- 1Department of Maize Breeding and Genetics, Agricultural Institute Osijek, Osijek, Croatia
- 2Centre of Excellence for Biodiversity and Molecular Plant Breeding, Zagreb, Croatia
Chlorophyll fluorescence (ChlF) parameters are reliable early stress indicators in crops, but their relations with yield are still not clear. The aims of this study are to examine genetic correlations between photosynthetic performance of JIP-test during flowering and grain yield (GY) in maize grown under two heat scenarios in the field environments applying quantitative genetic analysis, and to compare efficiencies of indirect selection for GY through ChlF parameters and genomic selection for GY. The testcrosses of 221 intermated recombinant inbred lines (IRILs) of the IBMSyn4 population were evaluated in six environments at two geographically distinctive locations in 3 years. According to day/night temperatures and vapor pressure deficit (VPD), the two locations in Croatia and Turkey may be categorized to the mild heat and moderate heat scenarios, respectively. Mild heat scenario is characterized by daytime temperatures often exceeding 33°C and night temperatures lower than 20°C while in moderate heat scenario the daytime temperatures often exceeded 33°C and night temperatures were above 20°C. The most discernible differences among the scenarios were obtained for efficiency of electron transport beyond quinone A (QA) [ET/(TR-ET)], performance index on absorption basis (PIABS) and GY. Under the moderate heat scenario, there were tight positive genetic correlations between ET/(TR-ET) and GY (0.73), as well as between PIABS and GY (0.59). Associations between the traits were noticeably weaker under the mild heat scenario. Analysis of quantitative trait loci (QTL) revealed several common QTLs for photosynthetic and yield performance under the moderate heat scenario corroborating pleiotropy. Although the indirect selection with ChlF parameters is less efficient than direct selection, ET/(TR-ET) and PIABS could be efficient secondary breeding traits for selection under moderate heat stress since they seem to be genetically correlated with GY in the stressed environments and not associated with yield performance under non-stressed conditions predicting GY during flowering. Indirect selection through PIABS was also shown to be more efficient than genomic selection in moderate heat scenario.
Introduction
Crop response to different stresses affected by weather anomalies including climate change is highly complex. It involves changes at the genetic and physiological levels that facilitate avoiding and/or coping with stress. The strategy of avoiding is commonly applied in agronomy where stress can be circumvented by agricultural practice (Jung and Müller, 2009; Teixeira et al., 2013). On the other hand, modern plant breeding aims to conduct studies on trait physiology, phenotyping, and genotyping on how to cope with stress (Araus et al., 2012; Fahad et al., 2017). Drought is one of the major stressors limiting the crop production in rainfed areas, and the likelihood of observing the anomalies in seasonal precipitation is increasing worldwide (IPCC, 2014). However, Lobell et al. (2013) demonstrated that extreme heat as a stressor had more critical role for maize production than drought in the United States, corroborating previous statistical studies of rainfed maize yields showing a strong negative yield response to accumulation of extreme temperatures (>30°C) and relative weak response to seasonal rainfall. In the future, up to 10 million tons of maize may be lost in the developing world each year as temperatures increase and precipitation patterns change due to the anthropogenic emission of greenhouse gasses. This yield loss could eventually affect 140 million people.
At the physiological level, the impact of heat on the photosynthetic apparatus is considered to be of particular significance since photosynthesis is the most sensitive of all plant cell processes to high temperatures (Sharkey and Schrader, 2006). It is often inhibited before other cell functions are impaired causing changes in the reduction-oxidation properties of PSII acceptors and reduce the efficiency of photosynthetic electron transport in both Photosystem I and II (Mathur et al., 2014). The effects of heat and other stresses are manifested in the behavior of the fluorescence transients (Rohacek and Bartak, 1999; Sayed, 2003) by reducing both the ratio of reduced primary acceptors to reaction center (RC) and the ratio of reduced secondary to primary acceptors. The ChlF induction transients (O-J-I-P) can be translated via JIP-test into several phenomenological and biophysical parameters (Strasser et al., 1995, 2000, 2004) that quantify PSII functioning and can reflect the activity of the whole photosynthetic machinery (Strasser et al., 2004). One of the most often employed ChlF parameters is Fv/Fm, which gives the information about the proportion of the light absorbed by chlorophyll in the PSII that is used in photochemical processes. However, the intensity of Fv/Fm is determined only by the changes in minimum (F0) and maximum (Fm) fluorescence and has been proved to be insensitive for assessing mildly stressed plants (e.g., Van Heerden et al., 2004; Oukarroum et al., 2007). The most powerful and most comprehensive parameters are the performance indexes (PIABS and PITotal) taking into the account all of the main photochemical processes. PIABS appeared to be very suitable and sensitive parameter to investigate plant overall photosynthetic performance in moderately stressed environments (Živčák et al., 2008; Šimić et al., 2014). The chlorophyll a fluorescence parameters are being used extensively in stress physiology in a range of plant species under controlled conditions and it is also adaptable to field conditions (Šimić et al., 2014). This is particularly important for crop improvement, because stress studies conducted under controlled conditions inadequately reflect natural environmental conditions. Additionally, chlorophyll a fluorescence measurement is non-destructive and fast. It generates considerable amount of data belonging to high-throughput phenotyping methods.
The relations between ChlF parameters and grain yield (GY) in the field environments are not elucidated. Kalaji et al. (2017) stressed that in quite a few studies ChlF parameters were considered as selection tools in plant breeding. They emphasized the importance of obtaining ChlF-related traits showing a high correlation with yield or plant performance in addition to ChlF-related traits that are specific for tolerance/resistance to the stress of interest. We assume that the use of certain ChlF parameters of JIP-test as secondary traits for selection under moderate heat stress would be an efficient breeding strategy if ChlF parameters would be genetically variable, genetically correlated with grain yield in the target environment, and not associated with any yield loss under non-stressed conditions. Ribaut et al. (2009) had similar expectations by reviewing secondary breeding traits under drought conditions. Furthermore, any secondary trait or trait related allele can help confer stress tolerance given the precise design of the right scenario as it helps to define the breeding strategies in the modeling process (Tardieu, 2011). Millet et al. (2016) showed the allelic effects vary with temperature scenarios, the night temperatures playing the major role. Variation of adaptive traits or combination of those traits in a set of specific environmental scenarios along with its shared variability and high heritability is crucial for adaptive trait to be useful in breeding for stress tolerance (Tardieu et al., 2018).
The objectives of this study were to examine genetic correlations between photosynthetic performance of JIP-test during flowering and GY in maize grown under two heat scenarios in the field environments by applying quantitative genetic analysis and to provide the genetic information about the usability and scalability of the ChlF data in maize breeding for heat stress tolerance. Additionally, we aimed to compare the efficiencies of indirect selection for GY through ChlF parameters and genomic selection for GY.
Materials and Methods
Plant Materials and Field Experiments
The maize IBMSyn4 population (Lee et al., 2002) was chosen according to the results presented by Šimić et al. (2014) for photosynthetic parameters in the population per se The 221 IBMSyn4 IRILs were included in the experiment (see the list in Supplementary Tables S1 and S2). From the cross between the IBMSyn4 IRILs and an Iodent elite inbred, property of the Agricultural Institute Osijek, 221 testcross genotypes were generated and used in this investigation.
The testcrosses were evaluated in six environments at two locations in Croatia and Turkey during three consecutive years 2014, 2015, and 2016. Each year-location combination was considered as an environment. Locations were Osijek, Croatia, 45°32′20.6″N 18°44′21.1″E (OS), and Altinova near Ayvalik, Turkey, 39°11′43″N 26°46′34″E (AY). Experimental locations OS and AY were found to match the heat scenarios defined by Millet et al. (2016). Thus, trials in location OS fell in category of Hot(day) scenario, while the environments in location AY were categorized as the Hot scenario. Characteristics of the Hot(day) scenario are hot daytime temperatures that often exceed 33°C, and night temperatures lower than 20°C. Hot scenario is characterized by the daytime temperatures that normally exceed 33°C and night (low) temperatures are above 20°C (Table 1). For brevity, we renamed the scenarios Hot and Hot(day) to mild heat and moderate heat scenarios, respectively, indicating different stress levels. Weather data for both locations was collected from the webpages of The Weather Underground (The Weather Channel Interactive, Atlanta, GA, United States) for the closest available meteorological stations to the experimental locations (18 km for AY in Ayvalik, and 9 km for OS). VPD of the air was applied to confirm the heat scenario classification. VPD was calculated following the guidelines from Allen et al. (1998) from temperature and air humidity data. Field trials consisting of the 221 IRIL testcrosses of the IBM population along with three checks were planted in randomized single row plots in two replications with 20 plants per row (plot size 7 m2) according to alpha-lattice design (Patterson and Williams, 1976). The experiments in all environments were planted at the end of April and harvested in the first decade of October. Usual local crop management practice for high-yielding maize was applied according to local rain-fed (OS) and watered (AY) regimes taking into account the soil characteristics and the previous cropping.
