- 1 Max Planck Institute for Molecular Plant Physiology, Potsdam-Golm, Brandenburg, Germany
- 2 Bioinformatics, Universität Potsdam, Potsdam-Golm, Germany
The regulation of protein function by modulating the surface charge status via sequence-locally enriched phosphorylation sites (P-sites) in so called phosphorylation “hotspots” has gained increased attention in recent years. We set out to identify P-hotspots in the model plant Arabidopsis thaliana. We analyzed the spacing of experimentally detected P-sites within peptide-covered regions along Arabidopsis protein sequences as available from the PhosPhAt database. Confirming earlier reports (Schweiger and Linial, 2010), we found that, indeed, P-sites tend to cluster and that distributions between serine and threonine P-sites to their respected closest next P-site differ significantly from those for tyrosine P-sites. The ability to predict P-hotspots by applying available computational P-site prediction programs that focus on identifying single P-sites was observed to be severely compromised by the inevitable interference of nearby P-sites. We devised a new approach, named HotSPotter, for the prediction of phosphorylation hotspots. HotSPotter is based primarily on local amino acid compositional preferences rather than sequence position-specific motifs and uses support vector machines as the underlying classification engine. HotSPotter correctly identified experimentally determined phosphorylation hotspots in A. thaliana with high accuracy. Applied to the Arabidopsis proteome, HotSPotter-predicted 13,677 candidate P-hotspots in 9,599 proteins corresponding to 7,847 unique genes. Hotspot containing proteins are involved predominantly in signaling processes confirming the surmised modulating role of hotspots in signaling and interaction events. Our study provides new bioinformatics means to identify phosphorylation hotspots and lays the basis for further investigating novel candidate P-hotspots. All phosphorylation hotspot annotations and predictions have been made available as part of the PhosPhAt database at http://phosphat.mpimp-golm.mpg.de.
Introduction
Protein phosphorylation is one of the most significant and best characterized posttranslational modifications involved a wide range of molecular regulatory and signaling mechanisms (Johnson, 2009). Conventionally, functionally relevant phosphorylation sites (P-sites) have been regarded as precisely defined sites within proteins. Their exact location along the protein sequence, and thus in the three-dimensional structure, was thought to be a key determinant for their exerted function, for example, by inducing conformational changes of the associated protein allowing for allosteric regulation (Barford et al., 1991). In agreement with this view, functionally relevant P-sites have been observed to be conserved in evolution such that their position in the sequence and structure of proteins is maintained across different bacteria (Macek et al., 2007), plants (Nakagami et al., 2010), vertebrates (Malik et al., 2008), and eukaryotes in general (Boekhorst et al., 2008). This conclusion has been challenged by other studies that reported that P-sites are no more conserved than expected by chance (e.g., in a comparison of mouse and human; Jimenez et al., 2007). In a recent survey of amino acid changing polymorphisms across many Arabidopsis thaliana accessions, it was concluded that serine, threonine, and tyrosine sites associated with phosphorylation events were indeed statistically more conserved than their non-phosphorylated counterparts. However, statistical significance was established at p = 0.03 only (Joshi et al., 2012). Given that several thousands of positions were included in the test, this weak significance is rather surprising.
An alternative view on the role of P-site localization and the consequences for protein function emerged when it was observed that, rather than individual sites with determined position, clusters of P-sites appear to be functionally significant, in which the exact location of individual P-sites belonging to the cluster seems less important (Moses et al., 2007). Instead, the combined effect of the individual sites on the local electrostatic surface potential appears to be relevant. For example, in an analysis of P-sites in cell-cycle dependent kinases (Cdk1) across several ascomycete species, it became evident that instead of the individual sites, a rather unspecific cluster of P-sites with evolutionarily rapidly changing positions of individual P-sites appeared to be conserved and thus functionally relevant for modifying protein–protein interactions (Holt et al., 2009). Similarly, the function of the protein Ste5, involved in the regulation of cell cycle in yeast in response to pheromones, was observed to be regulated via eight poorly conserved serine and threonine P-sites (Strickfaden et al., 2007). The regulatory switch was identified as a disruption of Ste5 binding to the inner leaflet of the cellular membrane caused by the negative charges transferred by phosphorylation. It has furthermore been argued that, if the exact location of a particular P-site is not conserved, the mechanism of phosphorylation-mediated signaling can still be preserved if alternative sites are phosphorylated by orthologous kinases in the respective organism (Tan et al., 2010). Consequently, effects mediated via the electrostatic surface potential alone rather than position-specific changes of protein structure were increasingly discussed as possible modes of action of phosphorylation-mediated regulation (Serber and Ferrell, 2007). For surface electrostatics to take effect, all that is required is a local accumulation of charges reflected by a tendency for P-sites to cluster along the sequence and structure of proteins. The precise location of P-sites would then be secondary in this respect. Indeed, such clusterings of P-sites, so called P-hotspots, have been observed for individual proteins, e.g., CDK targets (Moses et al., 2007) and on a broader proteomic scale in the plant A. thaliana (Riano-Pachon et al., 2010), as well as across several species (Schweiger and Linial, 2010). P-sites, especially phosphorylated serines (pS) and threonines (pT), and to a lesser extent tyrosines (pY), were shown to cluster more than expected by chance, which included accounting for the background distribution of serines, threonines, and tyrosines as well as taking into account the reported tendency of P-sites to preferentially occur in disordered regions (Dunker et al., 2002; Iakoucheva et al., 2004; Schweiger and Linial, 2010).
For the computational prediction of individual P-sites from protein sequence information alone, several software programs have been developed and are broadly available (for review, see Trost and Kusalik, 2011). Predicting P-hotspots in proteins then appears to simply be possible by choosing a preferred P-site prediction tool and then identifying clusterings of predicted sites along the sequence. However, here we show that this approach is hampered by the dense clustering of P-sites in hotspot themselves. When applied to the Arabidopsis genome, we demonstrate here that the Arabidopsis-trained P-site prediction method integrated in the PhosPhAt database cannot faithfully reproduce the actually observed distance relationships between consecutive experimentally determined P-sites. As the distance between consecutive P-sites is very short, 54% of all pS and pT sites are within four amino acids from another P-site (Schweiger and Linial, 2010), extracting a sequence motif for single and isolated sites is compromised by neighboring P-sites falling into the sequence window used in the prediction leading to poorly defined compositional profiles in the flanking sequences. For example, assuming that there may exist P-site tandems (pairs of sequence consecutive P-sites), for the N-terminal P-site, a C-terminal neighboring P-site (i.e., serines, threonines, or tyrosines) will be extracted, but for the second, C-terminal P-site an N-terminal position will appear enriched. Taken together, no clear sequence profile will be discernible.
