- 1Guangxi Key Laboratory for Cultivation and Utilization of Special Non-Timber Forest Crops, Guangxi Engineering and Technology Research Center for Woody Spices, Guangxi Forestry Research Institute, Nanning, China
- 2College of Environmental Sciences and Engineering, Central South University of Forestry and Technology, Changsha, China
- 3Shenzhen Branch, Guangdong Laboratory for Lingnan Modern Agriculture, Genome Analysis Laboratory of the Ministry of Agriculture, Agricultural Genomics Institute at Shenzhen, Chinese Academy of Agricultural Sciences, Shenzhen, China
- 4School of Pharmaceutical Sciences, Sun Yat-sen University, Guangzhou, China
- 5Kunpeng Institute of Modern Agriculture at Foshan, Foshan, China
- 6Virus and Prion Research Unit, National Animal Disease Center, The Agricultural Research Service (ARS) of the United States Department of Agriculture (USDA), Ames, IA, United States
Camphor tree [Cinnamomum camphora (L.) J. Presl], a species in the magnoliid family Lauraceae, is known for its rich volatile oils and is used as a medical cardiotonic and as a scent in many perfumed hygiene products. Here, we present a high-quality chromosome-scale genome of C. camphora with a scaffold N50 of 64.34 Mb and an assembled genome size of 755.41 Mb. Phylogenetic inference revealed that the magnoliids are a sister group to the clade of eudicots and monocots. Comparative genomic analyses identified two rounds of ancient whole-genome duplication (WGD). Tandem duplicated genes exhibited a higher evolutionary rate, a more recent evolutionary history and a more clustered distribution on chromosomes, contributing to the production of secondary metabolites, especially monoterpenes and sesquiterpenes, which are the principal essential oil components. Three-dimensional analyses of the volatile metabolites, gene expression and climate data of samples with the same genotype grown in different locations showed that low temperature and low precipitation during the cold season modulate the expression of genes in the terpenoid biosynthesis pathways, especially TPS genes, which facilitates the accumulation of volatile compounds. Our study lays a theoretical foundation for policy-making regarding the agroforestry applications of camphor tree.
Introduction
Top-geoherbalism, also known as “Daodi” in China and “Provenance” or “Terroir” in Europe, refers to traditional herbs grown in certain native ranges with better quality and efficacy than those grown elsewhere, in which the relevant characteristics are selected and shaped by thousands of years of the clinical application of traditional medicine (Brinckmann, 2015). The concept of top-geoherbalism is documented in the most ancient and classic Chinese Materia Medica (Divine Husbandman’s Classic of Materia Medica; Shen Nong Ben Cao Jing) from approximately 221 B.C. to 220 A.D. (Zhao et al., 2012), which reported that the origin and growing conditions of most herbs were linked to their quality. Historical literature documented cases where the misuse of traditional Chinese medicine (TCM) in prescriptions led to a reduction or absence of therapeutic effects. For example, the application of dried tender shoots of Cinnamomum cassia Presl (a component of Guizhi soup recorded in Prescriptions for Emergencies) from non-top-geoherb regions led to a deficiency in the treatment of fever, while the replacement of this TCM with materials from top-geoherb regions results in proper fever treatment. Currently, TCM from top-geoherb regions accounts for 80% of the market occupancy and economic profits of all TCMs (Huang, 2012), and the significance of top-geoherbs has been revived by the increasing trend of the protection of botanicals with “geographical indication” (GI) (Brinckmann, 2015). Thus, a deep understanding of the pattern and mechanism of top-geoherbalism will strongly guide producers and consumers of TCM and improve the standardization and internalization of the TCM market, especially in the economic context of the Belt and Road Initiative (Hinsley et al., 2020).
A substantial basis of the top-geoherbalism of TCMs is secondary metabolites that play principal roles in the therapeutic effects of herbs. The content of secondary metabolites is a continuous quantitative trait that is determined by three factors: Genotype, environment and the interaction between the genotype and environment. Li Q. et al. (2020) showed that cultivars of opium poppy with similar copy number variations in benzylisoquinoline alkaloid biosynthetic genes were likely to exhibit similar alkaloid contents. To identify the ecological and climatic factors driving the development top-geoherbs, the appropriate strategy is to grow herbs with the same genotype (eliminating the effect of genetic variation) in different environmental settings and study how environmental factors are correlated with secondary metabolites by altering gene expression. However, most published studies confound the effects of genetic variation and environmental factors. For example, Tan et al. (2015) identified metabolite markers for distinguishing Radix Angelica sinensis from top-geoherb regions and non-top-geoherb regions by analyzing the volatile metabolites of processed herbal medicine from drug stores in different regions. The roots of Paeonia veitchii showed higher bioactivities when grown at lower average annual temperatures and high elevations based on the analyses of environmental factors and phytochemicals of different samples from seven populations (Yuan et al., 2020). Liu et al. (2020) revealed a correlation between iridoid accumulation and increased temperature by examining 441 individuals from 45 different origins. The confounding factor of genetic variation led to the misinterpretation of how environmental factors alone affect secondary metabolites. In addition, top-geoherb TCMs have been annotated with their cultural properties, including their cultivation, harvesting and postharvest processing (Huang, 2012), which is outside of the scope of the current study.
Cinnamomum camphora (L.) J. Presl (Figure 1A), also known as camphor tree is native to China, India, Mongolia and Japan (Yoshida et al., 1969; Chen et al., 2017) and was later introduced to Europe and the southern United States (Ravindran et al., 2004; Hamidpour et al., 2012; Bottoni et al., 2021). Camphor trees are divided into five chemotypes according to their dominant volatile oil components as linalool, camphor, eucalyptol, borneol, and isonerolidol types (Luo et al., 2021). Their leaves are especially rich in volatile oil components, including monoterpenes, sesquiterpenes and diterpenes (Hou et al., 2020; Tian et al., 2021). Camphor tree has high medicinal, ornamental, ecological and economic value (Liu et al., 2019; Chen et al., 2020b; Zhang et al., 2020). As a TCM, C. camphora can be used to treat rheumatism and arthralgia, sores and swelling, skin itching, poisonous insect bites, etc. Among the many chemical components of the species, camphor is used as a cardiotonic, deodorant and stabilizer in medicine, daily-use chemical production and industry, and linalool is most frequently used as a scent in 60–80% of perfumed hygiene products and cleaning agents (Letizia et al., 2003; SLI Consulting Inc, 2021).
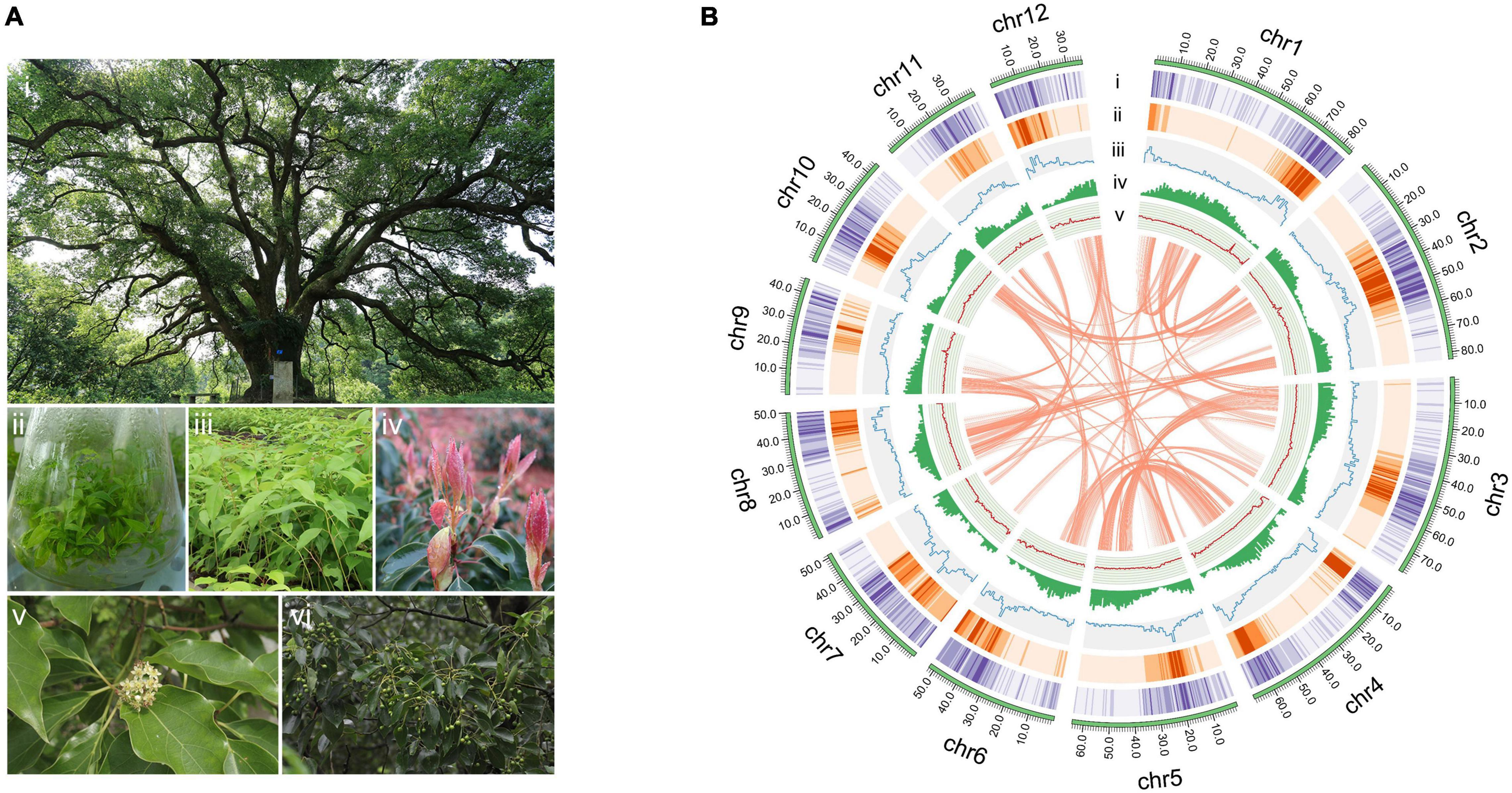
Figure 1. The camphor tree and landscape of its genome. (A) Images of a camphor tree and its multiple tissues. (i) An ancient camphor tree, (ii) tissue-cultured seedings, (iii) seedings, (iv) young leaves, (v) flowers and (vi) fruits. (B) Circos plot of the C. camphora genome assembly. Circles from outside to inside: (i) chromosomes, (ii) Gypsy LTR density, (iii) Copia LTR density, (iii) total LTR density, (iv) gene density and (v) GC content. These density metrics were calculated with 1 Mb non-overlapped sliding windows. The syntenic genomic blocks (> 300 kb) are illustrated with orange lines.
C. camphora belongs to Lauraceae within the magnoliid group comprising four orders (Laurales, Magnoliales, Canellales, and Piperales). Magnoliids are the third largest group of angiosperms, including approximately 9,000–10,000 species (Palmer et al., 2004; Massoni et al., 2015). From an evolutionary point of view, the mysterious phylogenetic position of magnoliids within angiosperms has been debated for decades. Recently, the debate on the phylogenetic position of magnoliids has focused on three main topologies (Moore et al., 2007; Endress and Doyle, 2009; Qiu et al., 2010; Zeng et al., 2014) positioning magnoliids as (a) a sister group to eudicots (Chaw et al., 2019; Chen et al., 2020d; Lv et al., 2020; Shang et al., 2020); (b) a sister group to monocots (Qin et al., 2021); or (c) a sister group to the clade of monocots and eudicots (Chen et al., 2019, 2020c; Hu et al., 2019; Rendon-Anaya et al., 2019). Furthermore, the different whole-genome duplication (WGD) events that have occurred in specific lineages of magnoliids and the divergences time between different magnoliid plants remain unclear (Chaw et al., 2019; Chen et al., 2019, 2020c; Hu et al., 2019; Rendon-Anaya et al., 2019; Lv et al., 2020; Shang et al., 2020; Qin et al., 2021).
Despite the economic and evolutionary value of C. camphora, the lack of a high-quality genome for the species has greatly restricted the progress of genetic research and the identification of the biosynthetic genes underlying the production of essential volatile compounds with medicinal effects (Chaw et al., 2019; Chen et al., 2019, 2020d; Hu et al., 2019; Rendon-Anaya et al., 2019; Lv et al., 2020; Shang et al., 2020). In our study, we de novo assembled the chromosome-scale genome of C. camphora, explored the genomic characteristics of C. camphora and investigated the genetic and climatic factors underlying the top-geoherbalism of this well-known TCM.
