- 1Unit Bioresources, Center for Health & Bioresources, AIT Austrian Institute of Technology, Tulln, Austria
- 2Saatzucht Edelhof GmbH, Zwettl, Austria
- 3Unit Assistive and Autonomous Systems, Center for Vision, Automation & Control, AIT Austrian Institute of Technology, Vienna, Austria
- 4Department of Crop Sciences, Institute of Agronomy, University of Natural Resources and Life Sciences Vienna, Tulln, Austria
- 5Human-Centered AI Lab, Department of Forest- and Soil Sciences, Institute of Forest Engineering, University of Natural Resources and Life Sciences Vienna, Vienna, Austria
- 6Verein zur Förderung einer nachhaltigen und regionalen Pflanzenzüchtung, Zwettl, Austria
Marker-assisted selection (MAS) plays a crucial role in crop breeding improving the speed and precision of conventional breeding programmes by quickly and reliably identifying and selecting plants with desired traits. However, the efficacy of MAS depends on several prerequisites, with precise phenotyping being a key aspect of any plant breeding programme. Recent advancements in high-throughput remote phenotyping, facilitated by unmanned aerial vehicles coupled to machine learning, offer a non-destructive and efficient alternative to traditional, time-consuming, and labour-intensive methods. Furthermore, MAS relies on knowledge of marker-trait associations, commonly obtained through genome-wide association studies (GWAS), to understand complex traits such as drought tolerance, including yield components and phenology. However, GWAS has limitations that artificial intelligence (AI) has been shown to partially overcome. Additionally, AI and its explainable variants, which ensure transparency and interpretability, are increasingly being used as recognised problem-solving tools throughout the breeding process. Given these rapid technological advancements, this review provides an overview of state-of-the-art methods and processes underlying each MAS, from phenotyping, genotyping and association analyses to the integration of explainable AI along the entire workflow. In this context, we specifically address the challenges and importance of breeding winter wheat for greater drought tolerance with stable yields, as regional droughts during critical developmental stages pose a threat to winter wheat production. Finally, we explore the transition from scientific progress to practical implementation and discuss ways to bridge the gap between cutting-edge developments and breeders, expediting MAS-based winter wheat breeding for drought tolerance.
1 Introduction
Water scarcity is seen as a key threat for the 21st century (Unesco, 2012), with global water demand expected to surpass supply by 40% by 2030 (Gilbert, 2010). Even under the ‘Green Path’ Shared Socioeconomic Pathway (SSP1), which envisions a future with increased sustainability and reduced resource and energy consumption (Riahi et al., 2017), Europe is projected to experience a rise in the maximum annual temperature of over 5°C, a decrease in precipitation of about -700 mm, and a reduction in soil water content of up to -62 kg/m2 by 2060 relative to 2020 (see Figure 1A). Given that agriculture is the primary user of freshwater, accounting for 70% of total withdrawal globally (FAO, 2010; Hoekstra and Mekonnen, 2012), it is crucial to develop new strategies to enhance crop water use efficiency through agronomy or breeding to tackle the impending water crisis (Sposito, 2013; Turner et al., 2014; Bodner et al., 2015).
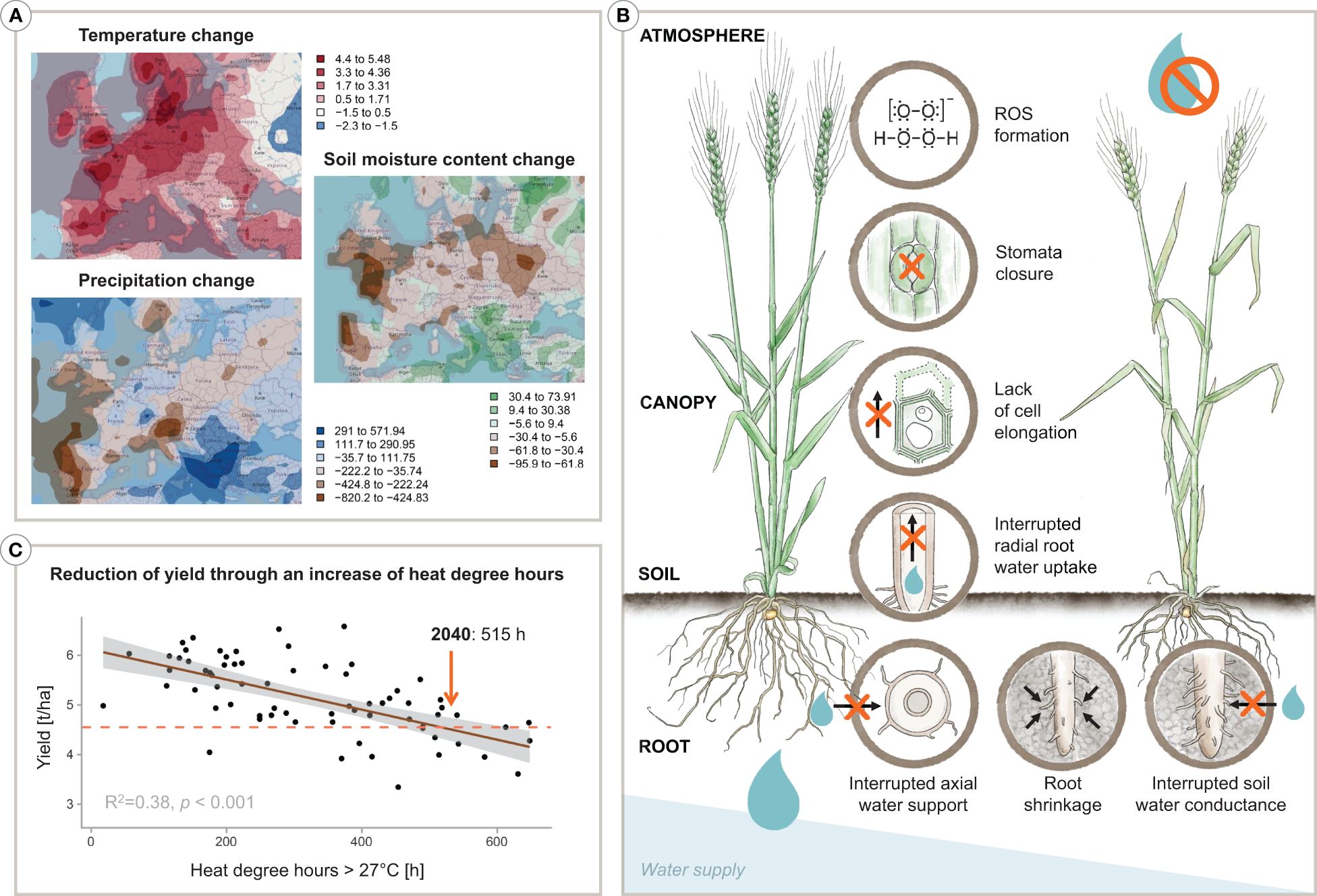
Figure 1 Infographic depicting (A) climatic projections by 2060 in Europe based on the SSP1 scenario, (B) an increase of heat degree hours results in a decrease of yield in wheat, and (C) a plant’s various physiological responses to water deprivation. Specifically, the projections in (A) show the change of the annual maximum temperature [°C], the change of precipitation [mm], and the change of the soil moisture content [kg/m2] by 2060 relative to 2020 considering the best case SSP1 narrative following the ‘Green Path’. In detail, maps are based on the GFDL-ESM4 model data provided by NOAA-GFDL, release year 2018 (Krasting et al., 2018) representing the SSP1-2.6 model made available through the Coupled Model Intercomparison Project, CMIP (Eyring et al., 2016). Furthermore, in (B), a decrease of wheat yield [tonnes/ha] can be seen with rising heat degree hours over the vegetation period. On-farm yield data and heat degree hours represent averages of six districts in Lower Austria during the years 2002–2014. The dashed line indicates a projection to 2040. An overview of the physiological reactions of a plant to drought stress is presented in (C). Designed by Tatjana Hirschmugl and Eva M. Molin.
The yield of wheat, one of the key staple crops worldwide and particularly in Western Europe (about 14% and 25% of total cropland area respectively; FAO, 2023), has seen a steady increase during the second half of the 20th century. However, this trend has shifted since the 1990s, with yields reaching a peak and partially even slightly decreasing, and showing an increasing variability year-to-year. Brisson et al. (2010) suggested two main factors for this shift: (i) the effects of climate change and (ii) a decrease in input intensity, primarily of N-fertiliser, due to EU agri-environmental regulations. Therefore, future production of key crops like wheat will have to cope with higher resource constraints, in terms of both water and nutrients, even in Europe’s temperate climate conditions. Particularly in sub-humid to semi-arid regions, the balance between soil water supply and crop water demand largely determines achievable yield levels (Lalic et al., 2013). With projected higher temperatures and more unpredictable rainfalls, the frequency of periods of crop water shortage is likely to increase (Qin et al., 2023). Additionally, the co-occurrence of heat and drought is expected to have the most significant impact on wheat yield, with a predicted global reduction of 3.9% (Heino et al., 2023). On a more regional scale, for instance, climate change projections for the Pannonian lowlands, an important wheat-producing region in Europe, indicate that the number of dry days with water deficit during the vegetation period will increase (Trnka et al., 2011; Lalic et al., 2013; Schils et al., 2018; van der Velde et al., 2018). Evaluation of past yield data and simulation model predictions point to a high risk for wheat production under climatic conditions with hot temperatures (>25°C; Lüttger and Feike, 2018; Figure 1B) and drought occurring at a sensitive developmental stage, such as germination, tillering, flowering or grain filling (Yu et al., 2018; Senapati et al., 2021; Xu et al., 2022). These factors underscore the urgency to speed up the breeding process for more drought (and heat) tolerant varieties to keep pace with the rate and scale of climate change.
One of the methods that has revolutionised plant breeding by improving its efficiency, speed and precision is marker-assisted selection (MAS) (Collard and Mackill, 2008). There are different MAS strategies such as MAS backcrossing, MAS pyramiding or early generation MAS (Jeon et al., 2023), all of which use DNA-based markers to help select lines with the desired traits. The limited number of markers per trait and its restricted use for traits under complex genetic control are major limitations of MAS. These limitations led to the development of other marker-based strategies such as genomic selection (GS) or crop growth models (Budhlakoti et al., 2022; Zhang et al., 2022). Unlike MAS, GS uses all available (genome-wide) markers to calculate a breeding value and has been shown to outperform MAS in several studies (Arruda et al., 2016; Degen and Müller, 2023). Despite these advancements, MAS is still extensively used to efficiently screen for traits of interest. For instance, MAS has been employed in wheat breeding to improve resistance to biotic and abiotic stresses and to maintain yield potential (Song et al., 2023; Subedi et al., 2023). A notable advantage of MAS may be that, compared to the genome-wide approach of GS, only a few markers ultimately need to be used by the breeder, making MAS –despite its limitations– an affordable solution for practical breeding.
However, for a MAS programme to be successful, certain prerequisites must be met: the generation of high-quality phenotypic and genotypic data, the understanding of marker-trait associations, the characterisation of reliable markers and, finally, the development of cost-efficient and easy-to-use genotyping approaches. In this review, we therefore attempt to cover this process using the example of winter wheat breeding for increased drought tolerance. As a starting point, (i) we revisit the physiological mechanisms and corresponding traits that have been associated with drought tolerance in winter wheat under different drought regimes (Section 2), (ii) we further discuss traditional and modern phenotyping approaches focusing on airborne technologies and time series records and provide a guide for airborne data acquisition for winter wheat (Section 3), (iii) we include genome-wide association studies (GWAS), an important computational approach that links the recorded phenotypes with the genotypes for the identification of genetic markers used in MAS (Sections 4 and 5), and finally, (iv) we address artificial intelligence (AI) models accompanied by explainable AI (xAI) methods that could support the breeding process at several steps in the context of smart agriculture (Section 6). Attempting to bridge the gap between scientific innovations and their application in practice, (v) we conclude this review with an overview of the practical work of plant breeders (Section 7) and where these (novel) cutting-edge approaches could fit in and help accelerate the breeding process.
2 Physiological mechanisms underlying drought tolerance
Historically, advances in wheat breeding have largely been driven by increased yield potential through better assimilate partitioning towards grain sinks, sustained by prolonged assimilate source activity due to extended green canopy duration (Lichthardt et al., 2020). However, under water-limited conditions, yield formation is a complex function of total water uptake, water use efficiency, and harvest index (Passioura, 1977). Ecophysiological theory has guided trait-based breeding by uncovering stress adaptation strategies in natural vegetation. Levitt’s scheme of dehydration avoidance, dehydration tolerance, and drought escape (Levitt, 1980) serves as a guiding framework for physiological breeding: plant traits underlying individual stress response types aid targeted selection for crop adaptation in water-limited environments (e.g., Richards, 2006; Araus et al., 2008; Cattivelli et al., 2008).
The selection of relevant traits involved in drought tolerance mechanisms that could potentially lead to better and more stable yields strongly depends on the time when the drought occurs (van Ginkel et al., 1998; Blum, 2011). For instance, the phenological adaptation (‘drought escape’) of early maturity might be especially sensitive to early drought events while thriving in summer-dry regions with water deficiency during the grain-filling stage. Dehydration avoidance by ‘water saving’ (Levitt, 1980) might result in suboptimal use of available water under moderate drought regimes, while in situations with more severe drought and crop growth largely dependent on stored soil moisture from off-season rainfalls, a ‘conservative’ water use preserves water for grain filling and yield formation (Mori et al., 2011).
As highlighted in Figure 1C, the regulation of plant water balance forms the physiological basis for identifying potential breeding traits for more drought-tolerant plants. Whether transpiration can meet the potential demand, driven by the atmospheric vapour pressure deficit, depends not only on the availability of soil water but also on the transport capacity of soil and plants under variable driving gradients (e.g., Maseda and Fernandez, 2006). In coarse to medium-textured soils, the transport of water through the tortuous soil pore system to the root surface drops sharply when larger pores drain upon successive soil drying, resulting in supply limitation (wilting) at a water content substantially higher than the permanent wilting point (Czyż et al., 2012). With successive drying, the root-soil contact can be lost due to root shrinkage and air gap formation as well as root mucilage becoming hydrophobic to protect root tissues from dehydration (Carminati and Vetterlein, 2013; Affortit et al., 2023). Stomata are the ultimate regulators of crop water transport, providing a mechanism to prevent plants from dehydration damage (cf. Figure 1C). Stomata thus act upon imbalances between vapour losses from and liquid water transport to the transpiring leaves. Root water uptake (Abdalla et al., 2022) and xylem transport (Cruiziat et al., 2002) are crucial for stomata regulation, mediated by chemical and hydraulic signals within plant-specific safety margins (Sperry and Love, 2015). Sustained xylem water flow under high-pressure gradients between soil and atmosphere without interruption of transport vessels by air embolism, leading to an eventual hydraulic failure of the transport system, has been suggested as one of the key bottlenecks for crop performance in dry environments (Sperry et al., 1998; Vadez et al., 2013; Vadez, 2014). Plants relying on high safety margins with sensitive stomata response to tissue dehydration (isohydric behaviour; Tardieu and Simonneau, 1998; Hochberg et al., 2018), also have to cope with increased leaf temperature and high radiation load at the leaf, which leads to an overproduction of reactive oxygen species that cause metabolic disorders and limit plant growth and development (Mukarram et al., 2021). Within this general framework of physiological mechanisms and related traits, Blum (2009) points to maximising water uptake as a focus for breeding because it is generally compatible with high yields, i.e. genotypes that fall into the category of ‘water wasters’ according to Levitt’s framework. Efficient water uptake by the root system is a desirable breeding objective (Vadez et al., 2007). In wheat, physiological and root research studies indicate a significant contribution of the root system to increased drought tolerance (e.g. MansChadi et al., 2008; Palta et al., 2011; Becker et al., 2016; Li et al., 2021).
To expand the germplasm sources of (novel) stress tolerance traits, landraces and crop wild relatives are a valuable resource offering a wealth of diversity (Galluzzi et al., 2020) that could be transferred into breeding programmes, as has been extensively reviewed for wheat (Valkoun, 2001; Reynolds et al., 2006; Trethowan and Mujeeb-Kazi, 2008; Nakhforoosh et al., 2015; Lehnert et al., 2022; Aloisi et al., 2023; Shokat et al., 2023). Specifically, cereal genetic resources could contribute to improved drought tolerance through higher water use efficiency (Konvalina et al., 2010), rapid early development (Mullan and Reynolds, 2010), stem reserve demobilisation, osmotic adjustment (Reynolds et al., 2006), and even plant waxiness (Patidar et al., 2023). Several studies also suggest a contribution of root traits (e.g., Reynolds et al., 2006; Sanguineti et al., 2007; Trethowan and Mujeeb-Kazi, 2008; Lopes and Reynolds, 2010; Nakhforoosh et al., 2014).
Despite these studies, further progress in physiological and trait-based breeding to accelerate wheat improvement for future environmental conditions critically depend on adequate selection strategies that combine (advanced) targeted trait phenotyping (see Section 3) with modern genetic tools (see Sections 4 and 5).
3 From traditional to airborne phenotyping
The practice of measuring phenotypic traits dates back to Neolithic agriculture when domesticated cereals were intentionally selected for traits such as broad kernels (Zohary et al., 2012). Today, one of the cornerstones of plant breeding is the selection of superior individuals based on phenotypic traits (e.g., grain yield), and more recently, the identification of genome regions controlling these traits (cf. Sections 4 and 5). With advancements in sensor technology, phenotyping has evolved into a high-throughput process, including remote sensing and machine learning (ML), offering solutions for precision agriculture and digital plant breeding (Walter et al., 2015; Pieruschka and Schurr, 2019; Holzinger et al., 2022a; Jeon et al., 2023). This diversity of phenotyping approaches is mirrored in the wide range of data and data formats obtained during the breeding process by different sensors (Thoday-Kennedy et al., 2022), such as visual scorings, direct measurements of plant phenotypic parameters, meteorological readings, and hyperspectral and multispectral measurements (Heremans et al., 2015; Adão et al., 2017; Becker and Schmidhalter, 2017; Hu et al., 2020; Saranya et al., 2023), which we aim to cover in this review with respect to wheat.
3.1 Traditional phenotyping
Modern plant breeding still depends on traditional phenotyping, which includes visual scorings, plant measurements, and destructive sampling followed by laboratory analysis (Furbank and Tester, 2011; Atkinson et al., 2018). Each type has unique characteristics in terms of precision and measurement speed. Non-destructive measurements are easily measured, such as plant height and visual assessments of disease occurrence, phenology, and plant architecture. These visual assessments are commonly used and are also applied for official national variety testing, e.g., in Austria (Kumar et al., 2016; Steiner et al., 2017; Anderegg et al., 2020; AGES, 2023; Lunzer et al., 2023). However, the precision of non-destructive measurements can be limited by various factors such as observer variability and lighting conditions. Conversely, destructive measurements involve the collection and analysis of plant samples to acquire data on above-ground dry matter, grain yield, and quality traits like protein content and baking quality. Despite offering high precision, these measurements are time-consuming, destructive, and often limited by cost considerations.
As for breeding experimental setups, they can be classified based on the degree of control over environmental conditions (Hammer and Hopper, 1997). Growth chambers provide highly controlled conditions, where numerous environmental variables such as temperature, light intensity, and CO2 concentration can be manipulated (Rezaei et al., 2018). Semi-controlled conditions, observed in, e.g., greenhouses and rain-out shelters, offer some control over environmental factors, with greenhouses affording greater control than rain-out shelters (Yadav, 2017; Rezaei et al., 2018). Finally, experiments under field conditions feature the lowest control over environmental variables. Nevertheless, field experiments are undoubtedly relevant, since most of them are conducted in the field under uncontrolled conditions (Hammer and Hopper, 1997). They allow for scientific testing of experimental factors under conditions similar to agriculture practice. Experimental factors can include varying genotypes, sowing times, fertilisation, plant protection, irrigation and disease occurrence due to natural pressure as well as artificial inoculation (Buerstmayr et al., 2000; Koppensteiner et al., 2022).
Observational units vary across setups, ranging from plots in field experiments and rain-out shelters to pots in greenhouses and growth chambers (Buerstmayr et al., 2000; Yadav, 2017; Rezaei et al., 2018; Koppensteiner et al., 2022). In field trials, units of observation include single seeds (Zhu et al., 2012), single rows (Buerstmayr et al., 2000), micro-plots (Miedaner et al., 2006), and large plots (Koppensteiner et al., 2022), e.g., 1.5 m by 7 m, depending on the amount of available seed material of a genotype in the respective stages of the breeding process. In the context of UAV-based sensor systems discussed in this review, micro-plots and large plots are the most relevant observation units. Measurements on more detailed levels are possible depending on the specifications of sensor systems and operational flight height. Despite the significance of field experiments, conducting field phenotyping is arduous, time-intensive, and susceptible to human and environmental variability. Therefore, there is a pressing need to enhance field phenotyping capabilities to facilitate accurate and high-throughput phenotyping, thus expediting crop breeding processes (Yang et al., 2020).
3.2 Remote sensing
Remote phenotyping techniques in digital agriculture are prized for their non-destructive nature and their ability to improve data collection accuracy and efficiency (Sishodia et al., 2020; Jeon et al., 2023). These techniques rely on remote sensing, which involves detecting electromagnetic radiation across various wavelengths emitted, reflected, or transmitted by objects. Remote sensing measurements are categorised into direct and indirect methods. Direct measurements involve directly gauging traits of interest, such as plant height using digital surface and terrain models (Holman et al., 2016), while indirect measurements estimate traits using statistical or ML models like biomass and water stress estimates (Wang et al., 2016; Das et al., 2021).
Remote phenotyping can be conducted at various scales: ground-based - handheld or vehicle-mounted (Kumar et al., 2020; Tang et al., 2023), aerial - via aircraft or UAVs (Fei et al., 2023; Nguyen et al., 2023), and satellite platforms like Sentinel-2 (Zhao et al., 2020; European Space Agency, 2023a), Landsat (Zhou et al., 2020; Darra et al., 2023; NASA, 2023), WorldView-2 and 3 (Tattaris et al., 2016; Yuan et al., 2017; European Space Agency, 2023b), or RapidEye (Eitel et al., 2007; European Space Agency, 2024). To contextualise these platforms, key remote sensing features are spatial, temporal, spectral, and radiometric resolutions (Verde et al., 2018). Spatial resolution refers to pixel size, temporal resolution to the time between measurements, spectral resolution to the number of spectral channels, and radiometric resolution to a sensor’s ability to detect varying energy quantities in a specific spectral channel. Each phenotyping platform presents trade-offs; for instance, ground-based techniques offer high spatial resolution but require dedicated manpower, leading to lower time resolution. Aerial technologies offer enhanced operational performance and sub-centimetre spatial resolution (Bhandari et al., 2020) but are weather-dependent, limiting time-series data availability. Satellites provide densely populated time series but sacrifice spatial resolution, with modern satellites offering resolutions as low as 31 cm in the case of Worldview-3 (European Space Agency, 2023b). Moreover, in general, increasing sensor-object distance or increasing the swath width of the satellite, i.e. the horizontal distance covered by a satellite sensor, can improve temporal resolution by allowing the sensor to revisit the same location more frequently. However, this enhancement comes at the cost of diminished spatial resolution (Kadhim et al., 2016). Other trade-offs do not depend on the spatial resolution, but, for UAV, the maximum weight of a payload determines the equipped camera and therefore the spectral resolution available to be measured (Mohsan et al., 2023).
