- 1ICAR-Indian Institute of Wheat and Barley Research, Karnal, India
- 2ICAR-Indian Agricultural Statistics Research Institute, New Delhi, India
- 3Lokbharti-Sanosara Centre, Bhavnagar, India
Wheat genetic improvement by integration of advanced genomic technologies is one way of improving productivity. To facilitate the breeding of economically important traits in wheat, SNP loci and underlying candidate genes associated with the 36 agro-morphological traits were studied in a diverse panel of 404 genotypes. By using Breeders’ 35K Axiom array in a comprehensive genome-wide association study covering 4364.79 cM of the wheat genome and applying a compressed mixed linear model, a total of 146 SNPs (-log10 P ≥ 4) were found associated with 23 traits out of 36 traits studied explaining 3.7–47.0% of phenotypic variance. To reveal this a subset of 260 genotypes was characterized phenotypically for six quantitative traits [days to heading (DTH), days to maturity (DTM), plant height (PH), spike length (SL), awn length (Awn_L), and leaf length (Leaf_L)] under five environments. Gene annotations mined ∼38 putative candidate genes which were confirmed using tissue and stage specific gene expression data from RNA Seq. We observed strong co-localized loci for four traits (glume pubescence, SL, PH, and awn color) on chromosome 1B (24.64 cM) annotated five putative candidate genes. This study led to the discovery of hitherto unreported loci for some less explored traits (such as leaf sheath wax, awn attitude, and glume pubescence) besides the refined chromosomal regions of known loci associated with the traits. This study provides valuable information of the genetic loci and their potential genes underlying the traits such as awn characters which are being considered as important contributors toward yield enhancement.
Introduction
Wheat (Triticum aestivum L.) crop provides one-fifth of total food calories and a quarter of protein in the human diet on daily basis1. To meet the increasing food demand of growing population, the breeders focused on the varieties having higher yield and yield stability, increased resistance/tolerance to biotic and abiotic stresses. Approximately 10,000 wheat varieties worldwide2 including 448 wheat varieties in India (Gupta et al., 2018) have been notified. Agro-morphological characterization of germplasm is fundamental in order to provide information for plant breeding programs. The QTL mapping methods based on bi-parental mapping populations identify the genomic regions with low resolution, whereas, Genome-wide association studies (GWAS), based on linkage disequilibrium (LD), take diverse genetic background into consideration to dissect the genetic architecture of complex traits with high resolution. The GWAS in wheat has started gaining importance in the recent past mainly focusing on yield and yield related traits (Liu et al., 2014; Liu Y. et al., 2017; Sukumaran et al., 2014, 2018; Arruda et al., 2015; Gao et al., 2015; Maccaferri et al., 2015; Arora et al., 2017).
Advances in next generation sequencing technology provided valuable wheat genomic and plant breeding resources including high quality genome data (Brenchley et al., 2012; Jia et al., 2013; International Wheat Genome Sequencing Consortium (IWGSC), 2014; Chapman et al., 2015). Several high throughput SNP arrays viz., 9K (Cavanagh et al., 2013), 90K (Wang, 2014), 820K (Winfield et al., 2016), 660K (Cui et al., 2017), 35K (Allen et al., 2017), and TaBW280K (Rimbert et al., 2018) have been developed and utilized in wheat. These SNP arrays have been successfully used for GWAS in European winter and spring wheat (Zanke et al., 2014), CIMMYT spring wheat (Sukumaran et al., 2014), United States elite wheat breeding genotypes (Lin et al., 2016), a panel of CIMCOG (CIMMYT Mexico core germplasm) Kazakhstan, Russian, and European wheat genotypes (Turuspekov et al., 2017) and Chinese bread wheat cultivars (Sun et al., 2017). A substantial number of novel SNP variants have been identified using 35K SNP Breeders arrays in the Watkins collection of landraces for further improvement of modern elite cultivars (Winfield et al., 2018). From the 820K SNP array (using global selection of germplasm including elite cultivars, landraces, progenitor, and ancestral species of wheat), Breeder’s 35K Axioms array was developed which contains only mapped SNPs that are tailored to be most informative for specific purposes (Wilkinson et al., 2012; Borrill et al., 2015). 35K SNP array holds promise for detecting large scale variation in secondary and tertiary gene pools (Rasheed et al., 2017).
There are several agro-morphological traits which have been studied intensively and for which markers have been identified (Sukumaran et al., 2018). At the same time there are certain less explored traits for instance awn characters which can be considered as an alternative target for the improvement of wheat grain yield through their known functions including photosynthesis and increased water use efficiency (Rebetzke et al., 2016). The present study includes these characters of future importance hitherto not explored much until now. Moreover, most of the agro-morphological traits undertaken in this study are also utilized for the characterization of the genotypes using Distinctiveness Uniformity and Stability (DUS)3.
Some of the studied traits and the associated markers will be of immense importance in future toward developing input use efficient wheat varieties. Amongst them, genomic regions associated with days to heading (DTH) shall enable development of early maturing wheat genotypes to avoid terminal heat stress and allowing an intervening legume crop before rice in the ensuing season (Tewolde et al., 2006; Joshi et al., 2007). For GWAS, size and diversity of the panel plays a significant role as it is suggested that the smaller panel (<384 accessions) and large LD blocks identified in association studies may lead to the identification of false positive associations (Turuspekov et al., 2017). Keeping this in account, a panel of 404 diverse genotypes comprising of indigenous collections, local landraces, released varieties and other improved genotypes was used. The panel also included registered genetic stocks characterized for early maturity, resistance/tolerance to biotic/abiotic stresses, adaptation to different environments, plant architecture etc. (Kundu et al., 2010). These are the major drivers for trait improvement program, using molecular breeding approach. Moreover, India has unique climatic variations rendering wheat germplasm diversity as gold mines. Genotypes adapted to different agro-climatic zones of the country are present in the genotypic panel. Therefore these may be considered as representative of the three major spring wheat growing mega-environments viz., ME 1, ME 4, and ME 5 described by the International Maize and Wheat Improvement Center (CIMMYT) spanning across all the 5 continents4. This will allow the breeders to utilize the information in developing genotypes specific to different adaptation conditions.
The present GWA study is the first attempt to undertake large scale evaluation of 35K Axiom array in a diverse panel of 404 Indian wheat genotypes. The 35K Breeders’ Array was selected for present work due to its proven efficacy on panel of 1807 accessions of hexaploid wheat (804 accessions from Watkins Collection, a collection of wheat landraces made by A. E. Watkins in the 1920s and 1930s and 1003 modern and elite accessions) from 32 countries (Winfield et al., 2018). The aim of the study was to (i) identify significant MTAs for 36 agro-morphological traits for future breeding and (ii) mining putative candidate genes underlying the corresponding traits of interest. Furthermore, tissue and growth stage-specific gene expression data was also examined providing support to the detected candidate genes. For complex quantitative traits, the association panel was phenotyped at two locations for 2 years. The outcome of this study could be used to make effective strategies for the development of new varieties coupled with economic traits.
Materials and Methods
Plant Material
A set of 404 bread wheat (T. aestivum L.) genotypes comprising of indigenous collections (91), landraces (8), released varieties (134), genetic stocks (43), and improved genotypes (128) was used to constitute a diverse association panel. The diverse lines were selected on the basis of pedigree, to reduce associations of spurious markers as it provides a buffer against skewness in terms of the environmental effects. Recently, we analyzed trait based diversity analysis using Shannon Index with 16 traits out of 36 traits with a collection of 7,000 diverse germplasm lines (unpublished work). Out of these, 450 genotypes comprising of indigenous collections, landraces, released varieties, improved genotypes and genetic stocks for various traits were selected from 7,000 lines which was downsized to 404 genotypes after eliminating admixtures, duplicates, etc. Comparing the trait based diversity of these selected 404 genotypes using Shannon Index endorsed it as good representative of 7,000 germplasm lines, thereby proving the suitability of 404 genotypes for GWA study (Supplementary Table S1). Seeds of 404 genotypes were obtained from the Germplasm Resource Unit, ICAR-IIWBR (Indian Institute of Wheat and Barley Research), Karnal, Haryana, India, which acts as a nodal center for wheat in the country. Detailed information with pedigree for each genotype is given in Supplementary Table S2.
Field Trials and Phenotyping
The 404 genotypes were evaluated for 30 qualitative characters at the experimental field of ICAR-IIWBR, Karnal during the crop season 2016–2017. A subset of 260 genotypes was phenotyped for six quantitative traits [days to heading (DTH), days to maturity (DTM), spike length (SL), plant height (PH), awn length (Awn_L), and leaf length (Leaf_L)] at three locations viz., Experimental field, Karnal (29°42′N, 77°02′E); Seed Farm, Karnal (29.7138° N, 76.9943°E), Haryana, India and Lokbharti-Sanosara Centre, Bhavnagar (21°46′ N 72°11′ E), Gujarat, India during year 2016–2017. Besides these three environments, an additional environment was taken by phenotyping the subset in crop season 2017–2018 at ICAR-IIWBR, Karnal. Experiment was conducted in two replications following alpha lattice design. To minimize the variations, every genotype was planted with a dibbling tool named IIWBR Dibbler (Sharma et al., 2016) having four rows. The plant to plant distance was 10 cm and row to row distance was maintained at 20 cm. This unique sowing method has helped in avoiding confounding effects of extraneous errors and improved the precision in phenotyping leading to moderate to high estimation of heritability (H2) thereby enhancing the probability of identifying genes of minor effects related to complex traits (Sharma et al., 2016).
Thirty six agro-morphological characters including coleoptiles anthocyanin coloration (C_Col), plant growth habit (PGH), foliage color (Fol_Col), flag leaf anthocyanin coloration of auricle (Aur_Col), flag leaf hairs on auricle (pubescence) (Aur_Pub), flag leaf attitude (Leaf_Att), ear emergence/days to heading (DTH), flag leaf waxiness of sheath (Wax_LS), flag leaf waxiness of blade (Wax_LB), ear waxiness (Wax_Ear), waxiness of peduncle (Wax_Ped), flag leaf length (Leaf_L), flag leaf breath (width) (Leaf_Br), PH, ear shape (Ear_S), ear density (Ear_D), ear (spike) length (SL), awn presence (Awn_P), awns length (Awn_L), awn color (Awn_Col), awn attitude (Awn_Att), outer glume pubescence (Glu_Pub), ear color (Ear_Col), lower glume shoulder width (Sh_Wid), lower glume: shoulder shape (Shl_Sh), beak length (Beak_L), beak shape (Beak_Sh), spike (peduncle) attitude (Ped_Att), grain coloration with phenol (Grn_Ph), grain color (Grn_Col), grain shape (Grn_Sh) grain germ width (Germ_Wid), brush hair length (Brush_L), seed (grain) size (Grn_Size), grain hardness (texture) (Grn_Tex) and DTM were recorded as per guidelines laid out by Protection of Plant Varieties and Farmers’ Right Authority (PPV and FRA, 2011)5. Procedure of recording the data for each trait is summarized in Supplementary File S1. Qualitative traits were recorded as binary (presence or absence), ordinal (visual scale of the expression intensity of a characteristic) and nominal (color or shape) (Supplementary Table S1). For association analysis, a total of five environments (E1–E5) were considered namely, E1 – average of two replications at ICAR-IIWBR, Karnal (2016); E2 – average of two replications at Seed Farm, Karnal (2016); E3–average of two locations of Karnal (2016); E4 – average of two replications at ICAR-IIWBR, Karnal (2017) and E5 – average of two replications at Bhavnagar, Gujarat (2016).
Statistical Analysis
Phenotypic data was analyzed using SAS v.9.3 (SAS Institute 20116). Pearson pairwise correlation was calculated for all the traits. Histograms were created in R (R Development Core Team, 2013) using the hist() function. The PROC CORR procedure was employed to calculate correlations among phenotypes. Variance components for the quantitative traits were analyzed using general linear model to detect the effect of genotypes, environment, replication and genotype × environment interaction. All sources of variation were considered as random effects. The broad sense heritability for the traits was estimated by the formula H2 = VG/(VG + GE) where VG and VE represent estimates of genetic and environmental variance, respectively.
SNP Genotyping and Filtering
Genomic DNA was extracted from 15 days old seedlings according to the CIMMYT Molecular Genetics Manual (Dreisigacker et al., 2013). A Nanodrop 1000 spectrophotometer was used for quantifying DNA at 260 nm absorbance (Biodrop Touch PC+125). The DNA samples were used for genotyping with 35K Axiom® Wheat Breeder’s Array (Affymetrix UK Ltd., United Kingdom). Quality preprocessing of 35,143 markers obtained from 35K chip was done by using PLINK software (Purcell et al., 2007). Markers with more than 5% missing values, less than 5% minor allele frequency (MAF) and individuals with more than 15% missing SNP calls were removed from the dataset. Markers with no known chromosomal positions, based on high density consensus map generated by using five mapping populations7 (Allen et al., 2017), were also removed. Duplicate markers were further removed by R/QTL software (Broman et al., 2003; Arends et al., 2010).
Genetic Diversity and Population Structure Analysis
The basic statistics such as genetic diversity (GD) and polymorphism information content (PIC) was evaluated by using PowerMarker v3.2.5 (Liu and Muse, 2005). The model-based Bayesian cluster analysis program, STRUCTURE v2.3.4 (Hubisz et al., 2009) was used to infer the population structure. A total 100,000 burn-in periods followed by 100,000 Markov Chain Monte Carlo (MCMC) iterations from K = 2 to K = 7 clusters were used to identify the optimal cluster (K). Three independent runs were generated for each K. The results of the analysis were used as input to the Structure Harvester tool (Earl and VonHoldt, 2011) to predict the best K-value based on Evanno method (Evanno et al., 2005). Principal component analysis (PCA) and Neighbor-joining (NJ) tree were created to validate population stratification with the software GAPIT (Lipka et al., 2012) and DARwin v6 (Perrier and Jacquemoud-Collet, 2006), respectively.