Results
Cinnamomum camphora Genome Assembly and Annotation
A haplotype-resolved genome assembly was obtained by using Hifiasm (Cheng et al., 2021) integrating HiFi long reads (24.95 Gb; ∼32.83X based on a previous flow cytometry-based estimated genome size of 760 Mb (Wu et al., 2014)) and Hi-C short reads (63.78 Gb; ∼83.92X) with the default parameters. The two contig-level haplotype genomes showed total sizes of 768.97 and 752.05 Mb, respectively, after removing redundant sequences with Purge_haplotigs (Roach et al., 2018). Compared with the earlier published C. kanehirae genome (Chaw et al., 2019), the contig N50 was 2.01 Mb for haplotype A and 2.25 Mb for haplotype B; these values are ∼2.2- and ∼2.5-fold higher, respectively, than the values of the previously published C. kanehirae genome (Chaw et al., 2019), respectively (Table 1). Benchmarking Universal Single-Copy Ortholog (BUSCO) analyses showed that the contig sets of haplotype A and haplotype B contained 94.1 and 96.0% complete sets of the core orthologous genes of viridiplantae, respectively (Supplementary Table 2). Subsequently, the two non-redundant contig sets were anchored to 12 chromosomes based on Hi-C contacts (Supplementary Figure 1). Overall, the assembled genome size was consistent with previous reports (Table 1 and Supplementary Table 3). As the chromosome-level haplotype A genome was much closer to the estimated genome size than the haplotype B genome, it was used for subsequent analyses.
The final assembled C. camphora genome consisted of 12 chromosomes, 1,025 scaffolds and 1,686 contigs, with a scaffold N50 of 64.34 Mb (Table 1 and Figure 1B). The number of assembled chromosomes was consistent with previous cytological observations.1 The length of the chromosomes ranged from 84.54 (Chr1) to 36.36 Mb (Chr12) (Supplementary Table 5). The high fidelity of the assembly was corroborated by multiple lines of evidence. First, the mapping rates of RNA-Seq paired-end reads against the assembled genome were high (93–95%). Second, the high completeness of this assembly was supported by a 96.2% BUSCO value (Table 1 and Supplementary Table 4), suggesting high completeness at the gene level, which was much better than the completeness of the C. kanehirae genome assembled via PacBio CLR sequencing. Third, the long terminal repeat (LTR) assembly index (LAI) score (Ou and Jiang, 2018) was 13.82, indicating the “reference” level of the genome and reflecting completeness at the transposable element (TE) level.
By combining ab initio prediction, orthologous protein and transcriptomic data, we annotated 24,883 protein-coding genes using the MAKER pipeline (Cantarel et al., 2008; Table 1). The average lengths of the gene regions, coding sequences (CDSs), exon sequences, and intron sequences were 9,295.00, 1,189.59, 309.29, and 1,548.48 bp, respectively (Table 1). Among the predicted protein-coding genes, 97.06% could be annotated in at least one of the following protein-related databases: NCBI non-redundant protein (NR) (97.04%), Swiss-Prot (80.87%), Pfam (72.12%), GO (46.59%) or KEGG (45.08%) (Table 1).
A total of 361.37 Mb of TEs were identified (Supplementary Table 6). Long-terminal repeat retrotransposons (LTR-RTs) were the most abundant type of repetitive sequence, accounting for 46.86% of the whole genome, similar to the proportion of LTRs found in C. kanehirae (Table 1). A total of 3,731 intact LTR-RTs were identified, and the frequency distribution of insertion times showed a burst of LTR-RTs 1–2 million years ago (Mya) (Supplementary Figure 2), consistent with previous reports in magnoliids. Gypsy elements were the largest LTR-RT superfamily in C. camphora, constituting 15.42% of the genome. The second largest superfamily was Copia, accounting for 7.69% of the genome. Other unclassified LTR-RTs encompassed 4.83% of the genome. Among DNA retrotransposons, terminal inverted repeat sequences (TIRs) and non-TIRs comprised 14.05 and 5.87% of the genome, respectively.
Phylogenetic and Whole-Genome Duplication Analyses
The concatenate phylogenetic tree constructed with 172 single-copy orthologous genes among 16 species showed that C. camphora was clustered with two other Lauraceae species and that the formed clade was then clustered with other magnoliids (Figure 2 and Supplementary Figure 3A). More importantly, the magnoliids were sister to the combined clade of eudicots and monocots rather than to either monocots or eudicots. In addition, the coalescence-based phylogenetic tree showed the same topology for magnoliids as the concatenation tree (Supplementary Figure 3B). This phylogenetic topology is consistent with some previous studies (Chen et al., 2019, 2020a; Hu et al., 2019; Rendon-Anaya et al., 2019) but contrasts with other reports (Chaw et al., 2019; Lv et al., 2020; Shang et al., 2020; Qin et al., 2021). The divergence time between magnoliids and the clade of monocots and eudicots was inferred to be 147.47–170.11 Mya (Figure 2) in MCMCTree with fossil calibration (Yang, 2007), coinciding with the estimates in other studies (Chen et al., 2019, 2020c; Hu et al., 2019; Rendon-Anaya et al., 2019). In the clade of magnoliids, the divergence time between Laurales and Magnoliales was approximately 138.96 Mya, after which the divergence of Lauraceae and Calycanthaceae occurred approximately 114.26 Mya, and finally, C. camphora diverged from the most recent common ancestor of C. camphora and C. kanehirae approximately 28.03 Mya.
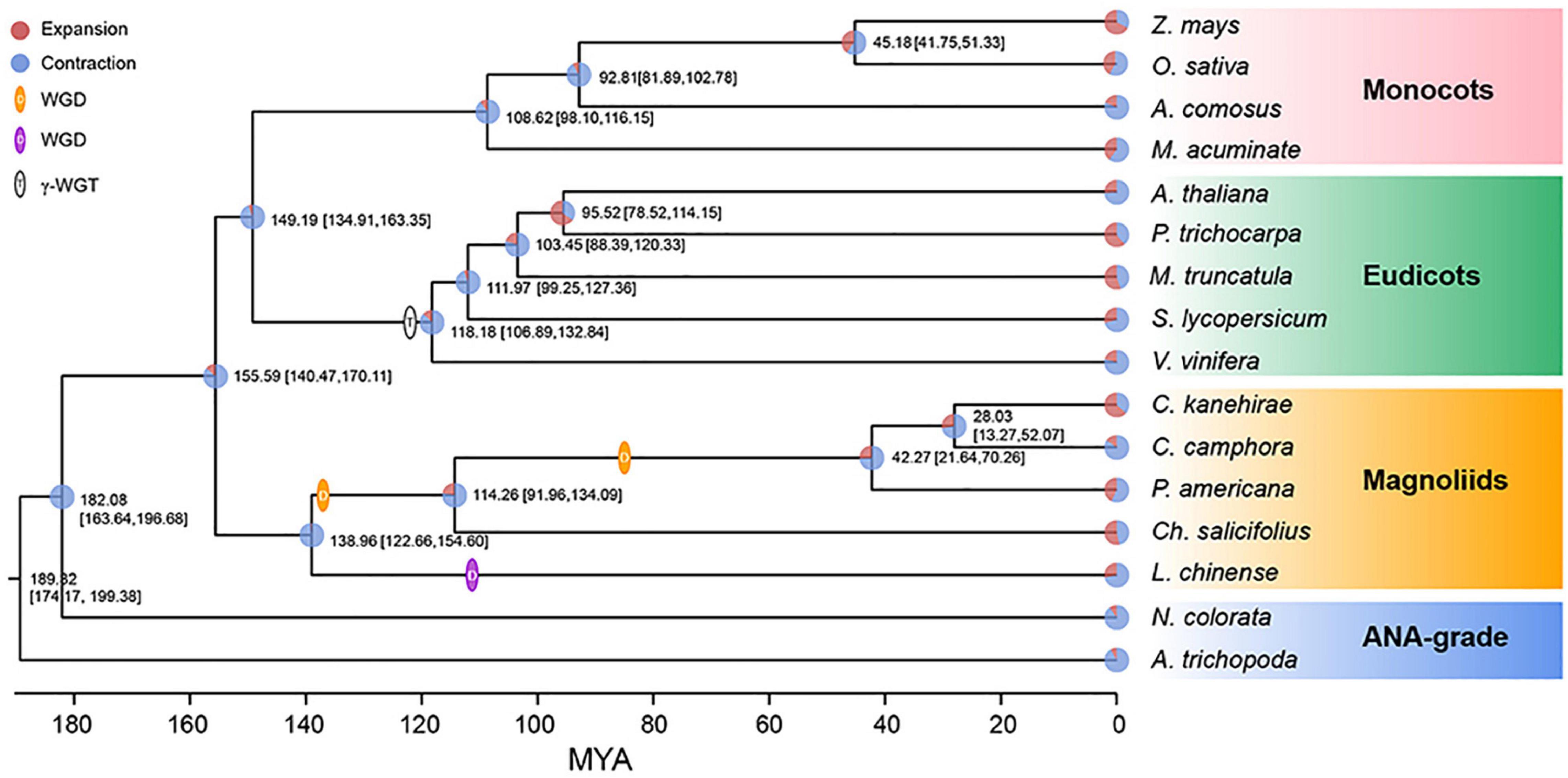
Figure 2. Phylogenetic analyses. The phylogenetic tree was constructed based on 172 single-copy orthologous genes of 16 species using two ANA-grade species as outgroups; node age and 95% confidence intervals are labeled. Pie charts show the proportions of gene families that underwent expansion or contraction. Predicted whole-genome duplication (WGD) events were only indicated for Laurales and Magnoliales.
The intragenomic collinearity and syntenic depth ratio of C. camphora showed clear syntenic evidence of ancient WGD events (Figure 1B and Supplementary Figure 4). Only the paralogous gene set derived from duplicates produced by WGD was used for the analyses of synonymous substitutions per site (Ks) (see “Materials and Methods” section). Two ancient WGD events shared within the Laurales lineage (C. camphora, C. kanehirae and Persea americana) occurred approximately 85.66 Mya and 137.90 Mya, represented by two signature peaks with Ks values of approximately 0.52 and 0.83 (Figure 3A). The recent Ks peak (85.66 Mya) occurred much earlier than the divergence time (42.27 Mya) between P. americana and the common ancestor of Cinnamomum, indicating that this round of WGD was shared between Cinnamomum and Persea (Figures 2, 3A). Additionally, the Ks peak (137.90 Mya) occurred earlier than the divergence (114.26 Mya) between Lauraceae species and Chimonanthus salicifolius, implying that this round of WGD was shared between Lauraceae and Calycanthaceae (Figures 2, 3A). We also detected a WGD event in Liriodendron chinense that occurred approximately 116.67 Mya, corresponding to a Ks peak of 0.70 (Figure 3A), which is consistent with a previous study (Chen et al., 2019).
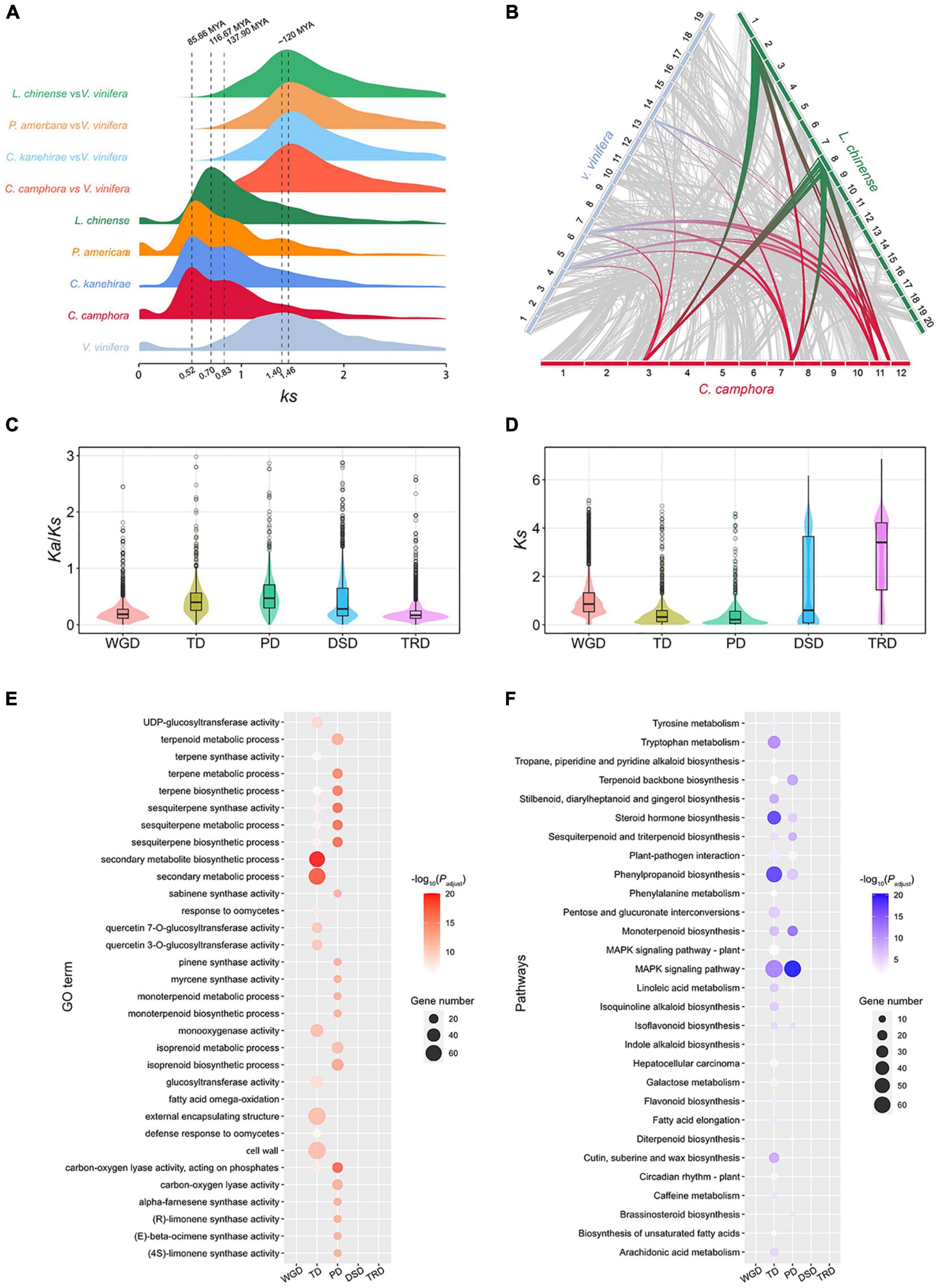
Figure 3. Gene duplication and evolution. (A) Ks distribution of paralogs in magnoliid species (C. camphora, C. kanehirae, P. americana and L. chinense) and orthologs between these magnoliids and V. vinifera. (B) Synteny blocks among C. camphora, L. chinense and V. vinifera. (C) Ka/Ks ratio distributions of gene pairs derived from different types of duplication. WGD, whole-genome duplication; TD, tandem duplication; PD, proximal duplication; TRD, transposed duplication; DSD, dispersed duplication. (D) Ks ratio distributions of gene pairs derived from different types of duplication. (E) GO enrichment analyses of genes from different types of duplication. The enriched GO terms with adjusted P-values < 0.01 are presented. The colors of the bubbles indicate the statistical significance of the enriched GO terms. The sizes of the bubbles indicate the number of genes associated with one GO term. (F) KEGG enrichment analyses of genes resulting from different types of duplication. Enriched KEGG pathways with adjusted P-values < 0.01 are presented. The colors of the bubbles represent the statistical significance of enriched KEGG pathways. The sizes of the bubbles indicate the number of genes associated with one KEGG pathway.