Another key concept in remote sensing and therefore in remote phenotyping is the Ground Sampling Distance (GSD), i.e. the spatial spacing between the centres of two consecutive pixels as measured on the ground. It is determined by several key factors: altitude (h), denoting the height above the ground at which the sensor is positioned and affecting the scale of the captured image; sensor size (s), representing the physical size of the sensor in the camera, typically measured in mm, larger sensors capturing more detail and impacting the GSD; focal length (f), the distance from the optical centre of the lens to the camera sensor, measured in mm, influencing the field of view and magnification of the captured image, and image resolution (r). The GSD is mathematically represented as:
This metric is important because it directly determines the spatial resolution of the imagery, affecting the level of detail that can be captured and the accuracy of any measurements or analyses conducted on the images. Generally, a smaller GSD indicates a higher spatial resolution and finer detail in the imagery. GSD values vary across different imaging platforms. For UAV imaging, GSD can vary depending on factors like altitude and sensor specifications, generally falling between 0.5 to 10 cm per pixel (Yuan et al., 2018). This range allows for moderately detailed aerial imagery suitable for various agricultural and environmental applications. On the other hand, satellite imaging offers broader coverage but typically lower spatial resolution. GSD for satellite imagery can range from 30 cm to several m per pixel, depending on the satellite platform, sensor, and imaging mode employed (Chawade et al., 2019).
In plant breeding, the field experimental plot is the typical unit of observation (Hammer and Hopper, 1997). While current satellite systems’ spatial resolution may be inadequate for precise phenotypic parameters at a plot level (Tattaris et al., 2016), ground-based and aerial remote sensing approaches offer suitable spatial resolution. UAVs, with their flexibility, extended operational times, lower cost, and high spatial resolution in the low centimetre range, emerge as promising phenotyping platforms for plant breeding and precision agriculture (Sishodia et al., 2020; Guo et al., 2021).
3.3 UAV-based remote phenotyping
UAV remote sensing coupled with ML provides a non-destructive method that enables repeated plant measurements over time. This is a significant improvement over traditional methods, which are laborious, time-consuming and expensive (Galieni et al., 2021; Nguyen et al., 2023). Therefore, the use of UAVs for remote phenotyping has become a well-established practise in plant breeding (Yang et al., 2020; Guo et al., 2021). Compared to other remote sensing platforms, UAVs offer several advantages. They are capable of swiftly collecting spectral data, outperforming the speed of handheld devices. They can capture data at a higher resolution compared to aerial cameras operated from a manned aircraft, and they are not dependent on satellite overpasses for data collection in the region of interest (Kim et al., 2019).
3.3.1 An overview of UAV sensor systems
UAVs can be equipped with passive sensors, such as multispectral, hyperspectral and thermal cameras, or active sensors, such as Light Detection And Ranging (LiDAR) (Thoday-Kennedy et al., 2022).
Since multispectral and hyperspectral cameras can capture data at various wavelengths (also outside the visible spectrum), their use in agricultural applications offers many benefits. They can identify and monitor crop health and stress (Yang et al., 2009; Virnodkar et al., 2020), determine and map corn emergence uniformity (Vong et al., 2022) and quickly detect diseases and pests (Prabhakar et al., 2012). Multispectral leaf reflectance data are very useful because they contribute to computing indices widely used in agriculture (see Table 1 for an overview of the main vegetation indices). Additionally, both multispectral and hyperspectral data can be utilised to estimate crop yields using ML methods (Fei et al., 2023; Joshi et al., 2023). In contrast to multispectral sensors, which typically capture broader spectral bands with spectral resolutions from 10 to 100 nm, hyperspectral sensors offer a much higher spectral resolution, often within 1 to 10 nm (Adão et al., 2017). They effectively capture a spectral continuum across hundreds of contiguous, narrow bands, enabling detailed pixel-by-pixel analysis. Hyperspectral cameras are capable of capturing not only the visible (400-700 nm) and near-infrared (NIR, 700-2500 nm) wavelength ranges but also radiation from the ultraviolet (UV, 100-400 nm) to thermal infrared (TIR, 3000-15000 nm) wavelengths. However, the large data storage required for hyperspectral data can limit its use in large-scale applications (Sun et al., 2019). Therefore, despite their significant advantages, hyperspectral applications in large-scale wheat phenotyping could face challenges related to data storage, management, and budget constraints (Ang and Seng, 2021).
Thermal imaging, which operates within the broader long-wave infrared (LWIR) wavelengths (from 8 to 15 µm), serves as a valuable tool for detecting plant stress. Thermal measurements can be used to evaluate the transpiration status, plant vigour, and the spread of diseases in wheat cultivars (Mahlein et al., 2012) or, together with measurement of the air temperature, to compute the Crop Water Stress Index (CWSI). This index can then be incorporated as a feature in an ML model to provide insights on canopy head evapotranspiration or to segment image data into temperature areas (Zhou et al., 2021b). Moreover, combining thermal imaging data with other phenotypic traits improves the holistic understanding of plant responses to environmental conditions. This synergy enables researchers, breeders and farmers to make well-informed decisions for optimal crop management and resource allocation (Khanal et al., 2017; Stutsel et al., 2021).
On the other hand, unlike camera-based systems that passively capture reflected, transmitted, or emitted light, LiDAR is an active technique that emits laser pulses and measures the time for these pulses to reflect off objects, providing precise distance and spatial data. This has been particularly useful in wheat breeding for estimating plant biomass and plant height (Hütt et al., 2023). Taking advantage of global navigation satellite systems (GNSS) and laser altimetry, and using GIS software, accurate crop height measurements can be obtained by subtracting a digital terrain model from a digital surface model representing the crop canopy surface (Jenal et al., 2021). Although LiDAR systems typically operate at a single wavelength, combining geometric measurement with spectral information is possible, such as registering multispectral camera images with LiDAR point clouds (Hakula et al., 2023), or using LiDAR systems with individual lasers at various frequencies, e.g., Optech Titan (GEO3D, 2023).
3.3.2 Spectral indices supporting smart wheat breeding
In the context of wheat breeding, an index is a mathematical formula designed to provide a comprehensive representation of various plant traits, physiological states and characteristics (Reynolds and Langridge, 2016). It combines different desired traits into a single numerical value, allowing breeders to assess and compare the overall performance of different wheat varieties more thoroughly (Myneni et al., 1995). The computation of these indices creates a multidimensional profile, enriching the complexity of the breeding problem and providing valuable input for machine-learning approaches. Consequently, indices are crucial tools that enable breeders to make informed decisions, optimise their breeding strategies, and ultimately develop wheat varieties that thrive in a wide range of agricultural and environmental conditions in modern research (Radočaj et al., 2023).
In precision agriculture, vegetation indices are broadly categorised into two main types: broadband and narrowband (Thenkabail et al., 2002). Broadband indices, such as the Normalised Difference Vegetation Index (NDVI) (Rouse et al., 1974), integrate information from relatively wide spectral bands, such as the NIR band. These indices offer a generalised measure of vegetation vigour and health. This approach is efficient and simple, making these indices suitable for large-scale agricultural monitoring and management tasks where rapid assessment is prioritised. In contrast, narrowband indices, such as the Chlorophyll Absorption Ratio Index (TCARI) (Haboudane et al., 2002), target specific narrow spectral bands within the electromagnetic spectrum. These indices focus on precise absorption features related to chlorophyll content, leaf structure, and other biochemical properties. Narrowband indices provide high spectral resolution making them valuable for tasks requiring in-depth analysis of plant health and stress. The choice of using either family of indices depends on the specific physiological traits under investigation.
Table 1 presents several indices common in remote sensing for wheat phenotyping. The practical rationale behind our selection of these indices is the ease of computing them with standard commercially available multispectral cameras (NIR - 700-2500 nm, RGB - 400-700 nm, SWIR - 2500-3000 nm, Red Edge-700-730 nm) and their recognised impact in assessing the plant water status, general stress condition and phenological traits. Vegetation Indices play a crucial role in assessing various aspects of vegetation health and physiological traits. The Normalised Difference Vegetation Index (NDVI) is widely utilised due to its computational simplicity, facilitating assessments of vegetation density, plant health, and water stress (Condorelli et al., 2018; Hassan et al., 2019; Huang et al., 2021). However, limitations such as computational approximations and instrument inaccuracies can occasionally hinder its effectiveness in evaluating plant stress (Khan et al., 2018).
To address these limitations, several alternative indices have been developed. The Enhanced Vegetation Index (EVI) enhances the vegetation signal in high biomass areas and corrects for aerosol factors (Khan et al., 2018). Additionally, the Soil-Adjusted Vegetation Index (SAVI) and Modified Soil-Adjusted Vegetation Index (MSAVI) correct for soil irradiation in areas with low canopy cover (Prudnikova et al., 2019). The Normalised Difference Water Index (NDWI) detects water presence and sensitivity to water content (Wu et al., 2009). The Green Normalised Difference Vegetation Index (GNDVI) specifically targets green vegetation, utilising the green band instead of red. Furthermore, the Normalised Difference Red Edge (NDRE) emphasises the red edge region of the spectrum instead of the red band. These last two indices correlate with leaf nitrogen content and are used for controlling nitrogen leaf status (Li et al., 2019).
For other physiological traits, specialised indices have been developed. The Transformed Chlorophyll Absorption Reflectance Index (TCARI) estimates chlorophyll content in vegetation and biomass (Wang et al., 2022). The Leaf Area Index (LAI) measures foliage density within a canopy by comparing leaf surface area to ground area. The Anthocyanin Reflectance Index (ARI) identifies the presence of anthocyanins, aiding in the assessment of plant stress, phenology, and disease infection (Koc et al., 2022). Lastly, the Canopy Chlorophyll Content Index (CCCI) estimates chlorophyll levels in vegetation by combining red and red edge bands (Cummings et al., 2021).
Each of these indices offers unique insights that can inform breeding decisions, including assessments of yield potential and drought resistance, thus necessitating careful selection among the myriad indices developed by the remote sensing community (Xue and Su, 2017).
3.3.3 Machine learning for interpreting high-throughput field phenotypic data
In these scenarios, ML techniques showcase their advantage over conventional approaches in predicting phenotypes (Ansarifar et al., 2021). As high-throughput phenotyping methods produce a large volume of data, the use of ML becomes pivotal in accurately interpreting and effectively leveraging this data, leading to more precise phenotype predictions (Shaikh et al., 2022). For example, Wang et al. (2016) presents how random forest (RF) models outperform simple multilayer perceptrons (MLPs) and support vector machines (SVMs) in predicting wheat biomass. Grinberg et al. (2020) provides a comparative study of different ML models on various phenotyping problems across different crops, including wheat. The advent of deep learning enhances the classification of crop images, offering unprecedented granularity in monitoring crop quality, assessing yield, and pinpointing water stress at a pixel-wise level (Chandel et al., 2021). Convolutional neural networks (CNNs) further boost the model’s capabilities, automatically extracting key features and patterns to make reliable phenotype predictions (Jiang and Li, 2020). Moreover, deep learning models have expanded the range of possible predictions to include disease detection, stress severity quantification, and yield (Mohanty et al., 2016; Giménez-Gallego et al., 2019; Zhou et al., 2021a). An intriguing direction that research has taken is semi-supervised approaches to the learning problem (Tang et al., 2023; Zhou et al., 2023). Semi-supervised deep learning is an ML paradigm where a model is trained using a combination of labelled and unlabelled data. It uses the limited labelled data to guide the learning process and improve the model’s performance on tasks such as classification or regression, while also benefiting from the larger pool of unlabelled data for generalisation and enhanced feature representation (Yang et al., 2021). Deep learning significantly improves the model’s ability to generalise and enables accurate and reliable phenotyping models for high-throughput approaches. However, a key drawback of deep learning approaches is that each solution needs to be tailored to the data and the phenotypic trait under investigation.
While traditional methods continue to hold their merits, integrating (UAV-based) remote sensing coupled with ML in phenotyping processes might be essential to obtain better and more resilient crop varieties (Yang et al., 2020). In addition, operational costs could be significantly reduced by cutting fixed costs such as laboratory equipment and workforce. This would lead to improved scalability in the approach and quicker results that are passed over in the data pipeline.
3.4 A guide for UAV-based data acquisition for winter wheat
Moving to the next stage, this Section presents a detailed overview of a potential high-throughput field phenotyping system specifically tailored for winter wheat. The main objective is to facilitate the acquisition of phenotypic data for GWAS (see Section 4) and MAS techniques in the frame of precision agriculture. A schematic representation of the key components of the pipeline is presented in Figure 2.
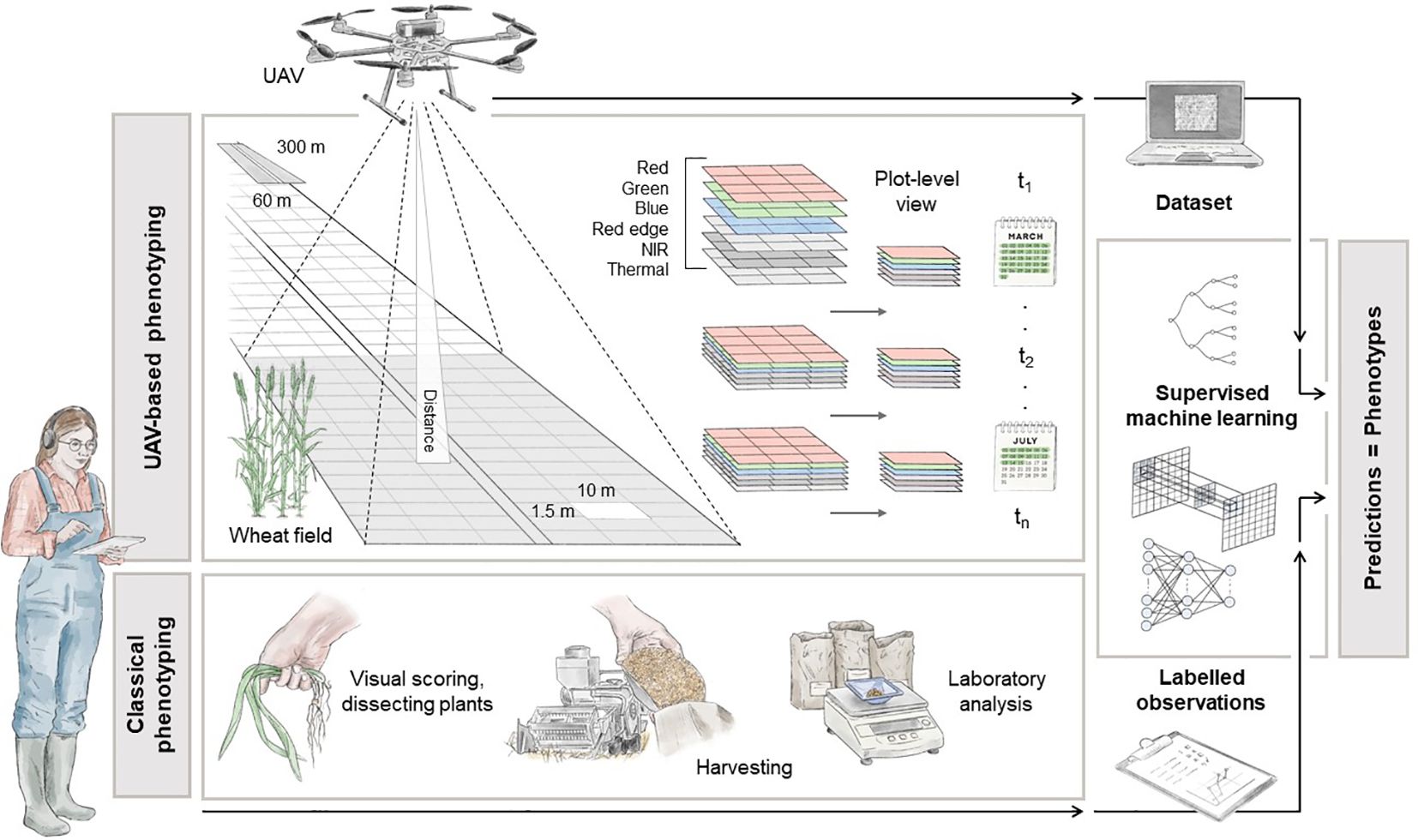
Figure 2 Illustration of a high-throughput UAV-based phenotyping configuration for plot-level analysis. The pipeline explains how to get from the raw data, in-situ phenotype acquisitions and raw images, to a structured and cohesive dataset to be employed in ML operations. The depicted dimensions, captured wavelengths, ML models, and traditional phenotyping methods serve as reference points. Designed by Tatjana Hirschmugl.
In this scenario, the fundamental premise revolves around the division of the test field into georeferenced experimental plots, overseen by experts tasked with gathering in-situ data. This experimental arrangement mirrors established methodologies seen across various research endeavours, aimed at facilitating controlled crop cultivation (Bai et al., 2016; Haghighattalab et al., 2016; Volpato et al., 2021). The grid structure delineating individual plots within the field is visually represented in Figure 2. Typically, experts conduct assessments and record measurements by visually inspecting these plots, as demonstrated in Koc et al. (2022). It’s also advantageous to conduct these measurements at specific intervals, tailored to the trait being studied. For instance, in Fernandez-Gallego et al. (2020), multispectral images were captured under direct sunlight on three dates: June 6th, 25th, and July 3rd, 2018, in Belgium, corresponding to the developmental stages flowering and ripening, to monitor wheat ear development and count. During each scheduled flight mission, a UAV systematically follows a predefined grid pattern, meticulously gathering data while traversing the agricultural field.
The UAV could be equipped with a camera capable of capturing a range of spectral information, including RGB, panchromatic, Red Edge, NIR and thermal measures, during its flight (Holman et al., 2016; Tattaris et al., 2016; Duan et al., 2017). The specific selection of spectral bands depends on the particular index to be computed, which in turn depends on the trait under investigation. Additionally, the camera must undergo radiometric calibration to ensure the acquisition of physically meaningful measurements. The spatial resolution of the data acquired is influenced by both the altitude of the UAV and the intrinsic parameters of the camera used. For example, a standard multispectral camera (e.g. AgEagle Aerial Systems Inc, 2023) with 3.2 MP captures images with 2.5 cm GSD at an altitude of 60 m above the ground. The collected data is typically processed using photogrammetric software like Pix4D or Agisoft (Zhu et al., 2019). These software applications are used to create a reflectance map of the agricultural field by orthorectification and stitching individual images to reconstruct a high-resolution representation of the target area. Subsequently, plot-level spectral information is extracted using geospatial software, e.g. GIS, and organised for easy access (Beltrame et al., 2024). This data is then linked with specific plots and expert-acquired labelled information (lower part of Figure 2) to create tuples for subsequent ML analysis. These calibrated, cleaned, and standardised datasets can be used in classical preprocessing operations, including image normalisation, data augmentation, and sub-/oversampling techniques. To fully harness the information-rich content obtained, it is essential to select models that can handle the spatial complexity inherent in high-resolution images. For instance, a basic deep learning architecture, such as CNNs, can be used to extract feature maps from images and make accurate phenotype predictions (Kattenborn et al., 2021; Nguyen et al., 2023).
Recent advancements in image analysis, data extraction, and augmentation (Shorten and Khoshgoftaar, 2019), coupled with innovative artificial image synthesis techniques (Lu et al., 2022), and transfer learning (Hutchinson et al., 2017) are greatly enhancing the development and the integration of remote sensing technologies in agriculture. These advancements are starting to contribute to overcoming the phenotyping bottleneck (Song et al., 2021) and significantly enhance the provision of high-quality phenotype data to genotype - phenotype association studies ultimately resulting in an efficient and reliable MAS.
4 GWAS - a playground for the identification of genetic markers
Besides a meticulous recording of phenotypic data, MAS depends on the availability of genetic markers linked to the phenotypic trait of interest. Identifying these genetic regions associated with a phenotype is often not a straightforward task: many traits are polygenic, which adds to the complexity of their relationship with the phenotype (Korte and Farlow, 2013; Boyle et al., 2017; Mills and Rahal, 2019; Pierce et al., 2020). The general approach of linking genetic regions to traits, known as genetic mapping, consists of two main strategies: (biparental) linkage mapping (LM) and association mapping (AM) (March, 1999). LM utilises closely related individuals to study the co-segregation of markers and traits due to physical proximity, while AM uses diverse, unrelated populations to detect statistical associations between markers and traits. AM, also known as linkage disequilibrium mapping, exploits linkage disequilibrium (Mackay and Powell, 2007), which is the nonrandom association pattern between alleles at different loci within a population (Nordborg and Tavaré, 2002; Gaut and Long, 2003). Since its introduction to plants (Tenaillon et al., 2001; Thornsberry et al., 2001), AM has become increasingly important in genetic research as cost-effective, high-throughput technologies for genotyping single nucleotide polymorphisms (SNPs) are now available, enabling dense marker coverage (Syvänen, 2005). A particular concept of AM, namely genome-wide association studies (GWAS), has become a common technique for understanding complex traits in plants in general and in many crop species, including wheat (Zhu et al., 2008; Cortes et al., 2021).
The primary advantage of GWAS is that it tests thousands to millions of genetic variants (e.g., SNPs) of many individuals from different populations on a genome-wide scale, allowing more complex genotype-phenotype relationships to be explained than with LM. However, for a genome-wide analysis, the knowledge about and the characterisation of SNPs is an essential part and is driven by the sequencing of the whole genome of the target organism. In the case of wheat, its genome was fully sequenced in 2018 (Appels et al., 2018) and has been continuously improved since then Shi and Ling (2018); Guan et al. (2020); Gao et al. (2023), including the creation of a pangenome (Montenegro et al., 2017; Jayakodi et al., 2021), which provides a valuable knowledge base for the development of a variety of high-density SNP arrays for high-throughput genotyping (Wang et al., 2014; Rimbert et al., 2018; Sun et al., 2020). Finally, to link these genotypic traits to the measured phenotypes, a wide range of GWAS-based tools and statistical methods are available, which have already been used in wheat, as shown in Table 2, which are described in the following Section in more detail.
4.1 GWAS modelling strategies
The modelling strategies underlying GWAS are diverse from a statistical perspective, of which linear and Bayesian models are the prevailing strategies. Linear models fit linear equations to the data (genetic and phenotypic data), testing each specific marker and its relationship with the phenotype independently, simplifying the computational complexity that could arise from the genetic intricacies in the data (Sabatti, 2013). Generalised linear models (GLMs), as described in Nelder and Wedderburn (1972), add an additional layer of complexity, including a link function to relate input and output, thus providing certain flexibility from the rigidity of linearity. Linear mixed models (LMMs) represent another logical extension of linear models for GWAS and are widely applied (cf. Table 2). LMMs include fixed and random effects to model phenotypes, and can account for confounding factors such as population stratification, family structure, etc (Alamin et al., 2022). LMMs also offer versatility as they can analyse many experimental designs (Yang, 2010). These models, as their name suggests, assume a linear relationship between genotype and phenotype. They also assume that the random effects are normally distributed and that there is homoscedasticity in the variance of their errors (Warrington et al., 2014). These are the two main concepts use for GWAS methods based on linearity.