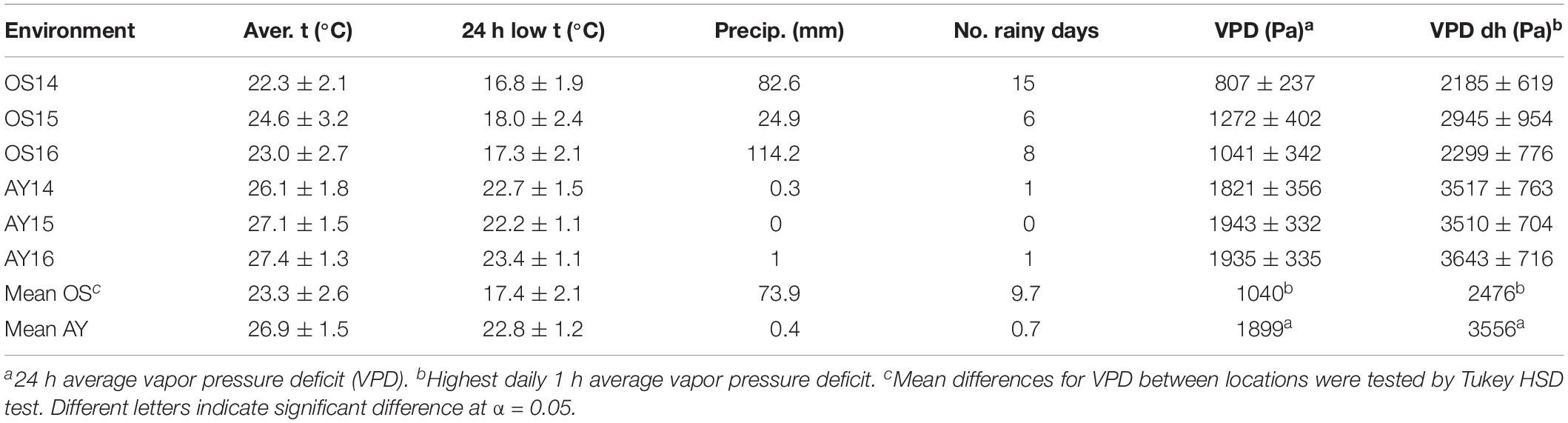
Table 1. Weather data for six environments during July in 3 years (2014–2016) in two locations Osijek, Croatia (OS) and Ayvalik, Turkey (AY).
Chlorophyll a Fluorescence Measurements
Chlorophyll a fluorescence (ChlF) was measured by Plant Efficiency Analyzer Handy-PEA (Hansatech, United Kingdom). All the measurements occurred between 6.30 am and 9.30 am after 30 min of sample dark adaptation. Measurements were performed on attached ear leaves of four tasseling plants in the middle of each plot. We chose ear leaf as it was found that it best represents total canopy chlorophyll content (Ciganda et al., 2009). Briefly, all plant samples exhibited polyphasic ChlF rise after the dark adaptation in the first second upon illumination with high-intensity light (3500 μmol m–2 s–1) (Strasser et al., 2000, 2004). The application of the saturating light pulse (red light, wavelength peak at 650 nm) induces chlorophyll a fluorescence increase from minimal fluorescence (F0, O step) when all reaction centers (RC) are open, to maximal fluorescence (Fm, P step) when all RC are closed. During the first 2 ms, changes were recorded every 10 ms, and every 1 ms thereafter. Data obtained were used to calculate several biophysical parameters that describe the photochemistry of PSII according to Strasser et al. (2004). Calculated parameters used in quantitative genetic analysis were: – RC/ABS; – ET/(TR-ET); – performance index (potential) for energy conservation from photons absorbed by PSII to the reduction of intersystem electron acceptors.
Quantitative Genetic Analysis
In the statistical analysis of individual trials, genotypic variance of the 221 IRIL testcrosses and checks, as well as block, replication and error variances for the three JIP-test traits and grain yield were calculated by analysis of variance (ANOVA). Generally, there were no significant effects of replication and block. This suggests that no considerable changes occurred in weather conditions during the measurement time span (6.30–9.30 am). In the combined ANOVA, effects of environment, genotype, genotype × environment interaction, and error were estimated on adjusted entry mean values from the individual trial analyses. Heritability on a genotype (entry) mean basis (Hallauer et al., 2010) were estimated as , where is the variance component due to genotype (IRIL testcrosses), is the variance component due to genotype × environment interaction and s2e the pooled error variance, whereas r is the number of replications per environment (2) and e is the number of environments (8). The genetic correlations were firstly estimated from genetic covariances via multivariate analysis of variance (MANOVA – Anderson, 1958; Mode and Robinson, 1959). Analysis of variance was performed, and genetic correlation coefficients according to MANOVA were calculated using PLABSTAT program, Version 3A (Utz, 2005). Additionally, the genetic correlations between genome-wide marker effects among the traits within a scenario and the genetic correlations between the means of the two scenarios were approximated using the method outlined by Ziyomo and Bernardo (2013). Briefly, the ridge regression best linear unbiased predictions (rrBLUP) model (Endelman, 2011) was fitted with the data from two scenarios, and the marker effects were obtained. The genetic correlation between the phenotypes was calculated as Pearson’s product-moment correlation between the marker effects for two phenotypes and tested for significance.
The R/sommer package (Covarrubias-Pazaran, 2016) was used for calculation of BLUPs with genotype as random factor on scenario basis. Genotypic BLUPs for each scenario were used as the input data for QTL mapping. We used a linkage map of the IBMSyn4 population anchored with 2178 molecular markers, mostly SNPs and SSRs (Andorf et al., 2010). Total map length was 7090 cM and the average distance between markers was 3.2 cM (Supplementary Figure S1). As IBM population consists of recombinant inbred lines developed through four successive generations of intermating Syn4 (recombination), centiMorgan of IBM population can be considered IBM centiMorgan, as there was the increase in both map size and density (Lee et al., 2002; Falque et al., 2005). QTL results reported here are in IBM cM (1 IBM cM ∼ 4 cM in F2) on IBM2 Neighbors map. QTL mapping procedure was conducted using inclusive composite interval mapping method (ICIM) in QTL IciMapping software, version 4.1 (Meng et al., 2015). LOD threshold to declare putative QTL significant was calculated on 1000 permutations basis. Calculated LOD thresholds in mild heat scenario were 3.48 for RC/ABS, 3.44 for ET/(TR-ET), 3.58 for PIABS and 3.60 for grain yield. In moderate heat scenario, the thresholds were 3.51 for RC/ABS, 3.73 for ET/(TR-ET), 3.54 for PIABS and 3.68 for grain yield. The largest P-value for entering variables into the stepwise regression was set to p = 0.001. Step size for the analysis was set to 1 cM. Confidence intervals of the detected QTL were determined based on one-LOD unit drop in both forward and backward directions. Since a common QTL for ET/(TR-ET) and grain yield was detected, we performed a joint QTL analysis for better understanding of the relationships between the traits. The maximum LOD score along the interval was taken as the position of the QTL. MCIM likelihood ratio test was used from joint analysis for BLUPs of ET/(TR-ET) and grain yield averaged across the environments, to determine if QTL detected by MCIM had pleiotropic effects. JZmapQTL procedure of the Windows QTL Cartographer software version 2.5 (Wang et al., 2011) was used for detecting pleiotropy. The LOD significance threshold for joint mapping was 4.72 generated by permutation analysis (α = 0.05, experiment wide).
Indirect Selection Efficiency and Genomewide Marker Effects
To test the usability of the ChlF parameters genetically correlated with yield for the indirect selection in testcrosses, the efficiency of indirect selection over direct phenotypic selection was calculated according to Bernardo (2010) as where | is the absolute value of genetic correlation, is heritability of secondary trait, and is the heritability of the trait of interest. Furthermore, we aimed to compare the efficiency of the indirect selection through the ChlF parameters with the efficiency of genomic selection. The genome-wide marker effects were calculated using the rrBLUP method as implemented in the R/rrBLUP mixed model solver with markers as random effects (Endelman, 2011). The rrBLUP model was chosen as it outperforms other models for genomic predictions in case of a large number of small-effect QTLs affecting the trait (Heffner et al., 2009; Kwong et al., 2017). The cross-validation procedure was run with 500 random folds of the 80% of individuals that comprised the training set used to calculate the marker effects. The rest of the set (20% of individuals) was used to obtain the genomic predictions (validation set). The accuracy of genomic predictions () was calculated in each of 500 cycles as correlation between observed and predicted values. The efficiency of the genomic selection was calculated according to Dekkers (2007) and Ziyomo and Bernardo (2013) as . The means of the 500 random folds were tested for significance of the differences from zero by the means of one sample t-test in R.