Here, we analyzed the largest available set of experimentally detected P-sites in A. thaliana from the perspective of P-site clustering along the sequence, thereby confirming findings reported in earlier studies using different datasets (Schweiger and Linial, 2010). We furthermore investigated the spacing characteristics considering both the limited experimental coverage of protein sequence regions as well as the underlying spacing tendencies of serine, threonine, and tyrosine amino acid residues themselves.
Motivated by the shortcomings of predictors of individual P-sites to faithfully identify P-hotspots, we present a novel approach to the prediction of P-hotspots based on the amino acid sequence of proteins alone. As, because of the variable spacing between consecutive P-sites, no characteristic P-hotspot sequence motif was detectable that would permit the use of associated prediction approaches such as Hidden Markov Models (HMMs), we used the amino acid composition of P-hotspots as the primary feature extracted from protein sequences combined subsequently with Support Vector Machine (SVM) based classification methods and show that P-hotspots can be predicted with reasonable accuracy. We applied our developed P-hotspot prediction method, named HotSPotter, to the Arabidopsis proteome and identified candidate hotspots in several thousands of proteins. Combined with their functional characterization via annotation information, the potentially high abundance of P-hotspots sheds further light on their significance in regulatory processes and offers new avenues for the experimental study of P-hotspot phenomena.
All P-hotspot annotations, both experimentally annotated and computationally predicted sites, have been made available as part of the PhosPhAt database at http://phosphat.mpimp-golm.mpg.de (Heazlewood et al., 2008; Durek et al., 2010).
Materials and Methods
Mass-Spectral Peptides/Phosphopeptides and Experimentally Determined P-sites/Arabidopsis Proteome
Experimentally determined P-sites were obtained from the PhosPhAt database (Heazlewood et al., 2008; Durek et al., 2010) with data as of March 2010. At the time of this study, PhosPhAt contained information about 61,445 peptide spectra obtained from Arabidopsis samples that map to 10,507 Arabidopsis proteins. Filtering the dataset for unambiguously assigned P-sites in proteolytic peptides resulted in a dataset of 7,214 phosphopeptides mapping to 4,326 Arabidopsis proteins with protein sequences obtained from TAIR, version 9 (Huala et al., 2001). This dataset included 5,238 serine (73%), 1,433 threonine (20%), and 543 tyrosine (7%) P-sites, respectively. For 2,940 of the total of 7,214 experimentally verified P-sites, no other P-site was reported in the same protein. Thus, they have been excluded from further analysis leaving 4,274 P-sites for further studies on pairwise P-site distances.
Inter-P-site Distances
Sequence distances between detected P-sites, di,j = Pi − Pj, with Pi and Pj corresponding to the respective sequence positions of two neighboring P-sites i and j were included in the analysis of their statistical distribution only if both sites mapped to a protein sequence segment that was continuously covered by experimentally measured peptides. Thus, potentially wrong distances due to incomplete peptide coverage were avoided as best as possible. For obtaining the statistics of inter-P-site distances, the respective closest P-site (the closest of either the next P-site in N-terminal or C-terminal sequence direction) was chosen, designated as dN.
Randomizations of P-site Distributions
The actual distance distribution between neighboring P-sites was compared to two sets of randomized P-site distributions. (a) P-flag-randomization: phosphorylation flags, i.e., detected phosphorylations of serine (S), threonine (T), or tyrosine (Y), were randomly reassigned to other S’s, T’s, or Y’s along the protein sequence or region with continuous peptide coverage. (b) Sequence-randomization: the entire protein sequence (or region with continuous peptide coverage) was randomized by randomly shuffling all amino acid residue positions. Method (a) tests whether the actually observed distances are merely a consequence of the underlying S, T, Y distributions, and their positional preferences. Method (b) destroys also these potential biases.
Predicted P-sites in the Arabidopsis Proteome
Computational predictions of individual P-sites were derived from the PhosPhAt P-site predictor (Durek et al., 2010). Only predictions resulting in a score >1 corresponding to high-confidence predictions were included in the analysis yielding 92,872 predicted P-sites associated with S, T, or Y in the Arabidopsis proteome. Thus, approximately 4% of all STY sites in the Arabidopsis proteome are predicted to be phosphorylated. Randomizations of predicted P-sites were created as described above for experimental sites.
P-hotspots in the Arabidopsis Proteome
Definition of P-hotspots
Sequence regions with occurrences of a least four consecutive experimentally detected P-sites along a protein sequence with a distance between neighboring sites of no more than 10 amino acids were considered as P-hotspots. The minimal hotspot length was set as 17 amino acid residues in order to provide a large enough sequence from which to derive a somewhat meaningful composition vector. Thus, sequence windows were padded by additional amino acid residues from the actual protein sequence beyond the terminal pSTY positions to result in hotspots of length 17, and minimally three residues on either side regardless of hotspot length.
Prediction of P-hotspots Using SVMs
Feature vectors, positive/negative training vectors
The prediction of P-hotspots was based on the amino acid composition of P-hotspots expressed as 420-dimensional vectors of the counts of occurrences of all 20 amino acids (A, …, Y) and all 400 possible amino acid dimers (AA, AC, …, YW, YY), both normalized by the considered P-hotspot sequence window length. N-to-C-terminal sequence directionality was considered in the amino acid pair counts such that amino acids were paired up only with their respective C-terminal amino acid residues in the same hotspot sequence resulting in asymmetric pair counts; i.e., some residual sequence order information is included in the prediction method.
The 79 P-hotspot sequences detected using experimentally identified P-sites ranging in length between 17 and 71 amino acid residues were subdivided into all possible segments of length 17 at a one amino acid increment resulting in 365 different segments and associated composition vectors representing the positive examples for the classification task. Thus, the considered sequence windows overlapped. For example, two neighboring sequence windows are identical in at least 16 positions. To account for this redundancy, in the training and testing the available P-hotspot sets were subdivided based on the P-hotspots. Identified P-hotspot sequences showed low mutual sequence identity with a median (maximal) pairwise sequence identity of 21.4% (50%) and no matching sequence segment longer than seven amino acid residues between any two hotspot sequences as determined from running an all-against-all sequence alignment using the program align0 (Myers and Miller, 1988). Lastly, it was checked that indeed all sequences and associated feature vectors were non-redundant.