Bayesian models have also been developed and used for GWAS (cf. Table 2), fitting all markers simultaneously while addressing the issue of data dimensionality, making them well suited for polygenic traits (Fernando and Garrick, 2013; Miao et al., 2019). These methods require the specification of prior distributions, allowing knowledge of the data to be incorporated into them to yield more accurate results, with the caveat that deviation from the specified distribution can impair performance and statistical power (Cortes et al., 2021). Bayesian GWAS aim to identify sections of the genome that explain more than a threshold of the variance (Fernando and Garrick, 2013; Cortes et al., 2021). The multiple methods developed assume different distributions for the calculation of the priors, having different performance according to the deviation from their actual distribution. Markov Chain Monte Carlo algorithms have been used to infer model parameters using Gibbs-type processes, as in Habier et al. (2011). The posterior probabilities of association, the odds of a specific SNP being actually related to the trait, can be calculated from the Bayes factor (Stephens and Balding, 2009).
4.2 Understanding the limitations of GWAS
Despite all these advancements, GWAS still have significant limitations in their design and application (Korte and Farlow, 2013; Wray et al., 2013; Tam et al., 2019; Cortes et al., 2021): they can be limited to the populations that are more represented in the studies, and there can exist a lack of transferability, as results may not extrapolate to other groups (Bouaziz et al., 2011), or the number of ostensible causal variations might be reduced if data from genetically diverse populations were used, so it is paramount to have an adequate representation of the population to reduce the possible biases that can arise from this (Clyde, 2019; Uffelmann et al., 2021). In addition, at this point, the causality or functionality of the linked SNPs is still elusive and only can be validated empirically through further experimentation (Hazelett et al., 2016; Gallagher and Chen-Plotkin, 2018). Non-normality of the data can also be a significant factor that increases error and reduces statistical power (Yoosefzadeh-Najafabadi et al., 2022). When applying GWAS, the risk of finding spurious correlations is ever-present, thus careful consideration must be taken into the model to correct when working with complex traits (Ball, 2013).
As the complexity of genetic architecture increases (Boyle et al., 2017), GWAS methods often fail to identify all genetic polymorphisms that have an effect on the phenotype. This phenomenon, known as missing heritability (Brachi et al., 2011), occurs when the genotype identified with these statistical methods does not fully explain the target characteristics. Missing heritability is thought to be caused partially by polymorphisms that have a small correlation with the target trait, and thus not being significant after Bonferroni correction (López-Cortegano and Caballero, 2019). Bonferroni correction is a method of adjusting p values when conducting multiple simultaneous tests on the same dataset; it involves dividing the initial p value by the number of hypotheses tested. In the context of GWAS, the relationship between specific SNPs and the desired trait is considered a comparison, so the p value is divided by the number of SNPs in the data (Napierala, 2012; Tam et al., 2019). However, Bonferroni correction has its drawbacks, for instance, when dealing with skewed phenotypic data (John et al., 2022). Since many GWAS methods are based on linear regression models, missing heritability could also be addressed with non-linear models (Peng, 2020). Nonetheless, some missing heritability might still be due to an underestimation of the effect sizes of common alleles, unidentified common and rare alleles, epigenetic changes, or in some cases, it might not even be found within genetic information (Marian, 2012; Bourrat et al., 2017). Colinearity is another potential source of reduced efficiency and statistical power for GWAS methodologies, and new strategies are needed to mitigate this limitation (Zhang et al., 2019). Finally, another important limitation of GWAS is high dimensionality of the data (n≪p), where the number of features (e.g. SNPs) is much larger than the number of cases (e.g. genotypes), a common issue with biological data (Ramstein et al., 2019). Several AI concepts have been applied to overcome these limitations and disadvantages of GWAS (Szymczak et al., 2009; Nicholls et al., 2020; Enoma et al., 2022), some of which already include certain explainability (e.g., Mieth et al. (2021), see also Section 6).
Many target traits of GWAS are highly quantitative and complex. Grain yield and drought stress tolerance, for instance, are affected by interactions between underlying component traits (Allard and Bradshaw, 1964; Hammer et al., 2006). In Section 2, for instance, a wealth of physiological mechanisms that influence drought stress tolerance are presented. These interactions, however, can be non-linear (Chang and Zhu, 2017), which is a relevant challenge in GWAS. In this context, Technow et al. (2015) proposes to incorporate a crop growth model (CGM) directly into genomic analysis. Crop growth models can simulate biological and physical processes in agricultural systems including plants, environment and management (Holzworth et al., 2014). Relevant CGMs in this context need to include genotype-specific parameters (Oliveira et al., 2021). As a result, these models can capture the effects of non-linear interactions between underlying component traits on target traits (Technow et al., 2015). Gu et al. (2014), for instance, applied QTL mapping and the crop growth model GECROS to investigate the effect of genetic variation in leaf photosynthetic rate on crop biomass in rice. Furthermore, CGMs can help in identifying ideotypes to improve target traits and suitability to specific weather and management conditions (Chang et al., 2019; Bogard et al., 2021). Collins et al. (2021), for example, investigated drought adaptation in Australian wheat using the crop growth model APSIM and suggests limited-transpiration rate at high evaporative demand as a promising trait for selection by breeders.
5 GWAS to dissect drought tolerance in wheat
Despite its limitations, GWAS has become a crucial method for discovering loci for traits of interest, as discussed in the previous section. Drought is one of the most important abiotic stressors affecting wheat yield (Heino et al., 2023), prompting scientists and breeders to identify loci associated with drought stress tolerance.
In addition to grain yield sensu stricto, numerous other drought stress-related traits have been studied in wheat, including plant height and root architecture, as well as phenological traits like days to heading, anthesis or maturity (Mwadzingeni et al., 2016; Molero et al., 2019; Khadka et al., 2020; Saini et al., 2022). A summary of selected characterised markers and their associated traits in the context of drought tolerance in wheat, including the GWAS method used, is given in Table 3 and will be further detailed in the subsequent sections.
5.1 Introduction to developmental stages and yield components in wheat
To characterise marker-trait associations (MTAs) in the context of drought, it is essential to understand the developmental stages of wheat and to know at which stage drought can impact the traits of interest that might also affect grain yield, e.g. yield components, as highlighted in Figure 3. For the classification of the developmental stages, we use the commonly applied BBCH-code (Hack et al., 1992). Yield components are generally targets of high importance in plant breeding (Araus et al., 2008). In cereals, grain yield is described as the number of grains per m2 multiplied by the average grain size. The number of grains per m2 can be further differentiated into the number of spikes per m2 and the number of grains per spike. Spikes per m2 and grains per spike are established during the vegetative stage before anthesis, while the average grain size is mainly determined later during the generative stage (Geisler, 1983).
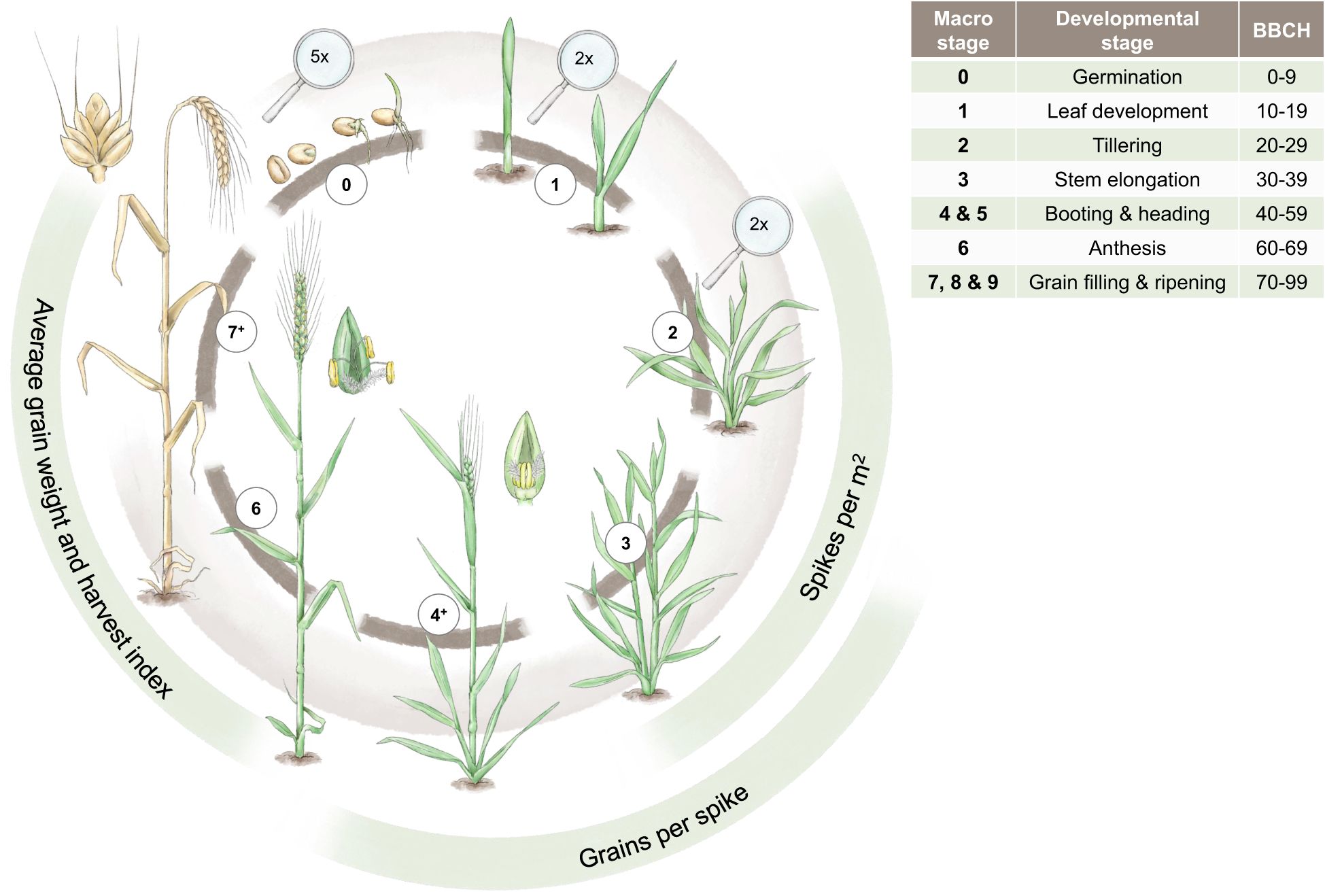
Figure 3 The main developmental stages of winter wheat from germination to ripening are depicted. These developmental stages are contrasted by the most important yield components (spikes/m2, grains/spike, and average grain weight and harvest index) as well as the BBCH classification in the adjacent table. The magnifying glasses indicate a magnification for the respective drawings. Designed by Tatjana Hirschmugl.
The number of spikes per m2 is the first yield component determined during plant development. During the tiller differentiation process (BBCH 20, tillering stage, cf. Figure 3), the maximum number of tillers is established. Transitioning from BBCH 20 to BBCH 30 (stem elongation stage, cf. Figure 3), the number of established tillers is reduced to productive, spike-bearing tillers. Both the differentiation and reduction process of tillers are affected by drought stress. The tiller reduction process, however, is much more sensitive to water shortage than the respective differentiation process (Geisler, 1983). The differentiation process of generative organs, e.g., grains, can be divided into the establishment of spikelets and florets, whereby the primordia of spikelets are already developed by the end of tillering stage (BBCH 20). During stem elongation (cf. Figure 3), most spikelets and florets differentiate, and the maximum number of spikelets and florets is present at the beginning of BBCH 50 (heading). Afterwards, reduction processes of spikelets and florets occur until anthesis. The developmental stages from heading until anthesis are especially sensitive to drought stress González-Navarro et al. (2015). If drought stress is too severe, shedding of fertilised florets can occur after anthesis. Furthermore, insufficient water supply can also shorten the period for spikelet differentiation and thus reduce the number of spikelets per spike. In comparison to the simultaneous tiller reduction processes during stem elongation stage, this effect is minor (Geisler, 1983). Starting with anthesis (BBCH 60), the differentiation process of the caryopsis (the grain) occurs, which determines average grain weight (cf. Figure 3). In general, the longer the grain filling period during the stages of grain development and ripening (BBCH 70 and 80), the higher the average grain weight is (Ozturk et al., 2006; Klepeckas et al., 2020). The duration of this phase, however, is highly affected by environmental conditions. High temperature and insufficient water supply lead to shorter grain filling periods and thus a low average grain weight and even shrivelled grains (Spiertz, 1974; Klepeckas et al., 2020), as well as a shorter duration for the translocation of assimilates to the grain and thus lowers the harvest index of wheat (Davidson and Campbell, 2011; Neugschwandtner et al., 2015; Koppensteiner et al., 2022).
5.2 Markers associated to yield components under drought stress
As the processes of differentiation and reduction for each yield component occur at different developmental stages, they can be significantly affected by temporal environmental conditions (Satorre and Slafer, 2000). For instance, high temperature and water shortages can result in (i) accelerated plant development and consequently shorter differentiation processes for yield components, (ii) more intense reduction processes of individual yield components, and (iii) decreased photosynthetic activity, resulting in fewer available assimilates for grain filling. Besides environmental effects, yield components generally also depend on the genotype and crop management practices, such as sowing, fertilisation, plant protection, and irrigation (Geisler, 1983).
Numerous MTAs have already been characterised in experiments comparing wheat varieties and their responses to drought (Table 3). For example, Mathew et al. (2019) discovered associations between markers and biomass allocation to grain yield. Mwadzingeni et al. (2017) identified 334 MTAs with high confidence for traits under both drought and non-drought conditions. However, these markers explain only 20% of the phenotypic variation, which could be a consequence of the statistical stringency inherent in the methodology. The study found that chromosome 5 in genome D included QTLs related to grain yield, as seen in Quarrie et al. (2005). Among the 29 MTAs found for grain yield, some were located in genes annotated as F-box family protein or Sentrin-specific protease, described to have a potential role in drought stress tolerance (Bhatta et al., 2018). Markers such as Xwmc273.3 and Xpsp3094.1 have been used in the context of MAS of the yield-related QTL Qyld.csdh.7AL to develop high-yielding drought tolerant genotypes (Gautam et al., 2021). Bilgrami et al. (2020) identified SNPs (IWB39005 and IWB44377) related to the number of fertile tillers and total tillers. Suliman et al. (2021) explored grain yield and found 192 related markers, where 25 highly significant SNPs on chromosome 5A have a notable effect on grain yield, making this chromosome a relevant target for yield improvement under drought conditions. Seedling length, days to wilting, and leaf wilting were analysed in Ahmed et al. (2021), who reported 104 associated markers. Multiple phenotypic traits related to yield were used by Qaseem et al. (2018) for GWAS, resulting in 136 MTAs relevant for winter wheat’s positive response to drought conditions.
5.3 Traits associated with phenology under drought conditions
It is well described that each developmental stage has its own specific water supply requirements. If drought occurs during water-sensitive developmental stages (cf. Figure 3), such as germination, tillering, flowering, or grain filling (Yu et al., 2018; Senapati et al., 2021; Xu et al., 2022), growth and subsequently yield can be significantly impacted (Khadka et al., 2020). Therefore, the effects of the concurrence of critical phenological stages and drought conditions are critical (Langridge and Reynolds, 2021). Thus, in traditional plant breeding, phenological parameters are measured by expert-assessed visual scorings. Selection based on phenological characteristics is then conducted by investigating the coincidence of critical developmental stages with drought, heat, or other harsh environmental conditions (Sallam et al., 2019). Maulana et al. (2020) describes drought-related MTAs at the seedling stage of wheat (Table 3). In addition, drought stress during stem elongation can lead to yield reduction up to 71.52% (Ding et al., 2018). Early vigour, the rapid development of leaf area, has been genetically determined by 41 markers associated either with the NDVI or the projected leaf area, which could be used to select for varieties equipped with early vigour in the future (Vukasovic et al., 2022). Farhad et al. (2023) discovered several QTLs (i.e. QDtb.bisa.2D.4) that significantly relate to a shift in the time until booting (days to booting) towards earlier planting. MTAs on chromosomes 2B, 3A and 3D have been found to be related to the number of days to anthesis (Molero et al., 2019). Utilising genetics to select suitable varieties based on phenology is an important technique to face intense drought events. Understanding the link between genotype and phenology is essential to maximise grain yield in these scenarios.
Although these findings are significant and represent a substantial step towards crop optimisation against drought, there remains a large portion of heritability that is unaccounted for (see Section 4). As a result, multiple markers that could be useful for MAS might have gone unidentified. This missing heritability could be due to multiple testing correction or because the statistical tests assume a different distribution than that present in the actual data (Brachi et al., 2011), needing the development of new methods to tackle these issues.
6 Accelerating plant breeding processes with explainable AI
Artificial intelligence (AI) is now applied in many areas of the life sciences, thanks to the significant success of ML and particularly neural networks (NNs) as problem solvers (Holzinger et al., 2023a), which also has been enabled by the constant increase in computing power and resources. AI has already made its way into modern crop breeding, being used in the analysis of the increasing amount of plant image data, as well as in the modelling of GS and GWAS, overcoming some of the limitations of commonly used statistical methods (Harfouche et al., 2019; Jeon et al., 2023; Najafabadi et al., 2023). However, many AI algorithms have their caveats, as they often lack explainability and transparency due to their complex architecture. This is commonly referred to as the ‘black box problem’ (Castelvecchi, 2016), which can ultimately lead to the inability to provide users with explanations for their decisions. The emerging field of explainable AI (xAI) introduces new methods aiming to make AI systems more transparent and understandable (Arrieta et al., 2020; Miller et al., 2022; Holzinger et al., 2022b), laying the foundation for the digital transformation of smart agriculture, and especially plant breeding (Harfouche et al., 2019; Holzinger et al., 2022a).
6.1 Introduction into xAI methods
Although numerous xAI methods have been developed, and new ones continue to emerge for various NN architectures, no single xAI method or combination fully explains the decision-making process of the models. Each of them sheds light on a different aspect of the AI model’s computation and many times it has been shown that there is no mutual consent between them, leading to the so-called ‘disagreement’ problem (Krishna et al., 2022). Currently, quality metrics for xAI methods (Doumard et al., 2023; Schwalbe and Finzel, 2023) and benchmarks for its evaluation are being defined (Agarwal et al., 2023) to motivate xAI research in directions that support trustworthy, reliable, actionable and causal explanations even if they don’t always align with human pre-conceived notions and expectations (Holzinger et al., 2019; Magister et al., 2021; Finzel et al., 2022; Saranti et al., 2022; Cabitza et al., 2023; Holzinger et al., 2023c).
xAI methods have a coarse division between post-hoc and ante-hoc methods: the post-hoc ones are applied after the training has produced ‘sufficiently’ good results in terms of performance. For example, local interpretable model-agnostic explanation (LIME) (Ribeiro et al., 2016), which constructs local linear explanation models from the synthetic neighbourhood around the inputs, and Shapley additive explanations (SHAPs) (Shapley, 1952; Staniak and Biecek, 2019; Frye et al., 2020; Gevaert et al., 2023), which use game-theoretic notions to measure how influential features are to the prediction of a model, are procedures that could give scientists an interpretation of the ‘black box’ (Bach et al., 2015; Montavon et al., 2019; Amparore et al., 2021; den Broeck et al., 2022; Holzinger et al., 2022b). Counterfactual explanations, inspired by the work of Judea Pearl (Pearl and Mackenzie, 2018), are defined as all possibilities that deviate from the main course of events. In similar terms, the question ‘what if’ is applicable to counterfactual explanations that aim to provide information about features that, if they had different values, would result in a different output for the classification/regression problem (Sokol and Flach, 2019; Dandl et al., 2020). On the other hand, ante-hoc methods do not consist of individual software components applied after the model has converged and its internal parameters have solidified. Instead, they are models with built-in explainability. Decision trees (DTs) are one of the most representative models in this category and are widely used. They divide the space of possibilities into parts separated by feature ranges, making this method one of the easiest to understand (Safavian and Landgrebe, 1991). Generalised Additive Models go beyond linear and logistic regression, allowing the output to be expressed as an additive combination of pre-specified non-linear functions (Wood, 2004). Typically, the family of B-splines provides a balance between good performance and interpretability since these functions can be considered as individual and non-interacting. Bayesian Rule Lists contain IF-THEN statements in a list that describes the decision of the model (Letham et al., 2015). The Bayesian rule comes with the definition of a Dirichlet prior that specifies the number of pseudo-counts for a probability distribution, which is defined by a human domain expert (Koller and Friedman, 2009; Holzinger et al., 2023b). The posterior distribution is computed by a Bayesian update rule and incorporation of the number of times one observed each output label.
Layer-wise relevance propagation (LRP) (Bach et al., 2015; Montavon et al., 2019) is a propagation-based method that uses the model’s internal decision parameters to redistribute explanatory factors over the layers of the model, reaching the input variables and obtaining how important those are to the prediction and the model. While the computation of relevance of each feature or input component is something that is achieved by other methods, like sensitivity analysis (SA) (Simonyan et al., 2013) or SHAP, LRP uniquely computes both positive and negative relevance values. This is particularly important since the components that have positive relevance ‘speak for’ the result (e.g., the predicted class in a classification task), whereas those with negative relevance denote elements that contain evidence against the prediction and weaken the prediction confidence of the model. While this method is applied after the training of the model is accomplished, it is not entirely agnostic about the internal structure of the model. LRP has different variations for different NN architectures; for example, Long short-term memory (Hochreiter and Schmidhuber, 1997) networks have an adequately adapted LRP variation (Arras et al., 2017) that enables perturbation analysis of the input sequence and correspondingly graph neural networks (GNNs) have GNN-LRP (Schnake et al., 2020; Xiong et al., 2022) that uncovers positively and negatively important graph paths. LRP has been used for uncovering spurious correlations (so-called Clever-Hans phenomena) between the input and the output of an NN and also for clustered explanations with Whole Dataset Analysis (Lapuschkin et al., 2019).
6.2 Explainable AI methods for modern plant breeding
The plant breeding process, in its entirety, necessitates a high degree of transparency and explainability. Breeders, for instance, need more than just a predictive value to support their selection of genotypes; they rely on a wealth of information to understand the underlying biology and environmental interactions (Harfouche et al., 2019). xAI can be used to confer these qualities into effective ML models at several steps of the breeding process and in a multitude of ways:
● Processing of UAV-sourced data: AI is required to uncover the complex relationships between remotely acquired visual feedback and phenotypical traits (see Section 3.3). This is often a statistically ill-posed problem due to the challenges of replicating exact conditions from one year to another, the high number of external factors, and the cost of acquiring large-scale datasets of carefully measured phenotypical traits (Cheng et al., 2023). This statistical ambiguity can lead to both under- and over- fitting depending on the case. In this context, both ante-hoc and post-hoc xAI methods are important, as exemplified in Srivastava et al. (2022) for winter wheat yield prediction. Ante-hoc methods intervene in the form of strong regularisation, or inductive biases, which limit the space of possible models to those that closely follow a human-defined formulation of the problem. For example, Ge et al. (2023) predict rice distribution using a physically interpretable model trained directly using feature interpretation methods. These heavily regularised models often take the form of simple, interpretable algorithmic bricks that are trained to solve specific sub-problems, such as Tang et al. (2022) who integrate the domain knowledge that edge-detection is important directly into their winter wheat lodging detection architecture. Post-hoc methods serve as a necessary human-in-the-loop validation to counteract the difficulty to acquire enough data for a statistically significant validation. They serve as sanity checks that verify if the features deemed important by the model can be traced back to a physically understandable relationship. Such examples abound both inside (Sun et al., 2023) and outside (Temenos et al., 2022) winter wheat literature.