Results
Weather conditions differed substantially between Osijek, Croatia (OS) and Ayvalik, Turkey (AY) (Table 1). During the three growing seasons in 2014, 2015, 2016, temperatures were consistently and significantly higher in AY than those in OS. In average, temperatures were higher in AY for 3.6°C in July. As expected, the differences in precipitation were even more obvious. VPD values (both 24 h average VPD and highest daily 1 h average VPD) varied considerably between OS and AY, and respective mean VPD values were significantly higher in AY (1899 Pa) than those in OS (1040 Pa) according to the Tukey HSD test.
Means for the three ChlF parameters measured during flowering on ear-leaf as well as GY (t/ha) in testcrosses of 221 IRILs of the IBMSyn4 maize population across the six environments were presented in Supplementary Tables S1, S2. Parameters RC/ABS, ET/(TR-ET) and PIABS as well as GY varied significantly either across the environments or between the two locations (Supplementary Table S3). The most discernable differences among environments and locations were obtained for ET/(TR-ET) (1.91–2.60), PIABS (3.14–5.21) and GY (5.76–12.15 t/ha). Considerably higher mean values were observed in OS environments for ET/(TR-ET) (2.39 in OS, 2.08 in AY) PIABS (4.70 in OS and 3.94 in AY) and grain yield (10.95 in OS and 8.33 in AY). In average, mean values for all ChlF traits and GY were significantly higher in OS than in AY. Variance components for RC/ABS, ET/(TR-ET), PIABS and grain yield were presented in Table 2 showing the greatest variance components due to environment (E), followed by the variance component due to genotype (G) and genotype × environment interaction (GE) whereby the GE interaction was not significant across the traits. Heritability estimates were the highest for PIABS (72.7%) and GY (70.8%).
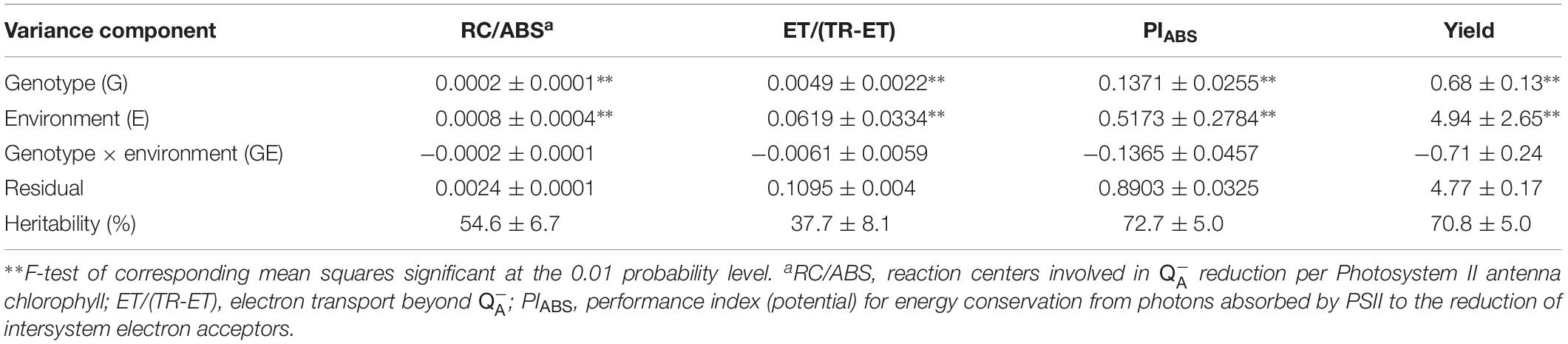
Table 2. Estimated variance components and heritabilities along with their standard errors for three JIP test parameters and grain yield in 221 testcrosses of the IBM IRILs combined over six environments.
As expected, the tightest genetic correlations estimated both from genetic covariances and from genome-wide marker effects were between PIABS and its components RC/ABS and ET/(TR-ET) in both scenarios (Table 3). Generally, the genetic correlations between all traits were considerably stronger under moderate heat scenario. The only exception was the genetic correlation estimated from genetic covariances between ET/(TR-ET) and PIABS. Genetic correlations from genetic covariances in mild heat scenario ranged from −0.32 between ET/(TR-ET) and GY to 0.82 between ET/(TR-ET) and PIABS, while genotypic correlations estimated from genome-wide marker effects ranged from −0.06 between PIABS and GY to 0.65 between ET/(TR-ET) and PIABS. In moderate heat scenario, the correlations estimated from genetic covariances ranged from 0.51 between RC/ABS and GY to 0.92 between RC/ABS and PIABS, while correlations from genome-wide marker effects were detected in range from 0.37 between ET/(TR-ET) and GY to 0.85 between RC/ABS and PIABS. Notably, the direction of genetic correlations between some ChlF parameters and GY, have changed from negative to positive and became stronger and significant under moderate heat scenario. Generally, the correlations estimated from genetic covariances agreed well with the correlations from genome-wide marker effects. The strongest correlations from genome-wide marker effects between scenarios were for PIABS, followed by GY.
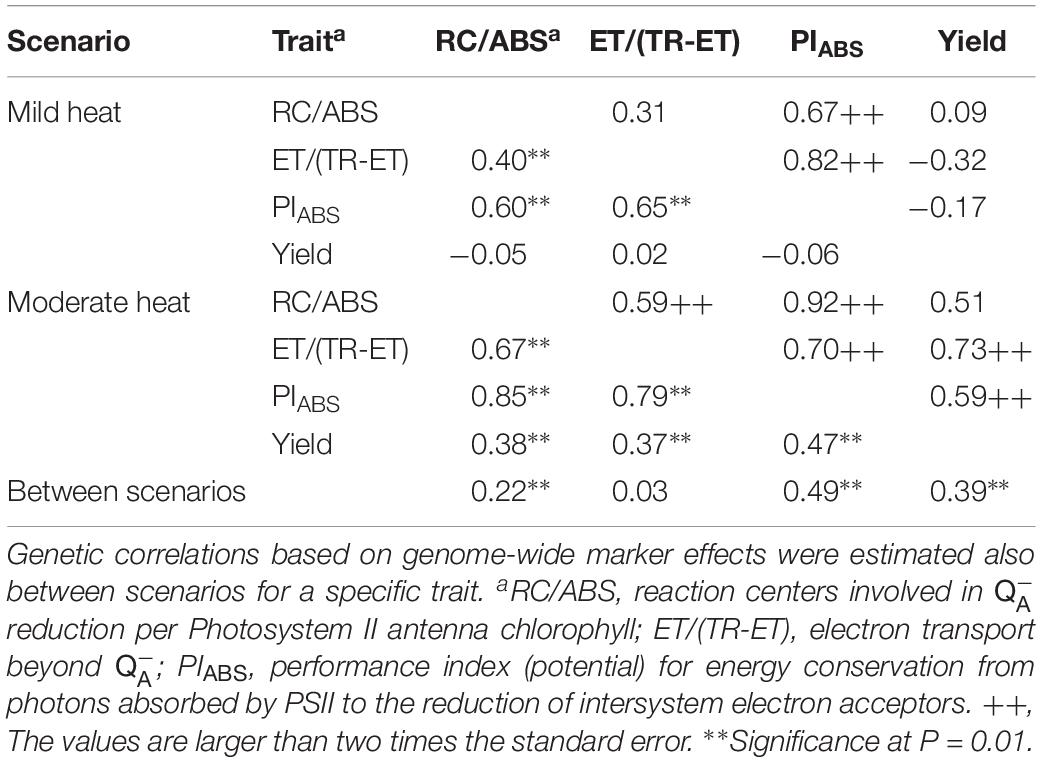
Table 3. Genetic correlations estimated from genetic variances and covariances (above the diagonal) and genetic correlations based on genome-wide marker effects (below the diagonal) for three JIP-test parameters and grain yield in the field experiments classified to two heat scenarios (mild heat and moderate heat) over 3 years in 221 testcrosses of the IBM IRILs.
Inclusive composite interval QTL mapping for four ChlF parameters and grain yield revealed five and six significant QTLs under mild heat and moderate heat scenarios, respectively (Table 4). The highest LOD score was detected for a QTL for RC/ABS under mild heat scenario. There were no common QTLs across the two heat scenarios. However, two pairs of QTLs were collocated under moderate heat scenario: QTLs for ET/(TR-ET) and grain yield on Chromosome 1, and QTLs for RC/ABS and PIABS on Chromosome 10. The QTL for ET/(TR-ET) explained the greatest percentage of phenotypic variation (8.27%). Almost all QTLs (except one for yield) had negative signs of the additive effect means. The direction of allelic effects means that almost all favorable alleles came from IBM population parental line B73.