To confirm that C. camphora underwent two rounds of ancient WGD, we performed intergenomic synteny analyses between the genomes of C. camphora and L. chinense together with Vitis vinifera. A “4:2” syntenic relationship was detected between C. camphora and L. chinense (Supplementary Figure 5A). Given the hexaploidy event (γ-WGT) shared among core eudicots, including V. vinifera, an overall “4:3” syntenic relationship was observed between C. camphora and V. vinifera (Supplementary Figure 5B). Furthermore, we discovered a “2:3” syntenic relationship between L. chinense and V. vinifera (Supplementary Figure 5C). Thus, the syntenic relationships among the L. chinense:V. vinifera:C. camphora genomes showed a 2:3:4 ratio (Figure 3B). Our results echoed previous studies (Chaw et al., 2019; Chen et al., 2019), indicating two rounds of ancient WGD in Lauraceae.
To determine the functional roles of the genes retained after WGD events, GO and KEGG enrichment analyses were performed. The results showed that the genes retained after WGD were enriched in basic physiological activities, processes and pathways, such as structural constituents of ribosomes, NADPH dehydrogenase activity, photosynthesis and plant hormone signal transduction, suggesting that two rounds of WGD events enhanced the adaptability of C. camphora to changing environments by improving basic physiological activities and primary metabolism (Supplementary Figures 6, 7 and Supplementary Tables 7, 12).
Tandem and Proximal Duplications Contribute to Terpene Synthesis in Cinnamomum camphora
A total of 18,938 duplicated genes were identified, including 7,043 WGD genes (37.19%), 1,597 tandem duplication (TD) genes (8.43%), 1,079 proximal duplication (PD) genes (5.70%), 5,127 dispersed duplication (DSD) genes (27.07%) and 3,779 transposed duplication (TRD) genes (19.95%) (Supplementary Figure 8). The Ks and Ka/Ks values among different types of duplicated genes were calculated (Figures 3C,D). The Ks values of TD and PD genes were much smaller than those of other types of duplications (Figure 3D), suggesting that TD and PD genes were formed recently. Additionally, the higher Ka/Ks ratios of TD and PD genes (Figure 3C) implies that they were subject to more rapid sequence divergence.
We also performed GO and KEGG enrichment analyses of the gene sets associated with the five duplication types (Supplementary Figures 6, 7 and Supplementary Tables 7–16). The GO results showed that the biological process (BP) and molecular function (MF) categories of secondary metabolite, monoterpene, sesquiterpene and terpene biosynthesis and metabolism were significantly enriched in the TD and PD gene sets, but no enrichment of these GO terms was found in the WGD, DSD and TRD gene sets (Figure 3E). Regarding the KEGG results, the TD and PD gene sets were significantly enriched in the terpenoid backbone, monoterpenoid, sesquiterpenoid and phenylpropanoid biosynthesis pathways. These secondary metabolite biosynthesis pathways were not enriched in the other three duplication gene sets (Figure 3F). In addition, KEGG pathways related to responses to environmental stimuli, such as the MAPK signaling pathway, steroid hormone biosynthesis and plant-pathogen interaction, were also significantly enriched in the TD and PD gene sets. All of these results indicated that the rapidly evolving TD and PD genes played essential roles in the synthesis of secondary metabolites, especially monoterpenes and sesquiterpenes, and the response to environmental stimuli in C. camphora.
Metabolic Reflection of Top-Geoherbalism
To evaluate how environmental factors affect metabolite accumulation, we grew tissue-cultured seedlings from a single mother plant in four major production locations, including Qinzhou, Nanning, Baise and Liuzhou, beginning in May 2018. Mature leaves were harvested from the four locations in November 2020 for RNA-Seq and metabolite assessment. Thus, we were able to compare the effects of environmental conditions on metabolite accumulation based on the fixed genotype.
Volatile metabolites are a substantial basis of the top-geoherbalism of camphor tree. Based on targeted gas chromatography-mass spectrometry (GC-MS) analyses, we identified 153 non-redundant volatile metabolites (Supplementary Table 17). The 153 volatile metabolites included 82 terpenes (53.60%), 14 esters (9.15%), 13 ketones (8.50%), 12 heterocyclic compounds (7.84%), 10 alcohols (6.54%), 9 aromatics (5.88%) and 13 additional metabolites (8.49%) that did not belong to these six main types (Figure 4A). For quality control, we performed hierarchical clustering and principal component analyses (PCA) of metabolic abundances in these 24 samples (Supplementary Figure 9). The first replicate in Baise (“Baise_1”) was identified as an outlier and was removed from further analyses.
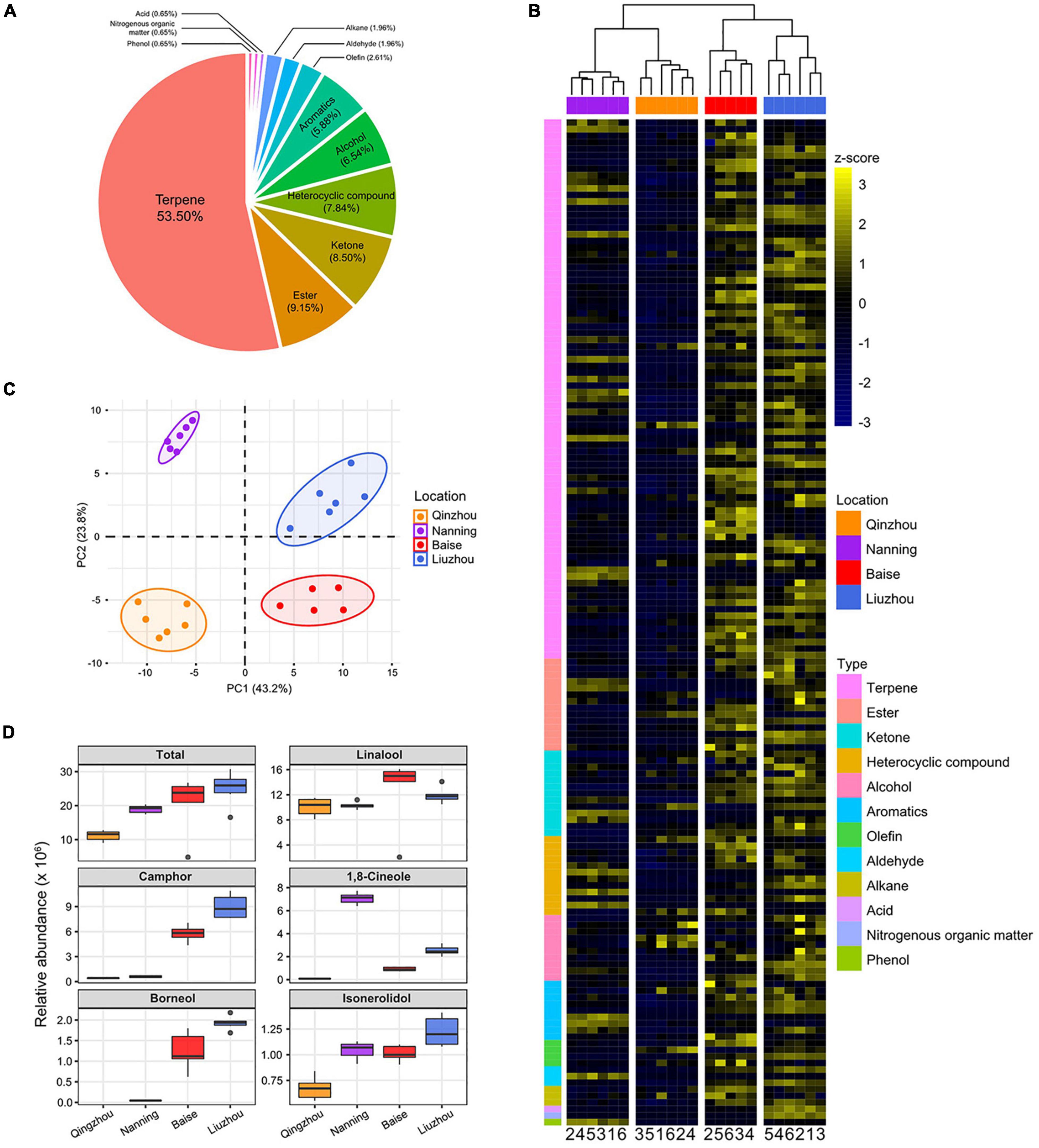
Figure 4. Abundance patterns of volatile metabolites in C. camphora planted in different locations. (A) Pie charts show the proportions of different types of metabolites identified in the current study. (B) Hierarchical clustering heatmap of metabolic abundance profiles in the four planting locations, including Qinzhou, Nanning, Baise and Liuzhou, indicated on the x-axis. Metabolic abundance was averaged and z-score transformed. The rows are clustered by the types of metabolites. (C) Principal component analyses (PCA) of metabolites of C. camphora planted in the four locations. The circles represent the 95% confidence intervals. (D) The relative abundances of linalool, borneol, camphor, 1,8-cineole and isonerolidol in the four planting locations.
The volatile metabolites of the samples from the four locations exhibited distinct clustering in the PCA plot (Figure 4C). Notably, the metabolites of samples from Baise and Liuzhou were clearly separated from those of Qinzhou and Nanning according to principal component 1 (PC1), which accounted for 43.2% of the total variance. The heatmap based on the relative abundances of volatile metabolites showed that samples from Baise and Liuzhou exhibited higher abundances than those from Qinzhou and Nanning, especially with regard to terpenes (Figure 4B and Supplementary Figure 10).
Linalool, borneol, camphor, 1,8-cineole and isonerolidol are the five main phytochemicals determining the medicinal value and top-geoherbalism of C. camphora. A detailed comparison of the five volatile terpenoids implied that samples from Liuzhou showed the highest total abundance, followed by the samples from Baise, while the abundance was lowest in Qinzhou (Figure 4D). The abundance profiling of camphor and borneol in the four locations echoed the trends of the total abundance of the five volatile terpenoids. The abundance of linalool and isonerolidol peaked in Baise and Liuzhou, respectively, while that of 1,8-cineole peaked in Nanning. Taken together, these results suggested that Baise and Liuzhou are top-geoherb regions of the camphor tree in Guangxi Province.