● Understanding genotype-phenotype relationships: AI can assist in unravelling the complex relationship between a plant’s genotype and its phenotype in response to environmental conditions. Especially xAI can identify genetic variants that contribute to these traits, particularly those that have non-linear interactions - something that GWAS cannot do (Santorsola and Lescai, 2023). Feed-forward NNs go beyond association testing and can use several individuals with many SNPs to predict traits with an acceptable performance (Sharma et al., 2020). After the end of the training process, the xAI method DeepLift (Shrikumar et al., 2017) can be applied and computed for each input SNP attribution score that can take both positive and negative values (indicating the direction of contribution to the target variable). The SNPs with the highest attribution values can be thought of as potential causal causes and be investigated further for plausibility although the results of this research show that in cases of highly correlated features, DeepLift can perceive for one and the same model different input features (SNPs) as important. Building on their previous work (Mieth et al., 2016), Mieth et al. (2021) demonstrated that xAI can enhance traditional GWAS methods: NNs combined with statistical testing driven by xAI can provide a robust framework to uncover SNPs that play a decisive role in the classification result of the NN. LRP (Bach et al., 2015; Montavon et al., 2019) computes the relevance of each SNP used in the classification as if they were p values used to compute statistically significant associations. This approach surpasses the deficiencies of previous architectures that required Bonferroni correction for false rejections and returns additional as well as weak associations that might be significant. It also reduces the return of an incorrect association (statistical noise). However, the biological plausibility of the newly discovered SNPs needs to be validated, particularly if there are no existing GWAS results for them yet. Epistasis, the non-linear, non-additive interaction between SNPs, is another important component of this relationship. It is often overlooked by classical GWAS methodologies, prompting the development of many techniques to try and dissect it (Niel et al., 2015). Romero (2022) describes an innovative process of extracting this behaviour from iterative RFs trained on this data (Basu et al., 2018). One of the advantages of using RF models is that their own architecture is easily interpretable Pfeifer et al. (2022).
● Understanding complex interactions: AI can be utilised for modelling and predicting how certain genotypes would react to conditions like drought. Unlike classical statistical multi-omics methods (Yazdani et al., 2022), AI is an effective tool for deciphering the complex interactions within a plant and those interactions a plant has with its environment, such as soil microbiome, weather, and other plants, which can influence its stress tolerance. xAI can provide insights into the reasoning behind these predictions, enhancing our understanding and facilitating targeted breeding strategies. In Niazian and Niedbała (2020), several cases of genotype-environment interactions (G × E) used by several AI models (having as input the genome sequence and output the phenotype) with their corresponding xAI methods were analysed, uncovering the decisive factors for these interactions (Streich et al., 2020). It is also shown that NNs outperform other AI models performance-wise on these tasks most of the time and the sensitivity analysis applied to the NNs detects the most important input variables for a prediction in different tasks such as assessment and classification of genetic diversity, yield component analysis and indirect selection (prediction), yield stability and G × E interaction, biotic and abiotic stress assessment, classical mating designs, and hybrid breeding programmes (Stein et al., 2022).
Scientific progress is based on understanding and explaining observable phenomena, and this is the advantage provided by the use of xAI. AI has been able to find complex relationships between genotype and phenotype, which could not have been found with other methods. However, it is important to apply these techniques with a higher degree of scientific rigour. Methods such as LRP, LIME, or SHAP are able to provide a deeper understanding of the behaviour of the model, and thus of the biological problem, a prerequisite in modern plant breeding (Harfouche et al., 2019).
7 Towards the implementation of modern tools for practical plant breeding
The previous sections have outlined the advantages of employing modern tools, such as GWAS for genetic marker characterisation, UAV-based remote sensing phenotyping, and the integration of xAI into the breeding process. In this concluding section, we aim to provide an overview of the practical tasks undertaken by plant breeders and how the aforementioned tools can improve the current state of the breeding process, as illustrated in Figure 4.
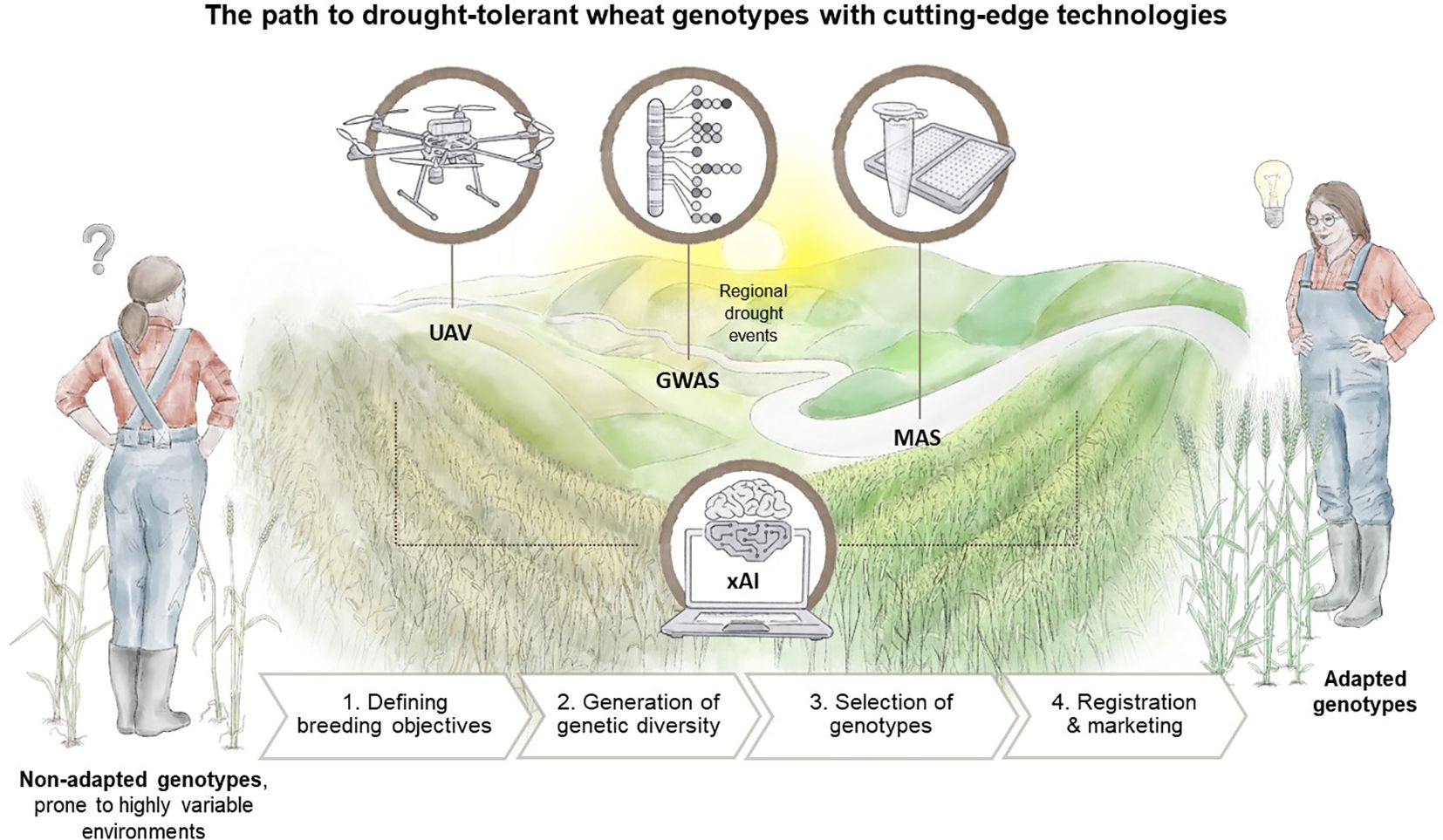
Figure 4 The road to drought-tolerant wheat genotypes remains tedious and time-consuming, but cutting-edge technologies promise to speed up the breeding process. UAV-based phenotyping, GWAS, and ultimately MAS are being increasingly used. However, a cross-process and crucial role is played by xAI, which is not only applied in data analysis and interpretation, but also in decision-making within the entire breeding process, from the definition of breeding goals to the final step of registration and marketing. Designed by Tatjana Hirschmugl.
The responsibilities of a plant breeder can generally be categorised into the following steps: (i) defining the breeding objectives, (ii) creating genetic diversity, and (iii) selecting genotypes. Ultimately, a new variety is registered, certified seed is multiplied, and marketed (cf. Figure 4). The first step involves identifying key traits that will define a future variety. The second step aims to generate a high genetic diversity, particularly in target traits defined in Step 1, often with limited resources, such as a limited number of crosses or mutagenesis treatments. The third step is centred on the selection of candidate genotypes. This step is heavily reliant on data, necessitating efficient data collection and decision-making, often with limited (financial and human) resources, such as scorings, measurements, samplings and laboratory analysis, as well as downstream data analysis. Consequently, the methods and protocols developed by scientists often need to be scaled down or simplified for easy application within the breeding process.
For example, in GWAS, the ultimate objective is to develop markers taking advantage of a plethora of tools (cf. Table 2) with enough precision to predict the presence of a trait of interest. Eventually, these markers (cf. Table 3) should be utilised by the breeder, for instance, for screening potential crossing partners (Step 2) and MAS (Step 3). Saini et al. (2022) reviewed, that 86,122 wheat varieties have been analysed with GWAS, resulting in 46,940 loci for various agronomic, physiological, and quality traits. However, their implementation often remains a challenge in many breeding programmes due to several constraints, such as lack of transferability or additional disproportionate costs. Transferability concerns in GWAS are prevalent mostly between different populations and environments, as was shortly discussed in Section 4.2 (Guo et al., 2014; Blake et al., 2020; Mohammadi et al., 2020). Limited transferability due to relevant genotype by environment interactions can be addressed by, e.g., the inclusion of crop growth models (Technow et al., 2015). Mid-range genotyping platforms like KASP™ (Semagn et al., 2014) or MassArray® (Irwin, 2008) offer a relatively flexible, user-friendly, and affordable solution for practical breeding by being capable of screening tens to hundreds of markers in several hundreds of individuals. Both platforms have already been used to design ready-to-use assays for MAS in diverse sets of diploid crop species (e.g., Bomers et al., 2022), and have been successfully applied in polyploid wheat (Bérard et al., 2009; Rasheed et al., 2016; Makhoul et al., 2020; da Costa Lima Moraes et al., 2023; Liu et al., 2023) or aim to do so (Molin, 2024).
The objective of remote sensing phenotyping is to provide fast and precise phenotypic measurements. Particularly for plant breeding, UAV-based phenotyping offers an optimal combination of spatial resolution and speed of measurement (Figure 2). This data can be used by plant breeders primarily to enhance genotype selection (Step 3), but also to identify phenotypic diversity (Step 2). Numerous studies have applied UAV-based phenotyping in the context of plant breeding in the past (White et al., 2012; Chapman et al., 2014; Araus et al., 2018; Thenkabail et al., 2018), using a variety of sensors including multi- and hyperspectral, thermal, RGB, and LiDAR to investigate traits such as yield, biomass, plant height, crop health and stress, diseases, pests, as well as nutrient and water content (Yang et al., 2009; Prabhakar et al., 2012; Virnodkar et al., 2020; Zhou et al., 2021b; Thoday-Kennedy et al., 2022; Hütt et al., 2023; Joshi et al., 2023). However, its application in practical breeding is still limited (Matese et al., 2023) due to the need for expertise in several areas, such as drone piloting, legislation, flight planning, photogrammetric processing as well as data processing, modelling, and analysis (White et al., 2012; Chapman et al., 2014; Reynolds et al., 2020; Guo et al., 2021).
In the current scientific dialogue, AI has emerged as a vital tool for problem-solving and knowledge discovery, particularly in the life sciences (Holzinger et al., 2023a). Its applications are manifold and extend to specialised fields like plant breeding. In this context, AI facilitates the analysis of plant image data and plays a crucial role in GWAS and genomic selection (Zhang et al., 2017; Parmley et al., 2019; Aono et al., 2022). AI’s usefulness extends beyond data analysis and permeates the entire decision-making pipeline as depicted in Figure 4, from initial data collection and preprocessing (step 1), to feature selection and modelling (step 2), and finally to evaluation and interpretation of results (step 3). The technology’s versatility and computational prowess allow it to process large datasets, discern patterns that may be overlooked by human experts, and provide actionable insights. Essentially, AI acts as a decision support system that enhances the abilities of domain specialists, such as plant breeders, by furnishing them with more accurate and comprehensive information.
The emergence of xAI further enhances the utility of AI in plant breeding. xAI aims to make the complex decision-making processes of AI algorithms transparent and understandable. This is achieved through various methods, such as feature importance ranking, DTs, and counterfactual explanations, among others Holzinger et al. (2021). The increased transparency provided by xAI not only unravels the black-box nature of complex algorithms but also promotes trust and acceptance among human decision-makers. The importance of xAI goes beyond mere understanding of AI’s operations; it addresses ethical and accountability concerns by ensuring that algorithmic decisions can be audited and justified Müller et al. (2022). This is particularly important in high-stakes applications like plant breeding, where decisions can have enduring impacts on agricultural productivity and sustainability. Therefore, the integration of xAI into decision-making processes enhances the trustworthiness and acceptance of AI systems, paving the way for more responsible and effective applications of AI in the life sciences (Holzinger et al., 2022a), including specialised domains such as plant breeding (Harfouche et al., 2019).
In summary, the cutting-edge tools reviewed in this study, encompassing UAV-based phenotyping, GWAS, MAS, bolstered by ML, and the integration of xAI, collectively represent a transformative shift in plant breeding (Figure 4). These innovative methods have the potential to revolutionise the way how breeders gather field data, interpret it, and ultimately make informed decisions throughout the entire breeding process, representing a new era in smart agriculture. By leveraging these technological capabilities, breeders can significantly accelerate the development of new crop varieties with improved traits, such as drought tolerance. This acceleration not only reflects the progress in science and technology but also holds the promise of addressing critical agricultural challenges, such as feeding an expanding global population and mitigating the effects of climate change on crop production.
Author contributions
IC-B: Writing – original draft, Writing – review & editing, Investigation. LJK: Conceptualization, Writing – original draft, Writing – review & editing, Investigation. LB: Writing – original draft, Conceptualization, Investigation, Writing – review & editing. GB: Writing – original draft, Writing – review & editing. AS: Writing – original draft, Writing – review & editing. JS: Writing – review & editing, Writing – original draft. PF-J: Investigation, Supervision, Writing – review & editing, Conceptualization. CS: Supervision, Writing – review & editing. FB: Writing – review & editing. FT: Writing – original draft, Writing – review & editing. MS-Z: Investigation, Writing – review & editing. EZ: Supervision, Writing – review & editing. AH: Funding acquisition, Supervision, Writing – original draft, Writing – review & editing. EMM: Conceptualization, Funding acquisition, Investigation, Project administration, Supervision, Visualization, Writing – original draft, Writing – review & editing.
Funding
The author(s) declare financial support was received for the research, authorship, and/or publication of this article. This review has been conducted in the frame of the d4agrotech initiative (www.d4agrotech.at), in which the project ‘WheatVIZ’ (WST3-F-5030665/018-2022) received funding from the Federal Government of Lower Austria (Grantholder: EMM). Parts of this work have been funded by the Austrian Science Fund (FWF), Project: P-32554 ‘explainable Artificial Intelligence’ (Grantholder: AH).
Acknowledgments
With regard to the data underlying Figure 1A, we acknowledge the World Climate Research Programme, which coordinated and promoted CMIP6, and we thank the climate modelling groups for producing and making available their model output, and the Earth System Grid Federation for archiving the data and providing access. A big thank you goes to Tatjana Hirschmugl (www.scillustration.at) for the design of all illustrations. Many thanks also go to Philipp Karoshi for providing expert knowledge.
Conflict of interest
Authors LJK and MS-Z are employed by the company Saatzucht Edelhof GmbH.
The remaining authors declare that the research was conducted in the absence of any commercial or financial relationships that could be construed as a potential conflict of interest.
The author(s) declared that they were an editorial board member of Frontiers, at the time of submission. This had no impact on the peer review process and the final decision.
Publisher’s note
All claims expressed in this article are solely those of the authors and do not necessarily represent those of their affiliated organizations, or those of the publisher, the editors and the reviewers. Any product that may be evaluated in this article, or claim that may be made by its manufacturer, is not guaranteed or endorsed by the publisher.
Glossary
References
Abdalla, M., Ahmed, M. A., Cai, G., Wankmüller, F., Schwartz, N., Litig, O., et al. (2022). Stomatal closure during water deficit is controlled by below-ground hydraulics. Ann. Bot. 129, 161–170. doi: 10.1093/aob/mcab141
Adão, T., Hruška, J., Pádua, L., Bessa, J., Peres, E., Morais, R., et al. (2017). Hyperspectral imaging: A review on uav-based sensors, data processing and applications for agriculture and forestry. mdpi.com 9, 1110. doi: 10.3390/rs9111110
Affortit, P., Ahmed, M. A., Grondin, A., Delzon, S., Carminati, A., Laplaze, L. (2023). Keep in touch: the soil–root hydraulic continuum and its role in drought resistance in crops. J. Exp. Bot. 75 (2), 584–593. doi: 10.1093/jxb/erad312
Agarwal, C., Queen, O., Lakkaraju, H., Zitnik, M. (2023). Evaluating explainability for graph neural networks. Sci. Data 10, 144. doi: 10.1038/s41597-023-01974-x
AgEagle Aerial Systems Inc (2023) Altum-pt - drone sensors. Available online at: https://ageagle.com/drone-sensors/altumpt-camera/.
AGES (2023). Österreichische Beschreibende Sortenliste 2023 Landwirtschaftliche Pflanzenarten, Schriftenreihe 21/2023. Vienna, Austria: Österreichische Agentur für Gesundheit und Ernährungssicherheit GmbH.
Ahmed, A. A., Mohamed, E. A., Hussein, M. Y., Sallam, A. (2021). Genomic regions associated with leaf wilting traits under drought stress in spring wheat at the seedling stage revealed by gwas. Environ. Exp. Bot. 184, 104393. doi: 10.1016/j.envexpbot.2021.104393
Akram, S., Arif, M. A. R., Hameed, A. (2021). A gbs-based gwas analysis of adaptability and yield traits in bread wheat (triticum aestivum l.). J. Appl. Genet. 62, 27–41. doi: 10.1007/s13353-020-00593-1
Alamin, M., Sultana, M. H., Lou, X., Jin, W., Xu, H. (2022). Dissecting complex traits using omics data: A review on the linear mixed models and their application in gwas. Plants 11, 3277. doi: 10.3390/plants11233277
Allard, R. W., Bradshaw, A. D. (1964). Implications of genotype-environmental interactions in applied plant breeding. Crop Sci. 4, 503–508. doi: 10.2135/cropsci1964.0011183X000400050021x
Aloisi, I., Yacoubi, I., Gadaleta, A., Schwember, A. R., Marcotuli, I. (2023). Editorial: Exploiting wheat biodiversity and agricultural practices for tackling the effects of climate change. Front. Plant Sci. 14. doi: 10.3389/fpls.2023.1257502
Amparore, E., Perotti, A., Bajardi, P. (2021). To trust or not to trust an explanation: using leaf to evaluate local linear xai methods. PeerJ Comput. Sci. 7, e479. doi: 10.7717/peerj-cs.479
Anderegg, J., Yu, K., Aasen, H., Walter, A., Liebisch, F., Hund, A. (2020). Spectral vegetation indices to track senescence dynamics in diverse wheat germplasm. Front. Plant Sci. 10. doi: 10.3389/fpls.2019.01749
Ang, K. L.-M., Seng, J. K. P. (2021). Big data and machine learning with hyperspectral information in agriculture. IEEE Access 9, 36699–36718. doi: 10.1109/Access.6287639
>Ansarifar, J., Wang, L., Archontoulis, S. V. (2021). An interaction regression model for crop yield prediction. Sci. Rep. 11, 17754. doi: 10.1038/s41598-021-97221-7
Aono, A. H., Ferreira, R. C. U., da Costa Lima Moraes, A., de Castro Lara, L. A., Pimenta, R. J. G., Costa, E. A., et al. (2022). A joint learning approach for genomic prediction in polyploid grasses. Sci. Rep. 12, 12499. doi: 10.1038/s41598-022-16417-7
Appels, R., Eversole, K., Stein, N., Feuillet, C., Keller, B., Rogers, J., et al. (2018). Shifting the limits in wheat research and breeding using a fully annotated reference genome. Science 361, eaar7191. doi: 10.1126/science.aar7191
Araus, J. L., Kefauver, S. C., Zaman-Allah, M., Olsen, M. S., Cairns, J. E. (2018). Translating high-throughput phenotyping into genetic gain. Trends Plant Sci. 23, 451–466. doi: 10.1016/j.tplants.2018.02.001
Araus, J. L., Slafer, G. A., Royo, C., Serret, M. D. (2008). Breeding for yield potential and stress adaptation in cereals. Crit. Rev. Plant Sci. 27, 377–412. doi: 10.1080/07352680802467736
Arras, L., Montavon, G., Müller, K. R., Samek, W. (2017). “Explaining recurrent neural network predictions in sentiment analysis,” in EMNLP 2017 - 8th Workshop on Computational Approaches to Subjectivity, Sentiment and Social Media Analysis, WASSA 2017 - Proceedings of the Workshop. 159–168. doi: 10.18653/v1/w17-5221
Arrieta, A. B., Díaz-Rodríguez, N., Ser, J. D., Bennetot, A., Tabik, S., Barbado, A., et al. (2020). Explainable artificial intelligence (xai): Concepts, taxonomies, opportunities and challenges toward responsible ai. Inf. Fusion 58, 82–115. doi: 10.1016/j.inffus.2019.12.012
Arruda, M., Lipka, A., Brown, P., Krill, A., Thurber, C., Brown-Guedira, G., et al. (2016). Comparing genomic selection and marker-assisted selection for fusarium head blight resistance in wheat (triticum aestivum l.). Mol. Breed. 36, 1–11. doi: 10.1007/s11032-016-0508-5
Atkinson, J. A., Jackson, R. J., Bentley, A. R., Ober, E., Wells, D. M. (2018). Field Phenotyping for the Future (Nottingham, UK: Wiley). doi: 10.1002/9781119312994.apr0651
Bach, S., Binder, A., Montavon, G., Klauschen, F., Müller, K.-R., Samek, W. (2015). On pixel-wise explanations for non-linear classifier decisions by layer-wise relevance propagation. PloS One 10, e0130140. doi: 10.1371/journal.pone.0130140
Bai, G., Ge, Y., Hussain, W., Baenziger, P. S., Graef, G. (2016). A multi-sensor system for high throughput field phenotyping in soybean and wheat breeding. Comput. Electron. Agric. 128, 181–192. doi: 10.1016/j.compag.2016.08.021
Ball, R. D. (2013). Designing a gwas: Power, sample size, and data structure. Methods Mol. Biol. 1019, 37–98. doi: 10.1007/978-1-62703-447-03/COVER
Basu, S., Kumbier, K., Brown, J. B., Yu, B. (2018). Iterative random forests to discover predictive and stable high-order interactions. Proc. Natl. Acad. Sci. 115, 1943–1948. doi: 10.1073/pnas.1711236115
Becker, S. R., Byrne, P. F., Reid, S. D., Bauerle, W. L., McKay, J. K., Haley, S. D. (2016). Root traits contributing to drought tolerance of synthetic hexaploid wheat in a greenhouse study. Euphytica 207, 213–224. doi: 10.1007/s10681-015-1574-1
Becker, E., Schmidhalter, U. (2017). Evaluation of yield and drought using active and passive spectral sensing systems at the reproductive stage in wheat. Front. Plant Sci. 8. doi: 10.3389/fpls.2017.00379
Beltrame, L., Salzinger, J., Fanta-Jende, P., Sulzbachner, C. (2024). “Practical strategies for automated phenotyping: from raw UAV data to multispectral time series for machine learning applications,” in Vereinigung der Pflanzenzüchter und Saatgutkaufleute Österreichs (Ed), 74. Jahrestagung 2023, 20-22 November, Raum-berg-Gumpenstein. (Vienna, Austria: University of Natural Resources and Life Sciences), 5–10.