The joint multi-trait composite interval mapping (MCIM) for ET/(TR-ET) and grain yield revealed other four significant QTLs on Chromosomes 1 and 8 according to MCIM likelihood ratio test (Figure 1). There were totally three peaks on Chromosome 1 and two peaks on Chromosome 8 that exceeded the threshold of LOD = 4.72. The highest peak was on Chromosome 8, position 80.2 reaching LOD score of 6.6.
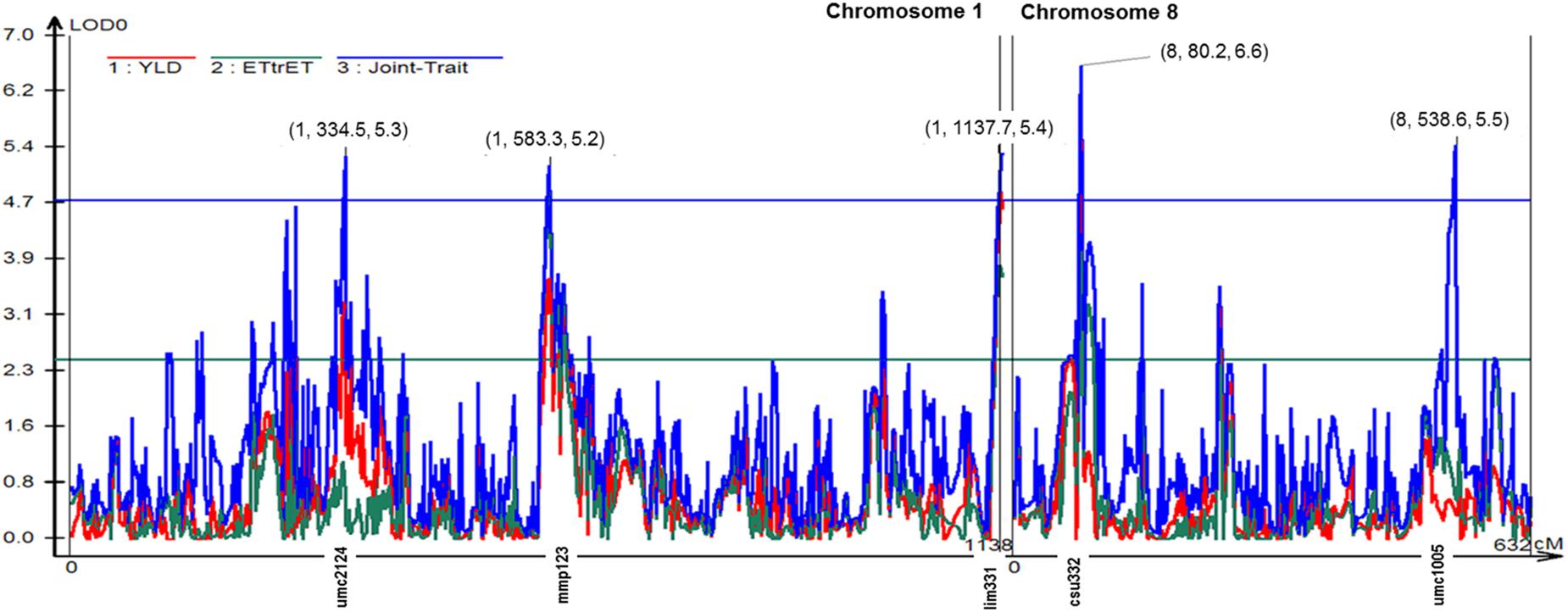
Figure 1. Likelihood odds ratio (LOD0) profile from multitrait composite interval mapping (MCIM) for grain yield (yld) and the chlorophyll fluorescence parameter ET/(TR-ET) (electron transport beyond ) mapped on chromosomes 1 and 8. The solid blue line indicates the MCIM LOD significance threshold of 4.72 for joint mapping generated by permutation analysis (α = 0.05, experiment wide). The numbers above the five peaks indicate (chromosome number, position in cM, the LOD score). Marker name associated with the respective peak was given along the x-axis.
The calculated efficiency of indirect selection for grain yield through ChlF parameters was lower than the efficiency of direct selection in both heat scenarios, ranging from −17.6 to 6.7% in mild heat and 38.7 to 60.9% in moderate heat scenario (Figure 2). The obtained efficiencies of genomic selection were also low at 11.0 and 32.1% of the efficiency of the direct selection for grain yield in mild and moderate heat scenarios, respectively. Remarkably, the highest efficiency of indirect selection was through PIABS in moderate heat scenario.
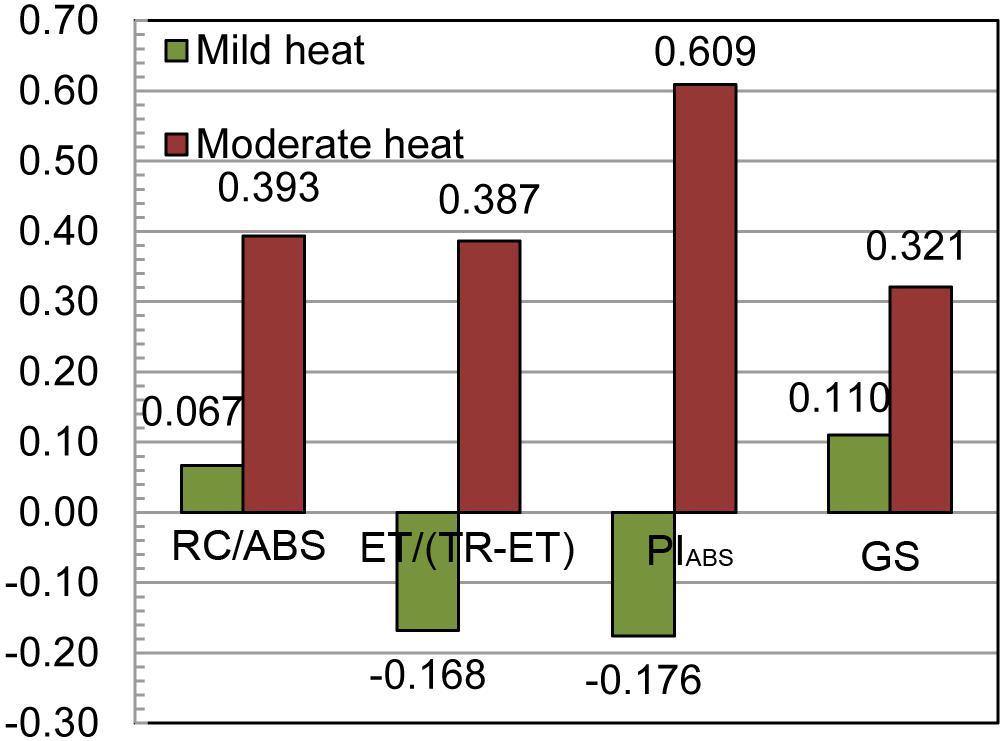
Figure 2. The relative efficiencies of the indirect selection for grain yield through chlorophyll a fluorescence parameters RC/ABS (reaction centers involved in reduction per Photosystem II antenna chlorophyll), ET/(TR-ET) (electron transport beyond ) and PIABS [performance index (potential) for energy conservation from photons absorbed by PSII to the reduction of intersystem electron acceptors] and genomic selection (GS) in two heat scenarios.
Discussion
Assigning individual field experiments to scenarios based on environmental conditions can be used for assessing the performance of genotypes and the contribution of genomic regions (QTLs) under current and future abiotic stress situations (Millet et al., 2016). On the other hand, a thorough understanding of physiological responses of plants to stress is imperative for developing crop plants with improved stress tolerance. In the context of heat stress, bringing together genetic and ecophysiological analyses should accelerate characterization and improvement of crop thermotolerance in breeding programs. VPD as a function of air moisture and temperature defines the difference between saturated air vapor pressure and actual saturation for a given temperature (Allen et al., 1998). Effects of VPD are manifold, but the most prominent is the effect on transpiration rate by inducing the decrease in stomatal conductance (Gholipoor et al., 2013) and increase in evaporative demand. Beside the components of photosystem are sensitive to heat stress, stomatal closure results in limitation of CO2 transfer to the sites of carboxylation in chloroplast stroma, directly limiting the photosynthetic rate (Perdomo et al., 2016). Alterations in various photosynthetic attributes is one of the physiological responses to heat stress (Wahid et al., 2007) where applying ChlF parameters plays important role in quantifying the alterations. The scenarios Hot and Hot(day) classified by Millet et al. (2016) are equivalent to the mild heat and moderate heat scenarios in our study additionally differentiated by different average Fv/Fm values during flowering. Thus, a heat scenario in our study was characterized by different daytime and night temperature, VPD and Fv/Fm during flowering evidenced by significantly higher mean values for all presented quantitative traits under mild heat conditions.