Modulation of Gene Expression Related to Top-Geoherbalism
TPSs are the rate-limiting enzymes in the production of terpenoids (Chen et al., 2011; Tholl, 2015), including monoterpenes, sesquiterpenes and diterpenoids (see “Materials and Methods” section). The identified TPS genes of C. camphora, C. kanehirae, P. americana, L. chinense and Arabidopsis thaliana were clustered into six clades in the phylogenetic tree, corresponding to the TPS-a, TPS-b, TPS-c, TPS-e, TPS-f, and TPS-g subfamilies (Chen et al., 2011), among which TPS-b and TPS-g encode the enzymes catalyzing the production of 10-carbon monoterpenoids from geranyl diphosphate (GPP), and TPS-a genes are responsible for catalyzing the production of 15-carbon sesquiterpenoids from farnesyl diphosphate (FPP) (Figures 5A,B). The copy number variation of the TPS genes showed that TPS-b was greatly expanded in C. kanehirae and C. camphora (Figures 5A,B), which resulted in the high abundance of monoterpenoids in Cinnamomum (Chen et al., 2020d). Specifically, 44 TPS-b genes were identified in C. camphora, accounting for 61% of all its TPS genes, consistent with the percentage observed in C. kanehirae (63%) and much higher than those in L. chinense (33%), A. thaliana (18%) and P. americana (12%). We also observed more copies of the TPS-g subfamily in C. camphora than in A. thaliana and P. americana. However, no expansion of TPS-a genes was detected in Cinnamomum.
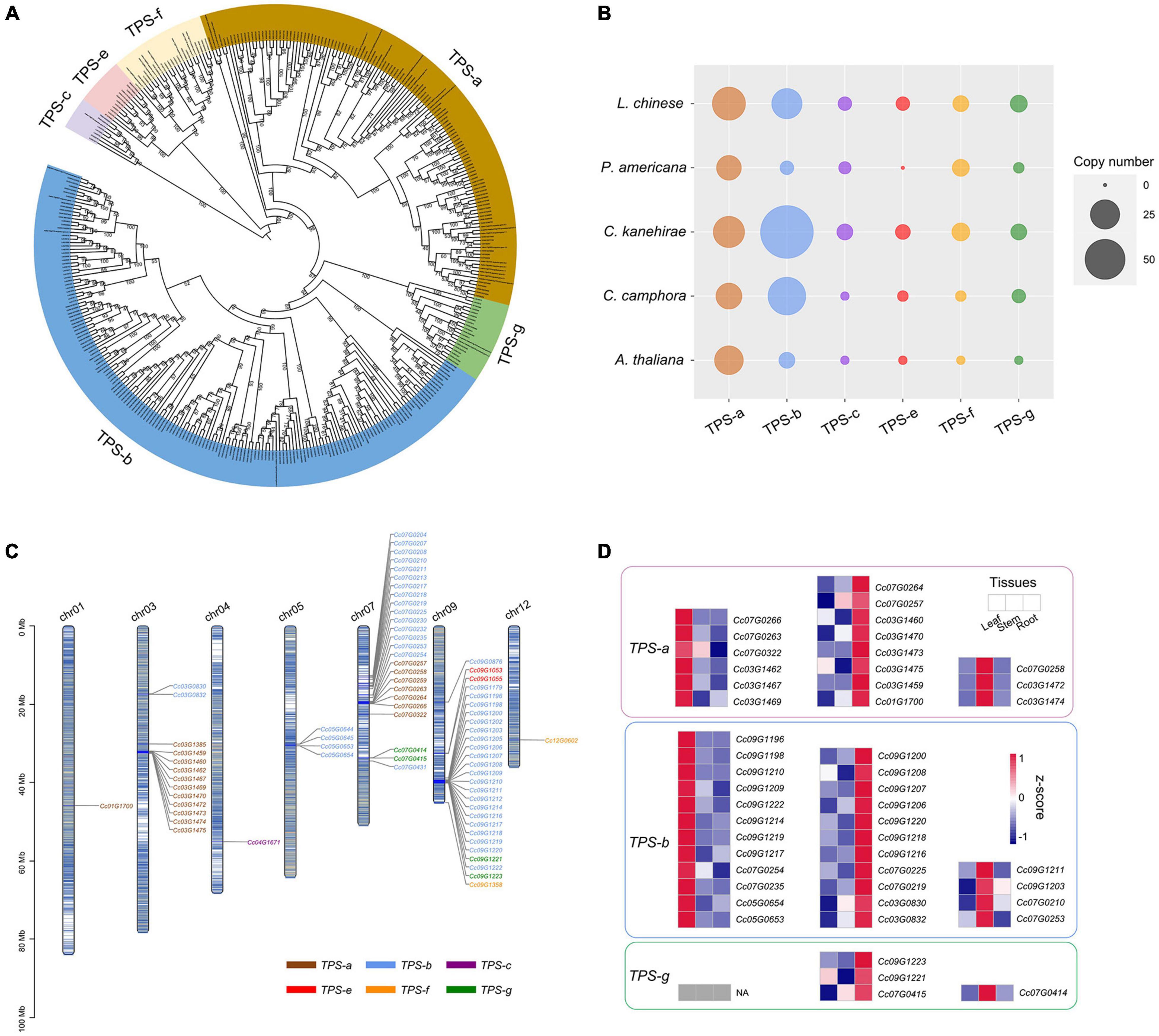
Figure 5. Genes involved in the biosynthesis of volatile terpenoids. (A) Phylogenetic analyses of TPS genes in C. camphora. The phylogenetic tree was constructed based on TPS gene sequences from four magnoliid genomes (C. camphora, C. kanehirae, P. americana and L. chinense) and A. thaliana. (B) Copy numbers of TPS genes in the genomes of four magnoliids and A. thaliana. (C) Distribution of the TPS genes on seven chromosomes of C. camphora. (D) Tissue-specific expressions of TPS-a, TPS-b and TPS-g subfamilies.
Next, we examined how the TPS genes were distributed across the genome. Chromosome 9 and chromosome 7 harbored the most TPS genes (27 and 25, respectively), followed by chromosome 3 (13 TPS genes) (Figure 5C and Supplementary Table 18). Interestingly, genes from the seven subfamilies were observed as tandem duplicates. Two large TPS-b gene clusters were identified on chromosome 9 (21 genes; ca. 38.78–40.12 Mb) and chromosome 7 (10 genes; ca. 12.70–16.03 Mb), respectively. In addition, two large TPS-a gene clusters were detected on chromosome 3 (10 genes; ca. 31.90–32.52 Mb) and chromosome 7 (6 genes; ca. 19.49–19.90 Mb), respectively. The remaining smaller TPS gene clusters were scattered throughout the genome of C. camphora.
Notably, the TPS genes exhibited a strong tissue-specific expression pattern (Figure 5D and Supplementary Table 19), especially TPS-a, TPS-b and TPS-g. We downloaded previously published RNA-Seq data2 from three tissues (leaf, stem and root) of C. camphora to determine the gene expression profile of TPS genes. As leaves are the major tissue used in medicine, we focused on the TPS genes with high expression levels in leaves. Six TPS-a genes and 12 TPS-b genes showed higher expression in leaves than in stems and roots. All of these results indicated that the expansion of the TPS-b subfamily and the tandem duplication of TPS-b genes probably contribute to monoterpenoid biosynthesis in C. camphora.
The biosynthetic pathways of terpenoids are derived from isopentenyl diphosphate (IPP) and dimethylallyl diphosphate (DMAPP) produced via the methylerythritol phosphate (MEP) and mevalonate (MVA) pathways, respectively (Li et al., 2019; Zhou and Pichersky, 2020). Comparative transcriptome analyses of samples from the four locations were performed to examine the expression patterns of genes involved in the MEP and MVA pathways. We combined four differentially expressed gene (DEG) sets identified in Liuzhou vs. Qinzhou, Liuzhou vs. Nanning, Baise vs. Qinzhou and Baise vs. Nanning (Supplementary Figure 11). We detected 22 and 23 DEGs in the MEP and MVA pathways, respectively (Figure 6 and Supplementary Table 20). Generally, the DEGs involved in all the steps of the MEP and MVA pathways showed higher expression in Baise and Liuzhou than in Qinzhou and Nanning, except for the 2-C-methyl-D-erythritol-2,4-cyclodiphosphate synthase (MDS) gene, which was absent in the combined DEG set. The same expression pattern was observed for the genes related to downstream steps, including isopentenyl diphosphate isomerase (IDI), geranyl diphosphate synthetase (GPPS), farnesyl diphosphate synthetase (FPPS), TPS-a, TPS-b and TPS-g. The modulation of gene expression in the terpenoid biosynthesis pathway echoed the higher accumulation of monoterpenoids and sesquiterpenoids observed in Baise and Liuzhou than in Qinzhou and Nanning.
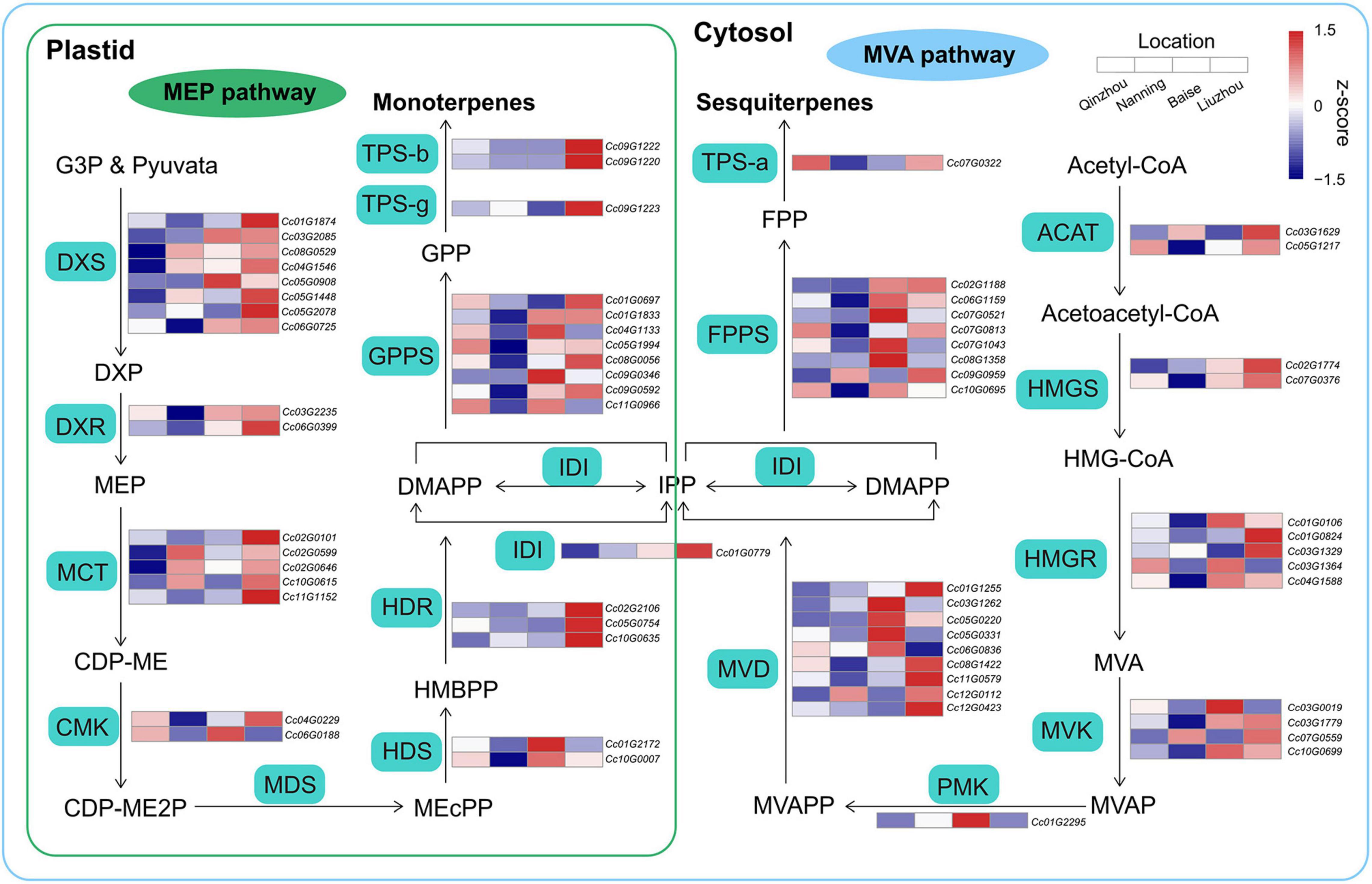
Figure 6. Biosynthetic pathways of monoterpenoids and sesquiterpenoids. Relative expression profiling of genes involved in volatile terpenoid biosynthesis among the four planting locations (Qinzhou, Nanning, Baise and Liuzhou). Gene expression was extracted from the combined differentially expressed gene (DEG) set (Liuzhou vs. Qinzhou, Liuzhou vs. Nanning, Baise vs. Qinzhou and Baise vs. Nanning). MEP, mevalonate pathway; MEP, methylerythritol phosphate pathway; ACAT, acyl-coenzyme A-cholesterol acyl-transferase; HMGS, hydroxymethylglutaryl coenzyme A synthase; HMGR, hydroxymethylglutaryl coenzyme A reductase; MVK, mevalonate kinase; PMK, phospho-mevalonate kinase; MVD, mevalonate diphosphate decarboxylase; DXS, 1-deoxy-D-xylulose 5-phosphate synthase; DXR, 1-deoxy-D-xylulose 5-phosphate reductoisomerase; MCT, 2-C-methyl-D-erythritol-4-phosphate cytidylyltransferase; CMK, 4-(cytidine-5-diphospho)-2-C- methyl-D-erythritol kinase; MDS, 2-C-methyl-D-erythritol-2,4-cyclodiphosphate synthase; HDS, (E)-4-hydroxy-3-methyl-but-2-enyl-pyrophosphate synthase; HDR, (E)-4-hydroxy-3-methyl-but-2-enyl-pyrophosphate reductase.