Bennani, S., Birouk, A., Jlibene, M., Sanchez-Garcia, M., Nsarellah, N., Gaboun, F., et al. (2022). Drought-tolerance qtls associated with grain yield and related traits in spring bread wheat. Plants 11, 986. doi: 10.3390/plants11070986
Bérard, A., Paslier, M. C. L., Dardevet, M., Exbrayat-Vinson, F., Bonnin, I., Cenci, A., et al. (2009). High-throughput single nucleotide polymorphism genotyping in wheat (¡i¿triticum¡/i¿ spp.). Plant Biotechnol. J. 7, 364–374. doi: 10.1111/j.1467-7652.2009.00404.x
Bhandari, M., Ibrahim, A. M., Xue, Q., Jung, J., Chang, A., Rudd, J. C., et al. (2020). Assessing winter wheat foliage disease severity using aerial imagery acquired from small unmanned aerial vehicle (uav). Comput. Electron. Agric. 176, 105665. doi: 10.1016/j.compag.2020.105665
Bhatta, M., Morgounov, A., Belamkar, V., Baenziger, P. (2018). Genome-wide association study reveals novel genomic regions for grain yield and yield-related traits in drought-stressed synthetic hexaploid wheat. Int. J. Mol. Sci. 19, 3011. doi: 10.3390/ijms19103011
Bilgrami, S. S., Ramandi, H. D., Shariati, V., Razavi, K., Tavakol, E., Fakheri, B. A., et al. (2020). Detection of genomic regions associated with tiller number in Iranian bread wheat under different water regimes using genome-wide association study. Sci. Rep. 10, 14034. doi: 10.1038/s41598-020-69442-9
Blake, R., Gina, B.-G., H., S. C., Mohsen, M. (2020). Transferability of marker trait associations in wheat is disturbed mainly by genotype × year interaction. Crop Breeding Genet. Genomics. 4. doi: 10.20900/cbgg20200013
Blum, A. (2009). Effective use of water (euw) and not water-use efficiency (wue) is the target of crop yield improvement under drought stress. Field Crops Res. 112, 119–123. doi: 10.1016/j.fcr.2009.03.009
Blum, A. (2011). Plant Breeding for Water-Limited Environments (Amsterdam, Netherlands: Springer New York). doi: 10.1007/978-1-4419-7491-4
Bodner, G., Nakhforoosh, A., Kaul, H.-P. (2015). Management of crop water under drought: a review. Agron. Sustain. Dev. 35, 401–442. doi: 10.1007/s13593-015-0283-4
Bogard, M., Hourcade, D., Piquemal, B., Gouache, D., Deswartes, J.-C., Throude, M., et al. (2021). Marker-based crop model-assisted ideotype design to improve avoidance of abiotic stress in bread wheat. J. Exp. Bot. 72, 1085–1103. doi: 10.1093/jxb/eraa477
Bomers, S., Sehr, E. M., Adam, E., von Gehren, P., Hansel-Hohl, K., Prat, N., et al. (2022). Towards heat tolerant runner bean (phaseolus coccineus l.) by utilizing plant genetic resources. Agronomy 12, 612. doi: 10.3390/agronomy12030612
Bouaziz, M., Ambroise, C., Guedj, M. (2011). Accounting for population stratification in practice: A comparison of the main strategies dedicated to genome-wide association studies. PloS One 6, e28845. doi: 10.1371/journal.pone.0028845
Bourrat, P., Lu, Q., Jablonka, E. (2017). Why the missing heritability might not be in the dna. BioEssays 39, 1700067. doi: 10.1002/bies.201700067
Boyle, E. A., Li, Y. I., Pritchard, J. K. (2017). An expanded view of complex traits: From polygenic to omnigenic. Cell 169, 1177–1186. doi: 10.1016/j.cell.2017.05.038
Brachi, B., Morris, G. P., Borevitz, J. O. (2011). Genome-wide association studies in plants: the missing heritability is in the field. Genome Biol. 12, 232. doi: 10.1186/gb-2011-12-10-232
Bradbury, P. J., Zhang, Z., Kroon, D. E., Casstevens, T. M., Ramdoss, Y., Buckler, E. S. (2007). Tassel: software for association mapping of complex traits in diverse samples. Bioinformatics 23, 2633–2635. doi: 10.1093/bioinformatics/btm308
Brisson, N., Gate, P., Gouache, D., Charmet, G., Oury, F. X., Huard, F. (2010). Why are wheat yields stagnating in europe? a comprehensive data analysis for France. Field Crops Res. 119, 201–212. doi: 10.1016/j.fcr.2010.07.012
Budhlakoti, N., Kushwaha, A. K., Rai, A., Chaturvedi, K., Kumar, A., Pradhan, A. K., et al. (2022). Genomic selection: a tool for accelerating the efficiency of molecular breeding for development of climate-resilient crops. Front. Genet. 13. doi: 10.3389/fgene.2022.832153
Buerstmayr, H., Steiner, B., Lemmens, M., Ruckenbauer, P. (2000). Resistance to fusarium head blight in winter wheat: Heritability and trait associations. Crop Sci. 40, 1012–1018. doi: 10.2135/cropsci2000.4041012x
Cabitza, F., Campagner, A., Malgieri, G., Natali, C., Schneeberger, D., Stoeger, K., et al. (2023). Quod erat demonstrandum? - towards a typology of the concept of explanation for the design of explainable ai. Expert Syst. Appl. 213, 118888. doi: 10.1016/j.eswa.2022.118888
Carlson, T. N., Ripley, D. A. (1997). On the relation between ndvi, fractional vegetation cover, and leaf area index. Remote Sens. Environ. 62, 241–252. doi: 10.1016/S0034-4257(97)00104-1
Carminati, A., Vetterlein, D. (2013). Plasticity of rhizosphere hydraulic properties as a key for efficient utilization of scarce resources. Ann. Bot. 112, 277–290. doi: 10.1093/aob/mcs262
Cattivelli, L., Rizza, F., Badeck, F. W., Mazzucotelli, E., Mastrangelo, A. M., Francia, E., et al. (2008). Drought tolerance improvement in crop plants: An integrated view from breeding to genomics. Field Crops Res. 105, 1–14. doi: 10.1016/j.fcr.2007.07.004
Chandel, N. S., Chakraborty, S. K., Rajwade, Y. A., Dubey, K., Tiwari, M. K., Jat, D. (2021). Identifying crop water stress using deep learning models. Neural Computing Appl. 33, 5353–5367. doi: 10.1007/s00521-020-05325-4
Chang, T.-G., Chang, S., Song, Q.-F., Perveen, S., Zhu, X.-G. (2019). Systems models, phenomics and genomics: three pillars for developing high-yielding photosynthetically efficient crops. silico Plants 1, diy003. doi: 10.1093/insilicoplants/diy003
Chang, C. C., Chow, C. C., Tellier, L. C., Vattikuti, S., Purcell, S. M., Lee, J. J. (2015). Secondgeneration plink: Rising to the challenge of larger and richer datasets. GigaScience 4, 7. doi: 10.1186/s13742-015-0047-8
Chang, T.-G., Zhu, X.-G. (2017). Source–sink interaction: a century old concept under the light of modern molecular systems biology. J. Exp. Bot. 68, 4417–4431. doi: 10.1093/jxb/erx002
Chapman, S., Merz, T., Chan, A., Jackway, P., Hrabar, S., Dreccer, M., et al. (2014). Pheno-copter: A low-altitude, autonomous remote-sensing robotic helicopter for high-throughput field-based phenotyping. Agronomy 4, 279–301. doi: 10.3390/agronomy4020279
Chawade, A., van Ham, J., Blomquist, H., Bagge, O., Alexandersson, E., Ortiz, R. (2019). Highthroughput field-phenotyping tools for plant breeding and precision agriculture. Agronomy 9, 258. doi: 10.3390/agronomy9050258
Cheng, X., Sun, Y., Zhang, W., Wang, Y., Cao, X., Wang, Y. (2023). Application of deep learning in multitemporal remote sensing image classification. Remote Sens. 15, 3859. doi: 10.3390/rs15194705
Clyde, D. (2019). Making the case for more inclusive gwas. Nat. Rev. Genet. 20, 500–501. doi: 10.1038/s41576-019-0160-0
Collard, B. C., Mackill, D. J. (2008). Marker-assisted selection: an approach for precision plant breeding in the twenty-first century. Philos. Trans. R. Soc. B: Biol. Sci. 363, 557–572. doi: 10.1098/rstb.2007.2170
Collins, B., Chapman, S., Hammer, G., Chenu, K. (2021). Limiting transpiration rate in high evaporative demand conditions to improve Australian wheat productivity. silico Plants 3, diab006. doi: 10.1093/insilicoplants/diab006
Condorelli, G. E., Maccaferri, M., Newcomb, M., Andrade-Sanchez, P., White, J. W., French, A. N., et al. (2018). Comparative aerial and ground based high throughput phenotyping for the genetic dissection of ndvi as a proxy for drought adaptive traits in durum wheat. Front. Plant Sci. 9. doi: 10.3389/fpls.2018.00893
Cortes, L. T., Zhang, Z., Yu, J. (2021). Status and prospects of genome-wide association studies in plants. Plant Genome 14, e20077. doi: 10.1002/tpg2.20077
Covarrubias-Pazaran, G. (2016). Genome-assisted prediction of quantitative traits using the r package sommer. PloS One 11, e0156744. doi: 10.1371/journal.pone.0156744
Cruiziat, P., Cochard, H., Améglio, T. (2002). Hydraulic architecture of trees: main concepts and results. Ann. For. Sci. 59, 723–752. doi: 10.1051/forest:2002060
Cummings, C., Miao, Y., Paiao, G. D., Kang, S., Fernández, F. G. (2021). Corn nitrogen status diagnosis with an innovative multi-parameter crop circle phenom sensing system. Remote Sens. 13, 401. doi: 10.3390/rs13030401
Czyż, E. A., Dexter, A. R., Czyż, E. A., Dexter, A. R. (2012). Plant wilting can be caused either by the plant or by the soil. Soil Res. 50, 708–713. doi: 10.1071/SR12189
Daba, S. D., Tyagi, P., Brown-Guedira, G., Mohammadi, M. (2018). Genome-wide association studies to identify loci and candidate genes controlling kernel weight and length in a historical United States wheat population. Front. Plant Sci. 9. doi: 10.3389/fpls.2018.01045
da Costa Lima Moraes, A., Sforc¸a, D. A., Mancini, M. C., Vigna, B. B. Z., de Souza, A. P. (2023). Polyploid snp genotyping using the massarray system. Methods Mol. Biol. (Clifton N.J.) 2638, 93–113. doi: 10.1007/978-1-0716-3024-27/COVER
Dallinger, H. G., Löschenberger, F., Azrak, N., Ametz, C., Michel, S., Bürstmayr, H. (2023). Genome-wide association mapping for pre-harvest sprouting in european winter wheat detects novel resistance qtl, pleiotropic effects, and structural variation in multiple genomes. Plant Genome e20301. doi: 10.1002/tpg2.20301
Dandl, S., Molnar, C., Binder, M., Bischl, B. (2020). “Multi-objective counterfactual explanations,” in Lecture Notes in Computer Science (including subseries Lecture Notes in Artificial Intelligence and Lecture Notes in Bioinformatics (Cham, Switzerland: Springer International Publishing), 12269, 448–469. doi: 10.1007/978-3-030-58112-131/FIGURES/6
Darra, N., Anastasiou, E., Kriezi, O., Lazarou, E., Kalivas, D., Fountas, S. (2023). Can yield prediction be fully digitilized? a systematic review. Agronomy 13, 2441. doi: 10.3390/agronomy13092441
Das, S., Christopher, J., Apan, A., Choudhury, M. R., Chapman, S., Menzies, N. W., et al. (2021). Evaluation of water status of wheat genotypes to aid prediction of yield on sodic soils using uav-thermal imaging and machine learning. Agric. For. Meteorology 307, 108477. doi: 10.1016/j.agrformet.2021.108477
Davidson, H. R., Campbell, C. A. (2011). Growth rates, harvest index and moisture use of manitou spring wheat as influenced by nitrogen, temperature and moisture. Can. J. Plant Sci. 64, 825–839. doi: 10.4141/CJPS84-114
Degen, B., Müller, N. (2023). Advanced marker-assisted selection versus genomic selection in breeding programs (Cold Spring Harbor Laboratory eprint). Available at: https://www.biorxiv.org/content/early/2023/02/22/2023.02.20.529263.full.pdf.
den Broeck, G. V., Lykov, A., Schleich, M., Suciu, D. (2022). On the tractability of shap explanations. J. Artif. Intell. Res. 74, 851–886. doi: 10.1613/jair.1.13283
Devate, N. B., Krishna, H., Parmeshwarappa, S. K. V., Manjunath, K. K., Chauhan, D., Singh, S., et al. (2022). Genome-wide association mapping for component traits of drought and heat tolerance in wheat. Front. Plant Sci. 13. doi: 10.3389/fpls.2022.943033
Ding, J., Huang, Z., Zhu, M., Li, C., Zhu, X., Guo, W. (2018). Does cyclic water stress damage wheat yield more than a single stress? PloS One 13, e0195535. doi: 10.1371/JOURNAL.PONE.0195535
Doumard, E., Aligon, J., Escriva, E., Excoffier, J. B., Monsarrat, P., Soulé-Dupuy, C. (2023). A quantitative approach for the comparison of additive local explanation methods. Inf. Syst. 114, 102162. doi: 10.1016/j.is.2022.102162
Duan, T., Chapman, S. C., Guo, Y., Zheng, B. (2017). Dynamic monitoring of ndvi in wheat agronomy and breeding trials using an unmanned aerial vehicle. Field Crops Res. 210, 71–80. doi: 10.1016/j.fcr.2017.05.025
Eitel, J. U., Long, D. S., Gessler, P. E., Smith, A. M. (2007). Using in-situ measurements to evaluate the new rapideye™ satellite series for prediction of wheat nitrogen status. Int. J. Remote Sens. 28, 4183–4190. doi: 10.1080/01431160701422213
Enoma, D. O., Bishung, J., Abiodun, T., Ogunlana, O., Osamor, V. C. (2022). Machine learning approaches to genome-wide association studies. J. King Saud Univ. - Sci. 34, 101847. doi: 10.1016/j.jksus.2022.101847
European Space Agency (2023a) Sentinel-2. Available online at: https://sentinel.esa.int/web/sentinel/missions/sentinel-2.
European Space Agency (2023b) Worldview-3. Available online at: https://earth.esa.int/eogateway/missions/worldview-3.
European Space Agency (2024) Rapideye dataset. Available online at: https://earth.esa.int/eogateway/missions/rapideyedatasection.
Eyring, V., Bony, S., Meehl, G. A., Senior, C. A., Stevens, B., Stouffer, R. J., et al. (2016). Overview of the coupled model intercomparison project phase 6 (cmip6) experimental design and organization. Geoscientific Model. Dev. 9, 1937–1958. doi: 10.5194/gmd-9-1937-2016
Fang, H., Baret, F., Plummer, S., Schaepman-Strub, G. (2019). An overview of global leaf area index (lai): Methods, products, validation, and applications. Rev. Geophysics 57, 739–799. doi: 10.1029/2018RG000608
FAO (2023) Faostat. License: CC BY-NC-SA 3.0 IGO. Available online at: https://www.fao.org/ (Accessed 07-06-2023).
Farhad, M., Tripathi, S., Singh, R., Joshi, A., Bhati, P., Vishwakarma, M., et al. (2023). Gwas for early-establishment qtls and their linkage to major phenology-affecting genes (vrn, ppd, and eps) in bread wheat. Genes 14, 1507. doi: 10.3390/genes14071507
Fei, S., Hassan, M. A., Xiao, Y., Su, X., Chen, Z., Cheng, Q., et al. (2023). Uav-based multi-sensor data fusion and machine learning algorithm for yield prediction in wheat. Precis. Agric. 24, 187–212. doi: 10.1007/s11119-022-09938-8
Fernandez-Gallego, J. A., Lootens, P., Borra-Serrano, I., Derycke, V., Haesaert, G., Roldán-Ruiz, I., et al. (2020). Automatic wheat ear counting using machine learning based on rgb uav imagery. Plant J. 103, 1603–1613. doi: 10.1111/tpj.14799
Fernando, R. L., Garrick, D. (2013). Bayesian methods applied to gwas. Methods Mol. Biol. 1019, 237–274. doi: 10.1007/978-1-62703-447-010/COVER
Finzel, B., Saranti, A., Angerschmid, A., Tafler, D., Pfeifer, B., Holzinger, A. (2022). Generating explanations for conceptual validation of graph neural networks: An investigation of symbolic predicates learned on relevance-ranked sub-graphs. KI - Kunstliche Intelligenz 36, 271–285. doi: 10.1007/s13218-022-00781-7
Fitzgerald, G., Rodriguez, D., O’Leary, G. (2010). Measuring and predicting canopy nitrogen nutrition in wheat using a spectral index—the canopy chlorophyll content index (ccci). Field Crops Res. 116, 318–324. doi: 10.1016/j.fcr.2010.01.010
Frye, C., Rowat, C., Feige, I. (2020). Asymmetric shapley values: incorporating causal knowledge into model-agnostic explainability. Adv. Neural Inf. Process. Syst. 33, 1229–1239. doi: 10.48550/arXiv.1910.06358
Furbank, R. T., Tester, M. (2011). Phenomics - technologies to relieve the phenotyping bottleneck. Trends Plant Sci. 16, 635–644. doi: 10.1016/j.tplants.2011.09.005
Gahlaut, V., Jaiswal, V., Balyan, H. S., Joshi, A. K., Gupta, P. K. (2021). Multi-locus gwas for grain weight-related traits under rain-fed conditions in common wheat (triticum aestivum l.). Front. Plant Sci. 12. doi: 10.3389/fpls.2021.758631
Galieni, A., D’Ascenzo, N., Stagnari, F., Pagnani, G., Xie, Q., Pisante, M. (2021). Past and future of plant stress detection: An overview from remote sensing to positron emission tomography. Front. Plant Sci. 11. doi: 10.3389/fpls.2020.609155
Gallagher, M. D., Chen-Plotkin, A. S. (2018). The post-gwas era: From association to function. Am. J. Hum. Genet. 102, 717–730. doi: 10.1016/j.ajhg.2018.04.002
Galluzzi, G., Seyoum, A., Halewood, M., Noriega, I. L., Welch, E. W. (2020). The role of genetic resources in breeding for climate change: The case of public breeding programmes in eighteen developing countries. Plants 9, 1129. doi: 10.3390/plants9091129
Gao, B. C. (1996). Ndwi—a normalized difference water index for remote sensing of vegetation liquid water from space. Remote Sens. Environ. 58, 257–266. doi: 10.1016/S0034-4257(96)00067-3
Gao, Z., Bian, J., Lu, F., Jiao, Y., He, H. (2023). Triticeae crop genome biology: an endless frontier. Front. Plant Sci. 14. doi: 10.3389/FPLS.2023.1222681
Gaut, B. S., Long, A. D. (2003). The lowdown on linkage disequilibrium. Plant Cell 15, 1502–1506. doi: 10.1105/tpc.150730
Gautam, T., Amardeep, Saripalli, G., Rakhi, Kumar, A., Gahlaut, V., et al. (2021). Introgression of a drought insensitive grain yield qtl for improvement of four Indian bread wheat cultivars using marker assisted breeding without background selection. J. Plant Biochem. Biotechnol. 30, 172–183. doi: 10.1007/s13562-020-00553-0
Ge, J., Zhang, H., Xu, L., Sun, C., Duan, H., Guo, Z., et al. (2023). A physically interpretable rice field extraction model for polsar imagery. Remote Sens. 15, 974. doi: 10.3390/rs15040974
Geisler, G. (1983). Ertragsphysiologie von Kulturarten des Gemässigten Klimas (Berlin and Hamburg, Germany: Parey).
GEO3D (2023) Open titan. Available online at: https://www.geo3d.hr/3d-laser-scanners/teledyne-optech/optech-titan.
Gevaert, A., Saranti, A., Holzinger, A., Saeys, Y. (2023). “Efficient approximation of asymmetric shapley values using functional decomposition,” in Machine Learning and Knowledge Extraction. Eds. Holzinger, A., Kieseberg, P., Cabitza, F., Campagner, A., Tjoa, A. M., Weippl, E. (Springer Nature Switzerland, Cham), 13–30. doi: 10.1007/978-3-031-40837-32
Giménez-Gallego, J., González-Teruel, J. D., Jiménez-Buendía, M., Toledo-Moreo, A. B., Soto-Valles, F., Torres-Sánchez, R. (2019). Segmentation of multiple tree leaves pictures with natural backgrounds using deep learning for image-based agriculture applications. Appl. Sci. 10, 202. doi: 10.3390/APP10010202
Gitelson, A. A., Merzlyak, M. N., Chivkunova, O. B. (2001). Optical properties and nondestructive estimation of anthocyanin content in plant leaves. Photochem. Photobiol. 74, 38–45. doi: 10.1562/0031-8655(2001)074<0038:OPANEO>2.0.CO;2
Gizaw, S. A., Godoy, J. G. V., Garland-Campbell, K., Carter, A. H. (2018). Genome-wide association study of yield and component traits in pacific northwest winter wheat. Crop Sci. 58, 2315–2330. doi: 10.2135/cropsci2017.12.0740
Gogna, A., Zhang, J., Jiang, Y., Schulthess, A. W., Zhao, Y., Reif, J. C. (2023). Filtering for snps with high selective constraint augments mid-parent heterosis predictions in wheat (triticum aestivum l.). Crop J. 11, 166–176. doi: 10.1016/j.cj.2022.06.009
González-Navarro, O. E., Griffiths, S., Molero, G., Reynolds, M. P., Slafer, G. A. (2015). Dynamics of floret development determining differences in spike fertility in an elite population of wheat. Field Crops Res. 172, 21–31. doi: 10.1016/j.fcr.2014.12.001
Grinberg, N. F., Orhobor, O. I., King, R. D. (2020). An evaluation of machine-learning for predicting phenotype: studies in yeast, rice, and wheat. Mach. Learn. 109, 251–277. doi: 10.1007/s10994-019-05848-5
Gu, J., Yin, X., Stomph, T.-J., Struik, P. C. (2014). Can exploiting natural genetic variation in leaf photosynthesis contribute to increasing rice productivity? a simulation analysis. Plant Cell Environ. 37, 22–34. doi: 10.1111/pce.12173
Guan, J., Garcia, D. F., Zhou, Y., Appels, R., Li, A., Mao, L. (2020). The battle to sequence the bread wheat genome: A tale of the three kingdoms. Genomics Proteomics Bioinf. 18, 221–229. doi: 10.1016/j.gpb.2019.09.005
Guo, W., Carroll, M. E., Singh, A., Swetnam, T. L., Merchant, N., Sarkar, S., et al. (2021). Uas-based plant phenotyping for research and breeding applications. Plant Phenomics 2021, e20077. doi: 10.34133/2021/9840192
Guo, Z., Tucker, D. M., Basten, C. J., Gandhi, H., Ersoz, E., Guo, B., et al. (2014). The impact of population structure on genomic prediction in stratified populations. Theor. Appl. Genet. 127, 749–762. doi: 10.1007/s00122-013-2255-x
Habier, D., Fernando, R. L., Kizilkaya, K., Garrick, D. J. (2011). Extension of the bayesian alphabet for genomic selection. BMC Bioinf. 12, 1–12. doi: 10.1186/1471-2105-12-186/FIGURES/2
Haboudane, D., Miller, J. R., Tremblay, N., Zarco-Tejada, P. J., Dextraze, L. (2002). Integrated narrow-band vegetation indices for prediction of crop chlorophyll content for application to precision agriculture. Remote Sens. Environ. 81, 416–426. doi: 10.1016/S0034-4257(02)00018-4
Hack, H., Bleiholder, H., Buhr, L., Meier, U., Schnock-Fricke, U., Weber, E., et al. (1992). Uniform coding of the phenological developmental stages of monocotyledonous and dicotyledonous plants, bbch scale, general. News Sheet German Plant Prot. Service 44, 265–270.