Although ChlF induction transients (O-J-I-P) can be translated via JIP-test into numerous phenomenological and biophysical parameters, just a few ChlF parameters are actual traits suitable for appropriate and worthwhile quantitative genetic analysis. Furthermore, apart from having inadequate statistical properties (Fv/Fm and TR/DI), they may not be satisfactorily genetically variable (RC/ABS) or may not have high heritability (ET/(TR-ET)). On the other hand, PIABS as a multivariate expression is sufficiently genetically variable and it may have higher heritability than GY. Along with positive and tight genetic correlations with GY in stress environments, it indicates that indirect selection for heat tolerance through PIABS is feasible.
Another way to improve the effectiveness of selection for thermotolerance is to select for molecular markers associated with performance traits under heat stress via QTL analysis. Our previous studies on ChlF parameters under moderate drought stress in maize inbred lines B73, Mo17 as well as their IRILs of the IBMSyn4 population demonstrated that they act as an excellent resource for physiological, genetic and genomic studies of photosynthetic alterations (Lepeduš et al., 2012; Šimić et al., 2014). Apart from detecting putative QTLs for thermotolerance, the premise in this study was using the linkage map of the IBMSyn4 population anchored with 2178 molecular markers in order to (i) compare genetic correlations from genetic covariance with genetic correlations from genome-wide marker effects, (ii) confirm genetic correlations through finding some common genes underlying the phenotypic variation in multiple traits, and (iii) compare efficiencies of indirect selection for GY through ChlF parameters and genomic selection for GY. Firstly, genetic correlations from genetic covariance agreed well with genetic correlations from genome-wide marker effects yielding similar results. Equivalent outcome for genetic correlations for drought tolerance in the IBMSyn4 population was reported by Ziyomo and Bernardo (2013).
By detecting putative QTLs, we restricted the choice of candidates to structural genes, which could be directly related to the examined traits in activating stress responsive mechanisms. The data about markers in the marker intervals of detected QTLs and co−localized putative candidate genes with their respective positions are publicly available in IBM 2008 Neighbors Map via Maize Genetics and Genomic Database at http://www.maizegdb.org (Schaeffer et al., 2008). According to this genetic map based on the IBM population, although there are several genes within the QTL intervals, only few or one of them are genes with known or assumed function and may be involved in the process of photosynthesis.
In the mild heat scenario QTL for RC/ABS on chromosome 4 was mapped close to gene cat3 coding for enzyme catalase. Catalase is one of the most important antioxidant enzymes related to maintaining the cell and cellular compartment redox balance. Catalase can also affect the process of photosynthesis (Mhamdi et al., 2012). Within the QTL interval for PIABS on chromosome 2, position 72 cM, gene ereb197 coding for AP2-EREBP-transcription factor is found. AP2/EREBP are known to be involved in regulation of transcription in various abiotic stresses in many plant species (Dietz et al., 2010), and PIABS is a sensitive indicator of many abiotic stress conditions (Oukarroum et al., 2007; Živčák et al., 2008; Stefanov et al., 2011; Lepeduš et al., 2012; Šimić et al., 2014). On chromosome 8, position 490 cM, the position of detected QTL overlapped with the position of the gene psy2 whose product is the enzyme phytoene synthase 2. Phytoene synthase gene family is essential for photosynthesis and photoprotection in plants and can influence the grain yield (Li et al., 2008).
The QTL detected on chromosome 1, position 483 cM for RC/ABS and PIABS in Moderate heat scenario is located in the region where the gene sig2A (sigma factor sig2A) is found. The role of plant sigma factors is the regulation of the expression of plastid genome (Lysenko, 2007) and they appear to be up-regulated by light. Another QTL for both RC/ABS and PIABS in Moderate heat scenario was detected on chromosome 10, position 403 cM. Within the QTL interval, transcription factor Zmsbp28 is found, belonging to the group of SQUAMOSA promoter binding protein-like (SPL) transcription factors (TFs). SPL TFs play a crucial role in maize growth and development but are also involved in plant response on abiotic stresses (Mao et al., 2016). Chao et al. (2017) found that SPL TFs in Arabidopsis can confer tolerance to high temperatures during the reproductive stages of development. Measurements of the ChlF in this current research were performed during the flowering, and the mentioned QTL were detected in moderate heat scenario.
We found that a region on a chromosome 1, position 583 cM holds a pleiotropic loci or two tightly linked genomic regions controlling grain yield and ChlF parameter ET/(TR-ET) also confirmed by MCIM procedure in Windows QTL Cartographer. In the proximity (6 IBM cM, 1.5 cM) from the peak of the QTL, gene zmm6 is found coding for MADS6, a well-known promoter of TPP (trehalose-phosphate-phosphatase) included in regulation of plant carbon budget and sugar signaling in response to the abiotic stresses (Griffiths et al., 2016). MADS6 plays an essential role in endosperm nutrient accumulation in reproductive organs, balancing the source-sink relations in maize. TPP product trehalose-6-phosphate (T6P) is tightly linked to regulation of plant free sucrose levels, and increased levels of T6P might be ensuring optimal gene expression for biosynthetic processes. Nuccio et al. (2015) overexpressed the gene encoding TPP in maize. The overexpression resulted in 31–123% increase in yield in water-stressed environments, and 9–49% in non-drought environments. It was found that overexpression led to reduction of T6P levels, and increase in levels of sucrose in ear spikelets. Increased levels of sucrose might also help maintain photosynthetic processes during stress.
MCIM procedure of Windows QTL Cartographer revealed four more pleiotropic loci for ET/(TR-ET) and Yield on chromosomes 1 and 8 (Figure 1). Pleiotropic QTL on chromosome 1, position 334.5 cM was detected in the genomic region near the gene sod4 coding for cytosolic superoxide dismutase 4 enzyme. Reactive oxygen species (ROS) are known to be generated as a consequence of biotic and abiotic stresses, and can impair photosystem (Gill and Tuteja, 2010). Expression of sod4 might have influenced the traits by alleviation of oxidative stress damage. On the end of chromosome 1, position 1137.7 cM, ZmAKINβγ1 gene is found coding for AKINβγ protein kinase. AKINβγ kinase is localized in cytosol and chloroplasts, and its activation is closely related to stress signaling and starch granule formation (Avila-Castañeda et al., 2014). Starch formation is a key process in maize yield formation and balancing the production of sucrose plays vital role for plants when carbon is sparse. AKINβγ seems to be one of the crucial enzymes in diurnal change and response of plants to daytime osmotic changes (Pokhilko and Ebenhöh, 2015). The QTL on chromosome 8, position 80.2 cM was detected in proximity of two tightly linked genes that might have influenced each individual trait, grain yield and ET/(TR-ET). The gene mem1 coding for MEM1 (Mesophyll Expression Module 1) is a cis-element in regulation of C4 carbonic anhydrase, an essential enzyme in function of maize photosynthetic apparatus. MEM 1 mediates the transport of carbon to photosynthesizing cells and is important in abiotic stress conditions for replenishment of cells with carbon dioxide (Kaul et al., 2011). Other gene found in the proximity of the detected QTL was sod3b coding for antioxidant enzyme superoxide-dismutase 3B. Another QTL detected on chromosome 8, position 538.6 cM was located near the gene cyc4 coding for cyclin-4, regulator of cyclin-dependent kinases involved in active progression of cell cycle (Buendía-Monreal et al., 2011) and regulation of growth in abiotic stress conditions (Aslam et al., 2015). Due to the canonical role of cyclins, and their regulatory role in whole plant growth and development, this gene might have had the effect on both traits. The detected loci were found in the gene rich regions and indicated the pleiotropy that possibly caused the genetic correlations between the ChlF traits and grain yield, although the amount of the genetic variance explained by the detected QTLs was generally low. QTL analysis captures only phenotypic variation of loci crossing the calculated threshold for significance, but many small-effect QTLs underlying the complex traits remain undetected. The approach to calculation of correlations between marker effects outlined by Ziyomo and Bernardo (2013) thus provides true estimate of correlation between the effects of genomic regions affecting the traits. In our study it was confirmed that the directions as well as the sizes of correlations are comparable between the genetic correlations calculated from variance-covariance matrix and rrBLUP marker effects.
Four generations of repeated intermating at the F2 stage of the IBM population have increased the observed numbers of recombinations and feasibly broken a tight linkage. Consequently, a close genetic association between the traits might result due to pleiotropy rather than linkage. Thus, in our study tight genetic correlations as well as colocalized QTLs on chromosome 1 for ET/(TR-ET) and grain yield would indicate pleiotropy. Moreover, MCIM revealed several pleiotropic QTLs on chromosomes 1 and 8. The joint−mapping approach offered the advantage of directly testing whether the two traits affected by a particular QTL and provided greater power to detect pleiotropy. Eventually, fine−scale mapping with additional markers and larger mapping populations is required to distinguish truly pleiotropic loci from tightly linked loci not controlled by the same underlying genes. The same is true for the two complement IBM Syn10 population with ten generations of random mating which were intermated after the F2 (Liu et al., 2015).