Proteolytic peptide-covered Arabidopsis protein sequences that are not identified as P-hotspots served as negative examples. As in the case for P-hotspots (positive examples), the corresponding consecutive sequence regions were subdivided into windows of length 17 and the 420-dimensional composition vectors were determined. Only those windows with serine, threonine, and tyrosine frequencies, respectively, as high as or greater than the lower limit determined for P-hotspots were selected as negative examples. This requirement was introduced to safeguard against trivial compositional differences resulting in increased phosphorylation rates as obviously fewer phosphorylatable amino acid residues will correlate with fewer P-sites. Furthermore, the risk of rather detecting unstructured regions as opposed to P-hotspots specifically is minimized. It is important to note that the negative set may contain individual P-sites. However, they were not sufficiently clustered to result in a P-hotspot assignment.
As the maximum negative set is substantially larger than the positive set, which would potentially bias the prediction toward specifically recognizing features associated with the negative rather with the positive set, the negative set was downsized to a ratio of 1:8 positive vs. negative examples by random selection.
SVM-based classification
The SVM-Light software package (http://svmlight.joachims.org/) was used as the classification engine. The radial basis function was used as the kernel. The associated parameters γ (kernel parameter), and the margin parameters C (the tolerance with regard to classification errors) and j (weight to balance errors of positive and negative examples) were identified by an exhaustive grid search implemented as a fivefold cross-validation based on maximal F-scores (see below). Intervals and increments were chosen as follows: C = (2−10, 2−9, 2−8, …, 224), γ = (2−10, 2−9, 2−8, …, 26), and j = (2−2, 2−1, 2−0, …, 24), thus covering large intervals for all parameters. Best performance was achieved for j = 23, C = 20, g = 22. Furthermore, the cost associated with false positive relative to false negative predictions was set to a ratio of 8:1 reflecting the difference in abundance in the training dataset. The performance results of the SVM-based P-hotspot predictions (named “HotSPotter”) were obtained in a fivefold cross-validation such that an average of the performance metric was obtained from five repeats of training/testing set splits of 80/20%. SVMs were trained on the 80%-set and performance parameters obtained from applying them to the 20% hold-out set. Here, the 79 original hotspot sequences were partitioned, and subsequently, the corresponding feature vectors were computed. As the hotspot sequences were largely dissimilar (see above), the performance assessment was effectively protected against overfitting. For the final application of HotSPotter to the Arabidopsis proteome with all protein sequences obtained from TAIR, version 9 (Huala et al., 2001), a final SVM was trained based on all 79 hotspots detected based on experimentally detected P-sites and applied to the Arabidopsis proteome in order to detect additional phosphorylation hotspots. Predictions were considered positive; i.e., a sequence window of length 17 was considered a P-hotspot segment, if the obtained SVM score was greater than initially 0, and subsequently when applied to the entire proteome, >1 to reduce the number of false positive predictions.
Post-prediction filtering and consolidation
Initially, all 12,866,960 possible overlapping 17-mers of the Arabidopsis proteome (33,410 proteins, TAIR-9; Huala et al., 2001) were subjected to the SVM classification. Subsequently, consecutive runs of positive predictions were consolidated into runs and filtered as follows. Every run consisting of consecutive positive predictions was tested to contain at least four S, T, or Y amino acid residues with the largest sequence spacing between them of no more than 10 amino acid residues; i.e., the same criteria as imposed on the experimental hotspots were applied. As prediction windows overlapped, predictions could potentially be conflicting for one and the same sequence position. For example, a 17-mer was predicted with positive score, while the adjacent 17-mer was predicted negative leading to conflicting assignments for 16 residue positions. In this case, the positive predictions took precedence. Furthermore, neighboring runs with fewer than or equal to 17 (window length) residues between their respective start positions were merged into a single run as they overlapped, but were interrupted by negative predictions.
GO Term Enrichment Analysis
Enrichment analysis of gene ontology (GO) terms in proteins observed and predicted to harbor P-hotspots was performed using the as described in Walther et al. (2007) applying a Fisher exact test with subsequent Benjamini–Hochberg correction (Benjamini and Hochberg, 1995) and using detailed GO and GO-slim term annotations as available from TAIR (Huala et al., 2001).
Tests were performed separately for the function, process, and component GO term categories. The entire Arabidopsis proteome minus the set of proteins to be tested and associated GO term annotations served as the respective reference sets.
Prediction of Disordered Regions in Arabiodpsis Proteins
Disordered regions in all 33,410 TAIR-9 Arabidopsis proteins were predicted using the program GlobPlot (Linding et al., 2003). GlobPlot was executed locally using the Globe Pipe script downloaded from http://globplot.embl.de/ and applying the recommended default parameters (webserver settings): SmoothFrame = 10, DOMjoinFrame = 15, DOMpeakFrame = 74, DISjoinFrame = 4, and DISpeakFrame = 5. Of all predicted disordered regions, only the 42,517 disordered regions of length 17 or greater were considered for further analyses to match the minimal length of predicted hotspot regions.
Overlap of P-hotspots and Disordered Regions, Randomization of P-hotspot Positions in the Arabidopsis Proteome
P-hotspots and disordered regions were considered overlapping if at least three consecutive amino acid residues were shared between them. To compare the actual overlap of predicted disordered regions and P-hotspots with random expectations, all 13,677 HotSPotter-predicted P-hotspots (SVM score >1) were reassigned to random positions in the Arabidopsis proteome by randomly choosing for all hotpots an Arabidopsis protein and then positioning the hotspot to a random position along the protein’s sequence and disallowing any hotspot overlaps. This process was repeated 10 times to obtain an average statistic.
Results
Distribution of P-sites in the Arabidopsis Proteome
We first characterized the sequence distances, dN, between P-sites to their respective closest neighboring P-site along the protein sequence as observed in peptide-covered regions in the Arabidopsis proteome. Because the obtained distance distributions for serine and threonine P-sites were found to be very similar, we treated them as a single event. As shown in Figure 1A and confirming earlier findings reported for a larger set of 51,000 P-sites from multiple organisms (Schweiger and Linial, 2010), we found that for phosphorylated serine and threonine residues and their distances to respective closest neighboring P-sites, there is a pronounced peak at distance dN = 2 with an overall decreasing frequency of pair distances with increasing sequence separation. Note that the decreasing frequency with increasing sequence separation is to be expected as the probability that a sequence region is not phosphorylated – qualifying it as a spacing sequence between two neighboring P-sites – also falls. In contrast, the distribution of sequence distances of pYs (Figure 1B) to any other P-site was clearly different (p = 0.0015 in a chi-squared test for homogeneity comparing d(pY, pSTY) and d(pST, pSTY), exhibiting a monotonically decreasing frequency for increasing sequence separation. Compared to randomized protein sequences (Figure 1C), the enrichment of close sequence positions of neighboring P-sites of actual P-sites becomes evident. While for real P-sites, about 10% of all P-neighbor distances are at dN = 1 and close to 50% of pairwise distances are found within dN < 6, the equivalent number is only about 1% for randomized sequences and only about 6% of all distance intervals at dN < 6. Thus, P-sites tend to cluster also when considering MS-peptide-covered sequence regions only.
