OsUSP2 and OsUSP23, OsUSP2 and OsUSP17, OsUSP4 and OsUSP29, OsUSP5 and OsUSP26, OsUSP6 and OsUSP25, OsUSP7 and OsUSP24, OsUSP9 and OsUSP29, OsUSP15 and OsUSP28, OsUSP18 and OsUSP31, and OsUSP2 and OsUSP23 (Supplementary Table 6). All of them are possessing segmental type of duplication, except one with tandem type. A negative selection was observed for all the duplicated pairs except one with the ratio of nonsynonymous (Ka) to synonymous (Ks) values more than one. The calculated divergence time of the duplication was varied from 28 to 150 Mya (Supplementary Table 6).
Chromosomal synteny analysis was carried out to understand the expansion and diversification of USP genes within the rice genome and to compare with other monocot (maize) and dicot (Arabidopsis) organisms (Figure 3B). Both segmental and tandem duplication were visible for USP genes within the rice genome, with the former dominating the latter. A total of sixteen paralogous USP genes were recognized within the rice genome that were localized on chromosomes 1, 2, 5, 6, 11, and 12. Whereas, a single tandem duplication was detected on chromosome 12 between OsUSP43 and OsUSP44. Interesting observations came to light during the syntenic comparison of OsUSP genes with Arabidopsis and maize. Starting with the dicot genome, only four OsUSP orthologs were detected in the Arabidopsis genome and were limited to chromosomes AtChr3 and AtChr5 (Figure 3B). In contrast to this, a relatively large number of OsUSP orthologs were detected in the monocot plant, maize. In total, 37 orthologous genes were spread throughout all maize chromosomes (Figure 3B). This likely indicates that OsUSP genes have diverged more from their counterparts in the Arabidopsis genome compared to the more closely related monocot, maize. Overall, this indicates that both duplication and intense diversification have played a characteristic role in the evolution of the OsUSP gene family.
Phylogeny of USPs From Three Species and Their Domain Architecture
To explore the evolutionary pattern of USP proteins in three species – rice, Arabidopsis, and E. coli, we carried out a phylogenetic analysis using their all USP members (Figure 4). The addition of domain architecture of these proteins to the tree showed two clear clusters – one with members that contain only a USP domain (Figure 4, green cluster) and the other with members that mostly contain an additional kinase domain on top of the USP domain (Figure 4, yellow cluster). Overall, the number of proteins with only a USP domain was higher than the other type with more structural diversity in terms of their domain architecture. Focusing on the yellow cluster, most members had a kinase domain in addition to the characteristic USP domain. For some members, the presence of a U-box domain can also be seen. Interestingly, two rice USPs that lack a kinase domain (OsUSP10 and 41) were also found to group in this cluster. With respect to comparison of USP evolution between species, all E. coli USPs grouped together in the first cluster (green). A closer inspection of the tree showed that USPs usually clustered closest to a paralog from the same species rather than its ortholog from another species. This is because only in a relatively small number of cases can clear orthologs between the two species be identified in the tree in Figure 4. For instance, clear orthologs in Arabidopsis could only be discerned for rice USPs – 9, 11, 14, 17, 25, and 37.
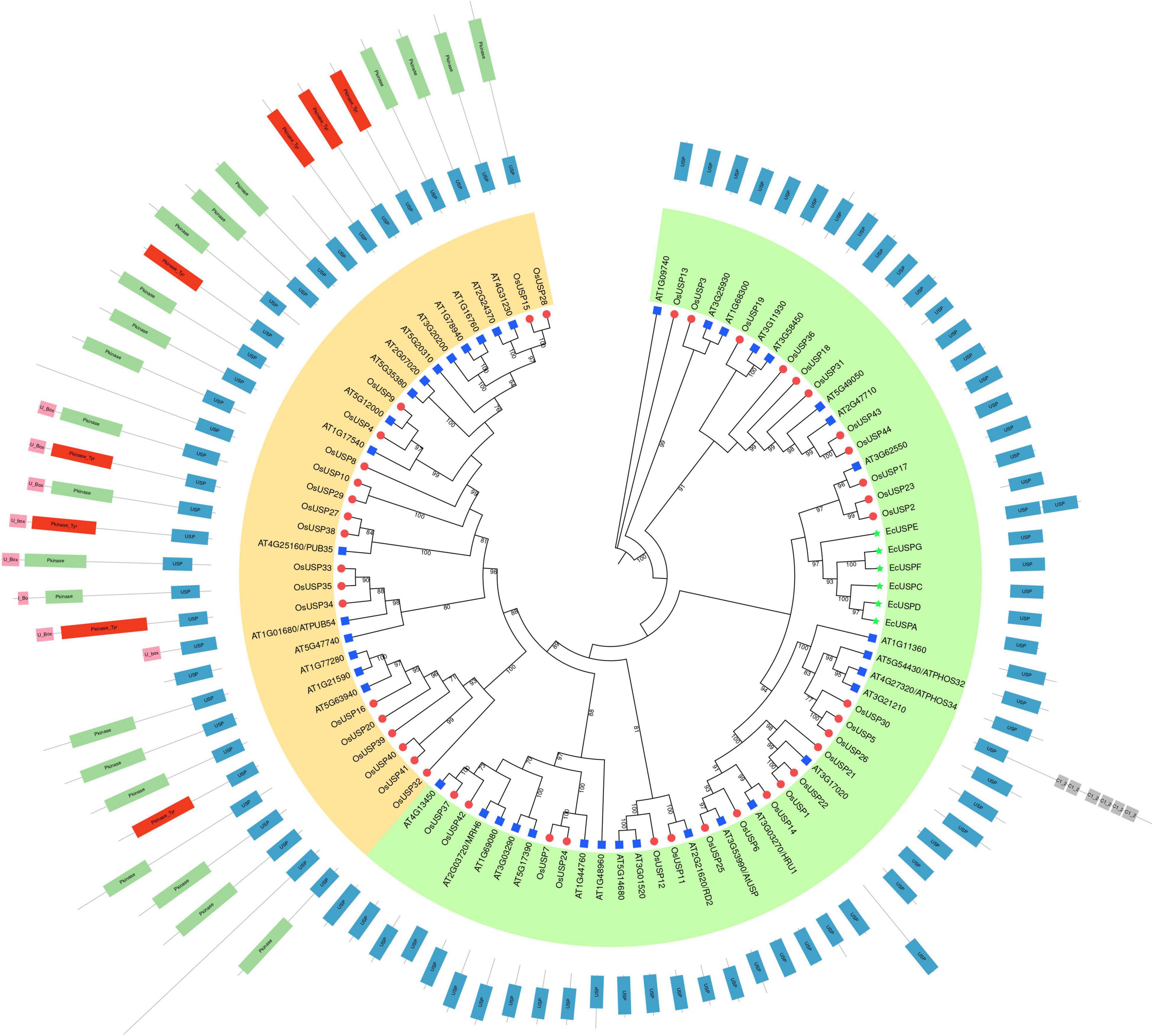
Figure 4. Phylogenetic relationship between rice, Arabidopsis thaliana, and E. coli USPs. A maximum-likelihood tree with 1000 ultrafast bootstrap replicates was produced using IQ-Tree. The final tree was visualized and edited in iTOL. USP members from the three different species are specified using different colored shapes – blue rectangles, red circles, and green stars sequentially specify Arabidopsis thaliana, Oryza sativa, and E. coli USPs. Overall, USPs were sub-grouped into two clusters – green and yellow based on the domain architecture of each protein. The green clusters exclusively contain protein that harbors only a USP domain. As for the yellow cluster, almost all members harbor an additional kinase or U-Box domain or both (except a few). Information regarding the presence and position of a domain was added in iTOL and the name of each domain is indicated within the corresponding boxes.
In silico Identification of SSRs, and Prediction of Glycosylation and Phosphorylation Sites
Molecular markers allow the identification of genes of interest within a particular location. A major concern of genome analysis is to explore molecular markers related to genetic factors underlying observable traits. Forty-four OsUSP genes with all splice variants were analyzed for SSR markers. Out of 61 sequences, 19 SSR markers were distributed among 15 sequences. The most abundant were trinucleotide repeats accounting for 89.47% (17 occurrences), followed by mononucleotide and dinucleotide repeats both with one occurrence accounting for 5.26% of all SSRs, no tetra, penta or hexanucleotide repeats were found. The dominant SSR motif was GCG with a frequency of 47.36 with nine occurrences followed by other mono, di and trinucleotide repeats (Supplementary Table 7). Higher repeat numbers were observed in trinucleotide SSRs. Several sequences possessing more than one SSR marker were found three. Furthermore, glycosylation plays a key role in secondary protein processing for the functioning of protein within cells and in determining protein structure, function, and stability. In this study, 25 out of 44 OsUSPs have shown one to many predicted glycosylation sites with the threshold of 0.5. A total of 68 glycosylation sites have been predicted in 25 OsUSP proteins. The highest number of glycosylation sites was found in OsUSP8 with nine sites followed by OsUSP10, OsUSP32, OsUSP35 (five sites) and OsUSP27, OsUSP33, OsUSP38 with four sites. N-Glycosylation score greater than 0.5 and jury agreement 9/9 or potential >0.75 indicates high specificity of glycosylation site. Among the total of 68 predicted sites, 19 sites in 13 OsUSP proteins have the potential score greater than 0.5 and jury agreement 9/9, and thus, have the highest chance of glycosylation mediated stable structure formation (Supplementary Table 8). Protein phosphorylation is the another most significant type of post-translational modification of cells in which protein kinases phosphorylate an amino acid residue, mostly Tyr, Thr, Ser in the case of eukaryotes by adding covalently bound phosphate groups. A significant number of phosphorylation sites were predicted in all the members of OsUSP (Supplementary Table 9). The highest number of phosphorylation sites were predicted in OsUSP20, followed by OsUSP29, OsUSP27 and OsUSP39. Predicted results also showed that serine (S) sites were more phosphorylated than threonine (T) and tyrosine (Y) sites. As for the types of kinases, the prevalence of the predicted ones was not specific, while PKC was the most common.