Haghighattalab, A., Pérez, L. G., Mondal, S., Singh, D., Schinstock, D., Rutkoski, J., et al. (2016). Application of unmanned aerial systems for high throughput phenotyping of large wheat breeding nurseries. Plant Methods 12, 1–15. doi: 10.1186/s13007-016-0134-6
Hakula, A., Ruoppa, L., Lehtomäki, M., Yu, X., Kukko, A., Kaartinen, H., et al. (2023). Individual tree segmentation and species classification using high-density close-range multispectral laser scanning data. ISPRS Open J. Photogrammetry Remote Sens. 9, 100039. doi: 10.1016/j.ophoto.2023.100039
Hammer, G., Cooper, M., Tardieu, F., Welch, S., Walsh, B., van Eeuwijk, F., et al. (2006). Models for navigating biological complexity in breeding improved crop plants. Trends Plant Sci. 11, 587–593. doi: 10.1016/j.tplants.2006.10.006
Hammer, P., Hopper, D. (1997). Experimental design (Cambridge, Massachusetts, USA: Iowa State University Ames).
Harfouche, A. L., Jacobson, D. A., Kainer, D., Romero, J. C., Harfouche, A. H., Mugnozza, G. S., et al. (2019). Accelerating climate resilient plant breeding by applying next-generation artificial intelligence. Trends Biotechnol. 37, 1217–1235. doi: 10.1016/j.tibtech.2019.05.007
Hassan, M. A., Yang, M., Rasheed, A., Yang, G., Reynolds, M., Xia, X., et al. (2019). A rapid monitoring of ndvi across the wheat growth cycle for grain yield prediction using a multi-spectral uav platform. Plant Sci. 282, 95–103. doi: 10.1016/j.plantsci.2018.10.022
Hazelett, D. J., Conti, D. V., Han, Y., Olama, A. A. A., Easton, D., Eeles, R. A., et al. (2016). Reducing gwas complexity. Cell Cycle 15, 22–24. doi: 10.1080/15384101.2015.1120928
Heino, M., Kinnunen, P., Anderson, W., Ray, D. K., Puma, M. J., Varis, O., et al. (2023). Increased probability of hot and dry weather extremes during the growing season threatens global crop yields. Sci. Rep. 13, 3583. doi: 10.1038/s41598-023-29378-2
Heremans, S., Dong, Q., Zhang, B., Bydekerke, L., Orshoven, J. V. (2015). Potential of ensemble tree methods for early-season prediction of winter wheat yield from short time series of remotely sensed normalized difference vegetation index and ¡i¿in situ¡/i¿ meteorological data. J. Appl. Remote Sens. 9, 97095. doi: 10.1117/1.JRS.9.097095
Hochberg, U., Rockwell, F. E., Holbrook, N. M., Cochard, H. (2018). Iso/anisohydry: A plant–environment interaction rather than a simple hydraulic trait. Trends Plant Sci. 23, 112–120. doi: 10.1016/j.tplants.2017.11.002
Hochreiter, S., Schmidhuber, J. (1997). Long short-term memory. Neural Comput. 9, 1735–1780. doi: 10.1162/neco.1997.9.8.1735
Hoekstra, A. Y., Mekonnen, M. M. (2012). The water footprint of humanity. Proc. Natl. Acad. Sci. 109, 3232–3237. doi: 10.1073/pnas.1109936109
Holman, F., Riche, A., Michalski, A., Castle, M., Wooster, M., Hawkesford, M. (2016). High throughput field phenotyping of wheat plant height and growth rate in field plot trials using uav based remote sensing. Remote Sens. 8, 1031. doi: 10.3390/rs8121031
Holzinger, A., Keiblinger, K., Holub, P., Zatloukal, K., Müller, H. (2023a). Ai for life: Trends in artificial intelligence for biotechnology. New Biotechnol. 74, 16–24. doi: 10.1016/j.nbt.2023.02.001
Holzinger, A., Langs, G., Denk, H., Zatloukal, K., Müller, H. (2019). Causability and explainability of artificial intelligence in medicine. Wiley Interdiscip. Reviews: Data Min. Knowledge Discovery 9, e1312. doi: 10.1002/widm.1312
Holzinger, A., Malle, B., Saranti, A., Pfeifer, B. (2021). Towards multi-modal causability with graph neural networks enabling information fusion for explainable ai. Inf. Fusion 71, 28–37. doi: 10.1016/j.inffus.2021.01.008
Holzinger, A., Saranti, A., Angerschmid, A., Finzel, B., Schmid, U., Mueller, H. (2023b). Toward human-level concept learning: Pattern benchmarking for ai algorithms. Patterns 4, 100788. doi: 10.1016/j.patter.2023.100788
Holzinger, A., Saranti, A., Angerschmid, A., Retzlaff, C. O., Gronauer, A., Pejakovic, V., et al. (2022a). Digital transformation in smart farm and forest operations needs human-centered ai: Challenges and future directions. Sensors 22, 3043. doi: 10.3390/s22083043
Holzinger, A., Saranti, A., Hauschild, A.-C., Beinecke, J., Heider, D., Roettger, R., et al. (2023c). Humanin-the-loop integration with domain-knowledge graphs for explainable federated deep learning. LNCS 14065, 45–64. doi: 10.1007/978-3-031-40837-34
Holzinger, A., Saranti, A., Molnar, C., Biecek, P., Samek, W. (2022b). “Explainable ai methods - a brief overview,” in Lecture Notes in Computer Science (including subseries Lecture Notes in Artificial Intelligence and Lecture Notes in Bioinformatics (Vienna, Austria: Springer). Vol. 13200. 13–38. doi: 10.1007/978-3-031-04083-2_2/FIGURES/3
Holzworth, D. P., Huth, N. I., deVoil, P. G., Zurcher, E. J., Herrmann, N. I., McLean, G., et al. (2014). Apsim–evolution towards a new generation of agricultural systems simulation. Environ. Model. Software 62, 327–350. doi: 10.1016/j.envsoft.2014.07.009
Hu, Y., Knapp, S., Schmidhalter, U. (2020). Advancing high-throughput phenotyping of wheat in early selection cycles. Remote Sens. 12, 574. doi: 10.3390/rs12030574
Huang, M., Liu, X., Zhou, Y., Summers, R. M., Zhang, Z. (2019). Blink: a package for the next level of genome-wide association studies with both individuals and markers in the millions. GigaScience 8, 1–12. doi: 10.1093/gigascience/giy154
Huang, S., Tang, L., Hupy, J. P., Wang, Y., Shao, G. (2021). A commentary review on the use of normalized difference vegetation index (ndvi) in the era of popular remote sensing. J. Forestry Res. 32, 1–6. doi: 10.1007/s11676-020-01155-1
Huete, A. (1988). A soil-adjusted vegetation index (savi). Remote Sens. Environ. 25, 295–309. doi: 10.1016/0034-4257(88)90106-X
Hutchinson, M. L., Antono, E., Gibbons, B. M., Paradiso, S., Ling, J., Meredig, B. (2017). doi: 10.48550/arXiv.1711.05099
Hütt, C., Bolten, A., Hüging, H., Bareth, G. (2023). Uav lidar metrics for monitoring crop height, biomass and nitrogen uptake: A case study on a winter wheat field trial. PFG - J. Photogrammetry Remote Sens. Geoinformation Sci. 91, 65–76. doi: 10.1007/S41064-022-00228-6
Irwin, D. (2008). The MassARRAY system for plant genomics (Cambridge, Massachusetts, USA: CABI). doi: 10.1079/9781845933821.0098
Jayakodi, M., Schreiber, M., Stein, N., Mascher, M. (2021). Building pan-genome infrastructures for crop plants and their use in association genetics. DNA Res. 28, 1–9. doi: 10.1093/dnares/dsaa030
Jenal, A., Hüging, H., Ahrends, H. E., Bolten, A., Bongartz, J., Bareth, G. (2021). Investigating the potential of a newly developed uav-mounted vnir/swir imaging system for monitoring crop traits—a case study for winter wheat. Remote Sens. 13, 1697. doi: 10.3390/rs13091697
Jeon, D., Kang, Y., Lee, S., Choi, S., Sung, Y., Lee, T.-H., et al. (2023). Digitalizing breeding in plants: A new trend of next-generation breeding based on genomic prediction. Front. Plant Sci. 14. doi: 10.3389/fpls.2023.1092584
Jiang, Y., Li, C. (2020). Convolutional neural networks for image-based high-throughput plant phenotyping: A review. Plant Phenomics 2020, 4152816. doi: 10.34133/2020/4152816
John, M., Ankenbrand, M. J., Artmann, C., Freudenthal, J. A., Korte, A., Grimm, D. G. (2022). Efficient permutation-based genome-wide association studies for normal and skewed phenotypic distributions. Bioinformatics 38, ii5–ii12. doi: 10.1093/bioinformatics/btac455
Joshi, A., Pradhan, B., Gite, S., Chakraborty, S. (2023). Remote-sensing data and deep-learning techniques in crop mapping and yield prediction: a systematic review. Remote Sens. 15, 2014. doi: 10.3390/rs15082014
Kadhim, N., Mourshed, M., Bray, M. (2016). Advances in remote sensing applications for urban sustainability. Euro-Mediterranean J. Environ. Integration 1, 7. doi: 10.1007/s41207-016-0007-4
Kang, H. M., Sul, J. H., Service, S. K., Zaitlen, N. A., yee Kong, S., Freimer, N. B., et al. (2010). Variance component model to account for sample structure in genome-wide association studies. Nat. Genet. 42, 348–354. doi: 10.1038/ng.548
Kattenborn, T., Leitloff, J., Schiefer, F., Hinz, S. (2021). Review on convolutional neural networks (cnn) in vegetation remote sensing. ISPRS J. Photogrammetry Remote Sens. 173, 24–49. doi: 10.1016/j.isprsjprs.2020.12.010
Khadka, K., Earl, H. J., Raizada, M. N., Navabi, A. (2020). A physio-morphological trait-based approach for breeding drought tolerant wheat. Front. Plant Sci. 11. doi: 10.3389/fpls.2020.00715
Khan, Z., Rahimi-Eichi, V., Haefele, S., Garnett, T., Miklavcic, S. J. (2018). Estimation of vegetation indices for high-throughput phenotyping of wheat using aerial imaging. Plant Methods 14, 20. doi: 10.1186/s13007-018-0287-6
Khanal, S., Fulton, J., Shearer, S. (2017). An overview of current and potential applications of thermal remote sensing in precision agriculture. Comput. Electron. Agric. 139, 22–32. doi: 10.1016/j.compag.2017.05.001
Kim, J., Kim, S., Ju, C., Son, H. I. (2019). Unmanned aerial vehicles in agriculture: A review of perspective of platform, control, and applications. IEEE Access 7, 105100–105115. doi: 10.1109/Access.6287639
Klepeckas, M., Januškaitienė, I., Vagusevičienė, I., Juknys, R. (2020). Effects of different sowing time to phenology and yield of winter wheat. Agric. Food Sci. 29, 346–358. doi: 10.23986/afsci.90013
Koc, A., Odilbekov, F., Alamrani, M., Henriksson, T., Chawade, A. (2022). Predicting yellow rust in wheat breeding trials by proximal phenotyping and machine learning. Plant Methods 18, 30. doi: 10.1186/s13007-022-00868-0
Koller, D., Friedman, N. (2009). Probabilistic graphical models: principles and techniques (Cambridge, Massachusetts, USA: MIT press).
Konvalina, P., Moudrý, J., Dotlačil, L., Stehno, Z., Moudrý, J. (2010). Drought tolerance of land races of emmer wheat in comparison to soft wheat. Cereal Res. Commun. 38, 429–439. doi: 10.1556/CRC.38.2010.3.13
Koppensteiner, L. J., Kaul, H.-P., Piepho, H.-P., Barta, N., Euteneuer, P., Bernas, J., et al. (2022). Yield and yield components of facultative wheat are affected by sowing time, nitrogen fertilization and environment. Eur. J. Agron. 140, 126591. doi: 10.1016/j.eja.2022.126591
Korte, A., Farlow, A. (2013). The advantages and limitations of trait analysis with gwas: a review. Plant Methods 9, 29. doi: 10.1186/1746-4811-9-29
Krasting, J. P., John, J. G., Blanton, C., McHugh, C., Nikonov, S., Radhakrishnan, A., et al. (2018). NOAA-GFDL GFDL-ESM4 model output prepared for CMIP6 CMIP. Earth System Grid Fed. doi: 10.22033/ESGF/CMIP6.1407
Krishna, S., Han, T., Gu, A., Pombra, J., Jabbari, S., Wu, S., et al. (2022). The disagreement problem in explainable machine learning: A practitioner’s perspective. arXiv [Preprint]. arXiv:2202.01602. doi: 10.48550/arXiv.2202.01602
Kumar, D., Kushwaha, S., Delvento, C., Žilvinas, L., Vivekanand, V., Svensson, J. T., et al. (2020). Affordable phenotyping of winter wheat under field and controlled conditions for drought tolerance. Agronomy 10, 882. doi: 10.3390/agronomy10060882
Kumar, S., Röder, M. S., Singh, R. P., Kumar, S., Chand, R., Joshi, A. K., et al. (2016). Mapping of spot blotch disease resistance using ndvi as a substitute to visual observation in wheat (triticum aestivum l.). Mol. Breed. 36, 95. doi: 10.1007/s11032-016-0515-6
Lalic, B., Eitzinger, J., Mihailovic, D. T., Thaler, S., Jancic, M. (2013). Climate change impacts on winter wheat yield change – which climatic parameters are crucial in pannonian lowland? J. Agric. Sci. 151, 757–774. doi: 10.1017/S0021859612000640
Langridge, P., Reynolds, M. (2021). Breeding for drought and heat tolerance in wheat. Theor. Appl. Genet. 134, 1753–1769. doi: 10.1007/s00122-021-03795-1
Lapuschkin, S., Wäldchen, S., Binder, A., Montavon, G., Samek, W., Müller, K.-R. (2019). Unmasking clever hans predictors and assessing what machines really learn. Nat. Commun. 10, 1096. doi: 10.1038/s41467-019-08987-4
Lehnert, H., Berner, T., Lang, D., Beier, S., Stein, N., Himmelbach, A., et al. (2022). Insights into breeding history, hotspot regions of selection, and untapped allelic diversity for bread wheat breeding. Plant J. 112, 897–918. doi: 10.1111/tpj.15952
Lehnert, H., Serfling, A., Friedt, W., Ordon, F. (2018). Genome-wide association studies reveal genomic regions associated with the response of wheat (triticum aestivum l.) to mycorrhizae under drought stress conditions. Front. Plant Sci. 9. doi: 10.3389/fpls.2018.01728
Letham, B., Rudin, C., McCormick, T. H., Madigan, D. (2015). Interpretable classifiers using rules and bayesian analysis: Building a better stroke prediction model. Ann. Appl. Stat 9, 1350–1371. doi: 10.1214/15-AOAS848
Levitt, J. (1980). Responses of plants to environmental stresses. Volume II. Water, radiation, salt, and other stresses. 1, 497.
Li, A., Hao, C., Wang, Z., Geng, S., Jia, M., Wang, F., et al. (2022). Wheat breeding history reveals synergistic selection of pleiotropic genomic sites for plant architecture and grain yield. Mol. Plant 15, 504–519. doi: 10.1016/j.molp.2022.01.004
Li, C., Li, L., Reynolds, M. P., Wang, J., Chang, X., Mao, X., et al. (2021). Recognizing the hidden half in wheat: root system attributes associated with drought tolerance. J. Exp. Bot. 72, 5117–5133. doi: 10.1093/jxb/erab124
Li, H., Zhang, Y., Lei, Y., Antoniuk, V., Hu, C. (2019). Evaluating different non-destructive estimation methods for winter wheat (triticum aestivum l.) nitrogen status based on canopy spectrum. Remote Sens. 12, 95. doi: 10.3390/rs12010095
Lichthardt, C., Chen, T.-W., Stahl, A., Stützel, H. (2020). Co-evolution of sink and source in the recent breeding history of winter wheat in Germany. Front. Plant Sci. 10. doi: 10.3389/fpls.2019.01771
Lipka, A. E., Tian, F., Wang, Q., Peiffer, J., Li, M., Bradbury, P. J., et al. (2012). Gapit: genome association and prediction integrated tool. Bioinformatics 28, 2397–2399. doi: 10.1093/bioinformatics/bts444
Liu, X., Huang, M., Fan, B., Buckler, E. S., Zhang, Z. (2016). Iterative usage of fixed and random effect models for powerful and efficient genome-wide association studies. PloS Genet. 12, e1005767. doi: 10.1371/journal.pgen.1005767
Liu, G., Liu, D., Zhang, A., Liu, H., Mia, M. S., Mullan, D., et al. (2023). Identification of kasp markers and candidate genes for drought tolerance in wheat using 90k snp array genotyping of nearisogenic lines targeting a 4bs quantitative trait locus. Theor. Appl. Genet. 136, 1–13. doi: 10.1007/s00122-023-04438-3
Lopes, M. S., Reynolds, M. P. (2010). Partitioning of assimilates to deeper roots is associated with cooler canopies and increased yield under drought in wheat. Funct. Plant Biol. 37, 147. doi: 10.1071/FP09121
López-Cortegano, E., Caballero, A. (2019). Inferring the nature of missing heritability in human traits using data from the gwas catalog. Genetics 212, 891–904. doi: 10.1534/genetics.119.302077
Lu, Y., Chen, D., Olaniyi, E. O., Huang, Y. (2022). Generative adversarial networks (GANs) for image augmentation in agriculture: A systematic review. Comput. Electron. Agric. 200, 107208. doi: 10.1016/j.compag.2022.107208
Lunzer, M., Buerstmayr, M., Grausgruber, H., Müllner, A. E., Fallbacher, I., Buerstmayr, H. (2023). Wheat (triticum aestivum) chromosome 6d harbours the broad spectrum common bunt resistance gene bt11. Theor. Appl. Genet. 136, 1–17. doi: 10.1007/s00122-023-04452-5
Lüttger, A. B., Feike, T. (2018). Development of heat and drought related extreme weather events and their effect on winter wheat yields in Germany. Theor. Appl. Climatology 132, 15–29. doi: 10.1007/s00704-017-2076-y
Ma, J., Zhao, D., Tang, X., Yuan, M., Zhang, D., Xu, M., et al. (2022). Genome-wide association study on root system architecture and identification of candidate genes in wheat (triticum aestivum l.). Int. J. Mol. Sci. 23, 1843. doi: 10.3390/ijms23031843
Mackay, I., Powell, W. (2007). Methods for linkage disequilibrium mapping in crops. Trends Plant Sci. 12, 57–63. doi: 10.1016/j.tplants.2006.12.001
Magister, L. C., Kazhdan, D., Singh, V., Liò, P. (2021). Gcexplainer: Human-in-the-loop concept-based explanations for graph neural networks. arXiv [Preprint]. arXiv:2107.11889. doi: 10.48550/arXiv.2107.11889
Mahlein, A.-K., Oerke, E.-C., Steiner, U., Dehne, H.-W. (2012). Recent advances in sensing plant diseases for precision crop protection. Eur. J. Plant Pathol. 133, 197–209. doi: 10.1007/s10658-011-9878-z
Makhoul, M., Rambla, C., Voss-Fels, K. P., Hickey, L. T., Snowdon, R. J., Obermeier, C. (2020). Overcoming polyploidy pitfalls: a user guide for effective snp conversion into kasp markers in wheat. Theor. Appl. Genet. 133, 2413–2430. doi: 10.1007/s00122-020-03608-x
Manickavelu, A., Hattori, T., Yamaoka, S., Yoshimura, K., Kondou, Y., Onogi, A., et al. (2017). Genetic nature of elemental contents in wheat grains and its genomic prediction: Toward the effective use of wheat landraces from Afghanistan. PloS One 12, e0169416. doi: 10.1371/journal.pone.0169416
MansChadi, A. M., Hammer, G. L., Christopher, J. T., deVoil, P. (2008). Genotypic variation in seedling root architectural traits and implications for drought adaptation in wheat (triticum aestivum l.). Plant Soil 303, 115–129. doi: 10.1007/s11104-007-9492-1
March, R. E. (1999). Gene mapping by linkage and association analysis. Appl. Biochem. Biotechnol. - Part B Mol. Biotechnol. 13, 113–122. doi: 10.1385/MB:13:2:113/METRICS
Marchini, J., Howie, B., Myers, S., McVean, G., Donnelly, P. (2007). A new multipoint method for genome-wide association studies by imputation of genotypes. Nat. Genet. 39, 906–913. doi: 10.1038/ng2088
Marian, A. J. (2012). Elements of ‘missing heritability’. Curr. Opin. Cardiol. 27, 197–201. doi: 10.1097/HCO.0b013e328352707d
Maseda, P. H., Fernandez, R. J. (2006). Stay wet or else: three ways in which plants can adjust hydraulically to their environment. J. Exp. Bot. 57, 3963–3977. doi: 10.1093/jxb/erl127
Matese, A., Czarnecki, J. M. P., Samiappan, S., Moorhead, R. (2023). Are unmanned aerial vehiclebased hyperspectral imaging and machine learning advancing crop science? Trends Plant Sci. 29, 196–209. doi: 10.1016/j.tplants.2023.09.001
Mathew, I., Shimelis, H., Shayanowako, A. I. T., Laing, M., Chaplot, V. (2019). Genome-wide association study of drought tolerance and biomass allocation in wheat. PloS One 14, e0225383. doi: 10.1371/journal.pone.0225383
Matsushita, B., Yang, W., Chen, J., Onda, Y., Qiu, G. (2007). Sensitivity of the enhanced vegetation index (evi) and normalized difference vegetation index (ndvi) to topographic effects: A case study in high-density cypress forest. Sensors 7, 2636–2651. doi: 10.3390/s7112636
Maulana, F., Huang, W., Anderson, J. D., Ma, X. F. (2020). Genome-wide association mapping of seedling drought tolerance in winter wheat. Front. Plant Sci. 11. doi: 10.3389/fpls.2020.573786
Miao, C., Yang, J., Schnable, J. C. (2019). Optimising the identification of causal variants across varying genetic architectures in crops. Plant Biotechnol. J. 17, 893–905. doi: 10.1111/pbi.13023
Miedaner, T., Wilde, F., Steiner, B., Buerstmayr, H., Korzun, V., Ebmeyer, E. (2006). Stacking quantitative trait loci (qtl) for fusarium head blight resistance from non-adapted sources in an european elite spring wheat background and assessing their effects on deoxynivalenol (don) content and disease severity. Theor. Appl. Genet. 112, 562–569. doi: 10.1007/s00122-005-0163-4
Mieth, B., Kloft, M., Rodr´ıguez, J. A., Sonnenburg, S., Vobruba, R., Morcillo-Suárez, C., et al. (2016). Combining multiple hypothesis testing with machine learning increases the statistical power of genomewide association studies. Sci. Rep. 6, 36671. doi: 10.1038/SREP36671
Mieth, B., Rozier, A., Rodriguez, J. A., Höhne, M. M. C., Görnitz, N., Müller, K.-R. (2021). Deepcombi: explainable artificial intelligence for the analysis and discovery in genome-wide association studies. NAR Genomics Bioinf. 3, Iqab065. doi: 10.1093/nargab/lqab065
Miller, T., Hoffman, R., Amir, O., Holzinger, A. (2022). Special issue on explainable artificial intelligence (xai). Artif. Intell. 307, 103705. doi: 10.1016/j.artint.2022.103705
Mills, M. C., Rahal, C. (2019). A scientometric review of genome-wide association studies. Commun. Biol. 2, 9. doi: 10.1038/s42003-018-0261-x
Mohammadi, M., Xavier, A., Beckett, T., Beyer, S., Chen, L., Chikssa, H., et al. (2020). Identification, deployment, and transferability of quantitative trait loci from genome-wide association studies in plants. Curr. Plant Biol. 24, 100145. doi: 10.1016/j.cpb.2020.100145
Mohanty, S. P., Hughes, D. P., Salathé, M. (2016). Using deep learning for image-based plant disease detection. Front. Plant Sci. 7. doi: 10.3389/fpls.2016.01419
Mohsan, S. A. H., Othman, N. Q. H., Li, Y., Alsharif, M. H., Khan, M. A. (2023). Unmanned aerial vehicles (uavs): practical aspects, applications, open challenges, security issues, and future trends. Intelligent Service Robotics. 16, 109–137. doi: 10.1007/s11370-022-00452-4
Molero, G., Joynson, R., Pinera-Chavez, F. J., Gardiner, L., Rivera-Amado, C., Hall, A., et al. (2019). Elucidating the genetic basis of biomass accumulation and radiation use efficiency in spring wheat and its role in yield potential. Plant Biotechnol. J. 17, 1276–1288. doi: 10.1111/pbi.13052
Molin, E. M. (2024). “Wheatviz - an interdisciplinary project to accelerate the breeding of drought-tolerant winter wheat via cutting-edge genomics and uav-based phenotyping by integrating explainable ai,” in Proceedings of the 74th Conference of the Vereinigung der Pflanzenzüchter und Saatgutkaufleute Österreichs, Raumberg-Gumpenstein, Irdning, Austria, 20-22 November 2023 (Vienna, Austria: University of Natural Resources and Life Sciences), 11–12.