Linkage Disequilibrium
For Linkage disequilibrium analysis, r2 (squared correlation coefficient) values among markers of all pairs of loci were calculated using PLINK 1.9 tool8 (Purcell et al., 2007). Default window size cut off of r2 value was used for this analysis. Finally, LD plotting was done for three sub genomes (A, B, and D genomes), on the basis of centiMorgans (cM) distance, using ggplot2 package of R Bioconductor (Wickham et al., 2009). The percentage of marker pairs below the critical LD (r2 > 0.02) was also compared in the sub-genomes. Pairwise LD estimates in the region of interest for significantly associated markers were investigated using Haploview 4.2 (Barrett et al., 2005).
Association Analysis
Association analysis was performed using compressed mixed linear model (CMLM) implemented by Genomic Association and Prediction Integrated Tool (GAPIT) in R (Lipka et al., 2012) which took into account a K-PC model (Zhao et al., 2007) where kinship information together with the first three principal components (PC) as covariates were included for GWAS, which further improves statistical power. Kinship matrix was iteratively calculated using the VanRaden method (VanRaden, 2008). The best fit of the model was evaluated on the Q-Q plots generated by the model. A threshold of -log10 P > 4 (-log10P ≥ 4 for quantitative traits) was used to state significant marker trait associations. Associations with false discovery rate (FDR) adjusted at 10% was used to determine the P-values thresholds.
Putative Candidate Gene Analysis and Expression Data
To find candidate genes or putative related proteins of SNP flanking-regions, BLASTx search was conducted for significant MTAs against recently released genome sequence IWGSC RefSeq v1.09. Each MTA was searched for IWGSC sequence information in Ensembl plant for T. aestivum10. The flanking sequence available for the SNP marker with maximum bases (1,000 bases before and after the SNP) was considered for BLASTx analysis. We also looked at the number of high confidence genes adjacent to the significant MTAs using the RefSeq v1.0.Gene Ontology (GO) annotation of the potential candidate genes was carried out using Blast2GO pro tool v.3.1.3 (Conesa and Götz, 2008). The expression profile of all the putative candidate genes associated with the identified SNPs were checked using wheat RNA-seq expression database of polyploid wheat11. This database consists of the transcript profile of five tissues (grain, leaf, root, spike, and stem) at 3 different time points (growth stages) each and environmental treatments (Pearce et al., 2015). Expression of the gene was measured in units of FPKM (Fragments Per Kilobase of transcript per Million mapped reads). Expression profile was carried out to further provide supporting evidence to corroborate candidate genes (tissue and stage of expression).
Results
Phenotypic Variation and Correlation Analysis
The frequency distribution of phenotypic data of 404 genotypes characterized for 30 traits is given in the Supplementary Figure S1. The phenotypic variations of six quantitative traits (DTH, DTM, PH, SL, Awn_L, and Leaf_L) was recorded in multiple environments. Phenotypic variation of these traits among genotypes was corroborated by mean, standard deviation, range and coefficient of variation (Supplementary Table S3). The mean value of DTH, DTM, PH, SL, Awn_L, Leaf_L varied from 66.72 to 92.0 days, 105.28 to 135.37 days, 94.45 to 113.18 cm, 10.33 to 13.30 cm, 0 to 19.50 cm, 16.25 to 41.40 cm, respectively. This data revealed extensive variation in the traits of the diverse set suggesting the suitability of genotypic panel for association studies. Phenotypic values for each of the six traits were found normally distributed (Supplementary Figure S2). Analysis of variance (ANOVA) was conducted to test the effects of genotype (G), environment (E) and their interactions (G × E). Significant differences were observed among the genotypes (p < 0.0001), the effect of environment and their interaction (G × E) indicating the environmental effect on these traits (Supplementary Table S4). Estimates of correlation coefficients of this combined analysis are shown in Supplementary Table S5 and in Figure 1, a positive correlation was observed for DTH with DTM (0.36), SL (0.18), and PH (0.17) while SL exhibited negative correlation with PH (-0.17).
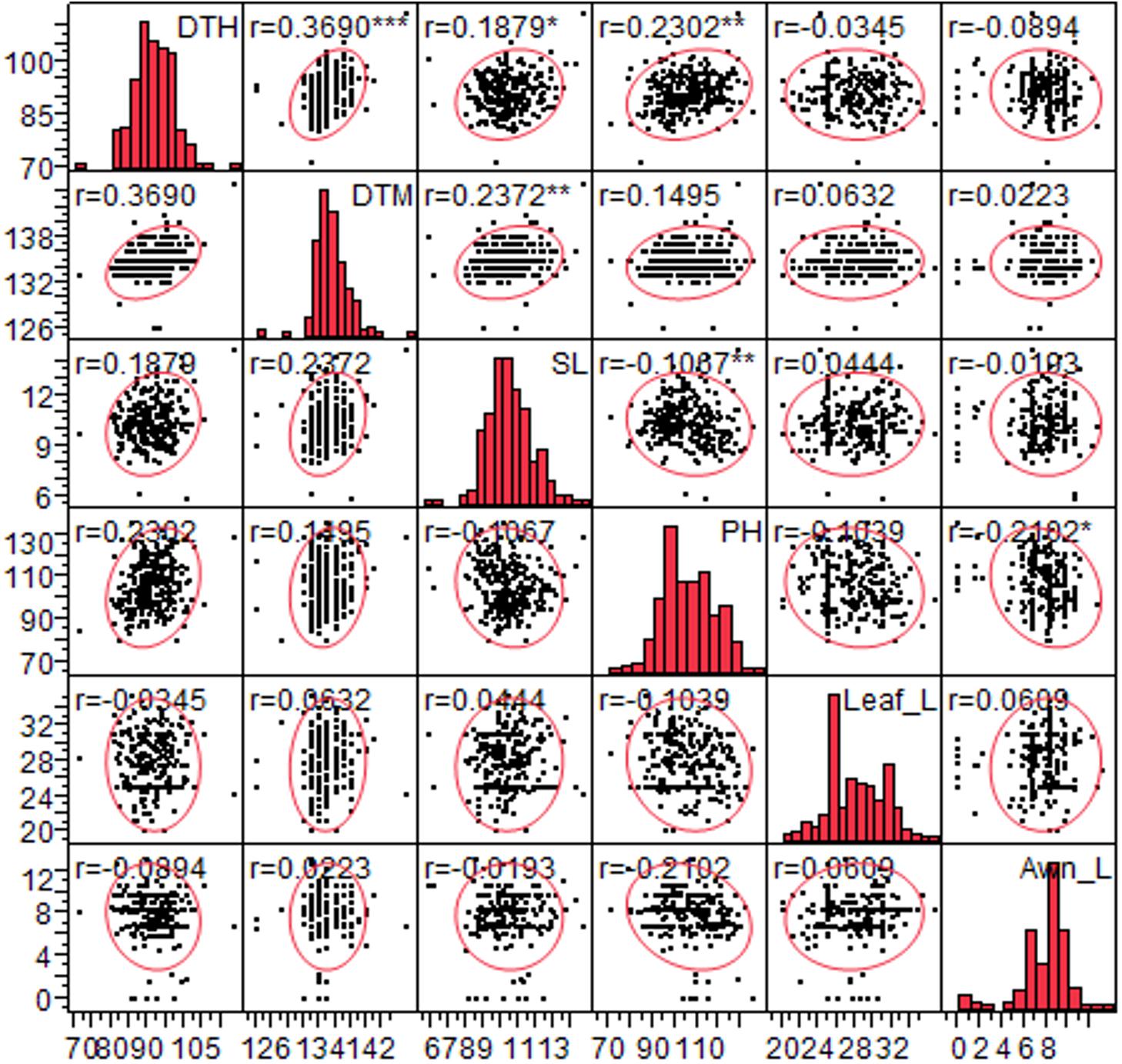
Figure 1. Correlation between six quantitative traits viz. Days to heading (DTH), days to maturity (DTM), spike length (SL), plant height (PH), leaf length (Leaf_L) and Awn length (Awn_L) for 260 genotypes. Significant correlations are designated with asterisk (∗).
SNP Markers Statistics
Quality preprocessing of 35,143 markers obtained from 35K chip was done by using PLINK software12 (Purcell et al., 2007). 6,041 monomorphic markers were excluded from the analysis. Out of 29,102 SNP markers, 8,673 SNPs failed frequency test (MAF <0.05) and 1,383 markers removed failing missingness test >0.05. Only 2 individuals for low genotyping (MIND >0.2) were removed. Further, 4,740 SNPs were excluded for lack of their physical position and 146 being duplicate markers. After filtering, 402 genotypes with 14,160 SNP markers were used for GWAS. These markers covered a genetic distance of 4364.79 cM, with an average density of 0.3 cM. Marker density was found highest for B genome (1029.6 markers per chromosome) followed by A (788.9 markers per chromosome) and D genome (207.8 markers per chromosome). Among the genome, chromosome 2B had the highest number of markers (1324) while 4D chromosome spanned the lowest number of markers (55) (Supplementary Table S6).
Population Structure and Linkage Disequilibrium
The mean GD and the PIC for the whole genome were 0.36 and 0.29, respectively. Both GD and PIC of the A genome (0.357 and 0.286) and B genome (0.372 and 0.291) were higher than the D genome (0.345 and 0.276). The number of markers, map length, GD and PIC for each chromosome are shown in Supplementary Table S6.
In the present study, the population structure of a diverse panel of 402 wheat genotypes was investigated on the basis of a ΔK method of model-based Bayesian clustering using 14,160 SNP markers. Population structure analysis clearly indicated the existence of three distinct major subpopulations in the bread wheat panel, which was found consistent with the results of the PCA and neighbor-joining (NJ) tree analysis (Figure 2). Subgroup I, the largest group with 169 accessions, was dominated by recently released varieties and breeding lines adapted to Northern wheat growing zone of the country and genetic stocks for biotic resistance (Rust and Karnal bunt). DPW621-50 (2011), HD2967 (2013), WH1105 (2013), HD3059 (2013), DBW88 (2014), HD3086 (2014), and DBW90 (2014) are some recently released varieties. The pedigree showed that the varieties DPW621-50, DBW88, and HD3059 had common pedigree (Supplementary Table S2). Breeding lines for instance HUW675, HUW666, HPW373, HD3133 and varieties MP1201, HS507, HS542 and WH1105 had MILAN in the parentage. Subgroup II consisted of 87 accessions, mainly comprising local landraces from pre green revolution era; Subgroup III had 146 accessions, predominantly from the warmer region of the country and also comprised of early maturing genotypes (short maturity duration of about 120 days) released for late sowing (toward end of November and to mid of December) in different agro climatic zones viz., K8962, Raj3765, DBW16, and MP3336 having HD2160 (a triple dwarf genotype) as a common progenitor in their background (Supplementary Table S2). Early Mexican cultivars that paved the way for green revolution, Sonalika, SONORA64, Safed Lerma appeared in this cluster along with the derivatives of Sonalika like UP262, HW2001, and Lok54.
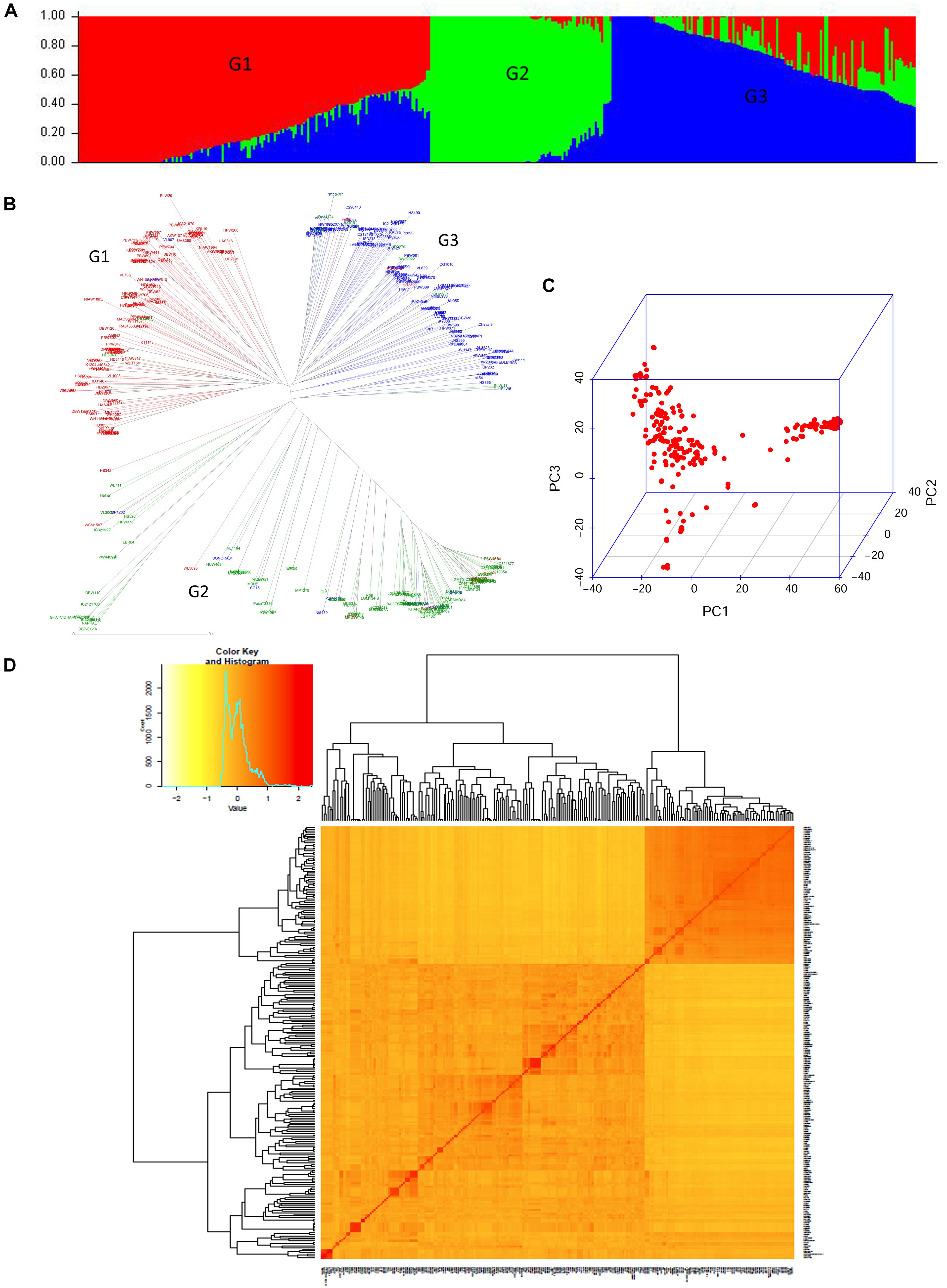
Figure 2. Population structure and diversity analysis of current GWA study panel. (A) Population structure based on STRUCTURE when K = 3. (B) Neighbor-joining based clustering observed in the study panel using 14160 SNP markers. (C) Three-dimensional plot of the first three principal components, and (D) heat map of pairwise kinship matrix of 402 genotypes.