Analysis of Expression Pattern of OsUSP Genes During Developmental Stages and in Various Tissues of Rice
To get a notion about the magnitude of OsUSP genes expression, we carried out an expression analysis using previously generated transcriptomics data for OsUSP genes during different developmental stages (Figure 5A) and anatomical tissues (Figure 5B). Most of the OsUSP genes consistently show high levels of expression during all the developmental stages (Figure 5A) except OsUSP18, OsUSP40, OsUSP41, and OsUSP42. These genes show relatively low expression levels in almost all stages. However, some interesting candidates show preferentially high expression during certain stages. For instance, OsUSP15 is highly expressed during the flowering and heading stage but less prominent during germination, seedling, tillering, and mature grain stage. A similar observation is true for OsUSP8 and OsUSP37 with the latter showing a stronger contrast in expression.
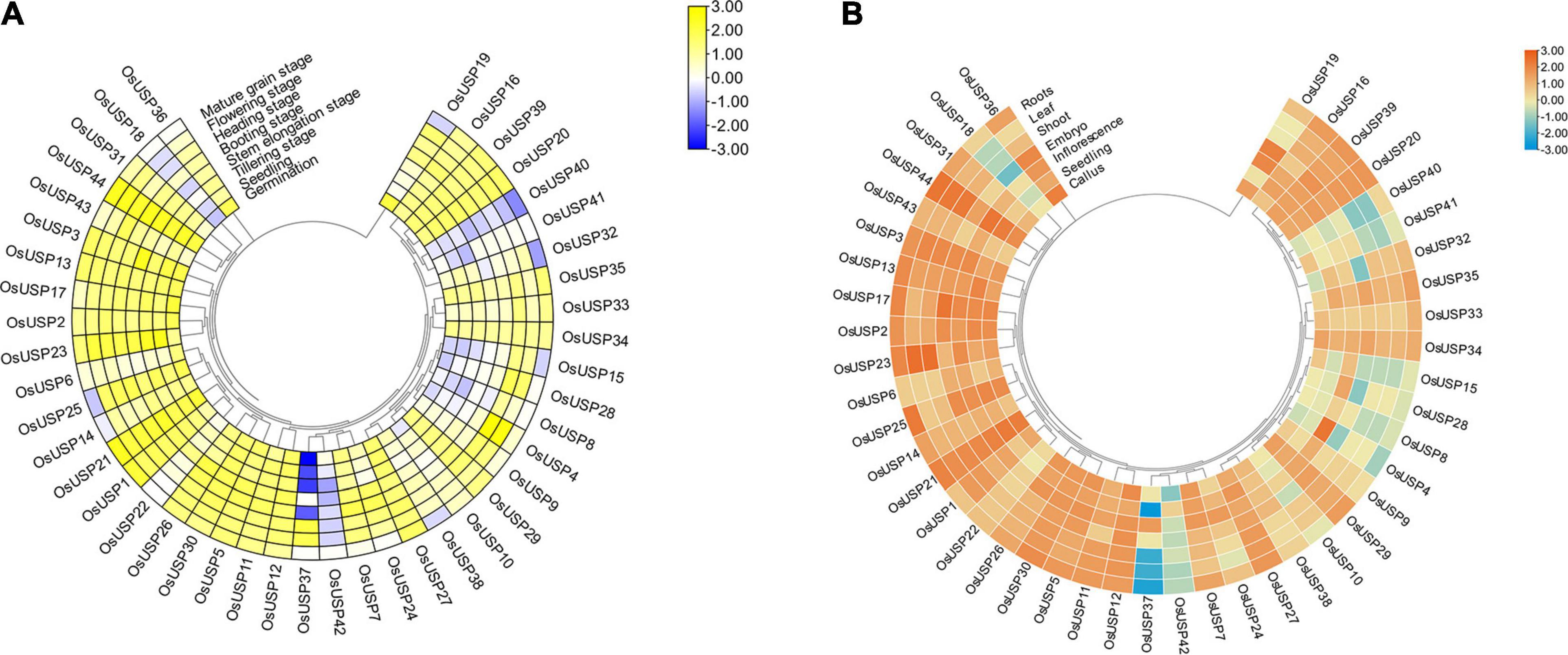
Figure 5. Overview of the developmental stage and tissue-specific expression profile of OsUSP genes. (A) Expression pattern of each OsUSP gene during multiple developmental stages of rice plants. (B) Expression pattern of OsUSP genes in different tissues of rice plants. The developmental stage and tissue-specific expression data were downloaded from Genevestigator using the corresponding gene IDs of rice USPs. Raw expression levels were log10-tranformed and visualized with “Heatmap Illustrator” option in TBtools (v.1.092). The scale indicates the magnitude of gene expression. The phylogenetic tree is same as the one shown in Figure 2.
Moving onto expression profiles in tissues, a similar pattern was observed where most OsUSP genes exhibited moderate to high expression levels in all tissues (Figure 5B), with exception of OsUSP4, OsUSP8, OsUSP15, OsUSP18, OsUSP28, OsUSP37, OsUSP40, OsUSP41, and OsUSP42. Some genes showed a mixed pattern, while some tissues are showing higher expression for few genes than others. OsUSP4 showed very high expression in inflorescence tissues but moderate to low level of expression in others. Comparably, OsUSP37 had a very low expression in roots, shoots, leaves, and seedlings but a high expression in inflorescence tissues. Overall, most of OsUSP genes showed an appreciable level of expression both during different developmental stages and tissues of the plant.
Analysis of Stress-Specific Expression Pattern of OsUSP Genes and Their Cis-Regulatory Elements
To evaluate the role of OsUSPs in stress conditions, analysis of the expression data of all the identified OsUSP genes were performed under multiple stress conditions including – heat, salt, drought, dehydration, and submergence (Figure 6A). Expectedly, most OsUSP genes showed strong upregulation or downregulation in response to these adversities. However, the number of upregulated genes was slightly higher than those that were downregulated. Some USP genes including – OsUSP2, OsUSP17, OsUSP22, OsUSP23, OsUSP26, OsUSP31, and OsUSP36 were strongly upregulated (even higher than 6-fold) under submergence conditions. All genes (except OsUSP26) showed a strong upregulation from the early onset of submergence. Some genes such as OsUSP1, OsUSP10, OsUSP16, OsUSP20, OsUSP28, OsUSP42 showed downregulation as well. However, the magnitude of downregulation was not as strong for those that were upregulated. In the case of heat stress, the expression of OsUSP genes varied quite a lot. The strongest upregulation was detected for OsUSP14, whereas some genes showed both upregulation and downregulation in a temporally separated fashion like OsUSP15, OsUSP22, OsUSP30, etc. Moving on to dehydration and drought stress, upregulation of several USP genes was more prominent at the early onset of stress application. For instance, genes OsUSP3, OsUSP5, OsUSP11, OsUSP14, OsUSP19, OsUSP22, OsUSP23, OsUSP26, and OsUSP36 showed considerable upregulation at the earlier stages of drought stress, but their expression varied in the later time points. Some of these genes such as OsUSP5 and OsUSP14, showed upregulation in both conditions and at all time points. There was also a mixed response of OsUSP genes in response to salt, with some being upregulated and others downregulated. The magnitude of upregulation was stronger mostly at the longer period of salt application (24 h). In terms of consistency across all stress conditions, the expression profile of OsUSP2, OsUSP3, OsUSP22, OsUSP32, OsUSP33, OsUSP39, and OsUSP44 was very interesting. In general, OsUSP3, OsUSP22, OsUSP39, and OsUSP44 were mostly upregulated and OsUSP2, OsUSP32, and OsUSP33 were mostly downregulated across the conditions (Figure 6A).