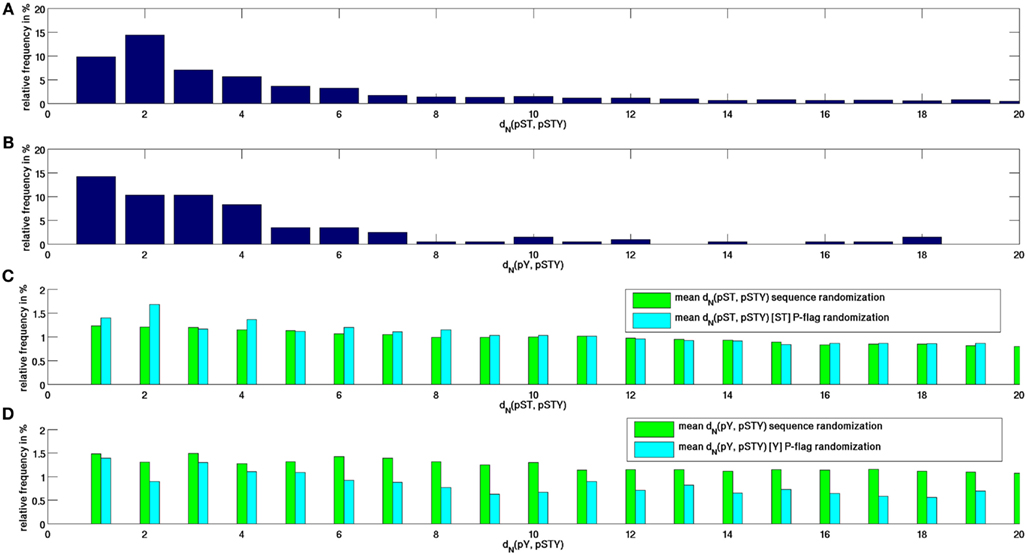
Figure 1. Frequency distribution of sequence distances between neighboring P-sites between (A) any pST and any other site P-site pSTY, (B) any pY and any other P-site. As for the respective neighboring site, no distinction was made as to what amino acid residue type (either S, T, or Y) was found phosphorylated. (C) Equivalent distributions for P-flag-randomized and sequence-randomized protein sequences averaged over 100 repeat runs for nearest pST and pSTY distances. (D) Similary for pY, pSTY distances. In P-flag, phosphorylation signals were randomly redistributed among the existing serines and tyrosines, whereas in sequence-randomized runs, the entire protein sequence was randomized.
Evidently, the distribution of P-sites along protein sequences is strongly influenced by the distribution of phosphorylate-able amino acid residue types themselves. We performed two different types of randomizations – one with randomly reassigning experimentally confirmed P-events to the real und unchanged positions of phosphorylate-able amino acid types, termed P-flag randomization, and another, termed sequence-randomization, in which the whole protein sequence was shuffled, thus destroying also any preferences of pairwise distances of phosphorylate-able amino acid types. Comparing both randomization approaches allows to better assess the influence of the underlying serine and threonine distributions on the observed actual distance distributions. Interestingly, the peak at interval dN = 2 is also evident in the P-flag randomization runs, while the decay in relative frequency is monotonic if the whole protein sequence is randomized. Thus, it can be concluded that the observed preference for the sequence interval dN = 2 for neighboring P-sites appears to be largely a consequence of the distribution of the serines and threonines themselves favoring distance intervals 2.
Characteristics of Actual P-neighbor Distance Distributions is Not Faithfully Reproduced by Predicted P-sites
The PhosPhAt database (Heazlewood et al., 2008; Durek et al., 2010) includes a set of computationally predicted P-sites using a prediction algorithm that was specifically trained using experimentally detected P-sites in the Arabidopsis proteome.
As shown in Figure 2, the frequency distribution between neighboring predicted P-sites differs substantially from the corresponding distribution observed for experimentally detected P-sites (Figure 1). Computational sites result in shallower distributions at close distances (only about 3% at dN = 1 compared to >10% for experimental sites and 12% of all distances at dN < 6 compared to close to 50% for real sites) and they exhibit another peak of locally preferred distances at dN = 4 not observed for experimental sites. The mean distance between respectively closest neighboring P-sites was determined at 94.1 for experimental sites and 63.5 for predicted sites. As there are many more predicted (93,972) than experimental sites (7,214), the lower mean distance between neighboring predicted sites is to be expected. However, in P-flag randomizations, the mean distance increased for experimental sites to 142.7, whereas it increases only marginally to 67.9 for predicted sites even though only about 4% of all STYs in the Arabidopsis proteome are predicted to be phosphorylated such that any clustering of actual prediction sites could be destroyed in P-flag randomizations. Thus, compared to random background, experimental sites exhibit a much greater tendency to cluster than predicted P-sites.
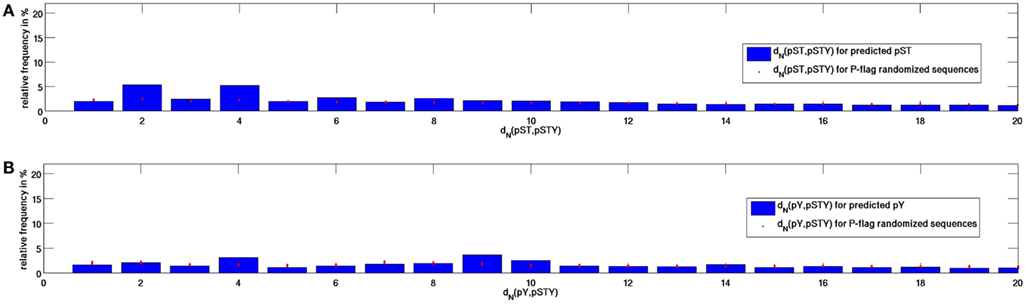
Figure 2. Frequency distribution of distances between closest neighboring predicted P-sites in the Arabidopsis genome. (A) Between any pST and the nearest pSTY, and (B) between any pY and the nearest pSTY. For comparison, results for 100 P-flag randomizations are given by red filled circles. Evidently, the nearest neighbor distance distribution differs from the distribution between experimentally identified sites (Figure 1) with a secondary peak at dN(pST, pSTY) = 4 and a more even distribution of dN(pY, pSTY) for predicted sites.
We conclude that the approach of using computationally predicted P-sites for the purpose of identifying P-hotspots in the Arabidopsis proteome is bound to produce less than optimal results as it does not faithfully predict the characteristics of P-site distance distributions and the tendency to cluster in sequence. Therefore, here we pursued an alternative approach that relies more on compositional preferences of a sequence segment rather than a sequence position-specific amino acid profile as commonly used in computational P-site prediction algorithms.