Linkage disequilibrium (LD) decay distance in the selected panel was found highest in the D genome which decayed at about 5 cM (r2 = 0.02) as compared to ∼2 cM in A and B genomes (Supplementary Figure S3). Faster LD decay in D genome vis-à-vis A or B genome has been reported earlier in GWAS of wheat (Lopes et al., 2013; Zhang et al., 2013). With an increase of the genetic distance, the r2 value of the A, B, and D genomes decreased gradually. Genome A (62.7%) showed the highest frequency of physically linked locus pairs followed by B (58.0%) and D (53.6%) genomes.
Genome–Wide Association Analysis
In order to detect the most significant marker-trait associations, CMLM was employed to deal with the confounding effect of the population structure. This was followed by the inspection of Q–Q plots and Manhattan plots for evidence of P-value inflation (Supplementary Figures S4, S5). Based on the stringent criterion of -log10 P > 4, we detected 99 significant MTAs ranging from 7.49 e-05 to 2.47 e-11 for 17 qualitative traits (Figure 3, Table 1, Supplementary Figure S4, and Supplementary Table S7) explaining 5.3–33.3% phenotypic variations. It is imperative to note that, not every gene is likely to be represented by 35K SNP array based markers. Therefore the markers in linkage disequilibrium indicates either it is the causative gene itself or might be in close linkage to the causative gene. For color related traits, a total of 22 SNPs were found associated with coleoptile color on five chromosomes, i.e., 2A, 4B, 6B, 5B, and 6A. However, the genomic region on chromosome 6B was represented by eighteen SNPs, mapped within genetic distance of 62.83–67.99 cM (distance interval of 5.15 cM) which collectively explained 23.9% of the phenotypic variation (Supplementary Figure S4 and Supplementary Table S7). For awn and ear color, a significant MTA was detected on chromosome 1B but at different loci, i.e., at 24.64 cM accounting for a phenotypic variation of 8.8% and at 8.24 cM explaining 10.5% phenotypic variation, respectively (Supplementary Figure S4 and Supplementary Table S7).
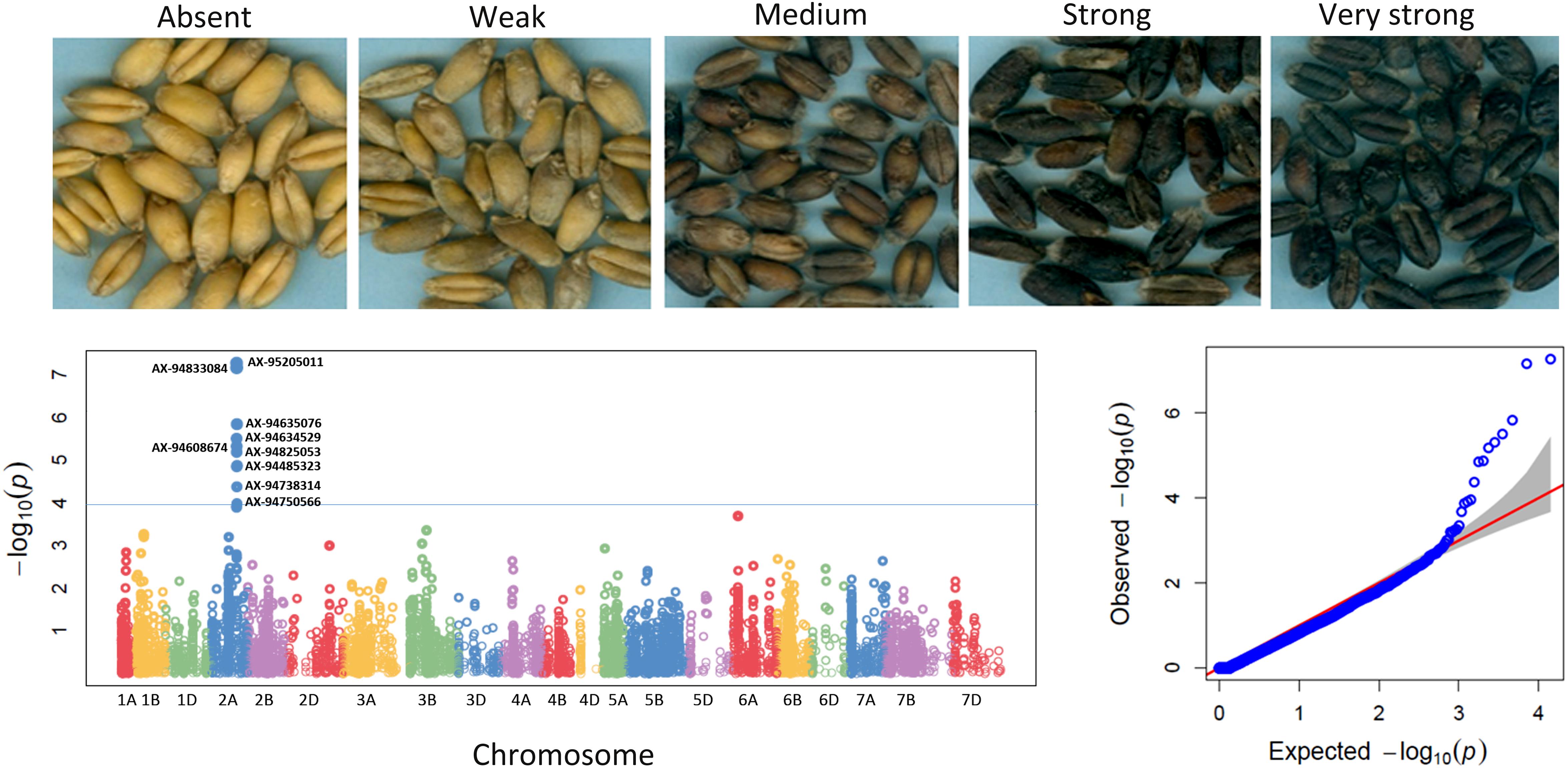
Figure 3. Five different categories of phenol coloration viz. absent, weak, medium, strong, and very strong are shown. These variations in coloration are based on the phenol oxidase activity present in the seed coat. Manhattan plot and Q–Q plot for the trait of grain coloration with phenol (Grn_Ph) as observed in the study.
For waxiness characters, two MTAs were identified for leaf sheath wax on chromosomes 6D (7.48 e-05), 3A (P < 0.0001) hitherto not reported (Supplementary Figure S4) and one MTA for peduncle wax on chromosome 3A (2.87 e-05). MTAs associated with leaf sheath wax and peduncle wax contributed to the trait negatively. For glume related traits, six MTAs were detected for glume pubescence on chromosomes 1A (2), 1B (1), and 2B (3) explaining phenotypic variation ranging from 8.9 to 12.0% with positive effect. For shoulder width and brush length, significant associations were detected on chromosome 2D and 3A, respectively (Supplementary Figure S4 and Supplementary Table S7). For awn related traits, a genomic region found associated with awn attitude represented by three SNP markers (AX-94613491, AX-94519690, and AX-94453668) on chromosome 5A spanning a region from 59.99 to 70.36 cM. The phenotypic variation explained by SNPs ranged from 24.2 to 25.0% and all the three SNPs showed positive effect on the awn attitude. For awn presence, several markers or regions were identified across the chromosomes (1A, 1B, 1D, 2A, 2B, 3A, 3B, 4A, 4B, 5A, 5B, 6B, 6D, 7A, and 7B) explaining phenotypic variation ranging from 28.7 to 33.9%. A chromosomal region of 12.22 cM (66.99–72.22 cM) on chromosome 5A harbored significant MTAs associated with multiple traits (awn length, auricle color, and awn presence).
For plant growth habit two SNPs were detected on chromosome 1B but at different loci, one at 8.24 cM and other at 38.86 cM indicating the role of two independent loci on chromosome 1B explaining phenotypic variation ranging from 20.0 to 21.2%.
For grain related traits, in the current study 9 SNPs on chromosome 2A spanning 0.71 cM region (124.18–124.89 cM) were found significantly associated with phenol color indicating the importance of this region. These SNPs explained 15.1–18.6% phenotypic variation. For grain texture (phenotype scored as hard or soft), a total of two regions were detected; one on chromosome 7A containing three markers (100.09 cM) and other on chromosome 6B comprising four markers (62.83 cM). Both these MTAs for grain texture contributed negatively to the trait. For germ width, significant association was detected on chromosome 4A. Only one MTA (AX-94670534) for ear shape was detected on chromosome 7B explaining 8.3% of phenotypic variance having negative effect. We did not find any significant MTA for ear density.
For the six quantitative traits (DTH, DTM, PH, Leaf_L, SL, and Awn_L), a total of 47 significant SNPs were identified in five environments which explained 5.2–47.3% of phenotypic variation (Table 2 and Supplementary Figure S5). We successfully detected both previously reported genomic regions and novel loci for the traits in wheat (Tables 1, 2 and Supplementary Figures S4, S5). Flowering time or DTH is a crucial trait which affects the adaptation of wheat in its target environment. A total of 5 SNPs for DTH were detected on chromosome 4A, 5A, and 7D with phenotypic contributions ranging from 19.1 to 32.5%. Out of the 5 SNPs associated with DTH, SNP AX-9454244 on chromosome 4A (78.09 cM) and SNP AX-95187165 (89.02 cM) on chromosome 5A showed pleiotropic effect on DTM. For DTM, a locus on chromosome 2A at 179.61 cM has been detected in the three environments (E2, E3, and E5; Table 2) explaining the average phenotypic variation of 22.9% suggesting the importance of this region while another locus on chromosome 2A at 83.23 cM was observed for three traits (DTM, PH, and SL). Two MTAs were detected on chromosome 5A (AX-95187165, 89.02 cM and AX-95652310, 72.22 cM), indicating the presence of two independent loci on chromosome 5A with a positive effect on DTM.
For PH, a total of six MTAs were identified, one each on chromosome 1B (24.64 cM), 2A (83.23 cM), 2B (104.59 cM), 7A (29.9 cM), and two on 5D (1.58 cM) considering all the environments (Table 2). MTAs significantly associated with SL were mainly distributed on chromosome 1B, 2A, 3A, 3B, 3D, 5A, 7A, and 7B. The phenotypic variation contributed by SNPs ranging from 8.3 to 27.6% for SL. The SNP AX-94517196 (83.23 cM) on chromosome 2A (Supplementary Figure S5 and Table 2) showed 15.6% of phenotypic variation having positive effect on the trait. Three MTA for flag leaf length were detected on chromosome 4A, 5A, and 7B explaining phenotypic variation ranging from 9.7 to 15.7% (Table 2). The SNP AX-95196340 and AX-94406861 on chromosome 7B and 5A, respectively, showed positive effect on leaf length. For awn length, a total of 8 significant MTAs were identified mainly distributed on chromosome 1B (1), 3B (1), 4B (2), 6D (2), and 7B (2) which collectively explained 18.0% of the phenotypic variation. Two loci, one at 1.72 cM on chromosome 7B and other at 17.65 cM on chromosome 6D shared association with awn length and awn presence.
Pleiotropy Effect
In the present study, we observed same SNPs with multiple traits which could be due to pleiotropy or different causal genes in LD for instance SNP AX-94656878 at 83.23 cM (chromosome 2A) explained variation for two traits (PH and DTM) (Table 2). Similarly, another locus (SNP AX-94527988) on chromosome 3A at 115.9 cM was found pleiotropic with LS_Wax and Ped_Wax (Table 1). Also, the two SNPs associated with DTH on chromosome 4A (78.09 cM) and 5A (89.02 cM) were found linked with DTM (Table 2). The pleiotropic effects observed in the study were in agreement with the Pearson’s correlations observed between the agronomic traits (Figure 1).
Identification of Putative Candidate Genes and Expression Analysis
We identified several putative candidate genes such as storage protein activator (spa), beta-amylase 2 (bmy2), cytochrome P450, shikimate kinase, b-ZIP transcription factor, for the phenotypic variations of the traits (Table 3). These putative proteins identified were highly homologous to different species of Triticum or Aegilops. Highest number of putative candidate genes were observed for MTAs associated with SL encoding a total of five candidate genes [actin-related protein subunit 3 (ARPC3), DIMINUTO, replication protein A, carboxypeptidase D, and basic region/leucine zipper].