The efficiency of indirect over the direct selection for grain yield was lower for all three ChlF traits in both scenarios. The higher detected efficiencies in moderate heat scenario were caused by the increase of the genetic correlations in this scenario, and the highest value of 60.9% of the efficiency of the direct selection with indirect selection through the PIABS was caused by both increased genetic correlation and relatively high heritability, although no significant pleiotropic loci were detected for PIABS and grain yield. As a multiparametric expression calculated from other parameters with low to moderate heritability and genetically correlated with yield, PIABS is expected to have higher heritability, and to be genetically correlated with yield. Despite the relative inefficiency of the indirect over direct selection, indirect selection is often used in selection for abiotic stress tolerance (Bernardo, 2010). Ziyomo and Bernardo (2013) used several drought-related traits for indirect selection in the IBMSyn4 biparental population, and obtained efficiencies ranging from 48% for grain moisture, to 104% for use of ASI as drought tolerance indicator. The authors also found that the performance in non-stressed environments is relatively efficient (78%) in selection for performance in stressed environments.
In this study, the rrBLUP models were set to compare the obtained efficiencies of indirect selection with the efficiencies of genomic selection, as the genomic selection is also a form of indirect selection with = 1, assuming no genotyping errors (Bernardo, 2010). The low efficiency of genomic selection was found in both scenarios although it was 21.1% higher in moderate heat scenario. The low relative efficiencies of genomic selection through environments in this same dataset were reported earlier (Galić, 2018). Low relative efficiencies of genomewide selection and small partition of variance explained by the detected QTLs can be attributed to the masking effects of the tester line. Namely, if the Iodent tester heterotic to both IBM parents is fixed for dominant alleles for grain yield, the masking effect of the segregating loci is present (Mihaljevic et al., 2005; Peng et al., 2013). Massman et al. (2013) also found that within the biparental populations with saturated genetic maps efficiencies of the genomic selection are very low. They attributed the small proportion of variance explained to the probable causes of poorly addressing the genotype × environment variance, as well as the QTL × genetic background interaction. In our study, however, the genotype × environment variances were not significant. Although it was found in this study that genomewide predictions and indirect selection through the ChlF traits both display some predictive ability of grain yield, the aims of the two methods are different but complementary. The main aim of the genomic selection is to predict the performance of the untested progenies, thus saving the time and space for conducting the experiments, allowing for more efficient resource allocation in a breeding program. On the other hand, the main proposed aim of the indirect selection for grain yield through the ChlF parameters is more precise identification of the heat-stress tolerant progenies already included in breeding trials, or as part of the training populations. As the ChlF measurements can be conducted during the whole growing season, additional information may be captured. However, the aim of this study was to test the physiological effects of the heat during pollination when plants are the most sensitive to temperature extremes (Hatfield and Prueger, 2015) and to use this data in indirect selection for grain yield. Heat stress during the flowering time in terms of high night temperatures accompanied with high daytime temperatures was shown to cause the decrease in allelic effects of loci affecting the grain yield in moderate conditions (Millet et al., 2016). Furthermore, there is a completely heat-scenario dependent set of loci affecting the grain yield in environments with high night temperatures. Nevertheless, the genomic selection and ChlF approaches can be considered complementary rather than mutually exclusive, as there is a growing need for new and precise high throughput phenotyping strategies in genomics era (Cobb et al., 2013; Fiorani and Schurr, 2013). Also, there is a need for improvement of the present state of precision of the crop growth models (Cooper et al., 2016).
Our results demonstrated that ChlF via JIP-test is an appropriate method for realizing real-time, non-destructive monitoring of maize performance during flowering under moderate heat stress in the field environments. From an agronomical point of view, it seems that ChlF parameters may be used for predicting grain yield when heat stress occurred during vegetative and reproductive growth stages suggesting possible inclusion of the parameters in crop growth models. From a plant breeding point of view, using ChlF parameters ET/(TR-ET) and PIABS as secondary traits for selection under moderate heat stress could be an efficient breeding strategy for heat tolerance The ChlF parameters prove to be genetically correlated with grain yield in the stressed environments; they are genetically variable and not associated with grain yield under non-stressed conditions. Additionally, a breeding program for heat stress tolerance may be optimized by examining ChlF prior to yield trials in a pre-selected set of progenies harboring the heat-stress tolerance related alleles. Further studies on other breeding materials and linkage/association mapping are necessary to validate the presented QTLs, as well as to detect the additional loci associated with ChlF parameters in different heat scenarios. Specifically, future quantitative genetic studies on more severe (extreme) heat stress scenario would be worthwhile to examine changes in genetic correlations between ChlF parameters and grain yield. Eventually, the converging approaches of crop physiology, modeling, quantitative genetics and genomic prediction promise to considerably advance crop breeding for complex traits including adaptation to stress (Cabrera-Bosquet et al., 2012; Messina et al., 2018).
Author Contributions
DS conceived and designed the experiments. AJ created and generated the testcross material. VG, MF, TL, AB, and ZZ organized and performed the experiments and collected the data. VG, MF, TL, and DS analyzed the data. VG and DS wrote the manuscript. All authors read and approved the final version of the manuscript.
Funding
This work has been supported by Croatian Science Foundation under the project IP-11-2013-5707 “Genetics and physiology of multiple stress tolerance in maize (MUST-MAIZE)” and the EU project KK.01.1.1.01.0005 “Biodiversity and Molecular Plant Breeding” of the Centre of Excellence for Biodiversity and Molecular Plant Breeding (CroP-BioDiv), Zagreb, Croatia.
Conflict of Interest Statement
The authors declare that the research was conducted in the absence of any commercial or financial relationships that could be construed as a potential conflict of interest.
Supplementary Material
The Supplementary Material for this article can be found online at: https://www.frontiersin.org/articles/10.3389/fpls.2019.00566/full#supplementary-material
Abbreviations
BLUPs, best linear unbiased predictions; ChlF, chlorophyll fluorescence; ET/(TR-ET), electron transport beyond ; Fv/Fm, maximum quantum yield of PSII; ICIM, inclusive composite interval mapping; IRILs, intermated recombinant inbred lines; LOD, logarithm of odds; PIABS, performance index (potential) for energy conservation from photons absorbed by PSII to the reduction of intersystem electron acceptors; PSII, Photosystem II; , quinone A; QTLs, quantitative trait loci; RC/ABS, reaction centers involved in reduction per Photosystem II antenna chlorophyll; REML, restricted maximum likelihood; TR/DI, maximum quantum yield of primary photochemistry; VPD, vapor pressure deficit
References
Allen, R. G., Pereira, L. S., Raes, D., and Smith, M. (1998). Crop Evapotranspiration - Guidelines for Computing Crop Water Requirements - FAO Irrigation and Drainage paper 56. Rome: FAO.
Anderson, T. W. (1958). An Introduction to Multivariate Statistical Analysis. New York, NY: John Wiley & Sons.
Andorf, C. M., Lawrence, C. J., Harper, L. C., Schaeffer, M. L., Campbell, D. A., and Sen, T. Z. (2010). The locus lookup tool at MaizeGDB: identification of genomic regions in maize by integrating sequence information with physical and genetic maps. Bioinformatics 26, 434–436. doi: 10.1093/bioinformatics/btp556
Araus, J. L., Serret, A. D., and Edmeades, G. O. (2012). Phenotyping maize for adaptation to drought. Front. Physiol. 3:305. doi: 10.3389/fphys.2012.00305
Aslam, M., Maqbool, M. A., and Cengiz, R. (2015). Drought Stress in Maize Zea mays L.). Cham: Springer International Publishing AG.
Avila-Castañeda, A., Gutiérrez-Granados, N., Ruiz-Gayosso, A., Sosa-Peinado, A., Martínez-Barajas, E., and Coello, P. (2014). Structural and functional basis for starch binding in the SnRK1 subunits AKINβ2 and AKINβγ. Front. Plant Sci. 5:199. doi: 10.3389/fpls.2014.00199
Bernardo, R. (2010). Breeding for Quantitative Traits in Plants, Second edition. Woodbury, MN: Stemma Press.