P-hotspots in the Arabidopsis Proteome
First, we identified P-hotspots based on experimentally detected P-sites in the Arabidopsis proteome. Sequence regions with occurrences of a least four consecutive experimentally detected P-sites along a protein sequence with a distance between neighboring sites of no more than 10 amino acids were considered P-hotspots. The longest such P-hotspot was detected in a serine/arginine-rich protein splicing factor protein (AT2G37340.1) with 12 P-sites along a sequence region comprising 64 out of the total of 290 amino acid residues in that protein. In total, 79 P-hotspots were detected in 75 Arabidopsis proteins based on experimental P-sites (Table 1). As described similarly by Riano-Pachon et al. (2010) using a P-hotspot definition based on comparisons to background distributions, P-hotspot proteins identified in this study were found to be involved primarily in RNA binding and splicing processes (GO term enrichment analysis yielding pFDR = 0.001 for GO function term “RNA binding,” pFDR = 4.2E−13 for GO process term “RNA splicing”), and are located in the “nuclear speck” (pFDR = 1.3E−09), the “plasma membrane” (pFDR = 4.7E−06), and the “splicosome” (pFDR = 0.05).
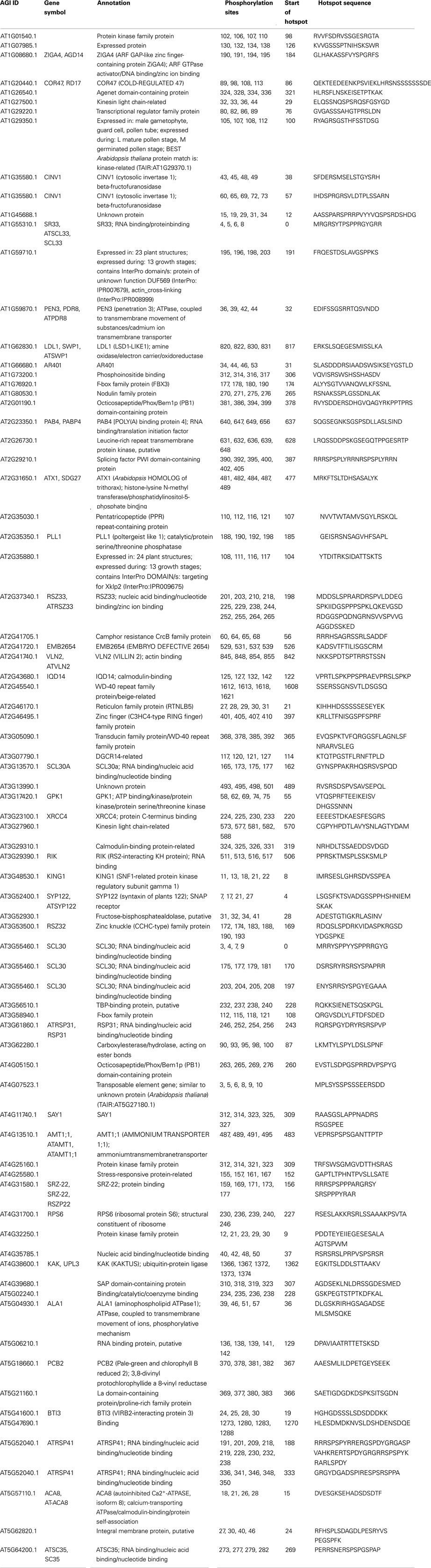
Table 1. Arabidopsis P-hotspot containing proteins detected based on experimentally identified phosphorylation sites.
P-hotspot Predictions
Performance of the SVM-based P-hotspot classification algorithm termed HotSPotter
Using the detected hotspots for training, we developed a SVM based computational algorithm, termed HotSPotter, to predict additional hotspots in the Arabidopsis proteome as outlined in the Section “Materials and Methods.” Instead of sequence motifs that are conventionally used to predict individual P-sites, our method relies on the amino acid composition of P-hotspot regions. In a fivefold cross-validation setting, the following performance parameters were obtained (Table 2). When tuning the performance, we deliberately tolerated relatively low true positive rates (63% achieved), in exchange for a low false positive rate (2%). In training, the proportion of positive to negative examples was adjusted in favor of positive examples, and not the dominating negative signal (see Materials and Methods). When applied to the entire Arabidopsis genome, low false positive rates were deemed critical as the number of true negatives is much higher than in training.
P-hotspot predictions in the Arabidopsis genome using HotSPotter
We applied the trained SVM classification algorithm to the prediction of phosphorylation hotspots in the Arabidopsis genome. Applying the original SVM cutoff score value, SSVM, for positive predictions of SSVM > 0 resulted in positive predictions for 7.3% of all tested sequence windows, length 17, and correspondingly, 23,525 (70%) of all Arabidopsis proteins. Despite the low false positive rate obtained in cross-validation of only 2% during training, this number seems very high. Therefore, we applied a more stringent cutoff of SSVM > 1, reducing the number of predicted hotspot proteins to 9,599 (28%) of all Arabidopsis proteins including 16 mitochondrial and 12 chromosomal proteins (Table 3).
Of the 75 proteins observed to contain P-hotspots based on experimental data, 57 (76%) were contained in the predicted set of hotspot containing proteins based on positive predictions at SVM score, SSVM > 1.
Biological role of hotspot proteins/GO term enrichment/depletion analysis
We performed a GO term enrichment analysis to characterize the proteins predicted to harbor P-hotspots with regard to their association to particular biological processes, functions, and components. Using GO-slim term annotations and probing both for enrichment as well as depletion of annotation terms in the set relative to all other Arabidopsis proteins, we found that hotspot proteins appear to be involved in DNA/RNA metabolism processes, as well as signal transduction (e.g., kinase and transcription factor functions) and developmental processes. With regard to location, predicted hotspot proteins appear to be targeted toward the chloroplast, nucleus (consistent with DNA/RNA binding functions), and associated with the plasma membrane. By contrast, as of yet uncharacterized Arabidopsis proteins as well as proteins involved in stress response, proteins performing transporter activities, and those with ribosomal or extracellular and cytosolic localization appear to be specifically depleted in the set of predicted P-hotspot proteins (Table 4).