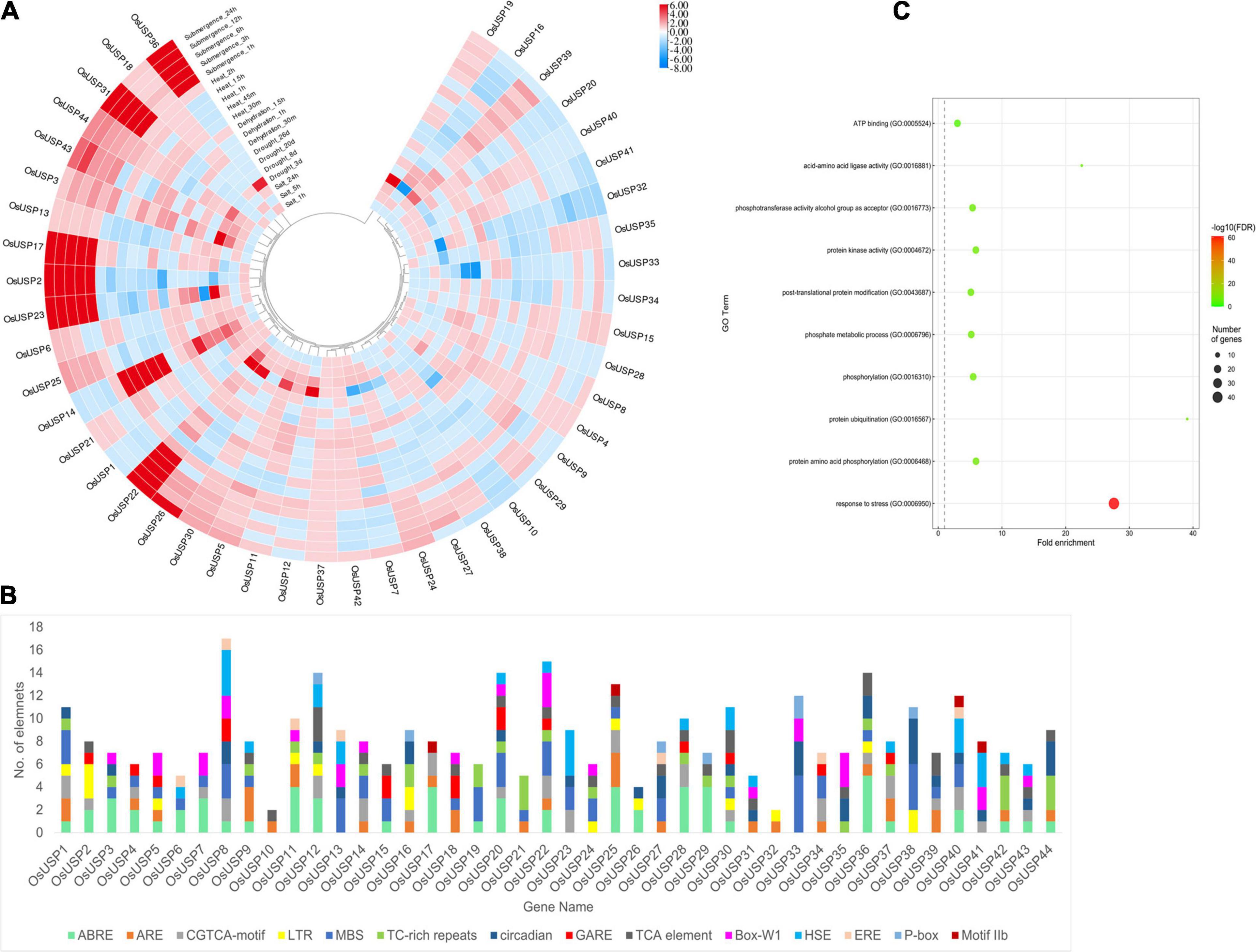
Figure 6. Overview of the stress-specific expression profile of OsUSP genes, the composition of their promoter regions, and functional annotation. (A) Modulation of OsUSP gene expression under several abiotic stress conditions – submergence, heat, dehydration, drought, and salt stress. Expression data of rice USP genes under multiple abiotic stresses were downloaded from Genevestigator using the corresponding gene IDs. Change in the expression pattern was calculated in terms of log2-fold change. The scale on the right indicates the magnitude of up or downregulation. For some genes, the amount of fold change was higher (or lower) than indicated by the scale. However, to maintain uniformity in the colors of the boxes the scale was limited to +6 and –8 manually. The phylogenetic tree is similar to the one shown in Figure 2. (B) The presence of different cis-regulatory elements in the 1000 bp – upstream region of OsUSP genes is shown using a stacked bar plot. Each color indicates a different cis-regulatory element (see figure for legends) and the length of the bar designates the number of elements. Several stress-responsive cis-regulatory elements were visible including – ABRE, ARE, MBS, LTR, HSE, P-box, etc. (C) GO-enrichment analysis on OsUSP genes showed enrichment for stress response in over 40 USP genes. The size of the circles indicates the number of gene and the color corresponds to the –Log10(FDR) values.
Additionally, we checked the composition of the putative promoter region of OsUSP genes concerning the presence of different cis-regulatory elements. After surveying the 1000 bp-upstream sequence of each gene, most rice USP promoters harbored numerous cis-regulatory elements which are known to be involved in stress-induced gene regulation (Figure 6B). The motifs that were most commonly found in the OsUSP promoter regions were – ABRE, ARE, LTR, MBS, and TC-rich repeats, which are recognized for attracting stress-specific transcription factors (TFs). To add to this line of evidence, a GO enrichment analysis on all the OsUSP genes was performed (Figure 6C). This showed that out of the 44 genes identified, 41 OsUSP genes were annotated to be involved in stress response (GO:0006950). In short, the stress-specific modulations of rice USP genes are clear.
Validation of Gene Expression Profile of Nine Selected OsUSP Genes in Response to Abiotic Stress
As an expression profile of genes under stress condition is important to understand their function, it is crucial to assess mRNA-seq expression patterns experimentally to ensure consistency. Thus, we carried out quantitative RT-PCR analysis of nine selected OsUSP genes in leaf tissue after 16 h of abiotic stress treatments including salt, drought, cold, heat, dehydration, H2O2 and hormonal treatment – ABA, SA, and GA3. The real-time PCR expression profile of the selected genes (Figure 7) reveal a good correlation with the mRNA-seq data (Figure 6A). Among nine genes, OsUSP2, OsUSP3, OsUSP6, OsUSP12, OsUSP14, OsUSP32, OsUSP33 showed considerable upregulation in heat stress whereas OsUSP22 and OsUSP36 showed downregulation. Consistent with the mRNA-seq expression data, OsUSP14 and OsUSP22 genes showed a similar pattern of expression under heat stress. Under salinity stress OsUSP2, OsUSP3, OsUSP6, OsUSP12, OsUSP14 and OsUSP32 showed low to medium upregulation while OsUSP22, OsUSP33 and OsUSP36 showed downregulation in rice leaves. Some genes such as, OsUSP2, OsUSP22 and OsUSP32 showed differential expression patterns in salt stress which is different from the mRNA-seq data. However, the expression level of OsUSP3 and OsUSP33 were consistent with the expression pattern mRNA-seq data. Furthermore, the qRT-PCR results revealed that the expression level of OsUSP2, OsUSP3, OsUSP12, OsUSP14, OsUSP32, OsUSP33 was upregulated under drought stress. Apart from that, OsUSP6 showed no fold change, compared to control under drought stress. In dehydrated condition, only OsUSP2, OsUSP12 and OsUSP33 showed reasonable upregulation, while OsUSP14 showed downregulation. The occasional inconsistency of gene expression of the selected genes between mRNA-seq and RT-PCR might be due to the differences in sampling time following stress/hormone treatment or the genotypic difference between the rice japonica variety and the BRRI-53 variety.