Climatic Factors Underlying the Top-Geoherbalism of Cinnamomum camphora
To determine what climatic factors caused the differences in volatile metabolites among the C. camphora plants of the same genotype grown in different locations, we downloaded the climate data of Qinzhou, Nanning, Baise and Liuzhou from 3 years (2018–2020) from the National Meteorological Information Centre.3 The 17 climatic factors could be classified into temperature-related, wind-related, pressure-related, precipitation-related, humidity-related and sunshine-related factors (Supplementary Tables 21, 22).
PCAs of the climatic factors showed that PC1 (accounting for 53.2% of the total variance) separated Liuzhou from Qinzhou, Nanning and Baise, while PC2 (accounting for 25.8% of the total variance) split Baise from Qinzhou, Nanning and Liuzhou (Figure 7A). To examine the contributions of climatic factors to PC1 and PC2, we loaded them in the PCA plot (Figure 7B). Temperature-related factors were the main variables contributing to PC1, including mean minimum temperature (16.88%), mean temperature (16.32%) and mean maximum temperature (14.50%) (Figure 7C), and precipitation-related factors mainly contributed to PC2, including daily precipitation (27.29%) and maximum daily precipitation (19.75%) (Figure 7D).
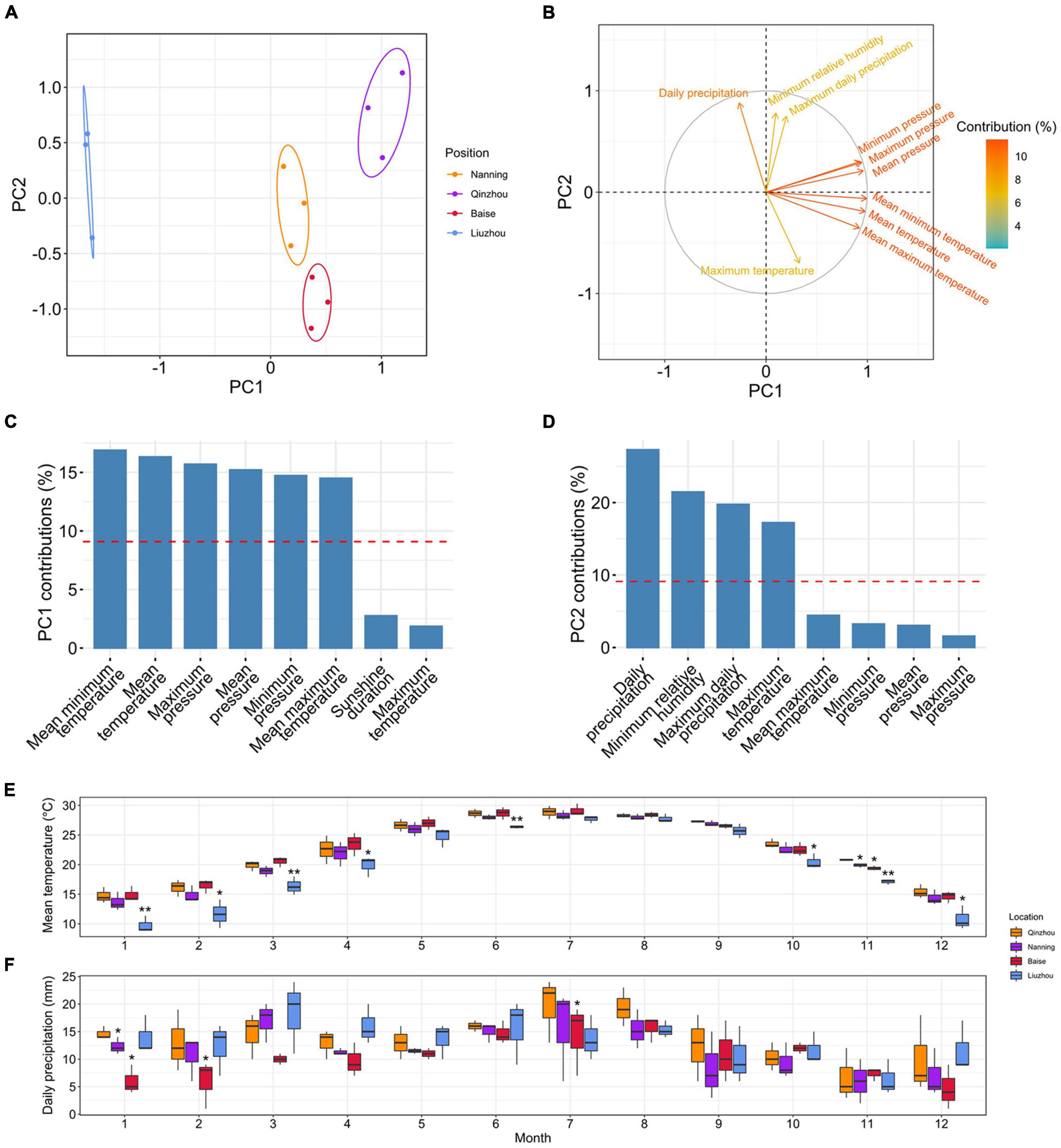
Figure 7. Analyses of climatic factors in different planting locations. (A) Principal component analyses (PCA) of seventeen climatic factors in the four planting locations in 2018, 2019, and 2020. (B) The loadings of climatic factors in the PCA plot. The colors of the arrows represent the percentages of the contributions of climatic factors to the PCs. (C) Histograms of the percentages of the contributions of different climatic factors to PC1. The red dashed lines indicate the average contributions of different climatic factors. Only the top eight climatic factors are shown. (D) Histograms of the percentages of the contributions of different climatic factors to PC2. The red dashed lines indicate the average contributions of different climatic factors. Only the top eight climatic factors are shown. (E) Monthly observations of the mean temperature in the four planting locations. Single and double asterisks indicate statistically significance levels of P < 0.05 and P < 0.01, respectively, between Liuzhou and Qinzhou/Nanning (paired-sample Student’s t-test). (F) Monthly observations of daily precipitation in the four planting locations. A single asterisk indicates the statistically significance levels of P < 0.05 between Baise and Qinzhou/Nanning (paired-sample Student’s t-test).
To examine the detailed differences in temperature- and precipitation-related factors in these four locations, we plotted the monthly observations of mean minimum temperature, mean temperature, mean maximum temperature, daily precipitation and maximum daily precipitation (main contributors to PC1 and PC2) from 2018 to 2020 (Figures 7E,F, Supplementary Figure 12, and Supplementary Table 22). The mean temperature of Liuzhou was much lower than those of the other locations, especially in the two colder quarters of the year. Additionally, daily precipitation was lower in Baise than in the other locations, especially in early spring. All of these results suggested that relatively low temperature and precipitation during the cold season imposes some degree of stress on the plants and thus stimulated the production of the desired terpenoids in C. camphora.
Discussion
Here, by decoding the genome of the camphor tree, we revealed that magnoliids are a sister group to the clade of eudicots and monocots. Chaw et al. (2019) deciphered the first Cinnamomum genome and found that magnoliids is a sister group to eudicot. However, their study was only included one magnoliid species to generate single-copy orthologs and construct the phylogenetic tree. Our sample cohort contains five magnoliids (more magnoliids genome sequences have been published since 2019), and the single-copy orthologs identified are more valid and robust for magnoliids. It is worth mentioning that Qin et al. (2021) found the placement of magnoliids as sister to monocots based on the ancient genomic rearrangements. Taken together, we think the phylogenetic placement of magnoliids is still inconsistent and awaits further investigation. The two rounds of WGD identified in C. camphora were dated to ca. 85.66 and 137.90 Mya, respectively. The former was shared with Lauraceae species, and the latter occurred before the divergence of Lauraceae and Calycanthaceae. We found that rapidly evolving TD genes play key roles in the synthesis of secondary metabolites, especially for monoterpenes and sesquiterpenes. By analyzing volatile metabolites in leaves sampled from plants of the same genotype grown in four different locations, we found higher accumulation of the key volatile metabolites in regions with lower temperature and precipitation in the cold season, which was attributed to the differential expression of genes related to the MVA and MEP pathways as well as their downstream steps in terpene biosynthesis. Our study confirmed that abiotic stress contributes to the development of top-geoherbs, laying a theoretical foundation for policy making on the agroforestry applications of camphor trees.
In addition to releasing the first chromosome-level genome of this significant economic tree species, our study is innovative in two regards. First, it is among the very few innovative three-dimensional evaluations of the top-geoherbalism of a TCM species, integrating phytochemical, genetic and climatic analyses of camphor tree germplasm (Li et al., 2017). Second, our study is the first to fix the genotypic variation of the germplasm so that it is feasible to investigate how climatic factors alone affect the accumulation of metabolites (Li Y. et al., 2020; Mandim et al., 2021). By integrating these two novel approaches, our study provides an example of how to comprehensively scrutinize the genetic and climatic factors affecting the composition of secondary metabolites.
The dominant climatic factors affecting the secondary metabolites of the camphor tree are not always the key factors affecting in other traditional herbs. For example, humidity and sunshine time are the chief limiting factors in the production of artemisinin in Artemisia annua L. (Li et al., 2017). Additionally, lower temperature in the coldest quarter imposes stress on camphor tree and increases the production of desired volatile compounds. In other cases, a higher temperature imposes stress and enhances the production of desired metabolites (Liu et al., 2020). The limiting ecological and climatic factors depended on the native ranges of the herbs and climatic conditions during their growing season (Korner, 2021). A thorough investigation of each specific case would be beneficial for decision making.
Some caveats need to be considered when interpreting our results. First, broader planting of the same genotype (i.e., covering all provinces in South China and even some Southeast Asian countries) would provide a thorough understanding of the optimal climatic variables resulting in the greatest consistency and efficacy of the medicinal components of camphor tree (Sharma et al., 2020). Second, the further experimental verification of the effect of temperature on metabolite contents and the functional validation of selected candidate genes (such as TPS-b, TPS-g, of TPS-a) of the biosynthetic pathway under controlled laboratory conditions would enhance our conclusions (Lau and Sattely, 2015; Nett et al., 2020). Third, soil characteristics (including nutritional status, humidity and rhizosphere microorganisms, etc.) are crucial for the accumulation of secondary metabolites (Ciancio et al., 2019; de Vries et al., 2020). However, owing to the absence of appropriate data for assessing these characteristics, we overlooked ecological factors such as soil conditions. Further studies addressing the abovementioned limitations would provide an in-depth framework for understanding the genetic and environmental factors related to top-geoherbalism.
Taken together, our results lay a theoretical foundation for the optimal production of this economically significant tree species. The distributional range of camphor tree largely overlaps with the land and maritime portions of the silk road of the Belt and Road Initiative (Hinsley et al., 2020); thus, products obtained from camphor tree, such as TCMs or essential oil, show a high chance of being exported to connected countries in the Middle West, Western Europe and even North Africa. As tissue culture methods for camphor trees are well established and the growth rate of the trees is relatively fast (Shi et al., 2010), the commercial cultivation of trees ex situ is probably a sustainable and economic method in addition to importation (Brinckmann, 2015). Thus, our research will provide guidance for policy making, genotype selection and the optimization of climatic conditions for growth by domestic and international government stakeholders, farmers, merchandisers and consumers.
Materials and Methods
Plant Materials
The sequences utilized for de novo genome assembly of the genome were obtained from the fresh leaves of a single camphor tree (C. camphora var. linaloolifera Fujita; NO.95), grown at Guangxi Forestry Research Institute.
Tissue-cultured seedlings were planted in four locations in Guangxi Province in China in May 2018, including Qinzhou (21°58′52”N, 108°39′14”E), Nanning (22°49′00”N, 108°19′39”E), Baise (23°54′09”N, 106°37′06”E) and Liuzhou (24°19′35.0”N, 109°25′41”E). Fresh leaves were collected from the four locations for RNA-Seq analyses and volatile compound quantification in November 2020.
DNA Sequencing
High molecular weight (HMW) genomic DNA was extracted by using a DNeasy Plant Mini Kit (Qiagen, United States), and 50 μg of the HMW DNA was used to generate SMRTbell™ libraries. The circular consensus sequencing (CCS) data were then produced on the PacBio Sequel II platform. Hi-C libraries were constructed from the tender sprouts of C. camphora with fragments labeled with biotin, and then sequenced based on the Illumina NovaSeq 6000 platform.
RNA Sequencing
Total RNA was extracted from each sample by using an RNAprep Pure Plant kit (TIANGEN, Beijing, China). cDNA was synthesized from 20 μg of total RNA using Rever Tra Ace (TOYOBO, Osaka, Japan) with oligo (dT) primers following the user manual. RNA sequencing was also performed on the Illumina NovaSeq 6000 platform.