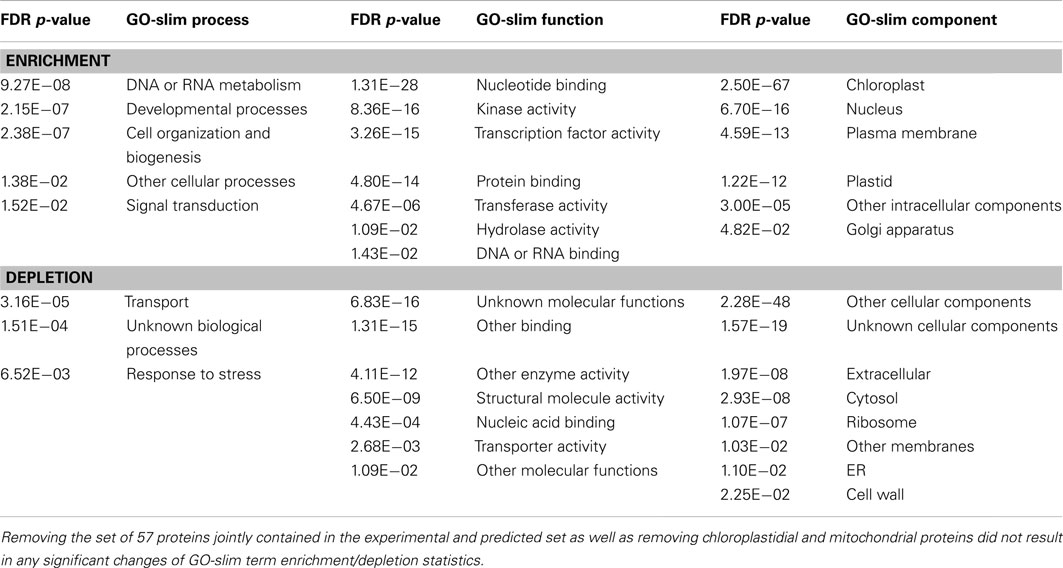
Table 4. Gene ontology-slim terms statistically enriched or depleted in the set of 9,599 Arabidopsis proteins (corresponding to 7,847 genes) predicted to contain P-hotspots based on SVM scores >1.
P-hotspots and disordered regions
It is known that P-sites tend to occur in sequence regions linking protein structural domains (Riano-Pachon et al., 2010) and, generally, in unstructured regions defined as the absence of secondary structure or any other regular folding patterns with a correspondingly relatively high structural flexibility. Those regions are characterized by their own compositional preferences that may primarily be associated with their unstructuredness and to a lesser extend with their phosphorylation (Iakoucheva et al., 2004). In order to assess the co-occurrence of P-hotspots and disordered regions and to determine whether P-hotspots are associated with specific amino acid compositional preferences, we scanned all Arabidopsis proteins for disordered regions using the GlobPlot program (Linding et al., 2003). GlobPlot relies on the propensities of all 20 amino acids to occur in disordered regions obtained from an analysis of experimentally determined protein structure and computed running averages over sequence windows of given lengths. Thus, the approach resembles the HotSPotter method by also exploiting sequence compositional preferences. Any significant co-segregation suggesting redundancy of the underlying compositional preferences is thus best discernible.
Of all 13,677 HotSPotter-predicted P-hotspots (required SVM score >1), 5,285 hotspots (39%) overlapped with GlobPlot-predicted disordered regions (see Materials and Methods) in the respected Arabidopsis proteins. By comparison, on average only 2,152 ± 25 randomly positioned hotspots (16%) overlapped with disordered regions. Thus, a significant preference for P-hotspots to occur in disordered regions is evident. However, by far not all predicted disordered regions (5,554 out of a total of 42,517 disordered regions of length 17 or longer) are simultaneously predicted as P-hotspots (Note that the number of overlapping hotspots with disordered regions may differ from the respective number of overlapping disordered regions and hotspots. For example, a single hotspot may overlap with two sequence-separated disordered regions.)
While there is a significant correlation between unstructuredness and the likelihood of being a P-hotspot, the two sets are sufficiently disjoint to conclude that P-hotspots are associated with specific amino acid compositional preferences picked up on by the developed HotSPotter methodology.
Availability of P-hotspot annotations and HotSPotter predictions under PhosPhAt
All hotspots based both on experimental P-sites as well as on predictions applying HotSPotter have been integrated into the PhosPhAt database and are available at http://phosphat.mpimp-golm.mpg.de. Figure 3 shows an example of a protein sequence containing both an experimental hotspot that was also predicted with SVM score >1. The HotSPotter predictions in the entire Arabidopsis proteome are available as Supplementary information of this publication (Table S1 in Supplementary Material) and under PhosPhat at http://phosphat.mpimp-golm.mpg.de/hotspots.html, where all training data are available as well.
Discussion
The potential of modulating the function of proteins by surface charge effects mediated by locally enriched phosphorylation events has gained increased attention in recent years (Serber and Ferrell, 2007; Strickfaden et al., 2007). Besides position-specific single phosphorylation events associated with defined structural changes or modulated binding affinities of the carrier protein, local enriched clusterings of several P-sites that are themselves less restricted to precise locations along a protein sequence, may have evolved as an efficient and mutation tolerant mechanism to allow modulated molecular responses to changed external conditions.
Here, we firstly confirmed earlier reports (Schweiger and Linial, 2010) that indeed P-sites exhibit a tendency to cluster along a protein sequence in A. thaliana. By using experimental data and considering only peptide-covered regions, thereby excluding possible artifacts from differential coverage, we validated this trend with increased confidence. In this context, it may be worthwhile to investigate the influence of the three-dimensional protein structure on the clustering in the one-dimensional sequence. If only solvent exposed sites can actually be phosphorylated and assuming that the polypeptide chain folds such that solvent exposed and surface buried segments alternate, P-site clustering would directly follow from structure alone.
We showed that the characteristic distances of any serine or threonine to the next P-site differ significantly from the equivalent distribution for tyrosine sites (Figure 1). This difference is likely caused by the differences in target recognition of serine/threonine kinases compared to tyrosine kinases (Sugiyama et al., 2008). For example, CDK kinases phosphorylating serines and threonines have been reported to be associated with P-clusters (Moses et al., 2007). By comparing the actual intervals between neighboring P-sites to randomized phosphorylation events using the unchanged protein sequence (referred to as P-flag randomization), we concluded that the characteristic preference of sequence interval 2 for pST sites to their respective next neighboring P-site is likely caused by the underlying preference of serines and threonines themselves to occur at this spacing.