Montavon, G., Binder, A., Lapuschkin, S., Samek, W., Müller, K.-R. (2019). “Layer-wise relevance propagation: An overview,” in Explainable AI: Interpreting, Explaining and Visualizing Deep Learning (Springer International Publishing, Cham), 193–209. doi: 10.1007/978-3-030-28954-610
Montenegro, J. D., Golicz, A. A., Bayer, P. E., Hurgobin, B., Lee, H., Chan, C. K., et al. (2017). The pangenome of hexaploid bread wheat. Plant J. 90, 1007–1013. doi: 10.1111/tpj.13515
Mori, M., Inagaki, M., Inoue, T., Nachit, M. (2011). Association of root water-uptake ability with drought adaptation in wheat. Cereal Res. Commun. 39, 551–559. doi: 10.1556/CRC.39.2011.4.10
Muhammad, M. (2021). Artificial Intelligence Algorithms for Polygenic Genotype-Phenotype Predictions. [Master’s thesis]. The University of Queensland.
Mukarram, M., Choudhary, S., Kurjak, D., Petek, A., Khan, M. M. A. (2021). Drought: Sensing, signalling, effects and tolerance in higher plants. Physiologia Plantarum 172, 1291–1300. doi: 10.1111/ppl.13423
Mullan, D. J., Reynolds, M. P. (2010). Quantifying genetic effects of ground cover on soil water evaporation using digital imaging. Funct. Plant Biol. 37, 703. doi: 10.1071/FP09277
Müller, H., Holzinger, A., Plass, M., Brcic, L., Stumptner, C., Zatloukal, K. (2022). Explainability and causability for artificial intelligence-supported medical image analysis in the context of the european in vitro diagnostic regulation. New Biotechnol. 70, 67–72. doi: 10.1016/j.nbt.2022.05.002
Mwadzingeni, L., Shimelis, H., Dube, E., Laing, M. D., Tsilo, T. J. (2016). Breeding wheat for drought tolerance: Progress and technologies. J. Integr. Agric. 15, 935–943. doi: 10.1016/S2095-3119(15)61102-9
Mwadzingeni, L., Shimelis, H., Rees, D. J. G., Tsilo, T. J. (2017). Genome-wide association analysis of agronomic traits in wheat under drought-stressed and non-stressed conditions. PloS One 12, e0171692. doi: 10.1371/journal.pone.0171692
Myneni, R. B., Hall, F. G., Sellers, P. J., Marshak, A. L. (1995). The interpretation of spectral vegetation indexes. IEEE Trans. Geosci. Remote Sens. 33, 481–486. doi: 10.1109/TGRS.1995.8746029
Najafabadi, M. Y., Hesami, M., Eskandari, M. (2023). Machine learning-assisted approaches in modernized plant breeding programs. Genes 14, 777. doi: 10.3390/genes14040777
Nakhforoosh, A., Grausgruber, H., Kaul, H.-P., Bodner, G. (2014). Wheat root diversity and root functional characterization. Plant Soil 380, 211–229. doi: 10.1007/s11104-014-2082-0
Nakhforoosh, A., Grausgruber, H., Kaul, H.-P., Bodner, G. (2015). Dissection of drought response of modern and underutilized wheat varieties according to passioura’s yield-water framework. Front. Plant Sci. 6. doi: 10.3389/fpls.2015.00570
NASA (2023) Landsat science. Available online at: https://landsat.gsfc.nasa.gov/.
Nelder, J. A., Wedderburn, R. W. M. (1972). Generalized linear models. J. R. Stat. Society. Ser. A (General) 135, 370. doi: 10.2307/2344614
Neugschwandtner, R. W., Böhm, K., Hall, R. M., Kaul, H. P. (2015). Development, growth, and nitrogen use of autumn- and spring-sown facultative wheat. Acta Agriculturae Scandinavica Section B — Soil Plant Sci. 65, 6–13. doi: 10.1080/09064710.2014.958522
Nguyen, C., Sagan, V., Skobalski, J., Severo, J. I. (2023). Early detection of wheat yellow rust disease and its impact on terminal yield with multi-spectral uav-imagery. Remote Sens. 15, 3301. doi: 10.3390/rs15133301
Niazian, M., Niedbała, G. (2020). Machine learning for plant breeding and biotechnology. Agriculture 10, 436. doi: 10.3390/agriculture10100436
Nicholls, H. L., John, C. R., Watson, D. S., Munroe, P. B., Barnes, M. R., Cabrera, C. P. (2020). Reaching the end-game for gwas: Machine learning approaches for the prioritization of complex disease loci. Front. Genet. 11. doi: 10.3389/fgene.2020.00350
Niel, C., Sinoquet, C., Dina, C., Rocheleau, G. (2015). A survey about methods dedicated to epistasis detection. Front. Genet. 6. doi: 10.3389/fgene.2015.00285
Nilson, T. (1971). A theoretical analysis of the frequency of gaps in plant stands. Agric. Meteorology 8, 25–38. doi: 10.1016/0002-1571(71)90092-6
Nordborg, M., Tavaré, S. (2002). Linkage disequilibrium: what history has to tell us. Trends Genet. 18, 83–90. doi: 10.1016/S0168-9525(02)02557-X
Oliveira, F. A., Jones, J. W., Pavan, W., Bhakta, M., Vallejos, C. E., Correll, M. J., et al. (2021). Incorporating a dynamic gene-based process module into a crop simulation model. silico Plants 3, diab011. doi: 10.1093/insilicoplants/diab011
Ozturk, A., Caglar, O., Bulut, S. (2006). Growth and yield response of facultative wheat to winter sowing, freezing sowing and spring sowing at different seeding rates. J. Agron. Crop Sci. 192, 10–16. doi: 10.1111/j.1439-037X.2006.00187.x
Palta, J. A., Chen, X., Milroy, S. P., Rebetzke, G. J., Dreccer, M. F., Watt, M. (2011). Large root systems: are they useful in adapting wheat to dry environments? Funct. Plant Biol. 38, 347. doi: 10.1071/FP11031
Parmley, K. A., Higgins, R. H., Ganapathysubramanian, B., Sarkar, S., Singh, A. K. (2019). Machine learning approach for prescriptive plant breeding. Sci. Rep. 9, 1–12. doi: 10.1038/s41598-019-53451-4
Passioura, J. B. (1977). Grain yield, harvest index, and water use of wheat. J. Aust. Institute Agric. Sci. 43, 117–120.
Patidar, A., Yadav, M. C., Kumari, J., Tiwari, S., Chawla, G., Paul, V. (2023). Identification of climate-smart bread wheat germplasm lines with enhanced adaptation to global warming. Plants 12, 2851. doi: 10.3390/plants12152851
Pearl, J., Mackenzie, D. (2018). The book of why: The new science of cause and effect. Science 361, 855–855. doi: 10.1126/science.aau9731
Peng, Z. (2020). A brief overview of gwas: discover genetic variations of diseases and phenotypes. E3S Web Conferences 185, 3014. doi: 10.1051/e3sconf/202018503014
Pfeifer, B., Holzinger, A., Schimek, M. G. (2022). Robust random forest-based all-relevant feature ranks for trustworthy ai. Stud. Health Technol. Inf. 294, 137–138. doi: 10.3233/SHTI220418
Pierce, S. E., Booms, A., Prahl, J., van der Schans, E. J. C., Tyson, T., Coetzee, G. A. (2020). Post-gwas knowledge gap: the how, where, and when. NPJ Parkinson’s Dis. 6, 23. doi: 10.1038/s41531-020-00125-y
Pieruschka, R., Schurr, U. (2019). Plant phenotyping: Past, present, and future. Plant Phenomics 2019, 7507131. doi: 10.34133/2019/7507131
Prabhakar, M., Prasad, Y. G., Rao, M. N. (2012). “Remote sensing of biotic stress in crop plants and its applications for pest management,” in Crop Stress and its Management: Perspectives and Strategies (Springer Netherlands, Dordrecht), 517–545. doi: 10.1007/978-94-007-2220-016
Prudnikova, E., Savin, I., Vindeker, G., Grubina, P., Shishkonakova, E., Sharychev, D. (2019). Influence of soil background on spectral reflectance of winter wheat crop canopy. Remote Sens. 11, 1932. doi: 10.3390/rs11161932
Purcell, S., Neale, B., Todd-Brown, K., Thomas, L., Ferreira, M. A., Bender, D., et al. (2007). Plink: A tool set for whole-genome association and population-based linkage analyses. Am. J. Hum. Genet. 81, 559–575. doi: 10.1086/519795
Qaseem, M. F., Qureshi, R., Muqaddasi, Q. H., Shaheen, H., Kousar, R., Röder, M. S. (2018). Genomewide association mapping in bread wheat subjected to independent and combined high temperature and drought stress. PloS One 13, e0199121. doi: 10.1371/journal.pone.0199121
Qaseem, M. F., Qureshi, R., Shaheen, H., Shafqat, N. (2019). Genome-wide association analyses for yield and yield-related traits in bread wheat (triticum aestivum l.) under pre-anthesis combined heat and drought stress in field conditions. PloS One 14, e0213407. doi: 10.1371/journal.pone.0213407
Qi, J., Chehbouni, A., Huete, A., Kerr, Y., Sorooshian, S. (1994). A modified soil adjusted vegetation index. Remote Sens. Environ. 48, 119–126. doi: 10.1016/0034-4257(94)90134-1
Qin, T., Feng, J., Zhang, X., Li, C., Fan, J., Zhang, C., et al. (2023). Continued decline of global soil moisture content, with obvious soil stratification and regional difference. Sci. Total Environ. 864, 160982. doi: 10.1016/j.scitotenv.2022.160982
Quarrie, S. A., Steed, A., Calestani, C., Semikhodskii, A., Lebreton, C., Chinoy, C., et al. (2005). A high-density genetic map of hexaploid wheat (triticum aestivum l.) from the cross chinese spring × sq1 and its use to compare qtls for grain yield across a range of environments. Theor. Appl. Genet. 110, 865–880. doi: 10.1007/s00122-004-1902-7
Radočaj, D., Šiljeg, A., Marinovič, R., Jurišić, M. (2023). State of major vegetation indices in precision agriculture studies indexed in web of science: A review. Agriculture 13, 707. doi: 10.3390/agriculture13030707
Rahimi, Y., Khahani, B., Jamali, A., Alipour, H., Bihamta, M. R., Ingvarsson, P. K. (2023). Genomewide association study to identify genomic loci associated with early vigor in bread wheat under simulated water deficit complemented with quantitative trait loci meta-analysis. G3 Genes—Genomes—Genetics 13, jkac320. doi: 10.1093/g3journal/jkac320
Rahman, M. M., Robson, A. J. (2016). A novel approach for sugarcane yield prediction using landsat time series imagery: A case study on bundaberg region. Adv. Remote Sens. 05, 93–102. doi: 10.4236/ars.2016.52008
Ramstein, G. P., Jensen, S. E., Buckler, E. S. (2019). Breaking the curse of dimensionality to identify causal variants in breeding 4. Theor. Appl. Genet. 132, 559–567. doi: 10.1007/s00122-018-3267-3
Rasheed, A., Wen, W., Gao, F., Zhai, S., Jin, H., Liu, J., et al. (2016). Development and validation of kasp assays for genes underpinning key economic traits in bread wheat. Theor. Appl. Genet. 129, 1843–1860. doi: 10.1007/s00122-016-2743-x
Reynolds, M., Chapman, S., Crespo-Herrera, L., Molero, G., Mondal, S., Pequeno, D. N., et al. (2020). Breeder friendly phenotyping. Plant Sci. 295, 110396. doi: 10.1016/j.plantsci.2019.110396
Reynolds, M., Dreccer, F., Trethowan, R. (2006). Drought-adaptive traits derived from wheat wild relatives and landraces. J. Exp. Bot. 58, 177–186. doi: 10.1093/jxb/erl250
Reynolds, M., Langridge, P. (2016). Physiological breeding. Curr. Opin. Plant Biol. 31, 162–171. doi: 10.1016/j.pbi.2016.04.005
Rezaei, E. E., Siebert, S., Manderscheid, R., Müller, J., Mahrookashani, A., Ehrenpfordt, B., et al. (2018). Quantifying the response of wheat yields to heat stress: The role of the experimental setup. Field Crops Res. 217, 93–103. doi: 10.1016/j.fcr.2017.12.015
Riahi, K., van Vuuren, D. P., Kriegler, E., Edmonds, J., O’Neill, B. C., Fujimori, S., et al. (2017). The shared socioeconomic pathways and their energy, land use, and greenhouse gas emissions implications: An overview. Global Environ. Change 42, 153–168. doi: 10.1016/j.gloenvcha.2016.05.009
Ribeiro, M. T., Singh, S., Guestrin, C. (2016). ““why should i trust you?” explaining the predictions of any classifier,” in Proceedings of the ACM SIGKDD International Conference on Knowledge Discovery and Data Mining, 13-17-August-2016. (New York, NY, United States), 1135–1144. doi: 10.1145/2939672.2939778
Richards, R. A. (2006). Physiological traits used in the breeding of new cultivars for water-scarce environments. Agric. Water Manage. 80, 197–211. doi: 10.1016/j.agwat.2005.07.013
Rimbert, H., Darrier, B., Navarro, J., Kitt, J., Choulet, F., Leveugle, M., et al. (2018). High throughput snp discovery and genotyping in hexaploid wheat. PloS One 13, e0186329. doi: 10.1371/journal.pone.0186329
Romero, J. C. (2022). Better understanding genomic architecture with the use of applied statistics and explainable artificial intelligence. [Phd thesis] University of Tennessee.
Rouse, J. W., Haas, R. H., Schell, J. A., Deering, D. W. (1974). Monitoring vegetation systems in the great plains with erts. NASA Spec. Publ 351, 309.
Sabatti, C. (2013). Multivariate linear models for gwas (Cambridge, UK: Cambridge University Press), 188–207. doi: 10.1017/CBO9781139226448.010
Safavian, S., Landgrebe, D. (1991). A survey of decision tree classifier methodology. IEEE Trans. Systems Man Cybernetics 21, 660–674. doi: 10.1109/21.97458
Saini, D. K., Chopra, Y., Singh, J., Sandhu, K. S., Kumar, A., Bazzer, S., et al. (2022). Comprehensive evaluation of mapping complex traits in wheat using genome-wide association studies. Mol. Breed. 42, 1. doi: 10.1007/s11032-021-01272-7
Sallam, A., Alqudah, A. M., Dawood, M. F. A., Baenziger, P. S., Börner, A. (2019). Drought stress tolerance in wheat and barley: Advances in physiology, breeding and genetics research. Int. J. Mol. Sci. 20, 3137. doi: 10.3390/ijms20133137
Sanguineti, M., Li, S., Maccaferri, M., Corneti, S., Rotondo, F., Chiari, T., et al. (2007). Genetic dissection of seminal root architecture in elite durum wheat germplasm. Ann. Appl. Biol. 151, 291–305. doi: 10.1111/j.1744-7348.2007.00198.x
Santorsola, M., Lescai, F. (2023). The promise of explainable deep learning for omics data analysis: Adding new discovery tools to ai. New Biotechnol. 77, 1–11. doi: 10.1016/j.nbt.2023.06.002
Saranti, A., Hudec, M., Mináriková, E., Takáč, Z., Großschedl, U., Koch, C., et al. (2022). Actionable explainable ai (axai): A practical example with aggregation functions for adaptive classification and textual explanations for interpretable machine learning. Mach. Learn. Knowledge Extraction 4, 924–953. doi: 10.3390/make4040047
Saranya, T., Deisy, C., Sridevi, S., Anbananthen, K. S. M. (2023). A comparative study of deep learning and internet of things for precision agriculture. Eng. Appl. Artif. Intell. 122, 106034. doi: 10.1016/j.engappai.2023.106034
Satorre, E. H., Slafer, G. A. (2000). Wheat: ecology and physiology of yield determination (Binghamton, NY, USA: Food Products Press).
Schils, R., Olesen, J. E., Kersebaum, K.-C., Rijk, B., Oberforster, M., Kalyada, V., et al. (2018). Cereal yield gaps across europe. Eur. J. Agron. 101, 109–120. doi: 10.1016/j.eja.2018.09.003
Schnake, T., Eberle, O., Lederer, J., Nakajima, S., Schütt, K. T., Müller, K.-R., et al. (2020). Higher-order explanations of graph neural networks via relevant walks. IEEE Trans. Pattern Anal. Mach. Intell. 44, 7581–7596. doi: 10.1109/TPAMI.2021.3115452
Schwalbe, G., Finzel, B. (2023). “A comprehensive taxonomy for explainable artificial intelligence: a systematic survey of surveys on methods and concepts,” in Data Mining and Knowledge Discovery. (Cham, Switzerland: Springer). doi: 10.1007/s10618-022-00867-8
Semagn, K., Babu, R., Hearne, S., Olsen, M. (2014). Single nucleotide polymorphism genotyping using kompetitive allele specific pcr (kasp): overview of the technology and its application in crop improvement. Mol. Breed. 33, 1–14. doi: 10.1007/s11032-013-9917-x
Senapati, N., Halford, N. G., Semenov, M. A. (2021). Vulnerability of european wheat to extreme heat and drought around flowering under future climate. Environ. Res. Lett. 16, 024052. doi: 10.1088/1748-9326/abdcf3
Shaikh, T. A., Rasool, T., Lone, F. R. (2022). Towards leveraging the role of machine learning and artificial intelligence in precision agriculture and smart farming. Comput. Electron. Agric. 198, 107119. doi: 10.1016/j.compag.2022.107119
Shapley, L. S. (1952). A value for n-person games. Princeton, New Jersey: Princeton University Press. doi: 10.7249/P0295
Sharma, D., Durand, A., Legault, M.-A., Perreault, L.-P. L., Lemac¸on, A., Dubé, M.-P., et al. (2020). Deep interpretability for gwas. arXiv [Preprint]. arXiv:2007.01516. doi: 10.48550/arXiv.2007.01516
Shi, X., Ling, H.-Q. (2018). Current advances in genome sequencing of common wheat and its ancestral species. Crop J. 6, 15–21. doi: 10.1016/j.cj.2017.11.001
Shokat, S., Großkinsky, D. K., Singh, S., Liu, F. (2023). The role of genetic diversity and pre-breeding traits to improve drought and heat tolerance of bread wheat at the reproductive stage. Food Energy Secur. 12, e478. doi: 10.1002/fes3.478
Shorten, C., Khoshgoftaar, T. M. (2019). A survey on image data augmentation for deep learning. J. Big Data 6, 60. doi: 10.1186/s40537-019-0197-0
Shrikumar, A., Greenside, P., Kundaje, A. (2017). “Learning important features through propagating activation differences,” in Proceedings of the 34th International Conference on Machine Learning (Sydney, Australia: PMLR), Vol. 70, 4844–4866.
Simonyan, K., Vedaldi, A., Zisserman, A. (2013). “Deep inside convolutional networks: Visualising image classification models and saliency maps,” in 2nd International Conference on Learning Representations, ICLR 2014 - Workshop Track Proceedings.