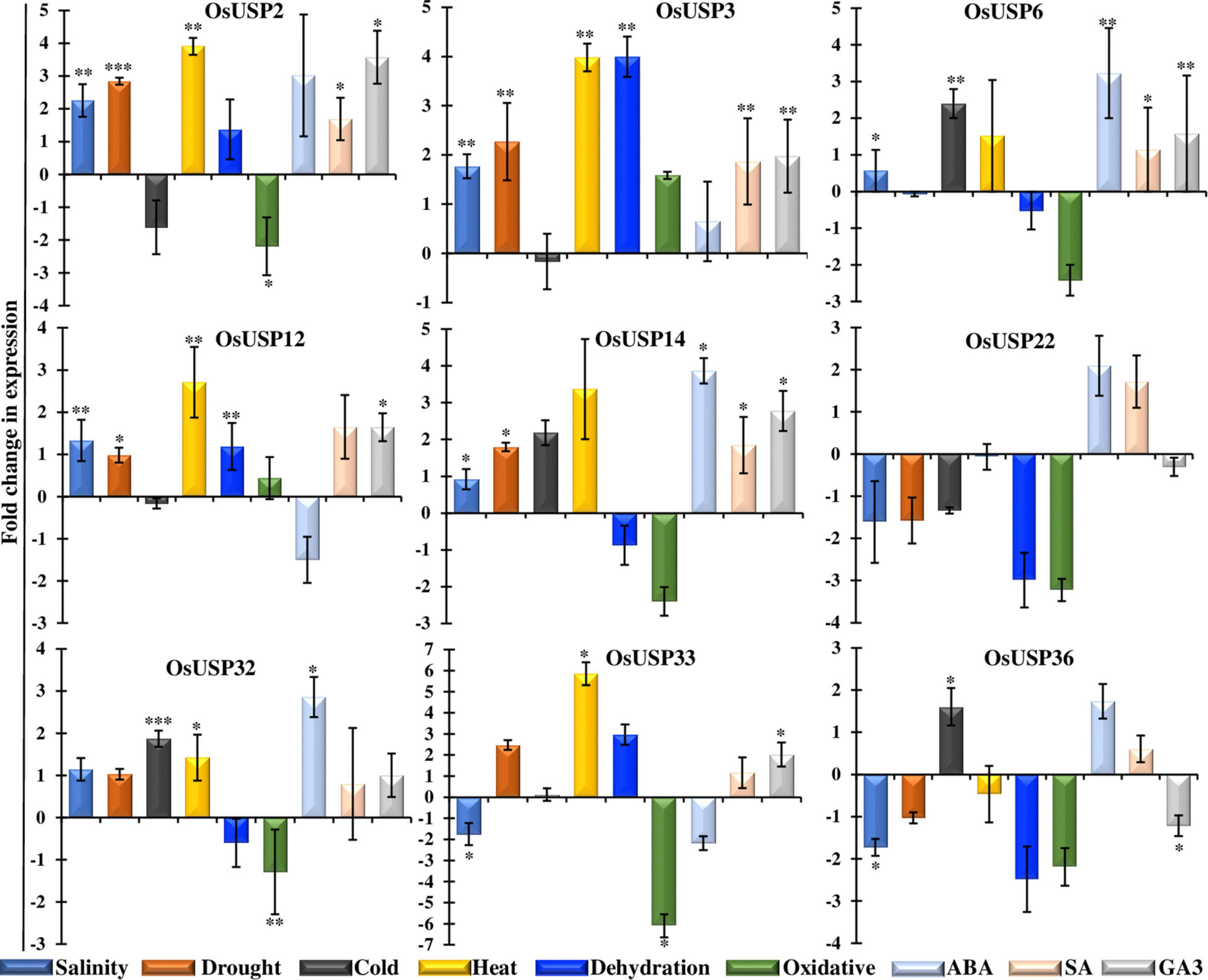
Figure 7. Functional validation of selective OsUSP genes expression in response to various abiotic stresses and hormonal treatments by qRT-PCR. Expression profiles of nine OsUSP genes were performed in response to salinity, drought, cold, heat, dehydration, and oxidative stresses, and ABA, SA and GA3 treatments in BRRI-53 rice variety. A scale showing Log2-fold change of each gene under each condition was presented at the left. The results were represented by the mean value of fold change ± standard deviation using rice eEF1 gene as a reference. Statistical significance was determined using two-tailed paired Student’s t-test and are represented with ∗, ∗∗, ∗∗∗ for a P-value < 0.05, <0.01, and <0.001; respectively.
Expression analysis of nine selected OsUSP genes were further conducted in other abiotic treatments such as cold, oxidative, and hormonal stress. In cold treatment, OsUSP genes showed low to medium upregulation in leaf samples. OsSUP6 does not show any expression in drought stress but is highly expressed in cold stress whereas OsUSP33 showed negligible up-regulation which is close to control. OsUSP14, OsUSP32, OsUSP36 showed up-regulation under cold stress. But we observed that the other USP genes such as OsUSP2, OsUSP3, OsUSP12, OsUSP22 and OsUSP36 were downregulated in cold. OsUSPs showed down-regulation in oxidative stress except OsUSP3 and OsUSP12, which need to be investigated further. Furthermore, OsUSP showed a differential regulation in rice leaf tissue under hormonal influences like ABA, SA and GA3 (Figure 7). The qRT-PCR results suggested that most of the USP genes such as OsUSP2, OsUSP3, OsUSP6, OSUSP14 and OsUSP32 up-regulated in all the hormonal stresses. Apart from that, OsUSP12 and OsUSP33 showed upregulation but downregulated in ABA stress. The varying responses of OsUSPs could thus be attributed to different abiotic and hormonal stresses.
Molecular Docking Analysis of Selected OsUSPs
Among 44 OsUSP proteins, 9 OsUSPs showed significant up and down-regulation to various stresses and were selected as receptors for docking study to check their affinity toward various inhibitors (Table 2). Of these 9, only two (OsUSP32, OsUSP33) have both USP and kinase domain, docked against UspA and PKC inhibitors. Rest seven contain only the USP domain, therefore, they were docked against only UspA inhibitors. All these selected nine proteins showed significant affinity toward UspA inhibitors (ZINC000104153710, ZINC000004268284, ZINC000000217308), and both OsUSP32 and OsUSP33 showed affinity toward four kinase inhibitors (Fisetin, Luteolin, Myricetin, Quercetin) (Table 2). Among the three USP inhibitors the highest affinity was found for Zinc000104153710 and in the case of four kinase inhibitors, Luteolin showed the highest affinity. Among all nine selected proteins, OsUSP32 showed the highest affinity toward UspA inhibitor Zinc000104153710 and PKC inhibitor luteolin with a binding energy of −9.6 kcal/mol and −9.2 kcal/mol, respectively, followed by OsUSP33 (−8.5 kcal/mol, −8.2 kcal/mol, respectively). The protein-ligand interaction of docked complexes was visualized using the Discovery Studio program (Figures 8A–D). The best-scored protein OsUSP32 with the highest binding affinity toward Zinc000104153710 formed one hydrogen bond with Asp443 having a distance of 2.21 Å, two pi-sigma bond with Val324 (3.93 Å), Leu432 (3.88 Å), one pi-alkyl bond with Ala359 (5.44 Å) and five alkyl bond with Ala306 (5.49 Å), Tyr311 (4.01 Å), Val314 (5.50 Å), Ala326 (4.62 Å, 5.06 Å) to stabilize within the binding pocket (Figure 8B). The highest binding affinity toward Luteolin was showed by OsUSP32 by forming one pi-pi-T-shaped bond with Phe374 (4.32 Å), two alkyl bond, one pi-alkyl bond, one pi-sigma with Ala306 (4.53 Å, 4.24 Å), Ala326 (5.35 Å), Val314 (4.43 Å), respectively (Figure 8A).
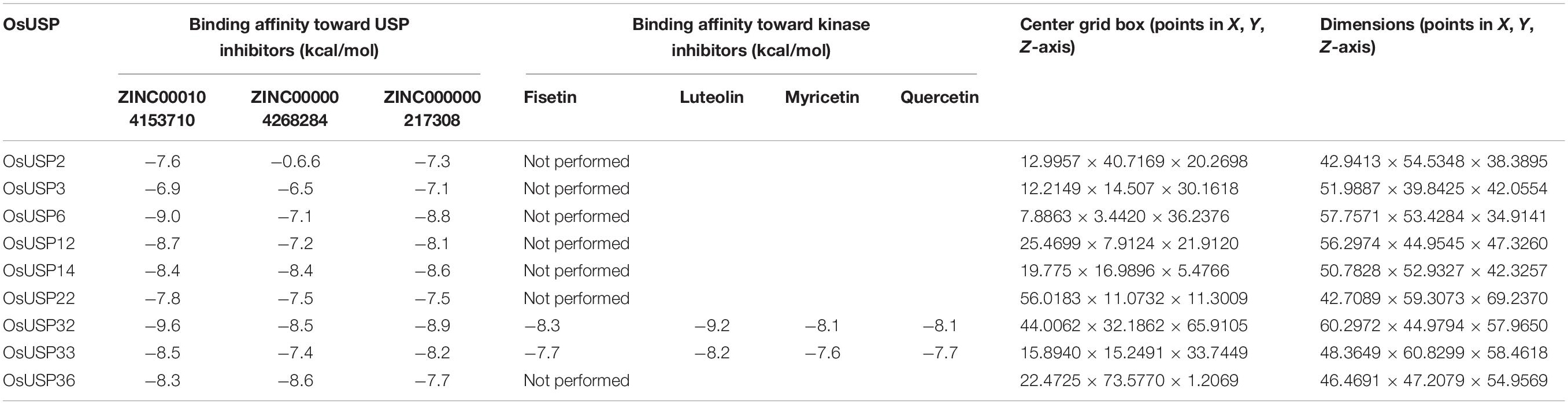
Table 2. Predicted binding affinity (kcal/mol) of selected OsUSP proteins against known USP and kinase inhibitors.