Based on experimentally identified P-sites in the Arabidopsis proteome, we detected 79 phosphorylation hotspots in 75 proteins. In this study, we set out to expand this set by computational predictions as it can be assumed that the actual set of P-hotspot in the Arabidopsis genome is much larger. The principal difficulty with all experimental approaches to detect phosphorylation events lies in the fact that actual P-sites may not be phosphorylated under the respective experimental conditions tested, but under different ones not yet known or tested. Computational approaches can be assumed to potentially overcome this issue by identifying characteristic molecular properties of clustered P-sites in general. The obvious strategy to first predict individual P-sites using an established and plant-specific P-site prediction program (Durek et al., 2010) was determined to lead to inaccurate results with resulting distance intervals between neighboring predicted P-sites differing significantly from the experimental ones (Figures 1 and 2). We believe that this may be caused by the details of the underlying prediction method that aims to exploit the position-specific flanking sequence around P-sites essentially translating them into motifs. However, if multiple P-sites are contained in the flanking sequence, the extracted sequence motif will be a mix of signals influenced also by the neighboring P-sites falling into the respective flanking region.
Inaccurate phosphorylation hotspots predictions based on individual predicted P-sites may also result from inaccuracies in the underlying experimental detection of clustered P-sites. In mass spectrometry, it is very difficult and often ambiguous to precisely interpret mass spectra of closely related phosphopeptides (MacLean et al., 2008). Particularly, if more than one of neighboring S/T/Y sites can be phosphorylated under a given condition, fragment mass spectra often result from different phosphorylated versions of the same peptide sequence.
An obvious idea for predicting hotspots may be to treat hotspots as a sort of structural domain and apply established recognition programs. We tested the HMMER program that is based on HMMs (Finn et al., 2011) for suitability of P-hotspot prediction. However, no satisfying alignment of the different hotspot sequences was possible [21% (50%) median (maximal) pairwise sequence identity as outlined in the see Materials and Methods], and thus, the obtained HMMs performed poorly and were dismissed. The failure to create robust alignments is certainly associated to the very characteristic of clustered sites that P-sites are locally enriched but without any apparent spacing constraints.
Thus, a method had to be devised that relies less on the position-specific sequence context and more on the properties of a region as a whole. For this purpose, we based our prediction efforts on the amino acid composition of hotspot sequence regions, thereby ignoring any precise sequence order information. Feeding amino acid composition vectors associated with P-hotspot and non-hotspot sequences into SVMs resulted in predictions of surprisingly high accuracy (Table 3) in a rigorous cross-validation setting.
In any computational classification tasks, overfitting; i.e., using too many features to describe the particular dataset at hand leading to poor generalizability of the classification algorithm by emphasizing the noise rather than the true relationship, is of great concern, especially when the available dataset size for training is small as is the case here with only 79 hotpot based on experimental data associated with 365 sequence windows of length 17. Despite using many features (420) and presenting them to the SVM classification engine, the imposed cross-validation protocol with very little sequence redundancy between any training and testing partition (see Materials and Methods) efficiently safeguarded against falsely obtaining good prediction results due to overfitting as generalizability is truly tested. Instead of using all amino acid types as separate variables, the number of features could be reduced by prior filtering of uninformative features or by combining several features into meta-features, e.g., amino acid types sharing similar physic-chemical properties.
The choice of features is one of the most crucial steps in any classification approach. With regard to P-hotspot prediction, there may exist better features and combinations thereof than those used here. For example, the feature set used in this study may even be augmented by adding additional, but orthogonal information such as secondary structural state or disorder score. However, given the discussed shortcomings of sequence motif based methods to reproduce the correct spacing between neighboring P-sites, our goal was to provide a proof of principle that methods that rely more on sequence-independent features such as composition can lead to reasonable results when applied to the prediction of P-hotspots.
Phosphorylation sites have been reported to occur in linker regions between structural domains and unstructured regions, in general (Iakoucheva et al., 2004; Riano-Pachon et al., 2010). To safeguard against falsely predicting unstructured regions rather than specifically P-hotspots, we used a set of negative examples with serine/threonine/tyrosine contents similar to positive hotspot examples. Furthermore, in training the SVM, we deliberately included regions as negative examples that also contained single, but isolated (no hotspot) P-sites. Nonetheless, if indeed there is a link between unstructuredness and the tendency of becoming phosphorylated, disentangling both contributions remains a challenging endeavor. While a significant overlap between unstructured regions and P-hotspot was evident, the two structural feature sets were still largely disjoint (61% of all predicted P-hotspots and 87% of all predicted disordered regions in the Arabidopsis proteome do not overlap with the respective other structural feature) leading us to conclude that the developed HotSPotter method does indeed specifically capture sequence compositional preferences associated with P-hotspots and not those of unstructured regions alone.
In light of the obtained classification results, we believe the obtained predictions for the whole Arabidopsis proteome may be sufficiently accurate to warrant additional studies on those proteins and their phosphorylation hotspots. The set of proteins predicted to harbor P-hotspots was found enriched in functions associated with signaling and binding events thus confirming the surmised role of P-hotspots as modulators of binding events and localization. We predict P-hotspots to be present in around 9,500 Arabidopsis proteins. Thus, P-hotspot regulation may be more frequent than previously acknowledged.
By making all P-hotspot predictions in Arabidopsis thaliana available we hope to offer to the scientific community a starting point for experimental verification and further study of phosphorylation hotspot mediated regulation processes.
Conflict of Interest Statement
The authors declare that the research was conducted in the absence of any commercial or financial relationships that could be construed as a potential conflict of interest.
Acknowledgments
We thank Liam Childs for configuring the GlobPlot disordered region prediction program.
Supplementary Material
The Supplementary Material for this article can be found online at http://www.frontiersin.org/Plant_Proteomics/10.3389/fpls.2012.00207/abstract
Table S1. Predicted hotspots in the Arabidopsis proteome based SVM score >1. (Excel Table). File also contains data sheet of Arabidopsis P-hotspot containing proteins detected based on experimentally identified phosphorylation sites.
References
Barford, D., Hu, S. H., and Johnson, L. N. (1991). Structural mechanism for glycogen phosphorylase control by phosphorylation and AMP. J. Mol. Biol. 218, 233–260.
Benjamini, Y., and Hochberg, Y. (1995). Controlling the false discovery rate – a practical and powerful approach to multiple testing. J. R. Stat. Soc. Series B Stat. Methodol. 57, 289–300.
Boekhorst, J., Van Breukelen, B., Heck, A. Jr., and Snel, B. (2008). Comparative phosphoproteomics reveals evolutionary and functional conservation of phosphorylation across eukaryotes. Genome Biol. 9, R144.
Dunker, A. K., Brown, C. J., Lawson, J. D., Iakoucheva, L. M., and Obradovic, Z. (2002). Intrinsic disorder and protein function. Biochemistry 41, 6573–6582.
Durek, P., Schmidt, R., Heazlewood, J. L., Jones, A., Maclean, D., Nagel, A., Kersten, B., and Schulze, W. X. (2010). PhosPhAt: the Arabidopsis thaliana phosphorylation site database. An update. Nucleic Acids Res. 38, D828–D834.
