- Chair of Plant Nutrition, Technical University of Munich, Munich, Germany
High-throughput, non-invasive phenotyping is promising for evaluating crop nitrogen (N) use efficiency (NUE) and grain yield (GY) formation under field conditions, but its application for genotypes differing in morphology and phenology is still rarely addressed. This study therefore evaluates the spectral estimation of various dry matter (DM) and N traits, related to GY and grain N uptake (Nup) in high-yielding winter wheat breeding lines. From 2015 to 2017, hyperspectral canopy measurements were acquired on 26 measurement dates during vegetative and reproductive growth, and 48 vegetation indices from the visible (VIS), red edge (RE) and near-infrared (NIR) spectrum were tested in linear regression for assessing the influence of measurement stage and index selection. For most traits including GY and grain Nup, measurements at milk ripeness were the most reliable. Coefficients of determination (R²) were generally higher for traits related to maturity than for those related to anthesis canopy status. For GY (R² = 0.26–0.51 in the three years, p < 0.001), and most DM traits, indices related to the water absorption band at 970 nm provided better relationships than the NIR/VIS indices, including the normalized difference vegetation index (NDVI), and the VIS indices. In addition, most indices including RE bands, notably NIR/RE combinations, ranked above the NIR/VIS group. Due to index saturation, the index differentiation was most apparent in the highest-yielding year. For grain Nup and total Nup, the RE/VIS index MSR_705_445 and the simple ratio R780_R740 ranked highest, followed by other RE indices. Among the vegetative organs, R² values were mostly highest and lowest for leaf and spike traits, respectively. For each trait, index and partial least squares regression (PLSR) models were validated across years at milk ripeness, confirming the suitability of optimized index selection. PLSR improved the prediction errors of some traits but not consistently the R² values. The results suggest the use of sensor-based phenotyping as a useful support tool for screening of yield potential and NUE and for identifying contributing plant traits—which, due to their expensive and cumbersome destructive determination are otherwise not readily available. Water band and RE indices should be preferred over NIR/VIS indices for DM traits and N-related traits, respectively, and milk ripeness is suggested as the most reliable stage.
Introduction
Spectral high-throughput sensing has gained increasing attention for efficient assessment of genotypic performance of plant breeding material (Furbank and Tester, 2011; Araus and Cairns, 2014). Various authors have stressed the suitability of using reflectance data measured earlier in the season for the estimation of grain yield (GY). This would enable plant breeders to focus on a limited set of promising genotypes for further rating, thus even making yield determination of dismissed lines unnecessary (Garriga et al., 2017). Besides GY, GY formation, its mechanisms, and the contribution of plant organs were less frequently addressed with spectral methods, especially for the discrimination of genotypes. However, a better understanding of these mechanisms could facilitate plant breeders to target specific traits (Acquaah, 2007), such as leaf area and nitrogen (N) concentration for increasing assimilation, and spike and culm characteristics for increasing the sink and storage size for assimilates, respectively (Schnyder, 1993; Feng et al., 2016).
The contribution of plant organs as sink or source for assimilates and N differs both between genotypes and between growth stages, so that their potential use as traits for indirect selection differs during the grain-filling phase (Barmeier and Schmidhalter, 2017; Prey et al., 2019b; Prey et al., 2019c). Evaluating the variation of these traits in breeding lines can provide valuable complementary information for plant breeders for optimized selection of N use efficiency (NUE), notably GY and grain N uptake (GNup). Additionally, estimating the translocation of dry matter (DM) and N from vegetative organs would contribute to the understanding of promising strategies of the temporal DM and N acquisition (Slimane et al., 2013; Prey et al., 2019a; Prey et al., 2019b) However, the determination of such traits is expensive and cumbersome, thus requiring low-cost robust high-throughput techniques (Nguyen and Kant, 2018).
Such spectral methods need to be optimized in terms of the selection of suitable spectral bands, growth stages for measurements, and spectral models. For GY, different spectral vegetation indices (SVI) were compared for the in-season estimation in durum (Aparicio et al., 2000; Royo et al., 2003), spring barley (Rischbeck et al., 2016; Barmeier et al., 2017), or wheat (Tucker et al., 1980; Raun et al., 2001; Freeman et al., 2003; Moges et al., 2004; Babar et al., 2006a; Babar et al., 2006b; Babar et al., 2007; Prasad et al., 2007a; Prasad et al., 2007b; Gizaw et al., 2016a; Gizaw et al., 2016b). A number of these studies that were often conducted in warm or drought-prone environments strengthened the suitability of band combinations from the near-infrared (NIR) spectrum, including the water absorption band around 970 nm (Babar et al., 2006b; Gutierrez et al., 2010b; Gizaw et al., 2016a; Rischbeck et al., 2016; Becker and Schmidhalter, 2017; Garriga et al., 2017) due to the relation of canopy water mass with biomass and water status with assimilation, respectively, as well as the lower saturation of these bands.