De novo Genome Assembly and Quality Assessment
The C. camphora genome was assembled by integrating the sequencing data obtained with PacBio CCS and the Hi-C technology using Hifiasm (Cheng et al., 2021). We assembled two contig-level genomes, including a monoploid genome (haplotype A) and an allele-defined genome (haplotype B). Before Hi-C scaffolding, we filtered the redundant contigs from the contig-level genomes by using Purge_haplotigs (Roach et al., 2018). The Hi-C reads were assessed with the HiC-Pro program (Servant et al., 2015). Juicer tools (Durand et al., 2016) and 3D-DNA pipelines (Dudchenko et al., 2017) were used to perform chromosome scaffolding. The BUSCO (Seppey et al., 2019) method was used to evaluate the completeness of the chromosome-level genomes.
Repetitive Sequences and Gene Annotation
We used the EDTA pipeline (Ou et al., 2019) to identify transposable elements in the C. camphora genome, including LTR, TIR and non-TIR elements. LAI assessment and LTR insertion time estimation were performed by LTR_retriever (Ou and Jiang, 2018) with the synonymous substitution rate set as 3.02e-9 (Cui et al., 2006). TRF software (Benson, 1999) with default parameters was applied to annotate tandem repeats.
For gene model annotation, we trained ab initio gene predictors, including AUGUSTUS (Stanke and Morgenstern, 2005) and SNAP (Korf, 2004), on the repeat-masked genome using a combination of protein and transcript data. We used the annotated proteome data of A. thaliana, L. chinense (Chen et al., 2019), C. kanehirae (Chaw et al., 2019) and P. americana (Rendon-Anaya et al., 2019) and the Swiss-Prot database as the protein data. We employed RNA-Seq data from the four locations as the transcript data. To train AUGUSTUS, BRAKER2 (Bruna et al., 2021) was applied with the transcript data from aligned RNA-Seq bam files produced by Hisat2 (Kim et al., 2015). SNAP was trained under MAKER (Cantarel et al., 2008) with two iterations. Transcript data were supplied in the form of a de novo assembled transcriptome generated in Trinity (Haas et al., 2013) and a reference-based assembly generated by StringTie (Pertea et al., 2016). After training, the AUGUSTUS and SNAP results were fed into MAKER again along with all other data to produced synthesized gene models.
Functional annotations of the protein-coding sequences were obtained via BLASTP (“-e-value 1e–10”) searches against entries in both the NR and Swiss-Prot databases. The prediction of gene sequence motifs and structural domains was performed using InterProScan (Jones et al., 2014). The annotations of the GO terms and KEGG pathways of the genes were obtained from eggNOG-mapper (Huerta-Cepas et al., 2017).
Phylogenetic Analyses and Estimation of Divergent Times
A total of 16 plant species, including five magnoliids (C. camphora, C. kanehirae, P. americana, Ch. Salicifolius, and L. chinense), four monocots (Zea mays, Oryza sativa, Ananas comosus, and Musa acuminata), five eudicots (A. thaliana, Populus trichocarpa, Medicago tuncatula, Solanum lycopersicum, and V. vinifera) and two ANA-grade species (Nymphaea colorata and Amborella trichopoda) were used to infer the phylogenetic tree. All genomes except for that of Ch. salicifolius4 were downloaded from JGI5 and Ensembl Plants.6 Paralogs and orthologs were identified among the 16 species by using the OrthoFinder pipeline (Emms and Kelly, 2019) with the default parameters, and the protein sequences of the 172 identified single-copy orthologous genes were used for the construction of the phylogenetic tree. The concatenated amino acid sequences were aligned with MAFFT (Katoh and Standley, 2013) and trimmed with trimAI (Capella-Gutierrez et al., 2009). A maximum likelihood (ML) phylogenetic tree was constructed using IQ-TREE (Nguyen et al., 2015) with ultrafast bootstrapping (–bb 1,000), using N. colorata and A. trichopoda as outgroups. The best-fitting substitution models were selected by ModelFinder (Kalyaanamoorthy et al., 2017). In addition, ASTRAL-III v5.7.3 (Zhang et al., 2018) was applied to infer the coalescence-based species tree with 172 gene trees. The species tree was then used as an input to estimate the divergence time in the MCMCTree program in the PAML package (Yang, 2007). The calibration time was obtained from TimeTree (Kumar et al., 2017): (1) The divergence time of V. vinifera and A. thaliana (107–135 Mya); (2) the divergence time of Z. mays and O. sativa (42–52 Mya); (3) the divergence time of O. sativa and M. acuminata (97–116 Mya); (4) the divergence time of A. trichopoda and A. thaliana (173–199 Mya). The fifth constraint used for time calibration is the fossil record of Magnoliids (110.87–247.2 Mya), cited from Morris et al. (2018). The expansion and contraction of gene families were inferred with CAFÉ (De Bie et al., 2006) based on the chronogram of the 16 species.
Genome Duplication and Syntenic Analyses
To identify the pattern of genome-wide duplications in C. camphora, we divided duplicated genes into five categories, WGD, TD, PD (duplicated genes separated by less than 10 genes on the same chromosome), TRD, and DSD (the remaining duplicates other than the four specified types) gene, using DupGen_Finder (Qiao et al., 2019) with the default parameters. The Ka, Ks, and Ka/Ks values were estimated for duplicated gene pairs based on the YN model in KaKs_Calculator2 (Wang et al., 2010), followed by the conversion of amino acid alignments into the corresponding codon alignments with PAL2NAL (Suyama et al., 2006). The genes in the five duplicate categories were further subjected to GO and KEGG analyses with the R package clusterProfiler (Yu et al., 2012). The enriched items were selected according to an FDR criterion of 0.05. The dating of ancient WGDs and ortholog divergence were estimated based on the formula T = Ks/2r, where Ks refers to the synonymous substitutions per site, and r (3.02e-9) is the synonymous substitution rate for magnoliids (Cui et al., 2006).
Genomic synteny blocks to be employed for intra- and interspecies comparisons among magnoliids were identified with MCscan software (Tang et al., 2008). We performed all-against-all LAST analyses (Kielbasa et al., 2011) and chained the LAST hits according to a distance cut-off of 10 genes, requiring at least five gene pairs per synteny block. The syntenic “depth” function implemented in MCscan was applied to estimate the duplication histories of the respective genomes. Genomic synteny was visualized with the Python version of MCscan, the R package RIdeogram (Hao et al., 2020) and Circos (Krzywinski et al., 2009).
Gene Expression Profiling
The raw RNA-Seq data were filtered by using FASTP (Chen et al., 2018). The clean data were aligned to our assembled C. camphora genome with Hisat2 (Kim et al., 2015), and the quantification of gene expression was calculated with StringTie (Pertea et al., 2016). The Python script preDE.py built into StringTie was used to convert the quantification results into a count matrix. DEGs were detected with the DESeq2 R package (Love et al., 2014) with an FDR < 0.05.
TPS Gene Family
TPS genes are classified into seven subfamilies, including TPS-a, TPS-b, TPS-c, TPS-d, TPS-e, TPS-f, and TPS-g (Chen et al., 2011). The TPS genes included in the TPS-a subfamily are responsible for forming 15-carbon sesquiterpenoids. The TPS-b and TPS-g superfamilies encode the enzymes producing 10-carbon monoterpenoids. The TPS-c, TPS-e, and TPS-f subfamilies encode diterpene synthases, which catalyze the formation of 20-carbon isoprenoids. The TPS-d subfamily is gymnosperm specific and encodes enzymes involved in the production of 20-carbon isoprenoids (Martin et al., 2004). The TPS genes were predicted based on both their conserved domains (PF01397 and PF03936) and BLAST analyses. Conserved domains were used as search queries against the predicted proteome using hmmsearch in HMMER.7 TPS protein sequences from A. thaliana and C. kanehirae were used as queries to identify the TPS genes of C. camphora, P. americana, L. chinense, and V. vinifera. The protein sequence hits of TPS genes were aligned with MAFFT (Katoh and Standley, 2013) and trimmed with trimAI (Capella-Gutierrez et al., 2009). The TPS gene tree was constructed using RAxML (Stamatakis, 2014) with 1,000 bootstrap replicates. The TPS-c subfamily was designated as the outgroup. The distribution of TPS genes on the chromosomes was visualized in TBtools (Chen et al., 2020a).
Identification of Genes Involved in the Mevalonate and Methylerythritol Phosphate Pathways
The MVA pathway involves six gene families, including the acyl-coenzyme A-cholesterol acyl-transferase (ACAT), hydroxymethylglutaryl coenzyme A synthase (HMGS), hydroxymethylglutaryl coenzyme A reductase (HMGR), mevalonate kinase (MVK), phospho-mevalonate kinase (PMK), and mevalonate diphosphate decarboxylase (MVD) genes. Seven gene families are involved in the MEP pathway, including the 1-deoxy-D-xylulose 5-phosphate synthase (DXS), 1-deoxy-D-xylulose 5-phosphate reductoisomerase (DXR), 2-C-methyl-D-erythritol-4-phosphate cytidylyltransferase (MCT), 4-(cytidine-5-diphospho)-2-C-methyl-D-erythritol kinase (CMK), MDS, (E)-4-hydroxy-3-methyl-but-2-enyl-pyrophosphate synthase (HDS), and (E)-4-hydroxy-3-methyl-but-2-enyl-pyrophosphate reductase (HDR) genes. The genes in both the MVA and MEP pathways are well documented in the model plants. To identify candidate genes related to the two pathways in the C. camphora genome, we collected the protein sequences of genes in the MVA and MEP pathways identified in A. thaliana. Using each A. thaliana gene as a query sequence, BLASTP (“-e-value 1e-10”) analyses was performed to identify orthologous genes in C. camphora.
Determination of Volatile Metabolites
Six biological replicates were sampled in each location. The samples were ground into powder in liquid nitrogen. Two grams of the powder was transferred to a 20 ml headspace vial. The vials were sealed using crimp-top caps with TFE-silicone headspace septa. In solid-phase microextraction (SPME) analyses, each vial was placed at 60°C for 12 min, and a 65 μm divinylbenzene/carboxene/polydimethylsilioxane fiber (Supelco, Bellefonte, PA, United States) was then exposed to the headspace of the sample for 30 min at 60°C. The desorption of the volatile metabolites from the fiber coating was carried out in the injection port of the GC apparatus at 250°C for 10 min in spitless mode. The identification and quantification of volatile metabolites were carried out with a 30 m x 0.25 mm × 1.0 μm DB-5MS (5% phenyl-polymethylsiloxane) capillary column based on the Agilent 7890B-7000D platform. Helium was used as the carrier gas at a velocity of 1.0 ml/min. The temperature of oven was programmed to increase from 40 at 5°C/min until it arrived at 280°C. The quadrupole mass detector, ion source and transfer line temperatures were set at 150, 230, and 280°C, respectively.
Climatic Factor Collection and Analyses
Data on 17 climatic factors from Qinzhou, Nanning, Baise and Liuzhou from 2018 to 2020 were downloaded from the National Meteorological Information Centre (see footnote 3). The analyses of the climatic factors were performed in the R package FactoMineR (Lê et al., 2008).
Data Availability Statement
The datasets presented in this study can be found in online repositories. The names of the repository/repositories and accession number(s) can be found in the article/Supplementary Material.
Author Contributions
LW, CL, KL, and RJ conceived and designed the study. RJ, XC, CZ, PW, and KL prepared the materials. CL, XC, XL, DP, and XH performed data analyses. RJ, XC, DH, CL, and LW wrote the manuscript. All authors read and approved the final draft.
Funding
This study was supported by the National Key Research and Development Program of China (Grant No. 2020YFA0907900), the Genomic Studies on the Differences in the Secondary Metabolism of Camphor Tree under Different Site Conditions (Grant Nos. JA-20-04-07 and 202102), the National Natural Science Foundation of China (Grant No. 32070242), the Shenzhen Science and Technology Program (Grant No. KQTD2016113010482651), special funds for Science Technology Innovation and Industrial Development of Shenzhen Dapeng New District (Grant Nos. RC201901-05 and PT201901-19), the China Postdoctoral Science Foundation (Grant No. 2020M672904), the Basic and Applied Basic Research Fund of Guangdong (Grant No. 2020A1515110912), and the USDA Agricultural Research Service Research Participation Program of the Oak Ridge Institute for Science and Education (ORISE) (Grant No. DE-AC05-06OR23100).
Conflict of Interest
The authors declare that the research was conducted in the absence of any commercial or financial relationships that could be construed as a potential conflict of interest.
Publisher’s Note
All claims expressed in this article are solely those of the authors and do not necessarily represent those of their affiliated organizations, or those of the publisher, the editors and the reviewers. Any product that may be evaluated in this article, or claim that may be made by its manufacturer, is not guaranteed or endorsed by the publisher.