Finn, R. D., Clements, J., and Eddy, S. R. (2011). HMMER web server: interactive sequence similarity searching. Nucleic Acids Res. 39, W29–W37.
Heazlewood, J. L., Durek, P., Hummel, J., Selbig, J., Weckwerth, W., Walther, D., and Schulze, W. X. (2008). PhosPhAt: a database of phosphorylation sites in Arabidopsis thaliana and a plant-specific phosphorylation site predictor. Nucleic Acids Res. 36, D1015–D1021.
Holt, L. J., Tuch, B. B., Villen, J., Johnson, A. D., Gygi, S. P., and Morgan, D. O. (2009). Global analysis of Cdk1 substrate phosphorylation sites provides insights into evolution. Science 325, 1682–1686.
Huala, E., Dickerman, A. W., Garcia-Hernandez, M., Weems, D., Reiser, L., Lafond, F., Hanley, D., Kiphart, D., Zhuang, M. Z., Huang, W., Mueller, L. A., Bhattacharyya, D., Bhaya, D., Sobral, B. W., Beavis, W., Meinke, D. W., Town, C. D., Somerville, C., and Rhee, S. Y. (2001). The Arabidopsis Information Resource (TAIR): a comprehensive database and web-based information retrieval, analysis, and visualization system for a model plant. Nucleic Acids Res. 29, 102–105.
Iakoucheva, L. M., Radivojac, P., Brown, C. J., O’Connor, T. R., Sikes, J. G., Obradovic, Z., and Dunker, A. K. (2004). The importance of intrinsic disorder for protein phosphorylation. Nucleic Acids Res. 32, 1037–1049.
Jimenez, J. L., Hegemann, B., Hutchins, J. R., Peters, J. M., and Durbin, R. (2007). A systematic comparative and structural analysis of protein phosphorylation sites based on the mtcPTM database. Genome Biol. 8, R90.
Joshi, H. J., Christiansen, K. M., Fitz, J., Cao, J., Lipzen, A., Martin, J., Smith-Moritz, A. M., Pennacchio, L. A., Schackwitz, W. S., Weigel, D., and Heazlewood, J. L. (2012). 1001 Proteomes: a functional proteomics portal for the analysis of Arabidopsis thaliana accessions. Bioinformatics 28, 1303–1306.
Linding, R., Russell, R. B., Neduva, V., and Gibson, T. J. (2003). GlobPlot: exploring protein sequences for globularity and disorder. Nucleic Acids Res. 31, 3701–3708.
Macek, B., Mijakovic, I., Olsen, J. V., Gnad, F., Kumar, C., Jensen, P. R., and Mann, M. (2007). The serine/threonine/tyrosine phosphoproteome of the model bacterium Bacillus subtilis. Mol. Cell. Proteomics 6, 697–707.
MacLean, D., Burrell, M. A., Studholme, D. J., and Jones, A. M. (2008). PhosCalc: a tool for evaluating the sites of peptide phosphorylation from mass spectrometer data. BMC Res. Notes 1, 30. doi:10.1186/1756-0500-1-30
Malik, R., Nigg, E. A., and Korner, R. (2008). Comparative conservation analysis of the human mitotic phosphoproteome. Bioinformatics 24, 1426–1432.
Moses, A. M., Heriche, J. K., and Durbin, R. (2007). Clustering of phosphorylation site recognition motifs can be exploited to predict the targets of cyclin-dependent kinase. Genome Biol. 8, R23.
Myers, E. W., and Miller, W. (1988). Optimal alignments in linear space. Comput. Appl. Biosci. 4, 11–17.
Nakagami, H., Sugiyama, N., Mochida, K., Daudi, A., Yoshida, Y., Toyoda, T., Tomita, M., Ishihama, Y., and Shirasu, K. (2010). Large-scale comparative phosphoproteomics identifies conserved phosphorylation sites in plants. Plant Physiol. 153, 1161–1174.
Riano-Pachon, D. M., Kleessen, S., Neigenfind, J., Durek, P., Weber, E., Engelsberger, W. R., Walther, D., Selbig, J., Schulze, W. X., and Kersten, B. (2010). Proteome-wide survey of phosphorylation patterns affected by nuclear DNA polymorphisms in Arabidopsis thaliana. BMC Genomics 11, 411. doi:10.1186/1471-2164-11-411
Schweiger, R., and Linial, M. (2010). Cooperativity within proximal phosphorylation sites is revealed from large-scale proteomics data. Biol. Direct 5, 6.
Serber, Z., and Ferrell, J. E. Jr. (2007). Tuning bulk electrostatics to regulate protein function. Cell 128, 441–444.
Strickfaden, S. C., Winters, M. J., Ben-Ari, G., Lamson, R. E., Tyers, M., and Pryciak, P. M. (2007). A mechanism for cell-cycle regulation of MAP kinase signaling in a yeast differentiation pathway. Cell 128, 519–531.
Sugiyama, N., Nakagami, H., Mochida, K., Daudi, A., Tomita, M., Shirasu, K., and Ishihama, Y. (2008). Large-scale phosphorylation mapping reveals the extent of tyrosine phosphorylation in Arabidopsis. Mol. Syst. Biol. 4, 193.
Tan, C. S., Jorgensen, C., and Linding, R. (2010). Roles of “junk phosphorylation” in modulating biomolecular association of phosphorylated proteins? Cell Cycle 9, 1276–1280.
Trost, B., and Kusalik, A. (2011). Computational prediction of eukaryotic phosphorylation sites. Bioinformatics 27, 2927–2935.
Keywords: protein phosphorylation, hotspots, Arabidopsis thaliana, support vector machines, regulation
Citation: Christian J-O, Braginets R, Schulze WX and Walther D (2012) Characterization and prediction of protein phosphorylation hotspots in Arabidopsis thaliana. Front. Plant Sci. 3:207. doi: 10.3389/fpls.2012.00207
Received: 11 July 2012; Accepted: 14 August 2012;
Published online: 05 September 2012.
Edited by:
Joshua L. Heazlewood, Lawrence Berkeley National Laboratory, USACopyright: © 2012 Christian, Braginets, Schulze and Walther. This is an open-access article distributed under the terms of the Creative Commons Attribution License, which permits use, distribution and reproduction in other forums, provided the original authors and source are credited and subject to any copyright notices concerning any third-party graphics etc.
*Correspondence: Dirk Walther, Max Planck Institute for Molecular Plant Physiology, Potsdam-Golm, Am Mühlenberg 1, Brandenburg 14476, Germany. e-mail:d2FsdGhlckBtcGltcC1nb2xtLm1wZy5kZQ==