Sishodia, R. P., Ray, R. L., Singh, S. K. (2020). Applications of remote sensing in precision agriculture: A review. Remote Sens. 12, 3136. doi: 10.3390/rs12193136
Sokol, K., Flach, P. (2019). Desiderata for interpretability: Explaining decision tree predictions with counterfactuals. Proc. AAAI Conf. Artif. Intell. 33, 10035–10036. doi: 10.1609/aaai.v33i01.330110035
Song, P., Wang, J., Guo, X., Yang, W., Zhao, C. (2021). High-throughput phenotyping: Breaking through the bottleneck in future crop breeding. Crop J. 9, 633–645. doi: 10.1016/j.cj.2021.03.015
Song, L., Wang, R., Yang, X., Zhang, A., Liu, D. (2023). Molecular markers and their applications in marker-assisted selection (mas) in bread wheat (triticum aestivum l.). Agriculture 13, 642. doi: 10.3390/agriculture13030642
Sperry, J. S., Adler, F. R., Campbell, G. S., Comstock, J. P. (1998). Limitation of plant water use by rhizosphere and xylem conductance: results from a model. Plant Cell Environ. 21, 347–359. doi: 10.1046/j.1365-3040.1998.00287.x
Sperry, J. S., Love, D. M. (2015). What plant hydraulics can tell us about responses to climate-change droughts. New Phytol. 207, 14–27. doi: 10.1111/nph.13354
Spiertz, J. (1974). Grain growth and distribution of dry matter in the wheat plant as influenced by temperature, light energy and ear size. Netherlands J. Agric. Sci. 22, 207–220. doi: 10.18174/njas.v22i3.17223
Sposito, G. (2013). Green water and global food security. Vadose Zone J. 12, 1–6. doi: 10.2136/vzj2013.02.0041
Srivastava, A. K., Safaei, N., Khaki, S., Lopez, G., Zeng, W., Ewert, F., et al. (2022). Winter wheat yield prediction using convolutional neural networks from environmental and phenological data. Sci. Rep. 12, 1–14. doi: 10.1038/s41598-022-06249-w
Staniak, M., Biecek, P. (2019). Explanations of model predictions with live and breakdown packages. R J. 10, 395. doi: 10.32614/RJ-2018-072
Stein, B. V., Raponi, E., Sadeghi, Z., Bouman, N., Ham, R. C. V., Back, T. (2022). A comparison of global sensitivity analysis methods for explainable ai with an application in genomic prediction. IEEE Access 10, 103364–103381. doi: 10.1109/ACCESS.2022.3210175
Steiner, B., Buerstmayr, M., Michel, S., Schweiger, W., Lemmens, M., Buerstmayr, H. (2017). Breeding strategies and advances in line selection for fusarium head blight resistance in wheat. Trop. Plant Pathol. 42, 165–174. doi: 10.1007/s40858-017-0127-7
Stephens, M., Balding, D. J. (2009). Bayesian statistical methods for genetic association studies. Nat. Rev. Genet. 10, 681–690. doi: 10.1038/nrg2615
Streich, J., Romero, J., Gazolla, J. G. F. M., Kainer, D., Cliff, A., Prates, E. T., et al. (2020). Can exascale computing and explainable artificial intelligence applied to plant biology deliver on the united nations sustainable development goals? Curr. Opin. Biotechnol. 61, 217–225. doi: 10.1016/j.copbio.2020.01.010
Stutsel, B., Johansen, K., Malbéteau, Y. M., McCabe, M. F. (2021). Detecting plant stress using thermal and optical imagery from an unoccupied aerial vehicle. Front. Plant Sci. 12. doi: 10.3389/fpls.2021.734944
Subedi, M., Ghimire, B., Bagwell, J. W., Buck, J. W., Mergoum, M. (2023). Wheat end-use quality: State of art, genetics, genomics-assisted improvement, future challenges, and opportunities. Front. Genet. 13. doi: 10.3389/fgene.2022.1032601
Suliman, S., Alemu, A., Abdelmula, A. A., Badawi, G. H., Al-Abdallat, A., Tadesse, W. (2021). Genome-wide association analysis uncovers stable qtls for yield and quality traits of spring bread wheat (triticum aestivum) across contrasting environments. Plant Gene 25, 100269. doi: 10.1016/j.plgene.2020.100269
Sun, D., Cen, H., Weng, H., Wan, L., Abdalla, A., El-Manawy, A. I., et al. (2019). Using hyperspectral analysis as a potential high throughput phenotyping tool in gwas for protein content of rice quality. Plant Methods 15, 54. doi: 10.1186/s13007-019-0432-x
Sun, C., Dong, Z., Zhao, L., Ren, Y., Zhang, N., Chen, F. (2020). The wheat 660k snp array demonstrates great potential for marker-assisted selection in polyploid wheat. Plant Biotechnol. J. 18, 1354–1360. doi: 10.1111/pbi.13361
Sun, H., Wang, B., Wu, Y., Yang, H. (2023). Deep learning method based on spectral characteristic rein-forcement for the extraction of winter wheat planting area in complex agricultural landscapes. Remote Sens. 15, 1301. doi: 10.3390/rs15051301
Syvänen, A.-C. (2005). Toward genome-wide snp genotyping. Nat. Genet. 37, S5–S10. doi: 10.1038/ng1558
Szymczak, S., Biernacka, J. M., Cordell, H. J., González-Recio, O., König, I. R., Zhang, H., et al. (2009). Machine learning in genome-wide association studies. Genet. Epidemiol. 33, 51–57. doi: 10.1002/gepi.20473
Tam, V., Patel, N., Turcotte, M., Bossé, Y., Paré, G., Meyre, D. (2019). Benefits and limitations of genome-wide association studies. Nat. Rev. Genet. 20, 467–484. doi: 10.1038/s41576-019-0127-1
Tang, Y., Liu, X., Wang, J., Li, M., Wang, Q., Tian, F., et al. (2016). Gapit version 2: An enhanced integrated tool for genomic association and prediction. Plant Genome 9. doi: 10.3835/plantgenome2015.11.0120
Tang, Z., Sun, Y., Wan, G., Zhang, K., Shi, H., Zhao, Y., et al. (2022). Winter wheat lodging area extraction using deep learning with gaofen-2 satellite imagery. Remote Sens. 14, 4887. doi: 10.3390/rs14194887
Tang, Z., Wang, M., Schirrmann, M., Dammer, K.-H., Li, X., Brueggeman, R., et al. (2023). Affordable high throughput field detection of wheat stripe rust using deep learning with semi-automated image labeling. Comput. Electron. Agric. 207, 107709. doi: 10.1016/j.compag.2023.107709
Tardieu, F., Simonneau, T. (1998). Variability among species of stomatal control under fluctuating soil water status and evaporative demand: modelling isohydric and anisohydric behaviours. J. Exp. Bot. 49, 419–432. doi: 10.1093/jxb/49.Special_Issue.419
Tattaris, M., Reynolds, M. P., Chapman, S. C. (2016). A direct comparison of remote sensing approaches for high-throughput phenotyping in plant breeding. Front. Plant Sci. 7. doi: 10.3389/fpls.2016.01131
Technow, F., Messina, C. D., Totir, L. R., Cooper, M. (2015). Integrating crop growth models with whole genome prediction through approximate bayesian computation. PloS One 10, e0130855. doi: 10.1371/journal.pone.0130855
Temenos, A., Tzortzis, I. N., Kaselimi, M., Rallis, I., Doulamis, A., Doulamis, N. (2022). Novel insights in spatial epidemiology utilizing explainable ai (xai) and remote sensing. Remote Sens. 14, 3074. doi: 10.3390/rs14133074
Tenaillon, M. I., Sawkins, M. C., Long, A. D., Gaut, R. L., Doebley, J. F., Gaut, B. S. (2001). Patterns of dna sequence polymorphism along chromosome 1 of maize (¡i¿zea mays¡/i¿ ssp. ¡i¿mays¡/i¿ l.). Proc. Natl. Acad. Sci. 98, 9161–9166. doi: 10.1073/pnas.151244298
Thenkabail, P. S., Lyon, J. G., Huete, A. (2018). Biophysical and biochemical characterization and plant species studies (Boca Raton, Florida, USA: CRC Press). doi: 10.1201/9780429431180
Thenkabail, P. S., Smith, R. B., De Pauw, E. (2002). Evaluation of narrowband and broadband vegetation indices for determining optimal hyperspectral wavebands for agricultural crop characterization. Photogrammetric Eng. Remote Sens. 68, 607–622.
Thoday-Kennedy, E., Good, N., Kant, S. (2022). “Basics of sensor-based phenotyping in wheat,” in Accelerated Breeding of Cereal Crops (Springer US, New York, NY), 305–331. doi: 10.1007/978-1-0716-1526-316
Thornsberry, J. M., Goodman, M. M., Doebley, J., Kresovich, S., Nielsen, D., Buckler, E. S. (2001). Dwarf8 polymorphisms associate with variation in flowering time. Nat. Genet. 28, 286–289. doi: 10.1038/90135
Tilling, A. K, O’Leary, G. J., Ferwerda, J. G., Jones, S. D., Fitzgerald, G. J., Rodriguez, D., et al. (2007). Remote sensing of nitrogen and water stress in wheat. Field Crops Res. 104, 77–85. doi: 10.1016/j.fcr.2007.03.023
Trethowan, R. M., Mujeeb-Kazi, A. (2008). Novel germplasm resources for improving environmental stress tolerance of hexaploid wheat. Crop Sci. 48, 1255–1265. doi: 10.2135/cropsci2007.08.0477
Trnka, M., Olesen, J. E., Kersebaum, K. C., Skjelvåg, A. O., Eitzinger, J., Seguin, B., et al. (2011). Agroclimatic conditions in europe under climate change. Global Change Biol. 17, 2298–2318. doi: 10.1111/j.1365-2486.2011.02396.x
Turner, N. C., Blum, A., Cakir, M., Steduto, P., Tuberosa, R., Young, N. (2014). Strategies to increase the yield and yield stability of crops under drought – are we making progress? Funct. Plant Biol. 41, 1199. doi: 10.1071/FP14057
Uffelmann, E., Huang, Q. Q., Munung, N. S., de Vries, J., Okada, Y., Martin, A. R., et al. (2021). Genomewide association studies. Nat. Rev. Methods Primers 1, 59. doi: 10.1038/s43586-021-00056-9
Vadez, V. (2014). Root hydraulics: The forgotten side of roots in drought adaptation. Field Crops Res. 165, 15–24. doi: 10.1016/j.fcr.2014.03.017
Vadez, V., Kholova, J., Zaman-Allah, M., Belko, N. (2013). Water: the most important ‘molecular’ component of water stress tolerance research. Funct. Plant Biol. 40, 1310. doi: 10.1071/FP13149
Vadez, V., Krishnamurthy, L., Kashiwagi, J., Kholova, J., Devi, J. M., Sharma, K. K., et al. (2007). Exploiting the functionality of root systems for dry, saline, and nutrient deficient environments in a changing climate. J. SAT Agric. Res. 4, 1–61.
Valkoun, J. J. (2001). Wheat pre-breeding using wild progenitors. Euphytica 119, 17–23. doi: 10.1023/A:1017562909881
van der Velde, M., Baruth, B., Bussay, A., Ceglar, A., Condado, S. G., Karetsos, S., et al. (2018). In-season performance of european union wheat forecasts during extreme impacts. Sci. Rep. 8, 15420. doi: 10.1038/s41598-018-33688-1
van Ginkel, M., Calhoun, D., Gebeyehu, G., Miranda, A., Tian-you, C., Lara, R. P., et al. (1998). Plant traits related to yield of wheat in early, late, or continuous drought conditions. Euphytica 100, 109–121. doi: 10.1023/A:1018364208370
Verde, N., Mallinis, G., Tsakiri-Strati, M., Georgiadis, C., Patias, P. (2018). Assessment of radiometric resolution impact on remote sensing data classification accuracy. Remote Sens. 10, 1267. doi: 10.3390/rs10081267
Virnodkar, S. S., Pachghare, V. K., Patil, V. C., Jha, S. K. (2020). Remote sensing and machine learning for crop water stress determination in various crops: a critical review. Precis. Agric. 21, 1121–1155. doi: 10.1007/s11119-020-09711-9
Volpato, L., Pinto, F., González-Pérez, L., Thompson, I. G., Borém, A., Reynolds, M., et al. (2021). High throughput field phenotyping for plant height using uav-based rgb imagery in wheat breeding lines: Feasibility and validation. Front. Plant Sci. 12. doi: 10.3389/fpls.2021.591587
Vong, C. N., Conway, L. S., Feng, A., Zhou, J., Kitchen, N. R., Sudduth, K. A. (2022). Corn emergence uniformity estimation and mapping using uav imagery and deep learning. Comput. Electron. Agric. 198, 107008. doi: 10.1016/j.compag.2022.107008
Vukasovic, S., Alahmad, S., Christopher, J., Snowdon, R. J., Stahl, A., Hickey, L. T. (2022). Dissecting the genetics of early vigour to design drought-adapted wheat. Front. Plant Sci. 12. doi: 10.3389/fpls.2021.754439
Walter, A., Liebisch, F., Hund, A. (2015). Plant phenotyping: from bean weighing to image analysis. Plant Methods 11, 14. doi: 10.1186/s13007-015-0056-8
Wang, S., Wong, D., Forrest, K., Allen, A., Chao, S., Huang, B. E., et al. (2014). Characterization of polyploid wheat genomic diversity using a high-density 90 000 single nucleotide polymorphism array. Plant Biotechnol. J. 12, 787. doi: 10.1111/pbi.12183
Wang, F., Yang, M., Ma, L., Zhang, T., Qin, W., Li, W., et al. (2022). Estimation of above-ground biomass of winter wheat based on consumer-grade multi-spectral uav. Remote Sens. 14, 1251. doi: 10.3390/rs14051251
Wang, L., Zhou, X., Zhu, X., Dong, Z., Guo, W. (2016). Estimation of biomass in wheat using random forest regression algorithm and remote sensing data. Crop J. 4, 212–219. doi: 10.1016/j.cj.2016.01.008
Warrington, N. M., Tilling, K., Howe, L. D., Paternoster, L., Pennell, C. E., Wu, Y. Y., et al. (2014). Robustness of the linear mixed effects model to error distribution assumptions and the consequences for genome-wide association studies. Stat. Appl. Genet. Mol. Biol. 13, 567–587. doi: 10.1515/sagmb-2013-0066
White, J. W., Andrade-Sanchez, P., Gore, M. A., Bronson, K. F., Coffelt, T. A., Conley, M. M., et al. (2012). Field-based phenomics for plant genetics research. Field Crops Res. 133, 101–112. doi: 10.1016/j.fcr.2012.04.003
Wood, S. N. (2004). Stable and efficient multiple smoothing parameter estimation for generalized additive models. J. Am. Stat. Assoc. 99, 673–686. doi: 10.1198/016214504000000980
Wray, N. R., Yang, J., Hayes, B. J., Price, A. L., Goddard, M. E., Visscher, P. M. (2013). Pitfalls of predicting complex traits from snps. Nat. Rev. Genet. 14, 507–515. doi: 10.1038/nrg3457
Wu, L., Chang, Y., Wang, L., Wang, S., Wu, J. (2021). Genome-wide association analysis of drought resistance based on seed germination vigor and germination rate at the bud stage in common bean. Agron. J. 113, 2980–2990. doi: 10.1002/agj2.20683
Wu, C., Niu, Z., Tang, Q., Huang, W. (2009). Predicting vegetation water content in wheat using normalized difference water indices derived from ground measurements. J. Plant Res. 122, 317–326. doi: 10.1007/s10265-009-0215-y
Xiong, P., Schnake, T., Montavon, G., Müller, K.-R., Nakajima, S. (2022). Efficient computation of higher-order subgraph attribution via message passing. Proc. Mach. Learn. Res. 162, 24478–24495.
Xu, J., Lowe, C., Hernandez-Leon, S. G., Dreisigacker, S., Reynolds, M. P., Valenzuela-Soto, E. M., et al. (2022). The effects of brief heat during early booting on reproductive, developmental, and chlorophyll physiological performance in common wheat (triticum aestivum l.). Front. Plant Sci. 13. doi: 10.3389/fpls.2022.886541
Xue, J., Su, B. (2017). Significant remote sensing vegetation indices: A review of developments and applications. J. Sensors 2017, 1–17. doi: 10.1155/2017/1353691
Yadav, H. (2017). Effects of rain shelter or simulated rain during grain filling and maturation on subsequent wheat grain quality in the uk. J. Agric. Sci. 155, 300–316. doi: 10.1017/S0021859616000411
Yang, R. C. (2010). Towards understanding and use of mixed-model analysis of agricultural experiments. Can. J. Plant Sci. 90, 605–627. doi: 10.4141/CJPS10049
Yang, W., Feng, H., Zhang, X., Zhang, J., Doonan, J. H., Batchelor, W. D., et al. (2020). Crop phenomics and high-throughput phenotyping: Past decades, current challenges, and future perspectives. Mol. Plant 13, 187–214. doi: 10.1016/j.molp.2020.01.008
Yang, Z., Rao, M., Elliott, N., Kindler, S., Popham, T. (2009). Differentiating stress induced by greenbugs and Russian wheat aphids in wheat using remote sensing. Comput. Electron. Agric. 67, 64–70. doi: 10.1016/j.compag.2009.03.003
Yang, X., Song, Z., King, I., Xu, Z. (2021). A survey on deep semi-supervised learning. IEEE Trans. Knowledge Data Eng. 35, 8934–8954. doi: 10.1109/TKDE.2022.3220219
Yazdani, A., Yazdani, A., Mendez-Giraldez, R., Samiei, A., Kosorok, M. R., Schaid, D. J. (2022). From classical mendelian randomization to causal networks for systematic integration of multi-omics. Front. Genet. 13. doi: 10.3389/fgene.2022.990486
Yoosefzadeh-Najafabadi, M., Eskandari, M., Belzile, F., Torkamaneh, D. (2022). Genome-wide association study statistical models: A review. Methods Mol. Biol. 2481, 43–62. doi: 10.1007/978-1-0716-2237-74/COVER
Yu, H., Zhang, Q., Sun, P., Song, C. (2018). Impact of droughts on winter wheat yield in different growth stages during 2001–2016 in eastern China. Int. J. Disaster Risk Sci. 9, 376–391. doi: 10.1007/s13753-018-0187-4
Yuan, L., Bao, Z., Zhang, H., Zhang, Y., Liang, X. (2017). Habitat monitoring to evaluate crop disease and pest distributions based on multi-source satellite remote sensing imagery. Optik 145, 66–73. doi: 10.1016/j.ijleo.2017.06.071
Yuan, W., Li, J., Bhatta, M., Shi, Y., Baenziger, P., Ge, Y. (2018). Wheat height estimation using lidar in comparison to ultrasonic sensor and uas. Sensors 18, 3731. doi: 10.3390/s18113731
Zhang, Y.-M., Jia, Z., Dunwell, J. M. (2019). [dataset] editorial: The applications of new multilocus gwas methodologies in the genetic dissection of complex traits. Front. Plant Sci. 10. doi: 10.3389/fpls.2019.00100
Zhang, J., Naik, H. S., Assefa, T., Sarkar, S., Reddy, R. V., Singh, A., et al. (2017). Computer vision and machine learning for robust phenotyping in genome-wide studies. Sci. Rep. 7, 1–11. doi: 10.1038/srep44048
Zhang, Q., Zhang, Q., Jensen, J. (2022). Association studies and genomic prediction for genetic improvements in agriculture. Front. Plant Sci. 13. doi: 10.3389/fpls.2022.904230
Zhao, Y., Potgieter, A. B., Zhang, M., Wu, B., Hammer, G. L. (2020). Predicting wheat yield at the field scale by combining high-resolution sentinel-2 satellite imagery and crop modelling. Remote Sens. 12, 1024. doi: 10.3390/rs12061024
Zhao, J., Sun, L., Gao, H., Hu, M., Mu, L., Cheng, X., et al. (2023). Genome-wide association study of yield-related traits in common wheat (triticum aestivum l.) under normal and drought treatment conditions. Front. Plant Sci. 13. doi: 10.3389/fpls.2022.1098560
Zhao, Y., Zeng, J., Fernando, R., Reif, J. C. (2013). Genomic prediction of hybrid wheat performance. Crop Sci. 53, 802–810. doi: 10.2135/cropsci2012.08.0463
Zhou, X., Kono, Y., Win, A., Matsui, T., Tanaka, T. S. T. (2021a). Predicting within-field variability in grain yield and protein content of winter wheat using uav-based multispectral imagery and machine learning approaches. Plant Production Sci. 24, 137–151. doi: 10.1080/1343943X.2020.1819165
Zhou, Z., Majeed, Y., Naranjo, G. D. (2021b). [dataset] assessment for crop water stress with infrared thermal imagery in precision agriculture: A review and future prospects for deep learning applications. Comput. Electron. Agric. 182, 106019. doi: 10.1016/j.compag.2021.106019
Zhou, X., Stephens, M. (2012). Genome-wide efficient mixed-model analysis for association studies. Nat. Genet. 44, 821–824. doi: 10.1038/ng.2310
Zhou, L., Xiao, Q., Taha, M. F., Xu, C., Zhang, C. (2023). Phenotypic analysis of diseased plant leaves using supervised and weakly supervised deep learning. Plant Phenomics 5, 0022. doi: 10.34133/plantphenomics.0022
Zhou, X., Zhang, J., Chen, D., Huang, Y., Kong, W., Yuan, L., et al. (2020). Assessment of leaf chlorophyll content models for winter wheat using landsat-8 multispectral remote sensing data. Remote Sens. 12, 2574. doi: 10.3390/rs12162574
Zhu, C., Gore, M., Buckler, E. S., Yu, J. (2008). Status and prospects of association mapping in plants. Plant Genome 1. doi: 10.3835/plantgenome2008.02.0089
Zhu, W., Sun, Z., Huang, Y., Lai, J., Li, J., Zhang, J., et al. (2019). Improving field-scale wheat lai retrieval based on uav remote-sensing observations and optimized vi-luts. Remote Sens. 11, 2456. doi: 10.3390/rs11202456
Zhu, D., Wang, C., Pang, B., Shan, F., Wu, Q., Zhao, C. (2012). Identification of wheat cultivars based on the hyperspectral image of single seed. J. Nanoelectronics Optoelectronics 7, 167–172. doi: 10.1166/jno.2012.1243
Keywords: drought tolerance, GWAS, MAS, plant breeding, winter wheat, XAI, UAV remote phenotyping, smart agriculture
Citation: Chang-Brahim I, Koppensteiner LJ, Beltrame L, Bodner G, Saranti A, Salzinger J, Fanta-Jende P, Sulzbachner C, Bruckmüller F, Trognitz F, Samad-Zamini M, Zechner E, Holzinger A and Molin EM (2024) Reviewing the essential roles of remote phenotyping, GWAS and explainable AI in practical marker-assisted selection for drought-tolerant winter wheat breeding. Front. Plant Sci. 15:1319938. doi: 10.3389/fpls.2024.1319938
Received: 11 October 2023; Accepted: 13 March 2024;
Published: 18 April 2024.
Edited by:
Baohua Wang, Nantong University, ChinaReviewed by:
Stephen Welch, Kansas State University, United StatesShawn Carlisle Kefauver, University of Barcelona, Spain
Copyright © 2024 Chang-Brahim, Koppensteiner, Beltrame, Bodner, Saranti, Salzinger, Fanta-Jende, Sulzbachner, Bruckmüller, Trognitz, Samad-Zamini, Zechner, Holzinger and Molin. This is an open-access article distributed under the terms of the Creative Commons Attribution License (CC BY). The use, distribution or reproduction in other forums is permitted, provided the original author(s) and the copyright owner(s) are credited and that the original publication in this journal is cited, in accordance with accepted academic practice. No use, distribution or reproduction is permitted which does not comply with these terms.
*Correspondence: Eva M. Molin, ZXZhLW1hcmlhLm1vbGluQGFpdC5hYy5hdA==
†These authors have contributed equally to this work and share first authorship