Buendía-Monreal, M., Rentería-Canett, I., Guerrero-Andrade, O., Bravo-Alberto, C. E., Martínez-Castilla, L. P., García, E., et al. (2011). The family of maize D-type cyclins: genomic organization, phylogeny and expression patterns. Physiol. Plant. 143, 297–308. doi: 10.1111/j.1399-3054.2011.01498.x
Cabrera-Bosquet, L., Crossa, J., von Zitzewitz, J., Serret, M. D., and Araus, J. L. (2012). High-throughput phenotyping and genomic selection: the frontiers of crop breeding converge. J. Integr. Plant Biol. 54, 312–320. doi: 10.1111/j.1744-7909.2012.01116.x
Chao, L. M., Liu, Y. Q., Chen, D. Y., Xue, X. Y., Mao, Y. B., and Chen, X. Y. (2017). Arabidopsis transcription factors SPL1 and SPL12 confer plant thermotolerance at reproductive stage. Mol. Plant 10, 735–748. doi: 10.1016/j.molp.2017.03.010
Ciganda, V., Gitelson, A., and Schepers, J. (2009). Non-destructive determination of maize leaf and canopy chlorophyll content. J. Plant Physiol. 166, 157–167. doi: 10.1016/j.jplph.2008.03.004
Cobb, J. N., DeClerck, G., Greenberg, A., Clark, R., and McCouch, S. (2013). Next-generation phenotyping: requirements and strategies for enhancing our understanding of genotype–phenotype relationships and its relevance to crop improvement. Theor. Appl. Genet. 126, 867–887. doi: 10.1007/s00122-013-2066-0
Cooper, M., Technow, F., Messina, C., Gho, C., and Totir, L. R. (2016). Use of crop growth models with whole-genome prediction: application to a maize multienvironment trial. Crop Sci. 56, 2141–2156. doi: 10.2135/cropsci2015.08.0512
Covarrubias-Pazaran, G. (2016). Genome-assisted prediction of quantitative traits using the R Package sommer. PLoS One 11:e0156744. doi: 10.1371/journal.pone.0156744
Dekkers, J. C. M. (2007). Prediction of response to marker-assisted and genomic selection using selection index theory. J. Anim. Breed. Genet. 124, 331–341. doi: 10.1111/j.1439-0388.2007.00701.x
Dietz, K. J., Vogel, M. O., and Viehhauser, A. (2010). AP2/EREBP transcription factors are part of gene regulatory networks and integrate metabolic, hormonal and environmental signals in stress acclimation and retrograde signaling. Protoplasma 245, 3–14. doi: 10.1007/s00709-010-0142-8
Endelman, J. B. (2011). Ridge regression and other kernels for genomic selection with R Package rrBLUP. Plant Genome 4, 250–255. doi: 10.3835/plantgenome2011.08.0024
Fahad, S., Bajwa, A. A., Nazir, U., Anjum, S. A., Farooq, A., Zohaib, A., et al. (2017). Crop production under drought and heat stress: plant responses and management options. Front. Plant Sci. 8:1147. doi: 10.3389/fpls.2017.01147
Falque, M., Décousset, L., Dervins, D., Jacob, A. M., Joets, J., Martinant, J. P., et al. (2005). Linkage mapping of 1454 new maize candidate gene Loci. Genetics 170, 1957–1966. doi: 10.1534/genetics.104.040204
Fiorani, F., and Schurr, U. (2013). Future scenarios for plant phenotyping. Annu. Rev. Plant Biol. 64, 267–291. doi: 10.1146/annurev-arplant-050312-120137
Galić, V. (2018). Quantitative Trait Loci Analysis and Genomewide Selection for Grain Yield in Testcrosses of Maize IBMSyn4 Population. Ph. D thesis, Josip Juraj Strossmayer University of Osijek, Osijek. Available at: http://rektorat.unios.hr/molekularna/dokumenti/storage/zavrseni_doktorati/vlatko.galic.pdf (accessed April 23, 2019).
Gholipoor, M., Choudhary, S., Sinclair, T. R., Messina, C. D., and Cooper, M. (2013). Transpiration response of maize hybrids to atmospheric vapour pressure deficit. J. Agron. Crop Sci. 199, 155–160. doi: 10.1111/jac.12010
Gill, S. S., and Tuteja, M. (2010). Reactive oxygen species and antioxidant machinery in abiotic stress tolerance in crop plants. Plant Physiol. Biochem. 48, 909–930. doi: 10.1016/j.plaphy.2010.08.016
Griffiths, C. A., Paul, M. J., and Foyer, C. H. (2016). Metabolite transport and associated sugar signalling systems underpinning source/sink interactions. Biochim. Biophys. Acta 1857, 1715–1725. doi: 10.1016/j.bbabio.2016.07.007
Hallauer, A. R., Carena, M. J., and Miranda Filho, J. B. (2010). Quantitative Genetics in Maize Breeding. New York, NY: Springer LLC, doi: 10.1007/978-1-4419-0766-0
Hatfield, J. L., and Prueger, J. H. (2015). Temperature extremes: effect on plant growth and development. Weather Clim. Extrem. 10, 4–10. doi: 10.1016/j.wace.2015.08.001
Heffner, E. L., Sorrells, M. E., and Jannik, J. L. (2009). Genomic selection for crop improvement. Crop. Sci. 49, 1–12. doi: 10.2135/cropsci2008.08.0512
IPCC (2014). Climate Shange 2014: Synthesis Rreport. Contribution of Working Groups I, II and III to the Fifth Assessment Report of the Intergovernmental Panel on Climate Change, eds Pachauri, R. K., and Meyer, L. A. (Geneva: IPCC).
Jung, C., and Müller, A. (2009). Flowering time control and applications in plant breeding. Trends Plant Sci. 14, 563–573. doi: 10.1016/j.tplants.2009.07.005
Kalaji, H. M., Schansker, G., Brestic, M., Bussotti, F., Calatayud, A., and Ferroni, L. (2017). Frequently asked questions about chlorophyll fluorescence, the sequel. Photosynth. Res. 132, 13–66. doi: 10.1007/s11120-016-0318-y
Kaul, T., Reddy, P. S., Mahanty, S., Thirulogachandar, V., Reddy, R. A., Kumar, B., et al. (2011). Biochemical and molecular characterization of stress-induced β -carbonic anhydrase from a C4 plant, Pennisetum glaucum. J. Plant Physiol. 168, 601–610. doi: 10.1016/j.jplph.2010.08.007
Kwong, Q. B., Ong, A. L., The, C. K., Chew, F. T., Tammi, M., Mayes, S., et al. (2017). Genomic selection in commercial perennial crops: applicability and improvement in oil palm (Elaeis guineensis Jacq.). Sci. Rep. 7:2872. doi: 10.1038/s41598-017-02602-6
Lee, M., Sharopova, N., Beavis, N., Grant, W. D., Katt, M., Blair, D., et al. (2002). Expanding the genetic map of maize with the intermated B73 × Mo17 (IBM) population. Plant Mol. Biol. 48, 453–461.
Lepeduš, H., Brkić, I., Cesar, V., Jurković, V., Antunović, J., Jambrović, A., et al. (2012). Chlorophyll fluorescence analysis of photosynthetic performance in seven maize inbred lines under water-limited conditions. Period. Biol. 114, 73–76.
Li, F., Vallabhaneni, R., Yu, J., Rocheford, T., and Wurtzel, E. (2008). The maize phytoene synthase gene family: overlapping roles for carotenogenesis in endosperm, photomorphogenesis, and thermal stress tolerance. Plant Physiol. 147, 1334–1346. doi: 10.1104/pp.108.122119
Liu, H., Niu, Y., Gonzalez-Portilla, P. J., Zhou, H., Wang, L., Zuo, T., et al. (2015). An ultra-high-density map as a community resource for discerning the genetic basis of quantitative traits in maize. BMC Genomics 16:1078. doi: 10.1186/s12864-015-2242-5
Lobell, D. B., Hammer, G. L., McLean, G., Messina, C., Roberts, M. J., and Schlenker, W. (2013). The critical role of extreme heat for maize production in the United States. Nat. Clim. Change 3, 497–501. doi: 10.1038/nclimate1832
Lysenko, E. A. (2007). Plant sigma factors and their role in plastid transcription. Plant Cell Rep. 26, 845–859. doi: 10.1007/s00299-007-0318-7
Mao, H. D., Yu, L. J., Li, Z. J., Yan, Y., Han, R., Liu, H., et al. (2016). Genome-wide analysis of the SPL family transcription factors and their responses to abiotic stresses in maize. Plant Gene 6, 1–12. doi: 10.1016/j.plgene.2016.03.003
Massman, J. M., Gordillo, A., Lorenzana, R. E., and Bernardo, R. (2013). Genomewide predictions from maize single-cross data. Theor. Appl. Genet. 126, 13–22. doi: 10.107/s00122-012-1955-y
Mathur, S., Agrawal, D., and Jajoo, A. (2014). Photosynthesis: limitations in response to high temperature stress. J. Photochem. Photobiol. B Biol. 137, 116–126. doi: 10.1016/j.jphotobiol.2014.01.010
Meng, L., Li, H., Zhang, L., and Wang, J. (2015). QTL IciMapping: integrated software for genetic linkage map construction and quantitative trait locus mapping in biparental populations. Crop J. 3, 269–283. doi: 10.1016/j.cj.2015.01.001
Messina, C. D., Technow, F., Tang, T., Totir, R. L., Gho, C., and Cooper, M. (2018). Leveraging biological insight and environmental variation to improve phenotypic prediction: integrating crop growth models (CGM) with whole genome prediction (WGP). Eur. J. Agr. 151–162. doi: 10.1016/j.eja.2018.01.007
Mhamdi, A., Noctor, G., and Baker, A. (2012). Plant catalases: peroxisomal redox guardians. Arch. Biochem. Biophys. 525, 181–194. doi: 10.1016/j.abb.2012.04.015
Mihaljevic, R., Schön, C. C., Utz, H. F., and Melchinger, A. E. (2005). Correlations and QTL correspondence between line Per Se and testcross performance for agronomic traits in four populations of European Maize. Crop Sci. 45, 114–122.