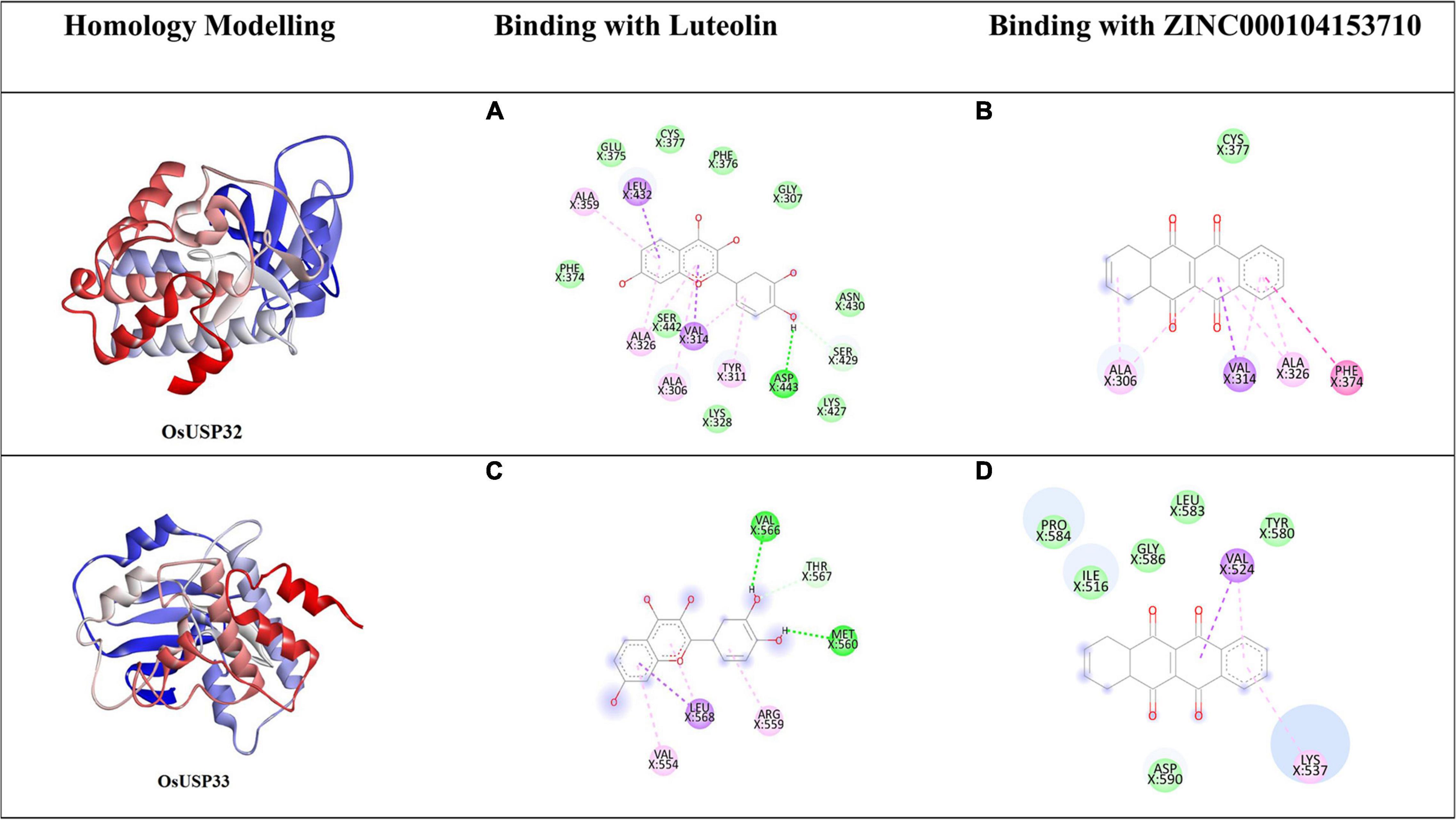
Figure 8. Homology modeling and molecular docking of two OsUSP (OsUSP32, OsUSP33) proteins having both USP and Pkinase domain. The first column represents the predicted 3-D structure, the second and third column represents the 2-D interaction of each protein with Luteolin (A,C) and ZINC000104153710 (B,D), respectively. The green, light green, pink, violet, purple represent residues involved in the hydrophobic interactions, carbon-hydrogen bond interactions, Pi-Pi-T shaped, Pi-Sigma and Pi-Alkyl interactions, respectively.
Discussion
Universal stress proteins have been shown to be induced under various abiotic stresses. To date, a diverse number of USP gene family have been identified from different plant species including, Arabidopsis (Bhuria et al., 2019), Brassica napus, Triticum aestivum, Brassica rapa, Solanum lycopersicum, Solanum tuberosum, Oryza sativa japonica, Vitis vinifera, Zea mays (Chi et al., 2019), Malus sieversii (Ledeb.) (Yang et al., 2019), Barley (Li et al., 2010), Pigeon pea (Sinha et al., 2016) etc. Usually, plant genomes harbor around twenty to fifty genes (Gou et al., 2020). However, more than a hundred members have been reported in the genome of Brassica napus and Triticum aestivum (Li et al., 2010; Chi et al., 2019). In the present study, a total of 44 OsUSP genes (Table 1) were identified in the rice genome.
The identified USP genes are spread throughout 11 out of 12 rice chromosomes (Figure 3A). The number of genes is slightly higher than previously reported (Bhowmick, 2019; Chi et al., 2019). Investigation into the structural properties of the identified genes and their corresponding protein showed that domain architecture played an important part in this. Analysis of synteny between rice USP genes showed that 16 out of 44 genes had traces of segmental duplication whereas two genes on chromosome 12 were likely derived from a tandem duplication event (Figure 3B). Thus, it appears that both segmental and tandem duplication played a part in the expansion of the USP gene family in rice. Further investigation into the syntenic relationship between rice USP genes and the genome of Arabidopsis thaliana and Zea mays (maize) revealed the presence of a higher number of orthologs in the monocot maize genome compared to the dicot Arabidopsis thaliana (Figure 3B). This indicates greater diversification of USP genes between the two angiosperms lineages – monocots and dicots compared to divergence within each lineage. In this regard, our study suggests that both duplication and diversification have played a major role in the evolution of the USP gene family in plants.
Analyses of the OsUSPs phylogeny revealed two clusters that finely coincides with the domain architecture of the proteins. The cluster (green) that groups with E. coli USPs likely represents those members that originated earlier in the evolutionary history of the USP gene family. In contrast, members from the other cluster (yellow) showed longer peptide lengths and the presence of a functional kinase domain. However, there were a few exceptions here, like OsUSP10 and OsUSP41. Two USP genes could have undergone mutations that have rendered an existing kinase domain non-functional, or they could be on the verge of developing a kinase domain. The latter case is less likely, as these two USPs do not group closer to the green cluster which supposedly originated first. The existence of diverse domains in USP members in the yellow cluster signifies the functional diversity of USP members in plants. These proteins may have evolved to serve varied functions in protecting the plant from diverse stressors. Thus, it is imperative to accurately identify and further explore the diversity of these genes.
Molecular markers can be used as a selection tool for investigating genetic diversity, transferability of genes for genome editing purposes. In our study, we identified 19 SSR markers based on their high reproducibility, polymorphic genetic information, and hypervariable nature. As they produce high allelic variations among very closely related varieties, these markers can be used for the identification of specific USP members in future. In concordance with previous studies in Viridiplantae, we also found trinucleotide type as the dominant motif (Isokpehi et al., 2011).
Looking at the expression pattern of OsUSP genes we found that several members showed modulation under different abiotic stress conditions (Figure 6A). A number of these genes exhibited strong upregulation when exposed to these stressors. These findings fit well with previous reports of the stress-specific regulation of USP genes (Loukehaich et al., 2012; Jung et al., 2015; Melencion et al., 2017). Moreover, it has been demonstrated that overexpression of certain USP genes lead to enhanced tolerance to heat and osmotic stress. In relation to this, candidate OsUSP genes may also have similar functional roles in stress tolerance. On top of this, we found the presence of multiple stress-responsive regulatory elements upstream of OsUSP genes. These include – ABRE (ABA response), ARE (anoxia), LTR (low temperature), MBS (drought), TC-rich repeats (stress and defense), HSE (heat shock), etc. A previous report by Bhuria et al. (2016) showed that AtUSP promoter is highly inducible by multiple abiotic stressors and was able to produce multi-stress tolerance. In another study in cotton plants, the promoter of a USP gene was found to be responsive to dehydration, ABA, salt, heavy metals, and gibberellic acid (Zahur et al., 2009). Moreover, Wild tomato USP genes were induced under ABA, ethylene, drought, salt, heat, wounding, oxidative, and cold stress (Loukehaich et al., 2012). Similarly, the SbUSP gene from Salicornia brachiata also showed expression under salt, drought, cold, and heat stress (Udawat et al., 2014). Overall, these provide extensive evidence for the differential modulation of rice USP genes under abiotic stress conditions and that their promoters have the capacity to induce stress resilience, making them good candidates to drive multiple stress-responsive expression of transgenes in genetically engineered plants.
In coherence with this, during in silico expression profiling of OsUSP genes, we also found that expression of most rice USP genes was closely associated with heat, salinity, drought, dehydration, and submergence. Next, we used qRT-PCR to correlate the stress-specific function of the selected USP genes under multiple abiotic stresses. These led to the confirmation of the modulation of these rice USP genes under varying environmental stressors. For example, OsUSP3 showed upregulation under all stress-conditions except cold which makes it a very suitable for downstream wet-lab validation (Figure 7). On the contrary, OsUSP22 was mostly downregulated in the abiotic stressors except ABA and SA, indicating a strong modulation under stress. Interestingly, we observed that only OsUSP3 and OsUSP12 have exhibit elevated expression under oxidative stress. In addition, the response of each USP to the variety of stressors varied widely, indicating the possibility of diverse modes of transcriptional regulation for members of this gene family in rice. Uncovering these exact natures of such regulatory modules and their functional consequences can be of great importance for plant stress-oriented research.