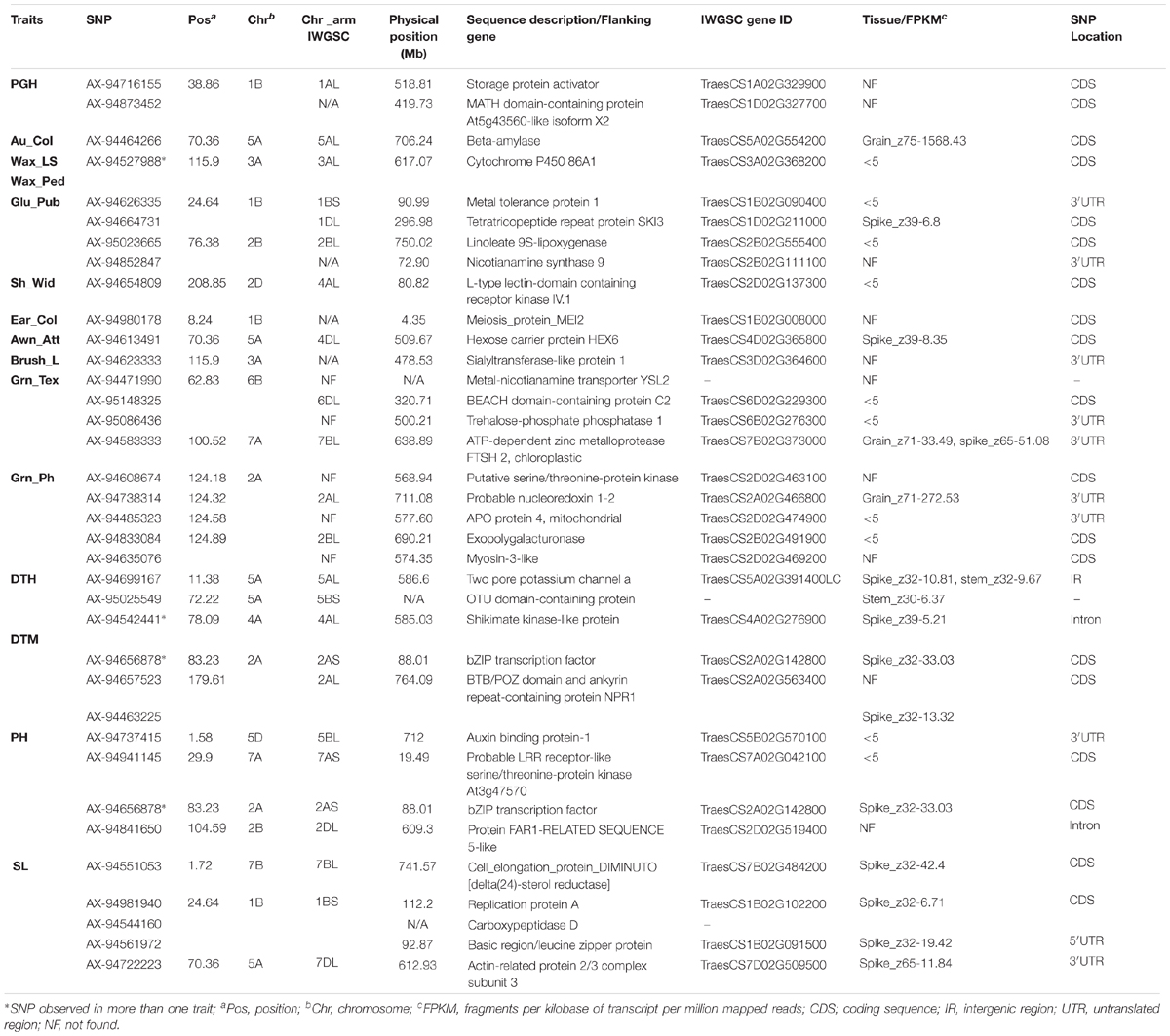
Table 3. SNPs significantly associated with agro-morphological traits and putative candidate genes identified in the study.
To determine the relative expression profile of the identified transcripts in broad range of tissues from different developmental stages, the published RNA-seq data and the Wheat-Exp web tool of the wheat cultivar, Chinese Spring was explored (Choulet et al., 2014; Pearce et al., 2015). The expression profile of significant SNPs encoded putative candidate genes is given in the Table 3 and Supplementary Table S8. FPKM value > 5 was considered for tissue and developmental specific expression check.
Effect of Favorable Alleles on Agronomic Traits
Early maturing, high yielding wheat genotypes are of immense importance toward increasing the cropping intensity as well as ensuring high input use efficiency particularly for inputs like water, which are going to be scarce. Therefore the present study dissecting important agronomic traits such as DTH, PH, and SL enables utilization of available diversity by exploiting associated markers. SNP alleles which led to decrease in DTH, PH and increase in SL were considered as “favorable alleles” and vice-versa was defined as “unfavorable alleles.” Figure 4 depicted higher frequency of favorable alleles which led to decrease in PH and DTH with phenotypic variation of 17.6 and 9.0%, respectively. Similarly, by increasing the number of favorable alleles, SL increased with R2 of 6.4%. Results of the study showed that favorable alleles exhibited significant positive effects on the phenotypic traits as compared to the unfavorable alleles. This would help in cultivar adaptation and finally to grain yield.
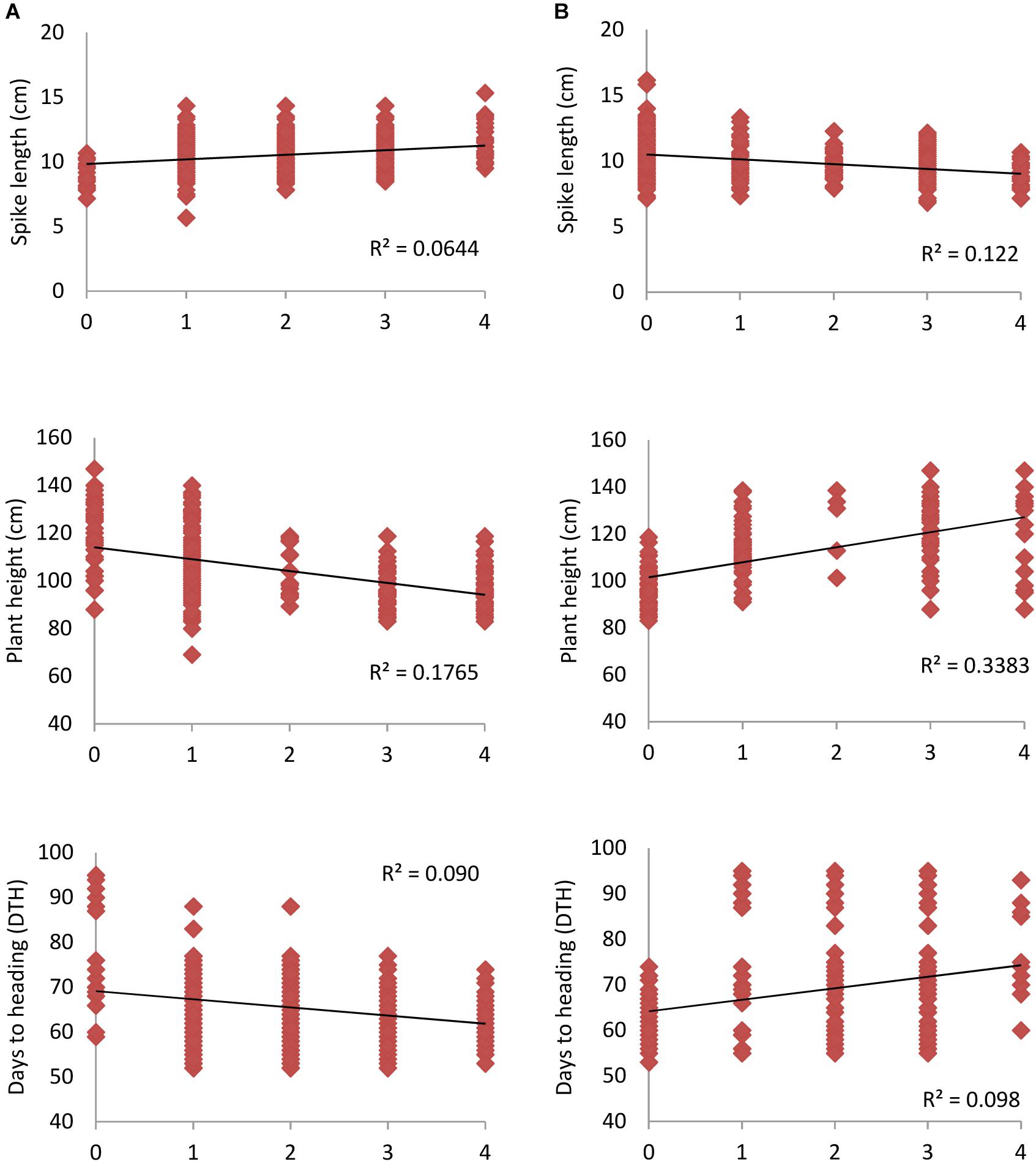
Figure 4. Linear regression between number of (A) favorable alleles (B) unfavorable alleles for spike length (SL), plant height (PH) and days to heading (DTH). Higher regression value (R2) was observed in case of PH when compared to SL and DTH for both favorable and unfavorable alleles.
Traits Sharing Co-localized Genomic Regions
In the present study, the most promising co-localized genomic region was identified on chromosome 1B at 24.64 cM associated with four traits (Glu_Pub, Awn_Col, SL, and PH) and 26.22 cM with Awn_L. The genomic co-location of loci (24.64 cM) with four traits implies either a strong physical linkage between genes underlying these important traits, or a pleiotropic effect. Therefore, to dissect the genetic cause of the observed association, LD patterns and candidate genes underlying the region and transcript profile of the targeted region were investigated (Figure 5). The SL and PH at this locus showed greater LD estimates (>0.8) indicating closely dependent biological processes. Whereas comparatively moderate and low LD was observed with Glu_Pub and Awn_Col, respectively, might be due to low overall recombination vis-à-vis greater recombination frequency with other genomic regions. Notably, this locus harbored three candidate genes for SL. SNP AX-94981940 (-log10 P = 4.21), was annotated as a replication protein A subunit and its transcript expression was almost solely abundant in the young spike at Zadoks 32 stage (Table 3). Replication protein A has important role as single strand DNA binding protein in various DNA metabolic pathways (Aklilu and Culligan, 2016). Similarly, the other SNP encoded a protein carboxypeptidase-D which functions as a positive regulator of grain size in rice (Li et al., 2011). The sequence of SNP AX-94561972 linked with SL annotated as basic region/leucine zipper protein. The bZIP transcription factor family plays an important role in growth, development, and response to abiotic or biotic stresses (Yin et al., 2017). It is interesting to note that PH shared common significant loci with SL showing high correlation between these traits in concurrence with the previous results (Sukumaran et al., 2014). SNP AX-94626335 (-log10 P = 5.10), associated for Glu_Pub at this locus (24.64 cM) was annotated as metal tolerance protein (MTP) which is known for its potential involvement in providing a sink for trace element storage in wheat grains (Vatansever et al., 2017). Earlier Echeverry-Solarte et al. (2015) also reported the influence of glume pubescence on SL by identification of a cluster of co-localizing QTL on same locus for both the traits. Another Glu_Pub associated SNP AX-94664731 on chromosome 1B at 24.64 cM annotated tetratricopeptide repeat protein SKI3, showed highest expression at Zadok39 growth stage of spike. The TaFlo2-A1 gene, an orthologous of rice Flo2 has four motifs of tetratricopeptides found associated with thousand grain weight (Sajjad et al., 2017) and F-box protein containing domains of tetratricopeptides known to regulate plant development and their abundance during spike development in wheat (Hong et al., 2012). The expression patterns of the putative candidate genes in different organs (Figure 5) are consistent with the RNA-seq FPKM expression patterns. The single genomic locus identified for these important related traits, needs further studies to fine map and validate the identity of the causal locus.