Supplementary Material
The Supplementary Material for this article can be found online at: https://www.frontiersin.org/articles/10.3389/fpls.2022.827890/full#supplementary-material
Footnotes
- ^ http://ccdb.tau.ac.il
- ^ https://www.ncbi.nlm.nih.gov/bioproject/PRJNA747104
- ^ http://data.cma.cn/en
- ^ http://xhhuanglab.cn/data/Chimonanthus_salicifolius.html
- ^ https://phytozome-next.jgi.doe.gov/pz/portal.html
- ^ http://plants.ensembl.org/info/data/ftp/index.html
- ^ https://www.ebi.ac.uk/Tools/hmmer/
References
Benson, G. (1999). Tandem repeats finder: a program to analyze DNA sequences. Nucleic Acids Res. 27, 573–580. doi: 10.1093/nar/27.2.573
Bottoni, M., Milani, F., Mozzo, M., Radice Kolloffel, D. A., Papini, A., Fratini, F., et al. (2021). Sub-Tissue localization of phytochemicals in Cinnamomum camphora (L.) J. Presl. growing in Northern Italy. Plants 10:1008. doi: 10.3390/plants10051008
Brinckmann, J. A. (2015). Geographical indications for medicinal plants: Globalization, climate change, quality and market implications for Geo-Authentic botanicals. World J. Trad. Chinese Med. 1, 16–23. doi: 10.15806/j.issn.2311-8571.2014.0020
Bruna, T., Hoff, K. J., Lomsadze, A., Stanke, M., and Borodovsky, M. (2021). BRAKER2: Automatic eukaryotic genome annotation with GeneMark-EP+ and AUGUSTUS supported by a protein database. NAR Genom. Bioinform. 3:a108. doi: 10.1093/nargab/lqaa108
Cantarel, B. L., Korf, I., Robb, S. M., Parra, G., Ross, E., Moore, B., et al. (2008). MAKER: An easy-to-use annotation pipeline designed for emerging model organism genomes. Genome Res. 18, 188–196. doi: 10.1101/gr.6743907
Capella-Gutierrez, S., Silla-Martinez, J. M., and Gabaldon, T. (2009). TrimAl: A tool for automated alignment trimming in large-scale phylogenetic analyses. Bioinformatics 25, 1972–1973. doi: 10.1093/bioinformatics/btp348
Chaw, S., Liu, Y., Wu, Y., Wang, H., Lin, C. I., Wu, C. S., et al. (2019). Stout camphor tree genome fills gaps in understanding of flowering plant genome evolution. Nat. Plants 5, 63–73. doi: 10.1038/s41477-018-0337-0
Chen, C., Zheng, Y., Liu, S., Zhong, Y., Wu, Y., Li, J., et al. (2017). The complete chloroplast genome of Cinnamomum camphora and its comparison with related Lauraceae species. PeerJ 5:e3820. doi: 10.7717/peerj.3820
Chen, C., Zhong, Y., Yu, F., and Xu, M. (2020b). Deep sequencing identifies miRNAs and their target genes involved in the biosynthesis of terpenoids in Cinnamomum camphora. Ind. Crop Prod. 145:111853. doi: 10.1016/j.indcrop.2019.111853
Chen, Y., Li, Z., Zhao, Y., Gao, M., Wang, J., Liu, K. W., et al. (2020d). The Litsea genome and the evolution of the laurel family. Nat. Commun. 11:1675. doi: 10.1038/s41467-020-15493-5
Chen, S., Sun, W., Xiong, Y., Jiang, Y., Liu, X., Liao, X. Y., et al. (2020c). The Phoebe genome sheds light on the evolution of magnoliids. Hortic. Res. 7:146. doi: 10.1038/s41438-020-00368-z
Chen, C., Chen, H., Zhang, Y., Thomas, H. R., Frank, M. H., He, Y., et al. (2020a). TBtools: An integrative toolkit developed for interactive analyses of big biological data. Mol. Plant 13, 1194–1202. doi: 10.1016/j.molp.2020.06.009
Chen, F., Tholl, D., Bohlmann, J., and Pichersky, E. (2011). The family of terpene synthases in plants: a mid-size family of genes for specialized metabolism that is highly diversified throughout the kingdom. Plant J. 66, 212–229. doi: 10.1111/j.1365-313X.2011.04520.x
Chen, J., Hao, Z., Guang, X., Zhao, C., Wang, P., Xue, L., et al. (2019). Liriodendron genome sheds light on angiosperm phylogeny and species-pair differentiation. Nat. Plants 5, 18–25. doi: 10.1038/s41477-018-0323-6
Chen, S., Zhou, Y., Chen, Y., and Gu, J. (2018). Fastp: An ultra-fast all-in-one FASTQ preprocessor. Bioinformatics 34, i884–i890. doi: 10.1093/bioinformatics/bty560
Cheng, H., Concepcion, G. T., Feng, X., Zhang, H., and Li, H. (2021). Haplotype-resolved de novo assembly using phased assembly graphs with hifiasm. Nat. Methods 18, 170–175. doi: 10.1038/s41592-020-01056-5
Ciancio, A., Pieterse, C., and Mercado-Blanco, J. (2019). Editorial: harnessing useful rhizosphere microorganisms for pathogen and pest biocontrol – second edition. Front. Microbiol. 10:1935. doi: 10.3389/fmicb.2019.01935
Cui, L., Wall, P. K., Leebens-Mack, J. H., Lindsay, B. G., Soltis, D. E., Doyle, J. J., et al. (2006). Widespread genome duplications throughout the history of flowering plants. Genome Res. 16, 738–749. doi: 10.1101/gr.4825606
De Bie, T., Cristianini, N., Demuth, J. P., and Hahn, M. W. (2006). CAFE: A computational tool for the study of gene family evolution. Bioinformatics 22, 1269–1271. doi: 10.1093/bioinformatics/btl097
de Vries, F. T., Griffiths, R. I., Knight, C. G., Nicolitch, O., and Williams, A. (2020). Harnessing rhizosphere microbiomes for drought-resilient crop production. Science 368, 270–274. doi: 10.1126/science.aaz5192
Dudchenko, O., Batra, S. S., Omer, A. D., Nyquist, S. K., Hoeger, M., Durand, N. C., et al. (2017). De novo assembly of the Aedes aegypti genome using Hi-C yields chromosome-length scaffolds. Science 356, 92–95. doi: 10.1126/science.aal3327
Durand, N. C., Shamim, M. S., Machol, I., Rao, S. S., Huntley, M. H., Lander, E. S., et al. (2016). Juicer provides a one-click system for analyzing loop-resolution hi-c experiments. Cell Syst. 3, 95–98. doi: 10.1016/j.cels.2016.07.002
Emms, D. M., and Kelly, S. (2019). OrthoFinder: phylogenetic orthology inference for comparative genomics. Genome Biol. 20:238. doi: 10.1186/s13059-019-1832-y
Endress, P. K., and Doyle, J. A. (2009). Reconstructing the ancestral angiosperm flower and its initial specializations. Am. J. Bot. 96, 22–66. doi: 10.3732/ajb.0800047
Haas, B. J., Papanicolaou, A., Yassour, M., Grabherr, M., Blood, P. D., Bowden, J., et al. (2013). De novo transcript sequence reconstruction from RNA-seq using the Trinity platform for reference generation and analysis. Nat. Protoc. 8, 1494–1512. doi: 10.1038/nprot.2013.084
Hamidpour, R., Hamidpour, S., Hamidpour, M., and Shahlari, M. (2012). Camphor (Cinnamomum camphora), a traditional remedy with the history of treating several diseases. Int. J. Case Rep. Images 4:86. doi: 10.5348/ijcri-2013-02-267-RA-1
Hao, Z., Lv, D., Ge, Y., Shi, J., Weijers, D., Yu, G., et al. (2020). RIdeogram: Drawing SVG graphics to visualize and map genome-wide data on the idiograms. PeerJ Comput. Sci. 6:e251. doi: 10.7717/peerj-cs.251
Hinsley, A., Milner-Gulland, E. J., Cooney, R., Timoshyna, A., Ruan, X., and Lee, T. M. (2020). Building sustainability into the belt and road initiative’s traditional chinese medicine trade. Nat. Sustain. 3, 96–100. doi: 10.1038/s41893-019-0460-6
Hou, J., Zhang, J., Zhang, B., Jin, X., Zhang, H., and Jin, Z. (2020). Transcriptional analysis of metabolic pathways and regulatory mechanisms of essential oil biosynthesis in the leaves of Cinnamomum camphora (L.) presl. Front. Genet. 11:598714. doi: 10.3389/fgene.2020.598714
Hu, L., Xu, Z., Wang, M., Fan, R., Yuan, D., Wu, B., et al. (2019). The chromosome-scale reference genome of black pepper provides insight into piperine biosynthesis. Nat. Commun. 10, 4702. doi: 10.1038/s41467-019-12607-6
Huerta-Cepas, J., Forslund, K., Coelho, L. P., Szklarczyk, D., Jensen, L. J., von Mering, C., et al. (2017). Fast genome-wide functional annotation through orthology assignment by eggnog-mapper. Mol. Biol. Evol. 34, 2115–2122. doi: 10.1093/molbev/msx148
Jones, P., Binns, D., Chang, H. Y., Fraser, M., Li, W., McAnulla, C., et al. (2014). InterProScan 5: genome-scale protein function classification. Bioinformatics 30, 1236–1240. doi: 10.1093/bioinformatics/btu031
Kalyaanamoorthy, S., Minh, B. Q., Wong, T., von Haeseler, A., and Jermiin, L. S. (2017). ModelFinder: fast model selection for accurate phylogenetic estimates. Nat. Methods 14, 587–589. doi: 10.1038/nmeth.4285
Katoh, K., and Standley, D. M. (2013). MAFFT multiple sequence alignment software version 7: Improvements in performance and usability. Mol. Biol. Evol. 30, 772–780. doi: 10.1093/molbev/mst010
Kielbasa, S. M., Wan, R., Sato, K., Horton, P., and Frith, M. C. (2011). Adaptive seeds tame genomic sequence comparison. Genome Res. 21, 487–493. doi: 10.1101/gr.113985.110
Kim, D., Langmead, B., and Salzberg, S. L. (2015). HISAT: a fast spliced aligner with low memory requirements. Nat. Methods 12, 357–360. doi: 10.1038/nmeth.3317
Korf, I. (2004). Gene finding in novel genomes. BMC Bioinformatics 5:59. doi: 10.1186/1471-2105-5-59
Korner, C. (2021). The cold range limit of trees. Trends Ecol. Evol. 36, 979–989. doi: 10.1016/j.tree.2021.06.011
Krzywinski, M., Schein, J., Birol, I., Connors, J., Gascoyne, R., Horsman, D., et al. (2009). Circos: an information aesthetic for comparative genomics. Genome Res. 19, 1639–1645. doi: 10.1101/gr.092759.109
Kumar, S., Stecher, G., Suleski, M., and Hedges, S. B. (2017). TimeTree: a resource for timelines, timetrees, and divergence times. Mol. Biol. Evol. 34, 1812–1819. doi: 10.1093/molbev/msx116
Lau, W., and Sattely, E. S. (2015). Six enzymes from mayapple that complete the biosynthetic pathway to the etoposide aglycone. Science 349, 1224–1228. doi: 10.1126/science.aac7202
Lê, S., Josse, J., and Husson, F. (2008). FactoMineR: an R package for multivariate analysis. J. Stat. Softw. 25, 1–18.