To further bolster our understanding the rice USP gene family, we focused on analyzing the protein products of these genes. Proper functioning of proteins depends greatly on the post-translational modifications (PTMs) of proteins, glycosylation and phosphorylation being major two. In this regard, the glycosylation process plays a major role in protein folding, stability and signal transduction (Arey, 2012), whereas phosphorylation plays a critical role in the activation or deactivation of proteins by altering structural conformation (Cohen, 2002) and plays a significant role in the signaling pathways (Cutillas, 2017), metabolism and in combating various stress conditions (Zhang et al., 2020). In response to abiotic stresses in crop plants, PTM of proteins have been reported in response to abiotic stresses in crop plants and is suggested to be involved in various biological processes under natural conditions (Wu et al., 2016). Previous studies on protein PTM in soybean and rice under stresses showed that glycosylation occurs in response to flood and cold (Wu et al., 2016). Here, we identified 68 predicted glycosylation sites, among which 19 sites showed the highest chance of glycosylation. This confirms the ability of OsUSP members to perform glycosylation mediated secondary structure modification that may regulate the protein functions in stress condition. Furthermore, various studies suggested that different kinases are activated following that their activation loop residues are phosphorylated (Ravala et al., 2015) and autophosphorylation of UspA protein of E. coli also occur in response to stasis (Freestone et al., 1997). Moreover, phosphorylation mediated PTM was found prominent in combating multiple abiotic stressors in rice, maize, wheat, and chickpea (Wu et al., 2016). Thus, the predicted phosphorylation sites with position and specific kinases from the present work could be used for further confirmation of the role of phosphorylation in the function of different OsUSPs by using various in vitro techniques.
Moreover, a deep understanding of the binding affinity and binding site residues of inhibitors to OsUSPs is needed for the improvement of abiotic stress-resistant crops. In this present study, we have performed blind docking (Hetényi and van der Spoel, 2002) method for exploration of possible binding sites and binding affinity of our proteins and toward some known USP and kinase inhibitors. In concordance with the previous study on UspA inhibitor in E. coli (Bandyopadhyay et al., 2021), ZINC000104153710 showed the best docking result, thus suggesting having an inhibitory effect on OsUSP activity. Furthermore, studies have found flavonoids acting as an inhibitor toward kinases (Cassidy and Setzer, 2010). Here, Luteolin showed the best result when docked against kinase domain containing OsUSPs. This strongly suggests that Luteolin may hinder kinase activity upon binding. This will facilitate further simulation, investigation regarding the interaction of other inhibitors to these binding sites and identification of some novel inhibitors that may inhibit the activity of USP proteins.
Conclusion
Overall, this study covers a comprehensive genome-wide scale investigation of USP gene family members in a staple crop, rice. In total, we were able to identify 44 USP genes in the monocot genome, a number slightly higher than previous reports. The key focus of this investigation was to perform an in-depth exploration of the structural and functional properties of the identified genes. This led us to discover the functional diversity of rice USP genes and their multi-stress regulatory nature. Moreover, using qRT-PCR, we were able validate this multi-stress responsive nature of promising OsUSP genes. However, further research is required to identify the functional contribution of these genes by generating overexpressing or knockout lines of USP genes alone or in combination. With the aid of modern biotechnological tools, the selected OsUSP genes could be targeted for improving not only rice but also other important crop species for developing multi-stress resilience plants.
Data Availability Statement
The raw data supporting the conclusions of this article will be made available by the authors, without undue reservation.
Author Contributions
TI conceived the idea and designed and supervised the experiments. SAr, AS, and SAk performed all the experiments and wrote the manuscript. TI and AS helped in data analysis. RS critically reviewed the manuscript. All authors read the manuscript and approved the final version.
Conflict of Interest
The authors declare that the research was conducted in the absence of any commercial or financial relationships that could be construed as a potential conflict of interest.
Publisher’s Note
All claims expressed in this article are solely those of the authors and do not necessarily represent those of their affiliated organizations, or those of the publisher, the editors and the reviewers. Any product that may be evaluated in this article, or claim that may be made by its manufacturer, is not guaranteed or endorsed by the publisher.
Acknowledgments
The authors gratefully acknowledge partial grant from Ministry of Information and Communication Technology (ICT), Government of People’s Republic of Bangladesh to pursue the study. The authors duly acknowledge University of Dhaka for providing partial publication fee. The authors acknowledge Plant Breeding and Biotechnology Laboratory, Department of Botany, University of Dhaka for the logistic support and laboratory facilities.
Supplementary Material
The Supplementary Material for this article can be found online at: https://www.frontiersin.org/articles/10.3389/fpls.2021.712607/full#supplementary-material
Supplementary Figure 1 | Quality and quantification of isolated RNA.
Supplementary Figure 2 | Homology modeling of seven OsUSP proteins having only USP domain.
Footnotes
- ^ http://rapdb.dna.affrc.go.jp/
- ^ http://pfam.sanger.ac.uk/
- ^ http://cello.life.nctu.edu.tw/
- ^ https://www.genscript.com/wolf-psort.html
- ^ http://www.cbs.dtu.dk/services/ChloroP/
- ^ https://plants.ensembl.org/info/data/ftp/index.html
- ^ http://viewer.shigen.info/oryzavw/maptool/MapTool.do
- ^ http://chibba.agtec.uga.edu/duplication/index/downloads
- ^ http://pgrc.ipk-gatersleben.de/misa/misa.html
- ^ http://www.cbs.dtu.dk/services/NetNGlyc/
- ^ https://services.healthtech.dtu.dk/service.php?NetPhos-3.1
- ^ https://genevestigator.com/gv/index.jsp
- ^ http://bioinformatics.psb.ugent.be/webtools/plantcare/html/
- ^ http://toolkit.tuebingen.mpg.de
- ^ http://www.pubchem.ncbi.nlm.nih.gov
References
Arey, B. J. (2012). “The role of glycosylation in receptor signaling,” in Glycosylation, ed. S. Petrescu (London: IntechOpen), doi: 10.5772/50262
Bailey, T. L., Boden, M., Buske, F. A., Frith, M., Grant, C. E., Clementi, L., et al. (2009). MEME SUITE: tools for motif discovery and searching. Nucleic Acids Res. 37, W202–W208. doi: 10.1093/nar/gkp335
Bandyopadhyay, D., Singh, G., Mukherjee, M., and Akhter, Y. (2021). Computational approach towards the design of novel inhibitors against Universal stress protein A to combat multidrug resistant uropathogenic Escherichia coli. J. Mol. Struc. 1238:130379. doi: 10.1016/j.molstruc.2021.130379
Barnabás, B., Jäger, K., and Fehér, A. (2008). The effect of drought and heat stress on reproductive processes in cereals. Plant Cell Environ. 31, 11–38. doi: 10.1111/j.1365-3040.2007.01727.x
Basso, M. F., Arraes, F. B. M., Grossi-de-Sa, M., Moreira, V. J. V., Alves-Ferreira, M., and Grossi-de-Sa, M. F. (2020). Insights into genetic and molecular elements for transgenic crop development. Front. Plant Sci. 11:509. doi: 10.3389/fpls.2020.00509
Beier, S., Thiel, T., Münch, T., Scholz, U., and Mascher, M. (2017). MISA-web: a web server for microsatellite prediction. Bioinformatics 33, 2583–2585. doi: 10.1093/bioinformatics/btx198
Berardini, T. Z., Reiser, L., Li, D., Mezheritsky, Y., Muller, R., Strait, E., et al. (2015). The Arabidopsis information resource: Making and mining the “gold standard” annotated reference plant genome. Genesis 53, 474–485. doi: 10.1002/dvg.22877
Bhowmick, R. (2019). Comprehensive analysis of universal stress proteins and their promoter sequences in rice. Int. J. Curr. Microbiol. App. Sci. 8, 1279–1286. doi: 10.20546/ijcmas.2019.807.152
Bhuria, M., Goel, P., Kumar, S., and Singh, A. K. (2016). The promoter of AtUSP Is Co-regulated by phytohormones and abiotic stresses in Arabidopsis thaliana. Front. Plant Sci. 7:1957. doi: 10.3389/fpls.2016.01957
Bhuria, M., Goel, P., Kumar, S., and Singh, A. K. (2019). Genome-wide identification and expression profiling of genes encoding universal stress proteins (USP) identify multi-stress responsive USP genes in Arabidopsis thaliana. Plant Physiol. Rep. 24, 434–445. doi: 10.1007/s40502-019-00468-6
BIOVIA (2021). Dassault Systèmes. in Discovery Studio Visualizer. v.21.10.20298. San Diego, CA: Dassault Systèmes.