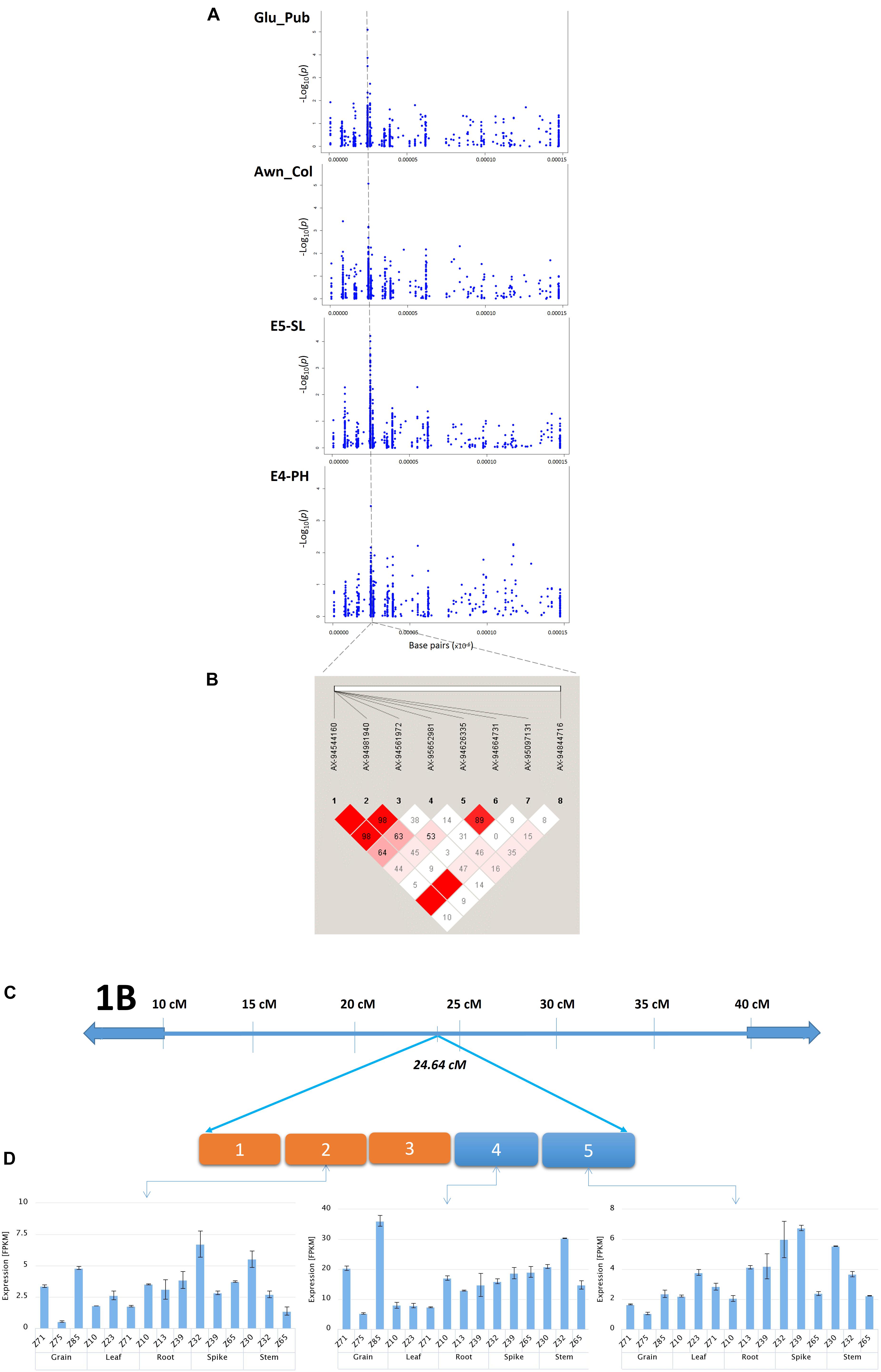
Figure 5. (A) Genomic region (24.64 cM) associated with Glu_Pub, Awn_Col, SL, and PH on chromosome 1B as identified by GWAS. (B) Pairwise LD estimates in the haplotype block for markers associated with these traits. (C) Graphical representation of a chromosome stretch showing putative candidate genes on a locus 24.64 cM (chromosome 1B). Five candidate genes are represented from 1 to 5 [(1) Basic region/leucine zipper protein, (2) replication protein A subunit, (3) carboxypeptidase D, (4) metal tolerance protein, (5) tetratricopeptide repeat protein SKI3]. (D) Tissue and stage specific expression profile of candidate genes 2, 4, and 5 (Wheat-Exp database).
Discussion
The diversity panel selected in this study has high GD (0.363) and PIC (0.29) indicating higher polymorphism than listed in the previous reports (Liu J. et al., 2017; Eltaher et al., 2018). Further, the B genome had higher GD and PIC followed by A and D genome, consistent with the previous report (Ain et al., 2015). The highest LD decay rate of 5 cM for D genome obtained in this study employing 14,160 SNP markers was found in congruence with 90K SNP (Sukumaran et al., 2014) and 9K (Lopes et al., 2013) marker data. The results from the three clustering methods (Structure, PCA, and NJ tree analysis) showed the presence of three subpopulations in this study consistent with the geographic origins and pedigrees of the selected panel. Thus the marker density, diversity and sample size of this study is sufficiently powered to capture allelic variations for the selected traits. Ma et al. (2013) reported that various imputation methods could be used to impute the data from low density to high density, i.e., from 3K to 54K, and subsequently from 54K to 777K. Therefore the data generated with 35K breeders array can be imputed to high density using 820K information.
In this study, a GWAS panel was characterized for 36 agro-morphological traits identified 146 MTAs (-log10 P ≥ 4) for 23 traits. For majority of the heritable traits, at high significant level single locus has been identified indicating that they are controlled by small number of loci, for instance PGH (1B), LS_wax (6D, 3A), Ped_Wax (3A), Sh_Wid (2D), Ear_Col (1B), Ear_Sh (7B), Awn_Col (1B), Awn_Att (5A), Brush_L (3A), Germ_Wid (4A), and Grn_Ph (2A). For coleoptile color, the genes that regulate anthocyanin biosynthesis pathway, have been cloned and mapped on homoeologous groups 3 and 6 (phenylalanine ammonia-lyase), homoeologous groups 1 and 2 (chalcone synthase), homoeologous group 5 (chalcone-flavanone isomerase) (Li et al., 1999). The presence of the dominant allele at the Rc-1 homeologous loci responsible for anthocyanin pigmentation in coleoptile was correlated with F3H (flavanone 3-hydroxylase) gene on chromosome 2A (Khlestkina et al., 2008). However, in this study, besides chromosome 2A, we also detected the loci for coleoptile color on chromosome 6B, 5B, 4B, and 6A. In agreement to this, Sutka (1977) identified the gene designated Rc4 for coleoptile color on chromosome 6B, however, it was not further confirmed in any study (Khlestkina et al., 2002; McIntosh et al., 2014) while suppressors playing role in the intensity of the coleoptile coloration were identified on chromosomes 2A, 2B, 2D, 4B, and 6A of “Mironovskaya 808” bread wheat variety. Hence the loci identified herein further confirmed the role of chromosome 6B for coleoptile color. For Awn_Col and Ear_Col, we identified loci on chromosome 1B but at different positions, i.e., 24.64 and 8.24 cM, respectively. Earlier, Zeven (1983) also reported a semi-dominant gene (Rg) on chromosome 1B responsible for the brown ear character of bread wheat. For Aur_Col, contrasting to the region reported on chromosome 4A and 5B (Zeven, 1985), we detected its locus on chromosomes 4B and 5A owing to the instability of its expression.
Epicuticular wax is associated with increased drought tolerance in wheat (Bennett and Schnurbusch, 2016), rice (Haque et al., 1992), maize (Meeks et al., 2012), barley (Febrero et al., 1998), and many other crops. Herein, we report an additional locus for LS_Wax on chromosome 6D besides previously identified genomic region on chromosome 3A for waxiness. Interestingly, the SNP AX-94527988 (chromosome 3A) was found associated with both LS_wax and Ped_wax thereby indicating its pleiotropic behavior. In fact, this result indicated that some casual gene(s) might exist in this genomic region for wax, as the common MTA AX-94527988 annotated cytochrome P450 protein which leads to a double-hydroxylation to the corresponding oxo-2-alkanol esters which are also previously detected for both peduncle and flag leaf waxes (Racovita et al., 2016).
Glume pubescence appears to have a beneficial influence on drought/cold tolerance (Borner et al., 2005). In this study, we identified significant MTA (1.47 e-07) on chromosome 1A which is in agreement with Sears (1953) who mapped a gene (Hg) responsible for Glu_Pub on chromosomes 1A. In addition to this, we detected genomic regions associated with Glu_Pub on chromosomes 1B (24.64 cM) and 2B (76.24, 76.38, and 104.59 cM) which might be considered as novel region controlling the trait. MTA AX-95023665 linked to Glu_Pub encoded fatty acid biosynthetic process. Glas et al. (2012) observed that methyl ketones which are produced during fatty acid biosynthesis were the major constituent of type VI trichomes of the wild tomato Solanum habrochaites f. glabratum and are very effective in protecting the plant from pests. Pubescent plants also produced a higher number of grains per spikelet compared to non-pubescent plants (Maes et al., 2001).
Several MTAs detected for awn presence were found distributed across the wheat chromosomes except for 2D, 3D, 4D, 5D, 6A, and 7D. However, we could not locate any awn development dominant inhibitor genes Hd, B1, B2 fine mapped on chromosome 4AS, 5A, and 6BL in hexaploid wheat (Sourdille et al., 2002; Yoshioka et al., 2017) which may be due to skewness for the awned genotypes in the diversity panel. For awn attitude, one QTL located on chromosome 5A (59.99–70.36 cM) was identified. To the best of our knowledge, none of the previous studies have reported a genomic region for Awn_Att, suggesting this could be a responsible locus for the trait. The MTA AX-94613491 encoded hexose carrier protein HEX 6 which is responsible for controlling the flux of carbon and plays a role in the carbohydrate transport and distribution in plant cells13.
A gene for high PPO activity responsible for grain color was mapped on the long arm of chromosome 2A in wheat mapping population (Simeone et al., 2002). Similarly, in the current study, 9 SNPs on chromosome 2A spanning 0.71 cM region (124.18–124.89 cM) were found significantly associated with phenol color indicating the importance of this region. These SNPs explained 15.1–18.6% phenotypic variation. Out of 9 SNPs associated with phenol color, 6 SNPs encoded 5 proteins (Table 3). The transcript profile of nucleoredoxin 1-2 (NRX1) gene encoded by SNP AX-94738314 associated with phenol color showed highest expression (FPKM-272.53) in grain at Zadok 72 stage (early milk). Protective effect of NRX1 boosted the H2O2 detoxification capacity of catalase, thereby protecting the plant cell from oxidative stress (Kneeshaw et al., 2017). Significant SNPs associated with grain texture (phenotype scored as hard or soft), encoded four proteins (Table 3). Most of the proteins identified in this study for grain traits were similar to proteins reported by Arora et al. (2017) for grain related traits.
The genetic architecture of the quantitative traits is complex as controlled by many loci with small effect. Several significant markers mined for six complex traits in this study were co-localized with the previously reported QTL regions (Table 2). Flowering is controlled by a complex network of genes integrating vernalization response genes (Vrn) on chromosomes 5A (Vrn-A1and Vrn-A2) and 7BS (Vrn-A3), photoperiod response gene on chromosome 2, and earliness genes on chromosomes 1A and 3A (Fowler and Laudencia-chingcuanco, 2016). The MTAs for DTH were detected on three chromosomes 4A, 5A, and 7D. MTA AX-95187165 (89.02 cM) identified for DTH on chromosome 5A was mapped at nearly same position of the functional gene Vrn-A1 (90 cM) indicating its role for determining DTH (Sukumaran et al., 2014). Another putative candidate, AX-94542441 (chromosome 4A) associated with DTH is an ortholog of shikimate kinase-like protein. Likewise in rice (Oryza sativa) two shikimate kinase isoforms OsSK1 and OsSK3 accumulate to high levels during the heading stage of panicle development and involved in floral organ development (Kasai et al., 2005). The most significant association for DTH was found on chromosome 7D stable in four environments (E1–E4). On similar lines, Lopes et al. (2013) also reported a significant QTL for DTH on chromosome 7D, associated for more than 30% of DTH variation but at different position.
The SNPs significantly associated with DTM were identified on chromosomes 3B, 5A, 5B, and 6A corresponding to the earlier reported genomic regions for DTM on chromosome 3B (Sukumaran et al., 2014, 2018; Okechukwu, 2017), 5A (Gahlaut et al., 2017), 5B (Gahlaut et al., 2017; Zou et al., 2017), and 6A (Sukumaran et al., 2014). The significant QTL for DTM harboring three SNPs were observed on chromosome 3B (61.38, 84.9, and 85.27 cM) were closely co-localized with the previously identified MTAs for DTM, indicating that these QTLs were stable and could be detected in different environments. Another noteworthy region, associated with DTM was identified on chromosome 2A (179.61 cM) consistent in three environments encoding BTB/POZ domain and ankyrin repeat-containing protein which plays key role in plant growth and development stages (Sharma and Pandey, 2016). Moreover, chromosome 2A associated with DTM also encompasses a region (83.23 cM) governing multiple traits (PH and SL) thereby representing the correlation of these traits in diverse panel in agreement with the previous reports (Kamani et al., 2017; Zhai et al., 2016).
For PH, as many as six MTAs were identified on chromosome 1B, 2A, 2B, 5D (2), and 7A. The genomic region of MTA (AX-94941145) identified at 29.9 cM on chromosome 7A falls in the region of the reduced height gene Rht22 (Xgwm471-29.5 cM, Xgwm350-20.1 cM) (Peng et al., 2011). Similarly, the MTA identified for PH on chromosome 2B (104.59 cM) and 5D (1.58 cM) found in proximity to the reduced height genes Rht4 (Xwmc317-106 cM) (Ellis et al., 2005) and Rht23 (Xgdm63-4.7 cM, Xbarc110-11.1 cM) (Chen et al., 2015). We also observed an MTA on chromosome 2A where Rht7 gene had already been reported but at different map position. For two correlated traits PH and DTM (Mohibullah et al., 2012), a significant MTA (AX-94656878) was detected on chromosome 2A annotated bZIP transcription factor. In plants, these factors regulate genes in response to abiotic stress, seed maturation, flower development and pathogen defense (Jakoby et al., 2002). Similarly, several studies reported a moderate, but significant correlation between heading time and PH (Sultana et al., 2002; Mohibullah et al., 2012). The MTA AX-94941145 identified on chromosome 7A annotated probable LRR receptor-like serine/threonine-protein kinase At3g47570. Further, we investigated the possibilities of semi dwarfing genes on chromosome 4B and 4D which is present in Indian cultivars (Sheoran et al., 2013) but could not detect any MTA linked to these genes (Rht-B1b and Rht-D1b) suggesting either these genes were eliminated during filtering or may not reach significant threshold level. Similarly, Ain et al. (2015) did not find any MTA for semi dwarfing genes on chromosome 4B and 4D employing iSelect 90K SNP chip. Several MTAs for PH have been reported previously on chromosomes 2A (Ain et al., 2015; Mengistu et al., 2016), 2B (Zanke et al., 2014; Ain et al., 2015; Gao et al., 2015) and 7A (Gao et al., 2015;Soriano et al., 2017).