Letizia, C. S., Cocchiara, J., Lalko, J., and Api, A. M. (2003). Fragrance material review on linalool. Food Chem. Toxicol. 41, 943–964. doi: 10.1016/S0278-6915(03)00015-2
Li, C., He, Q., Zhang, F., Yu, J., Li, C., Zhao, T., et al. (2019). Melatonin enhances cotton immunity to Verticillium wilt via manipulating lignin and gossypol biosynthesis. Plant J. 100, 784–800. doi: 10.1111/tpj.14477
Li, L., Josef, B. A., Liu, B., Zheng, S., Huang, L., and Chen, S. (2017). Three-Dimensional evaluation on ecotypic diversity of traditional Chinese medicine: a case study of Artemisia annua L. Front. Plant Sci. 8:1225. doi: 10.3389/fpls.2017.01225
Li, Q., Ramasamy, S., Singh, P., Hagel, J. M., Dunemann, S. M., Chen, X., et al. (2020). Gene clustering and copy number variation in alkaloid metabolic pathways of opium poppy. Nat. Commun. 11:1190. doi: 10.1038/s41467-020-15040-2
Li, Y., Kong, D., Fu, Y., Sussman, M. R., and Wu, H. (2020). The effect of developmental and environmental factors on secondary metabolites in medicinal plants. Plant Physiol. Biochem. 148, 80–89. doi: 10.1016/j.plaphy.2020.01.006
Liu, L., Zuo, Z., Xu, F., and Wang, Y. (2020). Study on quality response to environmental factors and geographical traceability of wild Gentiana rigescens Franch. Front. Plant Sci. 11:1128. doi: 10.3389/fpls.2020.01128
Liu, X., Meng, Y., Zhang, Z., Wang, Y., Geng, X., Li, M., et al. (2019). Functional nano-catalyzed pyrolyzates from branch of Cinnamomum camphora. Saudi J. Biol. Sci. 26, 1227–1246. doi: 10.1016/j.sjbs.2019.06.003
Love, M. I., Huber, W., and Anders, S. (2014). Moderated estimation of fold change and dispersion for RNA-seq data with DESeq2. Genome Biol. 15:550. doi: 10.1186/s13059-014-0550-8
Luo, Q., Xu, C., Zheng, T., Ma, Y., Li, Y., and Zuo, Z. (2021). Leaf morphological and photosynthetic differences among four chemotypes of Cinnamomum camphora in different seasons. Ind. Crop Prod. 169, 113651. doi: 10.1016/j.indcrop.2021.113651
Lv, Q., Qiu, J., Liu, J., Li, Z., Zhang, W., Wang, Q., et al. (2020). The Chimonanthus salicifolius genome provides insight into magnoliid evolution and flavonoid biosynthesis. Plant J. 103, 1910–1923. doi: 10.1111/tpj.14874
Mandim, F., Petropoulos, S. A., Dias, M. I., Pinela, J., Kostic, M., Soković, M., et al. (2021). Seasonal variation in bioactive properties and phenolic composition of cardoon (Cynara cardunculus var. Altilis) bracts. Food Chem. 33:127744. doi: 10.1016/j.foodchem.2020.127744
Martin, D. M., Faldt, J., and Bohlmann, J. (2004). Functional characterization of nine Norway Spruce TPS genes and evolution of gymnosperm terpene synthases of the TPS-d subfamily. Plant Physiol. 135, 1908–1927. doi: 10.1104/pp.104.042028
Massoni, J., Couvreur, T. L., and Sauquet, H. (2015). Five major shifts of diversification through the long evolutionary history of Magnoliidae (angiosperms). BMC Evol. Bio. 15:49. doi: 10.1186/s12862-015-0320-6
Moore, M. J., Bell, C. D., Soltis, P. S., and Soltis, D. E. (2007). Using plastid genome-scale data to resolve enigmatic relationships among basal angiosperms. Proc. Natl. Acad. Sci. U.S.A. 104, 19363–19368. doi: 10.1073/pnas.0708072104
Morris, J. L., Puttick, M. N., Clark, J. W., Edwards, D., Kenrick, P., Pressel, S., et al. (2018). Timescale of early land plant evolution. Proc. Natl. Acad. Sci. U.S.A. 115, E2274–E2283. doi: 10.1073/pnas.1719588115
Nett, R. S., Lau, W., and Sattely, E. S. (2020). Discovery and engineering of colchicine alkaloid biosynthesis. Nature 584, 148–153. doi: 10.1038/s41586-020-2546-8
Nguyen, L. T., Schmidt, H. A., von Haeseler, A., and Minh, B. Q. (2015). IQ-TREE: A fast and effective stochastic algorithm for estimating maximum-likelihood phylogenies. Mol. Biol. Evol. 32, 268–274. doi: 10.1093/molbev/msu300
Ou, S., and Jiang, N. (2018). LTR_retriever: a highly accurate and sensitive program for identification of long terminal repeat retrotransposons. Plant Physiol. 176, 1410–1422. doi: 10.1104/pp.17.01310
Ou, S., Su, W., Liao, Y., Chougule, K., Agda, J., Hellinga, A. J., et al. (2019). Benchmarking transposable element annotation methods for creation of a streamlined, comprehensive pipeline. Genome Biol. 20:275. doi: 10.1186/s13059-019-1905-y
Palmer, J. D., Soltis, D. E., and Chase, M. W. (2004). The plant tree of life: An overview and some points of view. Am. J. Bot. 91, 1437–1445. doi: 10.3732/ajb.91.10.1437
Pertea, M., Kim, D., Pertea, G. M., Leek, J. T., and Salzberg, S. L. (2016). Transcript-level expression analysis of RNA-seq experiments with HISAT, StringTie and Ballgown. Nat. Protoc. 11, 1650–1667. doi: 10.1038/nprot.2016.095
Qiao, X., Li, Q., Yin, H., Qi, K., Li, L., Wang, R., et al. (2019). Gene duplication and evolution in recurring polyploidization-diploidization cycles in plants. Genome Biol. 20:38. doi: 10.1186/s13059-019-1650-2
Qin, L., Hu, Y., Wang, J., Wang, X., Zhao, R., Shan, H., et al. (2021). Insights into angiosperm evolution, floral development and chemical biosynthesis from the Aristolochia fimbriata genome. Nat. Plants 7, 1239–1253. doi: 10.1038/s41477-021-00990-2
Qiu, Y., Li, L., Wang, B., Xue, J., Hendry, T. A., Li, R. Q., et al. (2010). Angiosperm phylogeny inferred from sequences of four mitochondrial genes. J. Syst. Evol. 48, 391–425. doi: 10.1111/j.1759-6831.2010.00097.x
Ravindran, P. N., Nirmal Babu, K., and Shylaja, M. (2004). Cinnamon And Cassia, The Genus Cinnamomum. Boca Raton, FL: CRC Press.
Rendon-Anaya, M., Ibarra-Laclette, E., Mendez-Bravo, A., Lan, T., Zheng, C., Carretero-Paulet, L., et al. (2019). The avocado genome informs deep angiosperm phylogeny, highlights introgressive hybridization, and reveals pathogen-influenced gene space adaptation. Proc. Natl. Acad. Sci. U.S.A. 116, 17081–17089. doi: 10.1073/pnas.1822129116
Roach, M. J., Schmidt, S. A., and Borneman, A. R. (2018). Purge Haplotigs: Allelic contig reassignment for third-gen diploid genome assemblies. BMC Bioinformatics 19:460. doi: 10.1186/s12859-018-2485-7
Seppey, M., Manni, M., and Zdobnov, E. M. (2019). BUSCO: assessing genome assembly and annotation completeness. Methods Mol. Biol. 1962, 227–245. doi: 10.1007/978-1-4939-9173-0_14
Servant, N., Varoquaux, N., Lajoie, B. R., Viara, E., Vert, J. P., Chen, C. J., et al. (2015). HiC-Pro: an optimized and flexible pipeline for Hi-C data processing. Genome Biol. 16, 259. doi: 10.1186/s13059-015-0831-x
Shang, J., Tian, J., Cheng, H., Yan, Q., Li, L., Jamal, A., et al. (2020). The chromosome-level wintersweet (Chimonanthus praecox) genome provides insights into floral scent biosynthesis and flowering in winter. Genome Biol. 21, 200. doi: 10.1186/s13059-020-02088-y
Sharma, S., Walia, S., Rathore, S., Kumar, P., and Kumar, R. (2020). Combined effect of elevated CO2 and temperature on growth, biomass and secondary metabolite of Hypericum perforatum L. In a western Himalayan region. J. Appl. Res. Med. Aroma. 16:100239. doi: 10.1016/j.jarmap.2019.100239
Shi, X., Dai, X., Liu, G., Zhang, J., Ning, G., and Bao, M. (2010). Cyclic secondary somatic embryogenesis and efficient plant regeneration in camphor tree (Cinnamomum camphora L.). In Vitro Cell. Dev. Pl. 46, 117–125. doi: 10.1007/s11627-009-9272-0
SLI Consulting Inc (2021). China’s Linalool Industry Market Development Scale And Forecast in 2021 [Online]. Available online at: https://www.sohu.com/a/482042910_252291 [Accessed Aug 09, 2021].
Stamatakis, A. (2014). RAxML version 8: A tool for phylogenetic analysis and post-analysis of large phylogenies. Bioinformatics 30, 1312–1313. doi: 10.1093/bioinformatics/btu033
Stanke, M., and Morgenstern, B. (2005). AUGUSTUS: A web server for gene prediction in eukaryotes that allows user-defined constraints. Nucleic Acids Res. 33, W465–W467. doi: 10.1093/nar/gki458
Suyama, M., Torrents, D., and Bork, P. (2006). PAL2NAL: Robust conversion of protein sequence alignments into the corresponding codon alignments. Nucleic Acids Res. 34, W609–W612. doi: 10.1093/nar/gkl315
Tan, H., Hu, D., Song, J., Xu, Y., Cai, S., Chen, Q. L., et al. (2015). Distinguishing Radix Angelica sinensis from different regions by HS-SFME/GC-MS. Food Chem. 186, 200–206. doi: 10.1016/j.foodchem.2014.05.152
Tang, H., Bowers, J. E., Wang, X., Ming, R., Alam, M., and Paterson, A. H. (2008). Synteny and collinearity in plant genomes. Science 320, 486–488. doi: 10.1126/science.1153917
Tholl, D. (2015). Biosynthesis and biological functions of terpenoids in plants. Adv. Biochem. Eng. Biotechnol. 148, 63–106. doi: 10.1007/10_2014_295
Tian, Z., Luo, Q., and Zuo, Z. (2021). Seasonal emission of monoterpenes from four chemotypes of Cinnamomum camphora. Ind. Crop Prod. 163:113327. doi: 10.1016/j.indcrop.2021.113327
Wang, D., Zhang, Y., Zhang, Z., Zhu, J., and Yu, J. (2010). KaKs_Calculator 2.0: A toolkit incorporating gamma-series methods and sliding window strategies. Genomics Proteom. Bioinform. 8, 77–80. doi: 10.1016/S1672-0229(10)60008-3
Wu, Y., Xiao, F., Xu, H., Zhang, T., and Jiang, X. (2014). Genome survey in Cinnamomum camphora L.Presl. J. Plant. Genet. Resour. 15, 149–152.
Yang, Z. (2007). PAML 4: phylogenetic analysis by maximum likelihood. Mol. Biol. Evol. 24, 1586–1591. doi: 10.1093/molbev/msm088
Yoshida, T., Muraki, S., Kawamura, H., and Komatsu, A. (1969). Minor constituents of japanese ho-leaf oil the structures of (+)-tagetonol and (-)-trans-Hotrienol. Agric. Biol. Chem. 3, 343–352. doi: 10.1080/00021369.1969.10859320
Yu, G., Wang, L. G., Han, Y., and He, Q. Y. (2012). ClusterProfiler: An R package for comparing biological themes among gene clusters. Omics 16, 284–287. doi: 10.1089/omi.2011.0118
Yuan, M., Yan, Z. G., Sun, D. Y., Luo, X. N., Xie, L. H., Li, M. C., et al. (2020). New insights into the impact of ecological factor on bioactivities and phytochemical composition of Paeonia veitchii. Chem. Biodivers. 17:e2000813. doi: 10.1002/cbdv.202000813
Zeng, L., Zhang, Q., Sun, R., Kong, H., Zhang, N., and Ma, H. (2014). Resolution of deep angiosperm phylogeny using conserved nuclear genes and estimates of early divergence times. Nat. Commun. 5:4956. doi: 10.1038/ncomms5956
Zhang, C., Rabiee, M., Sayyari, E., and Mirarab, S. (2018). ASTRAL-III: Polynomial time species tree reconstruction from partially resolved gene trees. BMC Bioinformatics 19:153. doi: 10.1186/s12859-018-2129-y
Zhang, Z., Wu, X., Lai, Y., Li, X., Zhang, D., and Chen, Y. (2020). Efficient extraction of bioenergy from Cinnamomum camphora Leaves. Front. Energy Res. 8:90. doi: 10.3389/fenrg.2020.00090
Zhao, Z., Guo, P., and Brand, E. (2012). The formation of daodi medicinal materials. J. Ethnopharmacol. 140, 476–481. doi: 10.1016/j.jep.2012.01.048
Keywords: Cinnamomum camphora, genome, top-geoherbalism, tandem duplication, climatic factors
Citation: Jiang R, Chen X, Liao X, Peng D, Han X, Zhu C, Wang P, Hufnagel DE, Wang L, Li K and Li C (2022) A Chromosome-Level Genome of the Camphor Tree and the Underlying Genetic and Climatic Factors for Its Top-Geoherbalism. Front. Plant Sci. 13:827890. doi: 10.3389/fpls.2022.827890
Received: 02 December 2021; Accepted: 24 February 2022;
Published: 21 April 2022.
Edited by:
Jianjun Chen, University of Florida, United StatesReviewed by:
Yafei Mao, University of Washington, United StatesYong Qi Zheng, Chinese Academy of Forestry, China
Copyright © 2022 Jiang, Chen, Liao, Peng, Han, Zhu, Wang, Hufnagel, Wang, Li and Li. This is an open-access article distributed under the terms of the Creative Commons Attribution License (CC BY). The use, distribution or reproduction in other forums is permitted, provided the original author(s) and the copyright owner(s) are credited and that the original publication in this journal is cited, in accordance with accepted academic practice. No use, distribution or reproduction is permitted which does not comply with these terms.
*Correspondence: Li Wang, d2FuZ2xpMDNAY2Fhcy5jbg==; Kaixiang Li, bGt4MjAyQDEyNi5jb20=; Cheng Li, bGljaGVuZ0BjYWFzLmNu
†These authors have contributed equally to this work