Blom, N., Gammeltoft, S., and Brunak, S. (1999). Sequence- and structure-based prediction of eukaryotic protein phosphorylation sites. J. Mol. Bio. 294, 1351–1362. doi: 10.1006/jmbi.1999.3310
Blom, N., Sicheritz-Ponten, T., Gupta, R., Gammeltoft, S., and Brunak, S. (2004). Prediction of post-translational glycosylation and phosphorylation of proteins from the amino acid sequence. Proteomics 4, 1633–1649. doi: 10.1002/pmic.200300771
Cassidy, C. E., and Setzer, W. N. (2010). Cancer-relevant biochemical targets of cytotoxic Lonchocarpus flavonoids: a molecular docking analysis. J. Mol. Mod. 16, 311–326. doi: 10.1007/s00894-009-0547-5
Chen, C., Chen, H., Zhang, Y., Thomas, H. R., Frank, M. H., He, Y., et al. (2020). TBtools: an integrative toolkit developed for interactive analyses of big biological data. Mol. Plant 13, 1194–1202. doi: 10.1016/j.molp.2020.06.009
Chi, Y. H., Koo, S. S., Oh, H. T., Lee, E. S., Park, J. H., Phan, K. A. T., et al. (2019). The physiological functions of universal stress proteins and their molecular mechanism to protect plants from environmental stresses. Front. Plant Sci. 10:750. doi: 10.3389/fpls.2019.00750
Cohen, P. (2002). The origins of protein phosphorylation. Nat. Cell Biol. 4, E127–E130. doi: 10.1038/ncb0502-e127
Cutillas, P. R. (2017). Targeted in-depth quantification of signaling using label-free mass spectrometry. Methods Enzymol. 585, 245–268. doi: 10.1016/bs.mie.2016.09.021
Dallakyan, S., and Olson, A. J. (2015). Small-Molecule library screening by docking with PyRx. Chem. Biol. 1263, 243–250. doi: 10.1007/978-1-4939-2269-7_19
Emanuelsson, O., Nielsen, H., and Heijne, G. (1999). ChloroP, a neural network-based method for predicting chloroplast transit peptides and their cleavage sites. Protein Sci. 8, 978–984. doi: 10.1110/ps.8.5.978
Finn, R. D., Bateman, A., Clements, J., Coggill, P., Eberhardt, R. Y., Eddy, S. R., et al. (2014). Pfam: the protein families database. Nucleic Acids Res. 42, D222–D230. doi: 10.1093/nar/gkt1223
Freestone, P., Nyström, T., Trinei, M., and Norris, V. (1997). The universal stress protein, UspA, of Escherichia coli is phosphorylated in response to stasis. J. Mol. Bio. 274, 318–324. doi: 10.1006/jmbi.1997.1397
Gabler, F., Nam, S. Z., Till, S., Mirdita, M., Steinegger, M., Söding, J., et al. (2020). Protein sequence analysis using the MPI bioinformatics toolkit. Curr. Protoc. Bioinfor. 72:e108. doi: 10.1002/cpbi.108
Gaut, B. S., Morton, B. R., McCaig, B. C., and Clegg, M. T. (1996). Substitution rate comparisons between grasses and palms: synonymous rate differences at the nuclear gene Adh parallel rate differences at the plastid gene rbcL. Proc. Natl. Acad. Sci. U.S.A. 93, 10274–10279. doi: 10.1073/pnas.93.19.10274
Gou, L., Zhuo, C., Lu, S., and Guo, Z. (2020). A Universal Stress Protein from Medicago falcata (MfUSP1) confers multiple stress tolerance by regulating antioxidant defense and proline accumulation. Environ. Exp. Bot. 178:104168. doi: 10.1016/j.envexpbot.2020.104168
Gupta, R., and Brunak, S. (2002). Prediction of glycosylation across the human proteome and the correlation to protein function. Pac. Symp. Biocomput. 2002, 310–322.
Gutiérrez-Beltrán, E., Personat, J. M., Torre, F., and Pozo, O. D. (2017). A universal stress protein involved in oxidative stress is a phosphorylation target for protein kinase CIPK6. Plant Phy. 173, 836–852. doi: 10.1104/pp.16.00949
Hasan, M. S., Singh, V., Islam, S., Islam, M. S., Ahsan, R., Kaundal, A., et al. (2021). Genome-wide identification and expression profiling of glutathione S-transferase family under multiple abiotic and biotic stresses in Medicago truncatula L. PLoS One 16:e0247170. doi: 10.1371/journal.pone.0247170
Hetényi, C., and van der Spoel, D. (2002). Efficient docking of peptides to proteins without prior knowledge of the binding site. Prot. Sci. 11, 1729–1737. doi: 10.1110/ps.0202302
Horton, P., Park, K. J., Obayashi, T., Fujita, N., Harada, H., Adams-Collier, C. J., et al. (2007). WoLF PSORT: protein localization predictor. Nucl. Acids Res. 35, W585–W587. doi: 10.1093/nar/gkm259
Howe, K. L., Contreras-Moreira, B., De Silva, N., Maslen, G., Akanni, W., Allen, J., et al. (2020). Ensembl Genomes 2020—enabling non-vertebrate genomic research. Nucleic Acids Res. 48, D689–D695. doi: 10.1093/nar/gkz890
Hruz, T., Laule, O., Szabo, G., Wessendorp, F., Bleuler, S., Oertle, L., et al. (2008). Genevestigator v3: a reference expression database for the meta-analysis of transcriptomes. Adv. Bioinform. 2008, 420747. doi: 10.1155/2008/420747
Islam, T., Manna, M., and Kaul, T. (2015). Genome-Wide dissection of Arabidopsis and rice for the identification and expression analysis of glutathione peroxidases reveals their stress-specific and overlapping response patterns. Plant Mol. Biol. Rep. 33, 1413–1427. doi: 10.1007/s11105-014-0846-6
Isokpehi, R. D., Simmons, S. S., Cohly, H. H. P., Ekunwe, S. I. N., Begonia, G. B., and Ayensu, W. K. (2011). Identification of drought-responsive universal stress proteins in Viridiplantae. Bioinform. Biol. Insights 5, 41–58. doi: 10.4137/BBI.S6061
Jung, Y. J., Melencion, S. M. B., Lee, E. S., Park, J. H., Alinapon, C. V., Oh, H. T., et al. (2015). Universal stress protein exhibits a redox-dependent chaperone function in Arabidopsis and enhances plant tolerance to heat shock oxidative stress. Front. Plant Sci. 6:1141. doi: 10.3389/fpls.2015.01141
Katoh, K., Rozewicki, J., and Yamada, K. D. (2019). MAFFT online service: multiple sequence alignment, interactive sequence choice and visualization. Brief. Bioinform. 20, 1160–1166. doi: 10.1093/bib/bbx108
Kim, S., Chen, J., Cheng, T., Gindulyte, A., He, J., He, S., et al. (2021). PubChem in 2021: new data content and improved web interfaces. Nucleic Acids Res. 49, D1388–D1395. doi: 10.1093/nar/gkaa971
Lee, T. H., Tang, H., Wang, X., and Paterson, A. H. (2012). PGDD: a database of gene and genome duplication in plants. Nucleic Acids Res. 41(D1), D1152–D1158. doi: 10.1093/nar/gks1104
Lescot, M. (2002). PlantCARE, a database of plant cis-acting regulatory elements and a portal to tools for in silico analysis of promoter sequences. Nucleic Acids Res. 30, 325–327. doi: 10.1093/nar/30.1.325
Lesk, C., Rowhani, P., and Ramankutty, N. (2016). Influence of extreme weather disasters on global crop production. Nature 529, 84–87. doi: 10.1038/nature16467
Letunic, I., and Bork, P. (2019). Interactive Tree Of Life (iTOL) v4: recent updates and new developments. Nucleic Acids Res. 47, W256–W259. doi: 10.1093/nar/gkz239
Li, W. T., Wei, Y. M., Wang, J. R., Liu, C. J., Lan, X. J., Jiang, Q. T., et al. (2010). Identification, localization, and characterization of putative USP genes in barley. Theor. Appl. Genet. 121, 907–917. doi: 10.1007/s00122-010-1359-9
Livak, K. J., and Schmittgen, T. D. (2001). Analysis of relative gene expression data using real-time quantitative PCR and the 2−ΔΔCT method. Methods 25, 402–408. doi: 10.1006/meth.2001.1262
Loukehaich, R., Wang, T., Ouyang, B., Ziaf, K., Li, H., Zhang, J., et al. (2012). SpUSP, an annexin-interacting universal stress protein, enhances drought tolerance in tomato. J. Exp. Bot. 63, 5593–5606. doi: 10.1093/jxb/ers220
Maqbool, A., Zahur, M., Husnain, T., and Riazuddin, S. (2009). GUSP1 and GUSP2, two drought-responsive genes in Gossypium arboreum have homology to universal stress proteins. Plant Mol. Biol. Rep. 27, 107–114. doi: 10.1007/s11105-008-0049-0
Melencion, S. M. B., Chi, Y. H., Pham, T. T., Paeng, S. K., Wi, S. D., Lee, C., et al. (2017). RNA chaperone function of a universal stress protein in Arabidopsis confers enhanced cold stress tolerance in plants. Int. J. Mol. Sci. 18:2546. doi: 10.3390/ijms18122546