Wheat domestication genes Q, compactum (C), sphaerococcum (S1) related to spike morphology have been detected on chromosomes 5A, 2D, and 3D, respectively (Johnson et al., 2008). In the present study, eight loci were detected on chromosomes 1B, 2A, 3A, 3B, 3D, 5A, 7A, and 7B for SL which were partially consistent with those of Zhou et al. (2017), who reported QTLs for SL on chromosome 3A, 3B, 5A and with those of Ma et al. (2007), who reported genomic regions for SL on chromosome 1B and 7A. These results indicated that multiple loci having unequal effects can influence the variations in the SL. It is interesting to note that PH shared common significant loci (83.23 cM) with SL showing a high correlation between these traits in concurrence with the previous results (Sukumaran et al., 2014). Besides MTAs on chromosome 1B (discussed earlier), another SL associated SNP AX-94722223 (chromosome 5A) harboring actin related protein (ARPC3) which has been known to play a key regulator of cytoskeleton dynamics controlling multiple developmental processes in a variety of tissues and cell types (Qi et al., 2017). We expected genes contributing to variation in SL to be most strongly expressed within the different growth stages of spike. In fact, 5 putative candidate genes identified for SL showed expression FPKM >5 in spike tissue depicting highest expression (FPKM 143.65) at growth stage 65 (Zadok’s Scale), corroborating its causal effect.
Awns were reported superior to the flag leaves on a cellular and physiological level during the grain filling period contributing 40–80% of the photosynthetic assimilates accumulated in the wheat grain (Li et al., 2006). Most significant MTAs for Awn_L were reported on several chromosomes viz., 1A, 1B, 2B, 2D, 3B, 4A, 5B, 6D, and 7B (Wu et al., 2016; Liu Y. et al., 2018). However, some of the chromosomal regions associated with Awn_L for instance 1.72 cM (7B) and 85.27 cM (3B) were detected for both Awn_L and SL. This study further corroborated the result of Echeverry-Solarte et al. (2015), who reported a novel QTL for Awn_L on chromosome 7B harboring two consistent loci associated to supernumerary spikelet (SS) and putative QTLs for PH, DTH, DTM making it an important loci for future studies. In the present investigation, cell elongation protein DIMINUTO predicted for Awn_L associated SNP locus (AX-95025537 and AX-95012310) on chromosome 7B (Supplementary Table S8) have been implicated in regulating cell elongation (Takahashi et al., 1995). The genomic regions that contributed to Leaf_L were found associated with chromosomes 4A and 5A with phenotypic variations ranging from 15.5 to 15.7%. In earlier reports several chromosomal regions viz., 2B, 3A, 4A, 4B, and 5A were detected for flag Leaf_L (Jia et al., 2013; Wu et al., 2016). Positive and significant correlation between flag Leaf_L and SL revealed their role in increasing yield (Wu et al., 2016).
Mining of superior/favorable alleles is essential for improving the complicated earliness trait in wheat using marker assisted selection. In recent years, association mapping has been widely used in exploring the elite alleles of many agronomic traits such as yield related traits (Sun et al., 2017), heading days and PH (Ain et al., 2015; Ogbonnaya et al., 2017) and water soluble carbohydrates (Dong et al., 2016) in wheat. In the present study, the phenotypic effect value of the favorable alleles of DTH, PH, and SL was evaluated and inferred to have positive effect on the respective traits. The candidate genes and the SNPs linked with the economic important traits identified in this study could help in designing new strategies to hoard superior alleles for these key traits in future marker based breeding. Some novel regions identified in the present investigation could have been previously detected, but comparison of the positions of the SNPs linked to the respective traits was not possible due to the limitations of the various marker system used in different studies.
This study identified 146 MTAs for 23 agro-morphological traits (Supplementary Table S9), and putative candidate genes using the recently released genome sequence by IWGSC RefSeq v1.0 (Appels et al., 2018). MTAs specific to less explored traits such as awn length and glume pubescence were targeted for visualizing future needs of breeders in developing efficient and resilient wheat varieties. The chromosomal region controlling multiple traits were also identified which should pave the way for selection and may prove effective for pyramiding favorable alleles. Here we discovered novel candidate genomic regions together with previously reported genes which require further validation and testing in the wheat germplasm. Therefore, the significant MTAs identified having known candidate genes are being subjected to conversion as Kompetitive Allele Specific PCR (KASP) markers that can be efficiently used to transfer alleles into elite wheat genotypes (Rasheed et al., 2016). These useful genomic resources and PCR based markers (KASP markers) could be utilized for introgression of traits through marker assisted selection (MAS). These will strongly enhance systematic study of the genetics, comparative genomics and evolution of wheat, and will expedite isolation and characterization of genes controlling agronomically important traits, such as yield and abiotic stress.
Author Contributions
RT and DiK conceived the theme of the study. SS, DeK, NR, RuS, SusP, SJ, MI, AJ, NK, UA, and SurP did the computational analysis. SS, SJ, MI, RaS, PS, RT, and DiK drafted the manuscript. DeK, NR, RuS, CS, and AG did the phenotyping. NR and DeK contributed in wet lab work. SJ, MI, DiK, AR, GS, and RT edited the manuscript. All authors read and approved the final manuscript.
Funding
This work was supported by Indian Council of Agricultural Research; Ministry of Agriculture and Farmer’s welfare, Government of India by providing financial assistance in the form of CABin grant (F. No. Agril. Edn.4-1/2013-A&P) to ICAR-IASRI and ICAR-IIWBR.
Conflict of Interest Statement
The authors declare that the research was conducted in the absence of any commercial or financial relationships that could be construed as a potential conflict of interest.
Acknowledgments
The authors are thankful to Indian Council of Agricultural Research, Ministry of Agriculture and Farmer’s welfare, Government of India for Advanced Supercomputing Hub for Omics Knowledge in Agriculture (ASHOKA) facility at ICAR-IASRI, New Delhi, India created under National Agriculture Innovation Project, funded by World Bank.
Supplementary Material
The Supplementary Material for this article can be found online at: https://www.frontiersin.org/articles/10.3389/fpls.2019.00527/full#supplementary-material
Footnotes
- ^www.fao.org/faostat/en
- ^www.wheatatlas.org/varieties
- ^http://www.upov.int/edocs/tgdocs/en/tg003.pdf
- ^http://wheatatlas.org/megaenvironments
- ^http://plantauthority.gov.in/pdf/PVJFebruary2011.pdf
- ^http://www.sas.com
- ^http://www.cerealsdb.uk.net/cerealgenomics/CerealsDB/Excel/35K_array/Supplementary_file_3.xlsx
- ^http://zzz.bwh.harvard.edu/plink/
- ^http://www.wheatgenome.org/
- ^http://plants.ensembl.org/Triticum_aestivum
- ^https://wheat.pw.usda.gov/WheatExp/
- ^http://pngu.mgh.harvard.edu/purcell/plink
- ^https://patents.justia.com/patent/6624343
References
Ain, Q., Rasheed, A., Anwar, A., Mahmood, T., Imtiaz, M., Mahmood, T., et al. (2015). Genome-wide association for grain yield under rainfed conditions in historical wheat cultivars from Pakistan. Front. Plant Sci. 6:743. doi: 10.3389/fpls.2015.00743
Aklilu, B. B., and Culligan, K. M. (2016). Molecular evolution and functional diversification of replication protein A1 in plants. Front. Plant Sci. 7:33. doi: 10.3389/fpls.2016.00033
Allen, A. M., Winfield, M. O., Burridge, A. J., Downie, R. C., Benbow, H. R., Barker, G. L. A., et al. (2017). Characterization of a wheat breeders’ array suitable for high-throughput SNP genotyping of global accessions of hexaploid bread wheat (Triticum aestivum). Plant Biotechnol. J. 15, 390–401. doi: 10.1111/pbi.12635
Appels, R., Eversole, K., Feuillet, C., Keller, B., Rogers, J., Stein, N., et al. (2018). Shifting the limits in wheat research and breeding using a fully annotated reference genome. Science (80-) 361:eaar7191. doi: 10.1126/science.aar7191
Arends, D., Prins, P., Jansen, R. C., and Broman, K. W. (2010). R/qtl: high throughput multiple QTL mapping. Bioinformatics 26, 2990–2992. doi: 10.1093/bioinformatics/btq565
Arora, S., Singh, N., Kaur, S., Bains, N. S., Uauy, C., Poland, J., et al. (2017). Genome-wide association study of grain architecture in wild wheat Aegilops tauschii. Front. Plant Sci. 8:886. doi: 10.3389/fpls.2017.00886
Arruda, M. P., Brown, P. J., Lipka, A. E., Krill, A. M., Thurber, C., and Kolb, F. L. (2015). Genomic selection for predicting head blight resistance in a wheat breeding program. Plant Genome 8, 1–12. doi: 10.3835/plantgenome2015.01.0003
Barrett, J. C., Fry, B., Maller, J., and Daly, M. J. (2005). Haploview: analysis and visualization of LD and haplotype maps. Bioinformatics 21, 263–265. doi: 10.1093/bioinformatics/bth457
Bennett, D., and Schnurbusch, T. (2016). Genetic dissection of grain yield and physical grain quality in bread wheat (Triticum aestivum L.) under water-limited environments author â€TM s personal copy. Theor. Appl. Genet. 125, 255–271. doi: 10.1007/s00122-012-1831-9
Borner, A., Schafer, M., Schmidt, A., Grau, M., and Vorwald, J. (2005). Associations between geographical origin and morphological characters in bread wheat (Triticum aestivum L.). Plant Genet. Resour. Charact. Util. 3, 360–372. doi: 10.1079/PGR200589
Borrill, P., Adamski, N., and Uauy, C. (2015). Genomics as the key to unlocking the polyploid potential of wheat. New Phytol. 208, 1008–1022. doi: 10.1111/nph.13533
Brenchley, R., Spannagl, M., Pfeifer, M., Barker, G. L. A., D’Amore, R., Allen, A. M., et al. (2012). Analysis of the allohexaploid bread wheat genome using whole genome shotgun sequencing. Nature 491, 705–710.
Broman, K. W., Wu, H., and Churchill, G. A. (2003). R / qtl?: QTL mapping in experimental crosses. Bioinformatics 19, 889–890. doi: 10.1093/bioinformatics/btg112
Cavanagh, C. R., Chao, S., Wang, S., Huang, B. E., Stephen, S., Kiani, S., et al. (2013). Genome-wide comparative diversity uncovers multiple targets of selection for improvement in hexaploid wheat landraces and cultivars. Proc. Natl. Acad. Sci. U.S.A. 110, 8057–8062. doi: 10.1073/pnas.1217133110
Chapman, J. A., Mascher, M., Buluç, A., Barry, K., Georganas, E., Session, A., et al. (2015). A whole-genome shotgun approach for assembling and anchoring the hexaploid bread wheat genome. Genome Biol. 16:26. doi: 10.1186/s13059-015-0582-8
Chen, S., Gao, R., Wang, H., Wen, M., Xiao, J., Bian, N., et al. (2015). Characterization of a novel reduced height gene (Rht23) regulating panicle morphology and plant architecture in bread wheat. Euphytica 203, 583–594. doi: 10.1007/s10681-014-1275-1
Choulet, F., Alberti, A., Theil, S., Glover, N., Barbe, V., Daron, J., et al. (2014). Structural and functional partitioning of bread wheat chromosome 3B. Science (80-) 345, 1249721. doi: 10.1126/science.1249721
Chu, C. G., Xu, S. S., Friesen, T. L., and Faris, J. D. (2008). Whole genome mapping in a wheat doubled haploid population using SSRs and TRAPs and the identification of QTL for agronomic traits. Mol. Breed. 22, 251–266. doi: 10.1007/s11032-008-9171-9
Conesa, A., and Götz, S. (2008). Blast2GO: a comprehensive suite for functional analysis in plant genomics. Int. J. Plant Genomics 2008:619832.
Cui, F., Zhang, N., Fan, X. L., Zhang, W., Zhao, C. H., Yang, L. J., et al. (2017). Utilization of a Wheat660K SNP array-derived high-density genetic map for high-resolution mapping of a major QTL for kernel number. Sci. Rep. 7:3788. doi: 10.1038/s41598-017-04028-6
Dong, Y., Liu, J., Zhang, Y., Geng, H., Rasheed, A., Xiao, Y., et al. (2016). Genome-wide association of stem water soluble carbohydrates in bread wheat. PLoS One 11:e0164293. doi: 10.1371/journal.pone.0164293
Dreisigacker, S., Tiwari R., and Sheoran, S. (2013). Laboratory Manual: ICAR-CIMMYT Molecular Breeding Course in Wheat. Available at: http://repository.cimmyt.org/xmlui/handle/10883/3221# (accessed April 1, 2016).