In contrast to GY, GNup and the underlying traits of the formation of GY and GNup were rarely assessed with spectral methods. Barmeier and Schmidhalter (2017) evaluated the spectral estimation of organ-level DM and N uptake (Nup) traits at anthesis and dough ripeness in spring barley and recommended the R780_R670 simple ratio index for DM traits to overcome the saturation of the normalized difference vegetation index (NDVI). The DM and Nup of leaf blades followed by those of culms were mostly better predicted than those of spikes and leaf sheaths. Using red edge (RE)-based SVIs in winter wheat breeding lines grown in small plots, Frels et al. (2018) found mostly weaker but significant relationships with DM and Nup at anthesis and maturity as well as with N harvest index (NHI), N utilization efficiency (NutEff), N uptake efficiency (NupEff), and post-anthesis N uptake (PANup). These authors recommended the RE Maccioni index and identified the early grain filling stage as the most promising. Testing many SVIs for predicting GY, GNup, and NUE traits, Pavuluri et al. (2015) confirmed related indices such as the R780_R740 (Mistele et al., 2004) and found better correlations under reduced N fertilization, that was ascribed to the lower saturation in thinner canopies. Various studies found SVIs to be highly heritable (Babar et al., 2007; Prasad et al., 2007a; Frels et al., 2018) or to be related to QTLs associated with GY (Gizaw et al., 2016a), thus to be used as promising indirect selection tools if sufficient correlations are found early enough in the season. Most studies that assessed traits related to N status in response to N fertilization predominantly agree on the usefulness of RE bands for vegetative N concentration (NC) (Mistele and Schmidhalter, 2008a; Li et al., 2010), Nup (Mistele and Schmidhalter, 2008b; Mistele and Schmidhalter, 2010; Li et al., 2013; Guo et al., 2017; Prey and Schmidhalter, 2019a), N status, biomass, and LAI (Hansen and Schjoerring, 2003), as well as grain NC and Nup (Li et al., 2014; Prey and Schmidhalter, 2019b), due to the shift in the RE reflection as indicator for the N status. RE indices were also found useful for the estimation of biomass traits due to their higher sensitivity in dense canopies (Pavuluri et al., 2015; Frels et al., 2018). Band combinations in the visible range were recommended to be useful for pigment-related traits (Peñuelas et al., 1995; Gitelson et al., 2002; Hansen and Schjoerring, 2003).
Besides SVIs, multivariate analysis such as partial least squares regression (PLSR) holds the advantage of including more spectral information than SVIs, but may be affected by overfitting, so that more calibration data may be required (Mehmood et al., 2012; Overgaard et al., 2013) Comparing SVIs and PLSR, improvements were found for biomass and NC but not for chlorophyll concentration and LAI (Hansen and Schjoerring, 2003). Similar GY predictions were found from PLSR and best SVIs for spring barley (Barmeier et al., 2017). These authors reported improved RMSE values by PLSR but similar coefficients of determination for estimating organ-level traits (Barmeier and Schmidhalter, 2017). While SVIs can be derived from both multi- and hyperspectral data, the potential improvements by multivariate analysis are restricted to hyperspectral data, which comes at the price of more expensive sensors, being less convenient to use in practice, for example on UAV-based platforms (Oehlschläger et al., 2018). Therefore, the benefit of PLSR for traits of NUE and yield formation remains to be evaluated for wheat.
The application of spectral phenotyping depends on the wheat type and environment (Gizaw et al., 2016b). Therefore, the assessment of the influence of measurement conditions regarding growth stage and plant phenology is essential. Yet, often only few growth stages were evaluated for GY, focusing on the period from booting until early grain filling. Several studies reported increasing correlations until milk ripeness (Freeman et al., 2003; Babar et al., 2006a; Gutierrez et al., 2010b; Christopher et al., 2014; Becker and Schmidhalter, 2017). While relationships peaked at anthesis in a rain-fed trial, they increased until maturity under irrigated conditions but were generally lower due to saturation effects in denser canopy (Aparicio et al., 2000). Under water-limited conditions, heading, anthesis (Aparicio et al., 2000; Becker and Schmidhalter, 2017) and stem elongation-heading (Pavuluri et al., 2015) were useful stages. Though year-specific differences were substantial, Frels et al. (2018) recommended the early grain filling stage for NUE traits, but a similar evaluation under high-yielding conditions is missing.
In contrast to the variation driven by altered N application, the detection of variation between genotypes is likely to be more affected by the influence of varying morphology, shifted phenology, and differing contributions of indirect DM and N traits to GY and GNup. Moreover, even if the methods are also promising in high-yielding environments (Gizaw et al., 2016b), many of the studies on spectral GY prediction were conducted on spring wheat (Babar et al. 2006a; Babar et al., 2007; Gutierrez et al. 2010a; Sultana et al., 2014) or in environments with low yield potential. This limits the transferability to high-yielding winter wheat, given that weaker relationships were often reported from irrigated trials or denser canopies compared to drought-stress trials (Aparicio et al., 2000; Babar et al., 2006a; Becker and Schmidhalter, 2017; Frels et al., 2018). Moreover, the optimized selection of SVIs depends on the availability of suitable sensors—characterized by band number, narrowness, and placement—and measurement stages (Thenkabail et al., 2000; Prey and Schmidhalter, 2019b).