Millet, E. J., Welcker, C., Kruijer, W., Negro, S., Coupel-Ledru, A., Nicolas, S. D., et al. (2016). Genome-wide analysis of yield in europe: allelic effects vary with drought and heat scenarios. Plant Physiol. 172, 749–764. doi: 10.1104/pp.16.00621
Mode, C. J., and Robinson, H. F. (1959). Pleiotropism and the genetic variance and covariance. Biometrics 15, 518–537.
Nuccio, M. L., Wu, J., Mowers, R., Zhou, H. P., Meghji, M., Primavesi, L. F., et al. (2015). Expression of trehalose-6-phosphate phosphatase in maize ears improves yield in well-watered and drought conditions. Nat. Biotechnol. 33, 862–869. doi: 10.1038/nbt.3277
Oukarroum, A., Madidi, S. E., Schansker, G., and Strasser, R. J. (2007). Probing the responses of barley cultivars (Hordeum vulgare L.) by chlorophyll a fluorescence OLKJIP under drought stress and rewatering. Environ. Exp. Bot. 60, 438–446. doi: 10.1016/j.envexpbot.2007.01.002
Patterson, H. N., and Williams, E. R. (1976). A new class of resolvable incomplete block designs. Biometrika 63, 83–92. doi: 10.1093/biomet/63.1.83
Peng, B., Li, Y., Wang, Y., Liu, C., Liu, Z., Zhang, Y., et al. (2013). Correlations and comparisons of quantitative trait loci with family per se and testcross performance for grain yield and related traits in maize. Theor. Appl. Genet. 126, 773–789. doi: 10.1007/s00122-012-2017-1
Perdomo, J. A., Carmo-Silva, E., Hermida-Carrera, C., Flexas, J., and Galmés, J. (2016). Acclimation of biochemical and diffusive components of photosynthesis in rice, wheat, and maize to heat and water deficit: implications for modeling photosynthesis. Front. Plant Sci. 7:1719. doi: 10.3389/fpls.2016.01719
Pokhilko, A., and Ebenhöh, O. (2015). Mathematical modelling of diurnal regulation of carbohydrate allocation by osmo-related processes in plants. J. R. Soc. Interface 12:20141357. doi: 10.1098/rsif.2014.1357
Ribaut, J. M., Betran, J., Monneveux, P., and Setter, T. (2009). “Drought tolerance in maize,” in Handbook of Maize: Its Biology, eds J. L. Bennetzen, and S. C. Hake (New York, NY: Springer), 311–344. doi: 10.1007/978-0-387-79418-1_16
Rohacek, K., and Bartak, M. (1999). Technique of the modulated chlorophyll fluorescence: basic concepts, useful parameters, and some applications. Photosynthetica 37, 339–363.
Sayed, O. H. (2003). Chlorophyll fluorescence as a tool in cereal crop research. Photosynthetica 41, 321–330. doi: 10.1023/b:phot.0000015454.36367.e2
Schaeffer, M. L., Sanchez-Villeda, H., and Coe, E. (2008). IBM 2008 Neighbors Map. Available at: http://www.maizegdb.org (accessed April 23, 2019).
Sharkey, T. D., and Schrader, S. M. (2006). High Temperature Stress in Physiology and Molecular Biology of Stress Tolerance in Plants. Berlin: Springer, 101–129.
Šimić, D., Lepeduš, H., Jurković, V., Antunović, J., and Cesar, V. (2014). Quantitative genetic analysis of chlorophyll a fluorescence parameters in maize in the field environments. J. Integr. Plant Biol. 56, 695–708. doi: 10.1111/jipb.12179
Stefanov, D., Petkova, V., and Denev, I. D. (2011). Screening for heat tolerance in common bean Phaseolus vulgaris L.) lines and cultivars using JIP-test. Sci. Hortic. 128, 1–6. doi: 10.1016/j.scienta.2010.12.003
Strasser, R. J., Srivastava, A., and Govindjee. (1995). Polyphasic chlorophyll a fluorescence transient in plants and cyanobacteria. Photochem. Photobiol. 61, 32–42. doi: 10.1111/j.1751-1097.1995.tb09240.x
Strasser, R. J., Srivastava, A., and Govindjee. (2004). “Analysis of the fluorescence transient,” in Chlorophyll a Fluorescence: a Signature of Photosynthesis. Advances in Photosynthesis and Respiration, Vol. 19, eds G. C. Papageorgiou and Govindjee (Dordrecht: Springer), 321–362. doi: 10.1007/978-1-4020-3218-9_12
Strasser, R. J., Srivastava, A., and Tsimili-Michael, M. (2000). “The fluorescent transient as a tool to characterize and screen photosynthetic samples,” in Probing Photosynthesis: Mechanisms, Regulation and Adaptation, eds M. Yunus, U. Pathre and P. Mohanty (London: Taylor and Francis), 445–483.
Tardieu, F. (2011). Any trait or trait-related allele can confer drought tolerance: just design the right scenario. J. Exp. Bot. 63, 25–31. doi: 10.1093/jxb/err269
Tardieu, F., Simonneau, T., and Muller, B. (2018). The physiological basis of drought tolerance in crop plants: a scenario-dependent probabilistic approach. Annu. Rev. Plant Biol. 69, 733–759. doi: 10.1146/annurev-arplant-042817-040218
Teixeira, E. I., Fischer, G., van Velthuizen, H., Walter, C., and Ewert, F. (2013). Global hot-spots of heat stress on agricultural crops due to climate change. Agric. For. Meteorol. 170, 206–215. doi: 10.1016/j.agrformet.2011.09.002
Utz, H. F. (2005). PLABSTAT—a Computer Program for Statistical Analysis of Plant Breeding Experiments, 3A. Germany: Universität Hohenheim.
Van Heerden, P. D., Strasser, R. J., and Krüger, G. H. (2004). Reduction of dark chilling stress in N2-fixing soybean by nitrate as indicated by chlorophyll a fluorescence kinetics. Physiol. Plant 121, 239–249. doi: 10.1111/j.0031-9317.2004.0312.x
Wahid, A., Gelani, S., Ashraf, M., and Foolad, M. R. (2007). Heat tolerance in plants: an overview. Environ. Exp. Bot. 61, 199–223. doi: 10.1016/j.envexpbot.2007.05.011
Wang, S. C., Basten, C. J., and Zeng, Z. B. (2011). Windows QTL Cartographer 2.5. Raleigh, NC: Department of Statistics, North Carolina State University.
Živčák, M., Brestiè, M., Olšovska, K., and Slamka, P. (2008). Performance Index as a sensitive indicator of water stress in Triticum aestivum. Plant Soil. Environ. 54, 133–139. doi: 10.17221/392-pse
Keywords: flowering, grain yield, heat, photosynthetic performance, pleiotropy, quantitative trait loci, Zea mays
Citation: Galic V, Franic M, Jambrovic A, Ledencan T, Brkic A, Zdunic Z and Simic D (2019) Genetic Correlations Between Photosynthetic and Yield Performance in Maize Are Different Under Two Heat Scenarios During Flowering. Front. Plant Sci. 10:566. doi: 10.3389/fpls.2019.00566
Received: 01 June 2018; Accepted: 15 April 2019;
Published: 30 April 2019.
Edited by:
Vasilij Goltsev, Sofia University, BulgariaReviewed by:
Just Jensen, Aarhus University, DenmarkPedro Martinez-Gomez, Spanish National Research Council (CSIC), Spain
Copyright © 2019 Galic, Franic, Jambrovic, Ledencan, Brkic, Zdunic and Simic. This is an open-access article distributed under the terms of the Creative Commons Attribution License (CC BY). The use, distribution or reproduction in other forums is permitted, provided the original author(s) and the copyright owner(s) are credited and that the original publication in this journal is cited, in accordance with accepted academic practice. No use, distribution or reproduction is permitted which does not comply with these terms.
*Correspondence: Domagoj Simic, domagoj.simic@poljinos.hr