Earl, D., and VonHoldt, B. (2011). STRUCTURE HARVESTER: a websiteand a program for vizualizing STRUCTURE output nad implementing the Evanno method. Conserv. Genet. Resour. 4, 359–361. doi: 10.1007/s12686-011-9548-7
Echeverry-Solarte, M., Kumar, A., Kianian, S., Mantovani, E. E., McClean, P. E., Deckard, E. L., et al. (2015). Genome-wide mapping of spike-related and agronomic traits in a common wheat population derived from a supernumerary spikelet parent and an elite parent. Plant Genome 8. doi: 10.3835/plantgenome2014.12.0089
Ellis, M. H., Rebetzke, G. J., Azanza, F., Richards, R. A., and Spielmeyer, W. (2005). Molecular mapping of gibberellin-responsive dwarfing genes in bread wheat. Theor. Appl. Genet. 111, 423–430. doi: 10.1007/s00122-005-2008-6
Eltaher, S., Sallam, A., Belamkar, V., Emara, H. A., Nower, A. A., Salem, K. F. M., et al. (2018). Genetic diversity and population structure of F(3:6) nebraska winter wheat genotypes using genotyping-by-sequencing. Front. Genet. 9:76. doi: 10.3389/fgene.2018.00076
Evanno, G., Regnaut, S., and Goudet, J. (2005). Detecting the number of clusters of individuals using the software STRUCTURE: a simulation study. Mol. Ecol. 14, 2611–2620. doi: 10.1111/j.1365-294x.2005.02553.x
Febrero, A., Fernández, S., Molina-Cano, J. L., and Araus, J. L. (1998). Yield, carbon isotope discrimination, canopy reflectance and cuticular conductance of barley isolines of differing glaucousness. J. Exp. Bot. 49, 1575–1581. doi: 10.1093/jxb/49.326.1575
Fowler, D. B., and Laudencia-chingcuanco, D. (2016). Quantitative trait loci associated with phenological development, low-temperature tolerance, grain quality, and agronomic characters in wheat (Triticum aestivum L.) quantitative trait loci associated with phenological development, low-temperature To. PLoS One 11:e0152185. doi: 10.1371/journal.pone.0152185
Gahlaut, V., Jaiswal, V., Tyagi, B. S., Singh, G., Sareen, S., Balyan, H. S., et al. (2017). QTL mapping for nine drought-responsive agronomic traits in bread wheat under irrigated and rain-fed environments. PLoS One 12:e0182857. doi: 10.1371/journal.pone.0182857
Gao, F., Wen, W., Liu, J., Rasheed, A., Yin, G., Xia, X., et al. (2015). Genome-Wide linkage mapping of QTL for yield components, plant height and yield-related physiological traits in the chinese wheat cross zhou 8425B/chinese spring. Front. Plant Sci. 6:1099. doi: 10.3389/fpls.2015.01099
Glas, J. J., Schimmel, B. C. J., Alba, J. M., Escobar-Bravo, R., Schuurink, R. C., and Kant, M. R. (2012). Plant glandular trichomes as targets for breeding or engineering of resistance to herbivores. Int. J. Mol. Sci. 13, 17077–17103. doi: 10.3390/ijms131217077
Gupta, A., Singh, C., Kumar, V., Tyagi, B. S., Tiwari, V., Chatrath, R., et al. (2018). Wheat Varieties Identified in India Since 1965. Karnal: ICAR-IIWBR.
Haque, M. M., Mackill, D. J., and Ingram, K. T. (1992). Inheritance of leaf epicuticular wax content in rice. Crop Sci. 32:865. doi: 10.2135/cropsci1992.0011183X003200040006x
Hong, M. J., Kim, D. Y., Kang, S. Y., Kim, D. S., Kim, J. B., and Seo, Y. W. (2012). Wheat F-box protein recruits proteins and regulates their abundance during wheat spike development. Mol. Biol. Rep. 39, 9681–9696. doi: 10.1007/s11033-012-1833-3
Hubisz, M. J., Falush, D., Stephens, M., and Pritchard, J. K. (2009). Inferring weak population structure with the assistance of sample group information. Mol. Ecol. Resour. 9, 1322–1332. doi: 10.1111/j.1755-0998.2009.02591.x
International Wheat Genome Sequencing Consortium (IWGSC) (2014). A chromosome-based draft sequence of the hexaploid bread wheat (Triticum aestivum) genome. Science, 345:1251788. doi: 10.1126/science.1251788
Jaiswal, V., Gahlaut, V., Meher, P. K., Mir, R. R., Jaiswal, J. P., Rao, A. R., et al. (2016). Genome wide single locus single trait, multi-locus and multi-trait association mapping for some important agronomic traits in common wheat (T. aestivum L.). PLoS One 11:e0159343. doi: 10.1371/journal.pone.0159343
Jakoby, M., Weisshaar, B., Vicente-carbajosa, J., Tiedemann, J., and Kroj, T. (2002). bZIP transcription factors in Arabidopsis. Trends Plant Sci. 7, 106–111. doi: 10.1016/s1360-1385(01)02223-3
Jia, J. Z., Zhao, S. C., Kong, X. Y., Li, Y. R., Zhao, G. Y., He, W. M., et al. (2013). Aegilops tauschii draft genome sequence reveals a gene repertoire for wheat adaptation. Nature 496, 91–95. doi: 10.1038/nature12028
Johnson, E. B., Nalam, V. J., Zemetra, R. S., and Riera-Lizarazu, O. (2008). Mapping the compactum locus in wheat (Triticum aestivum L.) and its relationship to other spike morphology genes of the Triticeae. Euphytica 163, 193–201. doi: 10.1007/s10681-007-9628-7
Joshi, A. K., Ortiz-Ferrara, G., Crossa, J., Singh, G., Sharma, R. C., Chand, R., et al. (2007). Combining superior agronomic performance and terminal heat tolerance with resistance to spot blotch (Bipolaris sorokiniana) of wheat in the warm humid gangetic plains of South Asia. F. Crop. Res. 103, 53–61. doi: 10.1016/j.fcr.2007.04.010
Kamani, D. L., Babariya, C. A., and Marviya, P. B. (2017). Correlation coefficient and path coefficient analysis for yield components in wheat (Triticum aestivum L.). Int. J. Pure Appl. Biosci. 5, 545–552. doi: 10.18782/2320-7051.2686
Kasai, K., Kanno, T., Akita, M., Ikejiri-Kanno, Y., Wakasa, K., and Tozawa, Y. (2005). Identification of three shikimate kinase genes in rice: characterization of their differential expression during panicle development and of the enzymatic activities of the encoded proteins. Planta 222, 438–447. doi: 10.1007/s00425-005-1559-8
Khlestkina, E. K., Pestsova, E. G., Röder, M. S., and Börner, A. (2002). Molecular mapping, phenotypic expression and geographical distribution of genes determining anthocyanin pigmentation of coleoptiles in wheat (Triticum aestivum L.). Theor. Appl. Genet. 104, 632–637. doi: 10.1007/s00122-001-0788-x
Khlestkina, E. K., Röder, M. S., and Salina, E. A. (2008). Relationship between homoeologous regulatory and structural genes in allopolyploid genome – A case study in bread wheat. BMC Plant Biol. 8:88. doi: 10.1186/1471-2229-8-88
Kneeshaw, S., Keyani, R., Delorme-Hinoux, V., Imrie, L., Loake, G. J., Le Bihan, T., et al. (2017). Nucleoredoxin guards against oxidative stress by protecting antioxidant enzymes. Proc. Natl. Acad. Sci. U.S.A. 114, 8414–8419. doi: 10.1073/pnas.1703344114
Kundu, S., Singh, C., Shoran, J., and Singh, S. S. (2010). An Update on Released Wheat Varieties and Registered Genetic Stock (Triticum L.). Karnal: Directorate of Wheat Research.
Li, W. L., Faris, J. D., Chittoor, J. M., Leach, J. E., Hulbert, S. H., Liu, D. J., et al. (1999). Genomic mapping of defense response genes in wheat. Theor. Appl. Genet. 98, 226–233. doi: 10.1007/s001220051062
Li, X., Wang, H., Li, H., Zhang, L., Teng, N., Lin, Q., et al. (2006). Awns play a dominant role in carbohydrate production during the grain-filling stages in wheat (Triticum aestivum). Physiol. Plant. 127, 701–709. doi: 10.1111/j.1399-3054.2006.00679.x
Li, Y., Fan, C., Xing, Y., Jiang, Y., Luo, L., Sun, L., et al. (2011). Natural variation in GS5 plays an important role in regulating grain size and yield in rice-S1. Nat. Genet. 43, 1266–1269. doi: 10.1038/ng.977
Lin, M., Zhang, D., Liu, S., Zhang, G., Yu, J., Fritz, A. K., et al. (2016). Genome-wide association analysis on pre-harvest sprouting resistance and grain color in US winter wheat. BMC Genomics 17:794. doi: 10.1186/s12864-016-3148-6
Lipka, A. E., Tian, F., Wang, Q., Peiffer, J., Li, M., Bradbury, P. J., et al. (2012). GAPIT: genome association and prediction integrated tool. Bioinformatics 28, 2397–2399. doi: 10.1093/bioinformatics/bts444
Liu, J., He, Z., Rasheed, A., Wen, W., Yan, J., Zhang, P., et al. (2017). Genome-wide association mapping of black point reaction in common wheat (Triticum aestivum L.). BMC Plant Biol. 17:220. doi: 10.1186/s12870-017-1167-3
Liu, Y., Lin, Y., Gao, S., Li, Z., Ma, J., Deng, M., et al. (2017). A genome-wide association study of 23 agronomic traits in Chinese wheat landraces. Plant J. 91, 861–873. doi: 10.1111/tpj.13614
Liu, K., and Muse, S. V. (2005). PowerMarker: an integrated analysis environment for genetic marker analysis. Bioinformatics 21, 2128–2129. doi: 10.1093/bioinformatics/bti282
Liu, K., Xu, H., Liu, G., Guan, P., Zhou, X., Peng, H., et al. (2018). QTL mapping of flag leaf-related traits in wheat (Triticum aestivum L.). Theor. Appl. Genet. 131, 839–849. doi: 10.1007/s00122-017-3040-z
Liu, Y., Tao, Y., Wang, Z., and Guo, Q. (2018). Identification of QTL for flag leaf length in common wheat and their pleiotropic effects. 38:11. doi: 10.1007/s11032-017-0766-x
Liu, W., Gowda, M., Reif, J. C., Hahn, V., Ruckelshausen, A., Weissmann, E. A., et al. (2014). Genetic dynamics underlying phenotypic development of biomass yield in triticale. BMC Genomics 15:458. doi: 10.1186/1471-2164-15-458
Lopes, M. S., Reynolds, M. P., McIntyre, C. L., Mathews, K. L., Jalal Kamali, M. R., Mossad, M., et al. (2013). QTL for yield and associated traits in the seri/babax population grown across several environments in Mexico, in the West Asia, North Africa, and South Asia regions. Theor. Appl. Genet. 126, 971–984. doi: 10.1007/s00122-012-2030-4
Ma, P., Brøndum, R. F., Zhang, Q., Lund, M. S., and Su, G. (2013). Comparison of different methods for imputing genome-wide marker genotypes in Swedish and Finnish Red Cattle. J. Dairy Sci. 96, 4666–4677. doi: 10.3168/jds.2012-6316
Ma, Z., Zhao, D., Zhang, C., Zhang, Z., Xue, S., Lin, F., et al. (2007). Molecular genetic analysis of five spike-related traits in wheat using RIL and immortalized F2populations. Mol. Genet. Genomics 277, 31–42. doi: 10.1007/s00438-006-0166-0
Maccaferri, M., Ricci, A., Salvi, S., Milner, S. G., Noli, E., Martelli, P. L., et al. (2015). A high-density, SNP-based consensus map of tetraploid wheat as a bridge to integrate durum and bread wheat genomics and breeding. Plant Biotechnol. J. 13, 648–663. doi: 10.1111/pbi.12288
Maes, B., Trethowan, R. M., Reynolds, M. P., Van Ginkel, M., and Skovmand, B. (2001). “Glume pubescence and its influence on spikelet temperature of wheat under freezing conditions BT – Wheat in a global environment,” in Proceedings of the 6th International Wheat Conference, 5–9 June 2000, Budapest, Hungary, eds Z. Bedö and L. Láng (Dordrecht: Springer), 463–470. doi: 10.1007/978-94-017-3674-9_61
McIntosh, R., Dubcovsky, J., Rogers, W., Morris, C., Appels, R., Xia, X., et al. (2014). Catalogue of Gene Symbols for Wheat: 2013-2014 Supplement. Avaiable at: http://www.shigen.nig.ac.jp/wheat/komugi/genes/macgene/supplement doi: 10.1007/978-94-017-3674-9_61 (accessed, December, 21 2017).
Meeks, M., Murray, S. C., Hague, S., Hays, D., and Ibrahim, A. M. H. (2012). Genetic variation for maize epicuticular wax response to drought stress at flowering. J. Agron. Crop Sci. 198, 161–172. doi: 10.1111/j.1439-037X.2011.00495.x
Mengistu, D. K., Kidane, Y. G., Catellani, M., Frascaroli, E., Fadda, C., Pè, M. E., et al. (2016). High-density molecular characterization and association mapping in Ethiopian durum wheat landraces reveals high diversity and potential for wheat breeding. Plant Biotechnol. J. 14, 1800–1812. doi: 10.1111/pbi.12538
Mohibullah, M., Rabbani, M. A., Irfanullah, Iqbal, M., Zakiullah, and Khattak, M. I. (2012). Correlation and frequency distribution for different parameters among bread wheat (Triticum aestivum L.) Accessions. Pak. J. Bot. 44, 255–259.
Ogbonnaya, F. C., Rasheed, A., Okechukwu, E. C., Jighly, A., Makdis, F., Wuletaw, T., et al. (2017). Genome-wide association study for agronomic and physiological traits in spring wheat evaluated in a range of heat prone environments. Theor. Appl. Genet. 130, 1819–1835. doi: 10.1007/s00122-017-2927-z
Okechukwu, E. C. (2017). Genome Wide Association of Heat Tolerance Loci of Wheat in Hotspots of Sudan And Syria. Nsukka: Univeristy Of Nigeria. . doi: 10.1007/s00122-017-2927-z
Pearce, S., Vazquez-Gross, H., Herin, S. Y., Hane, D., Wang, Y., Gu, Y. Q., et al. (2015). WheatExp: an RNA-seq expression database for polyploid wheat. BMC Plant Biol. 15:299. doi: 10.1186/s12870-015-0692-1
Peng, Z. S., Li, X., Yang, Z. J., and Liao, M. L. (2011). A new reduced height gene found in the tetraploid semi-dwarf wheat landrace Aiganfanmai. Genet. Mol. Res. 10, 2349–2357. doi: 10.4238/2011.October.5.5
Perrier, X., and Jacquemoud-Collet, J. (2006). DARwin Software. Available at: . doi: 10.4238/2011.october.5.5 (accessed November 25, 2016).