Merkouropoulos, G., and Tsaftaris, A. S. (2013). Differential expression of Gossypium hirsutum USP-related genes, GhUSP1 and GhUSP2, during development and upon salt stress. Plant Mol. Biol. Rep. 31, 1539–1547. doi: 10.1007/s11105-013-0630-z
Minh, B. Q., Schmidt, H. A., Chernomor, O., Schrempf, D., Woodhams, M. D., von Haeseler, A., et al. (2020). Corrigendum to: IQ-TREE 2: new models and efficient methods for phylogenetic inference in the genomic Era. Mol. Bio. Evol. 37, 2461–2461. doi: 10.1093/molbev/msaa131
Mistry, J., Chuguransky, S., Williams, L., Qureshi, M., Salazar, G. A., Sonnhammer, E. L. L., et al. (2020). Pfam: the protein families database in 2021. Nucleic Acids Res. 49, D412–D419. doi: 10.1093/nar/gkaa913
Morris, G. M., Huey, R., Lindstrom, W., Sanner, M. F., Belew, R. K., Goodsell, D. S., et al. (2009). AutoDock4 and AutoDockTools4: automated docking with selective receptor flexibility. J. Comput. Chem. 30, 2785–2791. doi: 10.1002/jcc.21256
Munns, R. (2002). Comparative physiology of salt and water stress: comparative physiology of salt and water stress. Plant, Cell Environ. 25, 239–250. doi: 10.1046/j.0016-8025.2001.00808.x
Nyström, T., and Neidhardt, F. C. (1992). Cloning, mapping and nucleotide sequencing of a gene encoding a universal stress protein in Escherichia coli. Mol. Microbiol. 6, 3187–3198. doi: 10.1111/j.1365-2958.1992.tb01774.x
O’Boyle, N. M., Banck, M., James, C. A., Morley, C., Vandermeersch, T., and Hutchison, G. R. (2011). Open Babel: an open chemical toolbox. J. Cheminform. 3:33. doi: 10.1186/1758-2946-3-33
Ravala, S. K., Singh, S., Yadav, G. S., Kumar, S., Karthikeyan, S., and Chakraborti, P. K. (2015). Evidence that phosphorylation of threonine in the GT motif triggers activation of PknA, a eukaryotic-type serine/threonine kinase from Mycobacterium tuberculosis. FEBS J. 282, 1419–1431. doi: 10.1111/febs.13230
Sakai, H., Lee, S. S., Tanaka, T., Numa, H., Kim, J., Kawahara, Y., et al. (2013). Rice annotation project database (RAP-DB): An integrative and interactive database for rice genomics. Plant Cell Physiol. 54:e6. doi: 10.1093/pcp/pcs183
Sauter, M. (2002). The novel ethylene-regulated gene OsUsp1 from rice encodes a member of a plant protein family related to prokaryotic universal stress proteins. J. Exp. Bot. 53, 2325–2331. doi: 10.1093/jxb/erf096
Shinozaki, K., and Yamaguchi-Shinozaki, K. (2007). Gene networks involved in drought stress response and tolerance. J. Exp. Bot. 58, 221–227. doi: 10.1093/jxb/erl164
Sinha, P., Pazhamala, L. T., Singh, V. K., Saxena, R. K., Krishnamurthy, L., Azam, S., et al. (2016). Identification and validation of selected universal stress protein domain containing drought-responsive genes in pigeon pea (Cajanus cajan L.). Front. Plant Sci. 6:1065. doi: 10.3389/fpls.2015.01065
Smith, S. A., and Dunn, C. W. (2008). Phyutility: a phyloinformatics tool for trees, alignments and molecular data. Bioinformatics 24, 715–716. doi: 10.1093/bioinformatics/btm619
Song, S., Tian, D., Zhang, Z., Hu, S., and Yu, J. (2019). Rice genomics: over the past two decades and into the future. Geno. Prot. Bio. 16, 397–404. doi: 10.1016/j.gpb.2019.01.001
Sousa, M. C., and McKay, D. B. (2001). Structure of the universal stress protein of Haemophilus influenzae. Structure 9, 1135–1141. doi: 10.1016/s0969-2126(01)00680-3
Tian, T., Liu, Y., Yan, H., You, Q., Yi, X., Du, Z., et al. (2017). agriGO v2.0: a GO analysis toolkit for the agricultural community, 2017 update. Nucleic Acids Res. 45, W122–W129. doi: 10.1093/nar/gkx382
Trott, O., and Olson, A. J. (2010). AutoDock Vina: improving the speed and accuracy of docking with a new scoring function, efficient optimization, and multithreading. J. Comput. Chem. 31, 455–461. doi: 10.1002/jcc.21334
Udawat, P., Jha, R. K., Sinha, D., Mishra, A., and Jha, B. (2016). Overexpression of a cytosolic abiotic stress responsive universal stress protein (SbUSP) mitigates salt and osmotic stress in transgenic tobacco plants. Front. Plant Sci. 7:518. doi: 10.3389/fpls.2016.00518
Udawat, P., Mishra, A., and Jha, B. (2014). Heterologous expression of an uncharacterized universal stress protein gene (SbUSP) from the extreme halophyte, Salicornia brachiata, which confers salt and osmotic tolerance to E. coli. Gene 536, 163–170. doi: 10.1016/j.gene.2013.11.020
UniProt Consortium (2021). UniProt: the universal protein knowledgebase in 2021. Nucleic Acids Res. 49, D480–D489. doi: 10.1093/nar/gkaa1100
Vollmer, A. C., and Bark, S. J. (2018). Twenty-Five years of investigating the universal stress protein: function, structure, and applications. Adv. App. Microbiol. 102, 1–36. doi: 10.1016/bs.aambs.2017.10.001
Wang, Y., Tang, H., DeBarry, J. D., Tan, X., Li, J., Wang, X., et al. (2012). MCScanX: a toolkit for detection and evolutionary analysis of gene synteny and collinearity. Nucleic Acids Res. 40:e49. doi: 10.1093/nar/gkr1293
Wu, X., Gong, F., Cao, D., Hu, X., and Wang, W. (2016). Advances in crop proteomics: PTMs of proteins under abiotic stress. Proteomics 16, 847–865. doi: 10.1002/pmic.201500301
Yang, M., Che, S., Zhang, Y., Wang, H., Wei, T., Yan, G., et al. (2019). Universal stress protein in Malus sieversii confers enhanced drought tolerance. J. Plant Res. 132, 825–837. doi: 10.1007/s10265-019-01133-7
Yu, C. S., Chen, Y. C., Lu, C. H., and Hwang, J. K. (2006). Prediction of protein subcellular localization. Proteins 64, 643–651. doi: 10.1002/prot.21018
Zahur, M., Maqbool, A., Ifran, M., Barozai, M. Y., Rashid, B., Riazuddin, S., et al. (2009). Isolation and functional analysis of cotton universal stress protein promoter in response to phytohormones and abiotic stresses. Mol. Biol. 43, 578–585. doi: 10.1134/S0026893309040086
Zarembinski, T. I., Hung, L. W., Mueller-Dieckmann, H. J., Kim, K. K., Yokota, H., Kim, R., et al. (1998). Structure-based assignment of the biochemical function hypothetical protein: a test case of structural genomics. Proc. Natl. Acad. Sci. U.S.A. 95, 15189–15193. doi: 10.1073/pnas.95.26.15189
Zhang, X., Zhuang, L., Liu, Y., Yang, Z., and Huang, B. (2020). Protein phosphorylation associated with drought priming-enhanced heat tolerance in a temperate grass species. Hortic. Res. 7:207. doi: 10.1038/s41438-020-00440-8
Keywords: universal stress proteins, rice, transcript alteration, abiotic stress, functional validation
Citation: Arabia S, Sami AA, Akhter S, Sarker RH and Islam T (2021) Comprehensive in silico Characterization of Universal Stress Proteins in Rice (Oryza sativa L.) With Insight Into Their Stress-Specific Transcriptional Modulation. Front. Plant Sci. 12:712607. doi: 10.3389/fpls.2021.712607
Received: 20 May 2021; Accepted: 07 July 2021;
Published: 28 July 2021.
Edited by:
Luis Fernando Saraiva Macedo Timmers, Universidade do Vale do Taquari - Univates, BrazilReviewed by:
Pandiyan Muthuramalingam, Alagappa University, IndiaCharu Lata, National Institute of Science Communication and Information Resources (CSIR), India
Copyright © 2021 Arabia, Sami, Akhter, Sarker and Islam. This is an open-access article distributed under the terms of the Creative Commons Attribution License (CC BY). The use, distribution or reproduction in other forums is permitted, provided the original author(s) and the copyright owner(s) are credited and that the original publication in this journal is cited, in accordance with accepted academic practice. No use, distribution or reproduction is permitted which does not comply with these terms.
*Correspondence: Tahmina Islam, c3ViYXJuYS5pc2xhbUBnbWFpbC5jb20=; dC5pc2xhbUBkdS5hYy5iZA==
†ORCID: Shatil Arabia, orcid.org/0000-0002-0392-4474; Asif Ahmed Sami, orcid.org/0000-0002-7670-4747; Saima Akhter, orcid.org/0000-0001-6165-4583; Rakha Hari Sarker, orcid.org/0000-0002-6195-8760; Tahmina Islam, orcid.org/0000-0002-0542-0050