Purcell, S., Neale, B., Todd-Brown, K., Thomas, L., Ferreira, M. A. R., Bender, D., et al. (2007). PLINK: a tool set for whole-genome association and population-based linkage analyses. Am. J. Hum. Genet. 81, 559–575. doi: 10.1086/519795
Qi, T., Wang, J., Sun, Q., Day, B., Guo, J., and Ma, Q. (2017). TaARPC3, contributes to wheat resistance against the stripe rust fungus. Front. Plant Sci. 8:1245. doi: 10.3389/fpls.2017.01245
R Development Core Team (2013). R: A Language and Environment for Statistical Computing. Available at: http://www.R-project.org (accessed March 20, 2018).
Racovita, R. C., Hen-Avivi, S., Fernandez-Moreno, J. P., Granell, A., Aharoni, A., and Jetter, R. (2016). Composition of cuticular waxes coating flag leaf blades and peduncles of Triticum aestivum cv. Bethlehem. Phytochemistry 130, 182–192. doi: 10.1016/j.phytochem.2016.05.003
Rasheed, A., Hao, Y., Xia, X., Khan, A., Xu, Y., Varshney, R. K., et al. (2017). Crop breeding chips and genotyping platforms: progress, challenges, and perspectives. Mol. Plant 10, 1047–1064. doi: 10.1016/j.molp.2017.06.008
Rasheed, A., Wen, W., Gao, F., Zhai, S., Jin, H., Liu, J., et al. (2016). Development and validation of KASP assays for genes underpinning key economic traits in bread wheat. Theor. Appl. Genet. 129, 1843–1860. doi: 10.1007/s00122-016-2743-x
Rebetzke, G. J., Bonnett, D. G., and Reynolds, M. P. (2016). Awns reduce grain number to increase grain size and harvestable yield in irrigated and rainfed spring wheat. J. Exp. Bot. 67, 2573–2586. doi: 10.1093/jxb/erw081
Rimbert, H., Darrier, B., Navarro, J., Kitt, J., Choulet, F., Leveugle, M., et al. (2018). High throughput SNP discovery and genotyping in hexaploid wheat. PLoS One 13:e0186329. doi: 10.1371/journal.pone.0186329
Sajjad, M., Ma, X., Khan, S. H., Shoaib, M., Song, Y., Yang, W., et al. (2017). TaFlo2-A1, an ortholog of rice Flo2, is associated with thousand grain weight in bread wheat (Triticum aestivum L.). BMC Plant Biol. 17:164. doi: 10.1186/s12870-017-1114-3
Sharma, D., Singh, R., Rane, J., Gupta, V. K., Mamrutha, H. M., and Tiwari, R. (2016). Mapping quantitative trait loci associated with grain filling duration and grain number under terminal heat stress in bread wheat (Triticum aestivum L.). Plant Breed. 135, 538–545. doi: 10.1111/pbr.12405
Sharma, M., and Pandey, G. K. (2016). Expansion and function of repeat domain proteins during stress and development in plants. Front. Plant Sci. 6:1218. doi: 10.3389/fpls.2015.01218
Sheoran, S., Singh, V., Malik, R., Kundu, S., Tiwari, R., Kumar, R., et al. (2013). Distribution of dwarfing genes Rht-B1b and Rht-D1b in Indian wheat (Triticum aestivum) cultivars detected by functional markers. Ind. J. Agric. Sci. 83, 820–825.
Simeone, R., Pasqualone, A., Clodoveo, M. L., and Blanco, A. (2002). Genetic mapping of polyphenol oxidase in tetraploid wheat. Cell. Mol. Biol. Lett. 7, 763–769.
Sofalian, O., Mohammadi, S. A., Aharizad, S., Moghaddam, M., and Shakiba, M. R. (2008). Mapping of QTLs for frost tolerance and heading time using SSR markers in bread wheat. Afr. J. Biotechnol. 9, 5260–5264.
Soriano, J. M., Malosetti, M., Roselló, M., Sorrells, M. E., and Royo, C. (2017). Dissecting the old Mediterranean durum wheat genetic architecture for phenology, biomass and yield formation by association mapping and QTL meta-analysis. PLoS One 12:e0178290. doi: 10.1371/journal.pone.0178290
Sourdille, P., Cadalen, T., Gay, G., Gill, B., and Bernard, M. (2002). Molecular and physical mapping of genes affecting awning in wheat. Plant Breed. 121, 320–324. doi: 10.1046/j.1439-0523.2002.728336.x
Sukumaran, S., Dreisigacker, S., Lopes, M., Chavez, P., and Reynolds, M. P. (2014). Genome-wide association study for grain yield and related traits in an elite spring wheat population grown in temperate irrigated environments. Theor. Appl. Genet. 128, 353–363. doi: 10.1007/s00122-014-2435-3
Sukumaran, S., Reynolds, M. P., and Sansaloni, C. (2018). Genome-Wide association analyses identify QTL hotspots for yield and component traits in durum wheat grown under yield potential, drought, and heat stress environments. Front. Plant Sci. 9:81. doi: 10.3389/fpls.2018.00081
Sultana, S., Islam, M. A., Islam, M. R., Morshed, M. M., and Islam, M. R. (2002). Correlation and regression analysis for heading date, yield and yield contributing characters in wheat under water and phosphorus stress. Pak. J. Biol. Sci. 5, 149–151. doi: 10.3923/pjbs.2002.149.151
Sun, C., Zhang, F., Yan, X., Zhang, X., Dong, Z., Cui, D., et al. (2017). Genome-wide association study for 13 agronomic traits reveals distribution of superior alleles in bread wheat from the Yellow and Huai Valley of China. Plant Biotechnol. J. 15, 953–969. doi: 10.1111/pbi.12690
Sutka, J. (1977). The association of genes for purple coleoptile with chromosomes of the wheat variety Mironovskaya 808. Euphytica 26, 475–479. doi: 10.1007/BF00027010
Takahashi, T., Gasch, A., Nishizawa, N., and Chua, N.-H. (1995). The DIMINUTO gene of Arabidopsis is involved in regulating cell elongation. Genes Dev. 9, 97–107. doi: 10.1101/gad.9.1.97
Tewolde, H., Fernandez, C. J., and Erickson, C. A. (2006). Crop/stress physiology wheat cultivars adapted to post-heading high temperature stress. Crop Sci. 120, 111–120. doi: 10.1111/j.1439-037x.2006.00189.x
Turuspekov, Y., Baibulatova, A., Yermekbayev, K., Tokhetova, L., Chudinov, V., Sereda, G., et al. (2017). GWAS for plant growth stages and yield components in spring wheat (Triticum aestivum L.) harvested in three regions of Kazakhstan. BMC Plant Biol. 17:190. doi: 10.1186/s12870-017-1131-2
VanRaden, P. M. (2008). Efficient methods to compute genomic predictions. J. Dairy Sci. 91, 4414–4423. doi: 10.3168/jds.2007-0980
Vatansever, R., Filiz, E., and Eroglu, S. (2017). Genome-wide exploration of metal tolerance protein (MTP) genes in common wheat (Triticum aestivum): insights into metal homeostasis and biofortification. BioMetals 30, 217–235. doi: 10.1007/s10534-017-9997-x
Wang, S. (2014). Characterization of polyploid wheat genomic diversity using a high-density 90,000 SNP array. Plant Biotechnol. J. 12, 787–796. doi: 10.1111/pbi.12183
Wickham, M., Faulks, R., and Mills, C. (2009). In vitro digestion methods for assessing the effect of food structure on allergen breakdown. Mol. Nutr. Food Res. 53, 952–958. doi: 10.1002/mnfr.200800193
Wilkinson, P. A., Winfield, M. O., Barker, G. L. A., Allen, A. M., Burridge, A., Coghill, J. A., et al. (2012). CerealsDB 2.0: an integrated resource for plant breeders and scientists. BMC Bioinformatics 13:219. doi: 10.1186/1471-2105-13-219
Winfield, M. O., Allen, A. M., Burridge, A. J., Barker, G. L. A., Benbow, H. R., Wilkinson, P. A., et al. (2016). High-density SNP genotyping array for hexaploid wheat and its secondary and tertiary gene pool. Plant Biotechnol. J. 14, 1195–1206. doi: 10.1111/pbi.12485
Winfield, M. O., Allen, A. M., Wilkinson, P. A., Burridge, A. J., Barker, G. L. A., Coghill, J., et al. (2018). High-density genotyping of the A.E. Watkins collection of hexaploid landraces identifies a large molecular diversity compared to elite bread wheat. Plant Biotechnol. J. 16, 165–175. doi: 10.1111/pbi.12757
Worland, A. J. (1996). The influence of flowering time genes on environmental adaptability in European wheats. Euphytica 89, 49–57. doi: 10.1007/BF00015718
Wu, Q., Chen, Y., Fu, L., Zhou, S., Chen, J., Zhao, X., et al. (2016). QTL mapping of flag leaf traits in common wheat using an integrated high-density SSR and SNP genetic linkage map. Euphytica 208, 337–351. doi: 10.1007/s10681-015-1603-0
Yin, W., Cui, P., Wei, W., Lin, Y., and Luo, C. (2017). Genome-wide identification and analysis of the basic leucine zipper (bZIP) transcription factor gene family in Ustilaginoidea virens. Genome 60, 1051–1059. doi: 10.1139/gen-2017-0089
Yoshioka, M., Iehisa, J. C. M., Ohno, R., Kimura, T., Enoki, H., Nishimura, S., et al. (2017). Three dominant awnless genes in common wheat: fine mapping, interaction and contribution to diversity in awn shape and length. PLoS One 12:e0176148. doi: 10.1371/journal.pone.0176148
Zanke, C. D., Ling, J., Plieske, J., Kollers, S., Ebmeyer, E., Korzun, V., et al. (2014). Whole genome association mapping of plant height in winter wheat (Triticum aestivum L). PLoS One 9:e113287. doi: 10.1371/journal.pone.0113287
Zeven, A. C. (1983). The character brown ear of bread wheat: a review. 32, 299–310. doi: 10.1007/bf00021438
Zeven, A. C. (1985). The genetics of auricle colour of wheat (Triticum aestivum L.): a review. Euphytica 34, 233–236. doi: 10.1007/BF00022914
Zhai, H., Feng, Z., Li, J., Liu, X., Xiao, S., Ni, Z., et al. (2016). QTL analysis of spike morphological traits and plant height in winter wheat (Triticum aestivum L.) using a high-density SNP and SSR-based linkage map. Front. Plant Sci. 7:1617. doi: 10.3389/fpls.2016.01617
Zhang, K., Wang, J., Zhang, L., Rong, C., Zhao, F., Peng, T., et al. (2013). Association analysis of genomic loci important for grain weight control in elite common wheat varieties cultivated with variable water and fertiliser supply. PLoS One 8:e57853. doi: 10.1371/journal.pone.0057853
Zhao, B., Wei, X., Li, W., Udan, R. S., Yang, Q., Kim, J., et al. (2007). Inactivation of YAP oncoprotein by the Hippo pathway is involved in cell contact inhibition and tissue growth control. Genes Dev. 21, 2747–2761. doi: 10.1101/gad.1602907
Zhou, Y., Conway, B., Miller, D., Marshall, D., Cooper, A., Murphy, P., et al. (2017). Quantitative trait loci mapping for spike characteristics in hexaploid wheat. Plant Genome 10. doi: 10.3835/plantgenome2016.10.0101
Keywords: 35K Axiom array, agro-morphological, GWAS, SNP, wheat
Citation: Sheoran S, Jaiswal S, Kumar D, Raghav N, Sharma R, Pawar S, Paul S, Iquebal MA, Jaiswar A, Sharma P, Singh R, Singh CP, Gupta A, Kumar N, Angadi UB, Rai A, Singh GP, Kumar D and Tiwari R (2019) Uncovering Genomic Regions Associated With 36 Agro-Morphological Traits in Indian Spring Wheat Using GWAS. Front. Plant Sci. 10:527. doi: 10.3389/fpls.2019.00527
Received: 20 December 2018; Accepted: 04 April 2019;
Published: 25 April 2019.
Edited by:
Dragan Perovic, Julius Kühn-Institut, GermanyReviewed by:
Marion S. Röder, Leibniz-Institut für Pflanzengenetik und Kulturpflanzenforschung (IPK), GermanyAwais Rasheed, International Maize and Wheat Improvement Center, Mexico
Šurlan-Momirović, University of Belgrade, Serbia
Copyright © 2019 Sheoran, Jaiswal, Kumar, Raghav, Sharma, Pawar, Paul, Iquebal, Jaiswar, Sharma, Singh, Singh, Gupta, Kumar, Angadi, Rai, Singh, Kumar and Tiwari. This is an open-access article distributed under the terms of the Creative Commons Attribution License (CC BY). The use, distribution or reproduction in other forums is permitted, provided the original author(s) and the copyright owner(s) are credited and that the original publication in this journal is cited, in accordance with accepted academic practice. No use, distribution or reproduction is permitted which does not comply with these terms.
*Correspondence: Dinesh Kumar, ZGluZXNoLmt1bWFyQGljYXIuZ292Lmlu Ratan Tiwari, cmF0YW4udGl3YXJpQGljYXIuZ292Lmlu
†These authors have contributed equally to this work