The present study, therefore, tested the performance of 48 SVIs for the estimation of GY, GNup, and 45 further organ- and plant-level DM and N traits from hyperspectral passive proximal canopy sensing acquired from leaf development until dough ripeness over three years in a high-yielding West-European environment, based on a previous evaluation of the included DM and N reference traits (Prey et al., 2019b). The questions addressed are (i) the detectability of reference traits, (iii) the influence of optimized selection of specific SVIs, (iii) the selection of optimum growth stages for measurements, and (iv) the use of PLSR in comparison to SVIs.
Materials and Methods
Field Experiments and Plant Sampling
The field experiment was conducted over three years from 2014/2015 to 2016/2017 for evaluating traits with influence on NUE and yield formation in a diverse population of winter wheat double haploid breeding lines. The population's parents consisted of elite cultivars and breeding lines provided by regional plant breeders. The population had undergone pre-selection, which removed genotypes peculiar in terms of extreme flowering date, plant height, and disease susceptibility. The trials comprised 75 lines in two replicates in 2014/2015, 75 lines in four replicates in 2015/2016, and 32 selected lines representing the overall yield variation in 4 replicates in 2016/2017. In addition, three high performance cultivars were included as references. The plot width was 1.5 m, and the plot length was 6.5 m. The trial was located approximately 25 km North of Munich (48.406 N, 11.692 E). The soil consisted mainly of homogeneous Cambisols of loamy clay. The precipitation in the main wheat growing period from October to August was 714 mm in 2014/15, 746 mm in 2015/16 and 690 nm in 2016/2017. During the grain filling period in 2015, heat and lack of precipitation caused moderate drought stress whereas grain filling in 2016 was influenced by fungal pathogens. The preceding crop was winter wheat in the first and second year and grass-clover in the third year.
Biomass sampling was conducted at anthesis (Zadoks growth stage 6), and at physiological maturity (stage 9). Sampling dates were determined for each genotype by visual scoring. For sampling at anthesis, 20 randomly selected spiked culms were cut directly at stem base in 2015 and 30 culms in 2016 and 2017, and at maturity 30 culms in 2015 and 50 culms in 2016 and 2017, respectively. The plants were manually separated into leaf blades, stems including leaf sheaths, and spikes. In 2016, only a subset of 34 genotypes was separated into vegetative organs. At maturity, spikes were threshed. Plant samples were oven-dried at 50°C until constant weight was reached and DM weight was determined by weighing. N concentration (NC) of the plant material was analyzed by means of NIR spectroscopy in a Foss Rapid Content Analyzer for leaves and spikes and in a Bruker Vector 22/N for the remaining organs. Final GY per plot was determined using a combined harvester. Spike density per plot was calculated by dividing GY per area by yield per spike. Nitrogen uptake (Nup) was calculated by multiplying DM with NC. Further indirect traits related to yield components, DM and N translocation and N uptake and utilization efficiency were calculated (Table 1). Reference traits were categorized into DM and N traits (Prey et al., 2019b). Moreover, these are either direct traits, which were directly retrieved from plant sampling either at anthesis or maturity like DM per ha, NC or Nup, or indirectly derived DM and N traits, which were predominantly calculated using data from both sampling dates or from different plant organs (Table 1). See Prey et al. (2019b) for details on the plant sampling, descriptive statistics and correlations of the plant traits. Plant height had been included in the analysis of the reference traits, but was not considered in the spectral analysis since it is easily assessable from height sensors (Barmeier et al., 2016), which were not available on all measurement dates.
Spectral Measurements
Spectral measurements were conducted using the PhenoTrac 4 multi-sensor platform during various growth stages throughout the season (Table 2). In 2015, measurements were performed on only four dates, in 2016 on 12 dates and in 2017 on 10 dates, with the highest frequency during the grain filling phase due to the more rapid canopy development and the expected better relationships with maturity traits. The PhenoTrac 4 is equipped with a hyperspectral bidirectional passive point sensor spectrometer (tec5, Oberursel, Germany), measuring at a nominal resolution of approximately 3.3 nm between 300 and 1000 nm. The measurement distance was approx. 80 cm above the canopy. Measurements were registered at a frequency of 5 Hz together with the GPS coordinates from the TRIMBLE RTK-GPS (real-time kinematic global positioning system; Trimble, Sunnyvale, CA, USA). See Kipp et al. (2014) and Erdle et al. (2011) for further description of the sensor system.
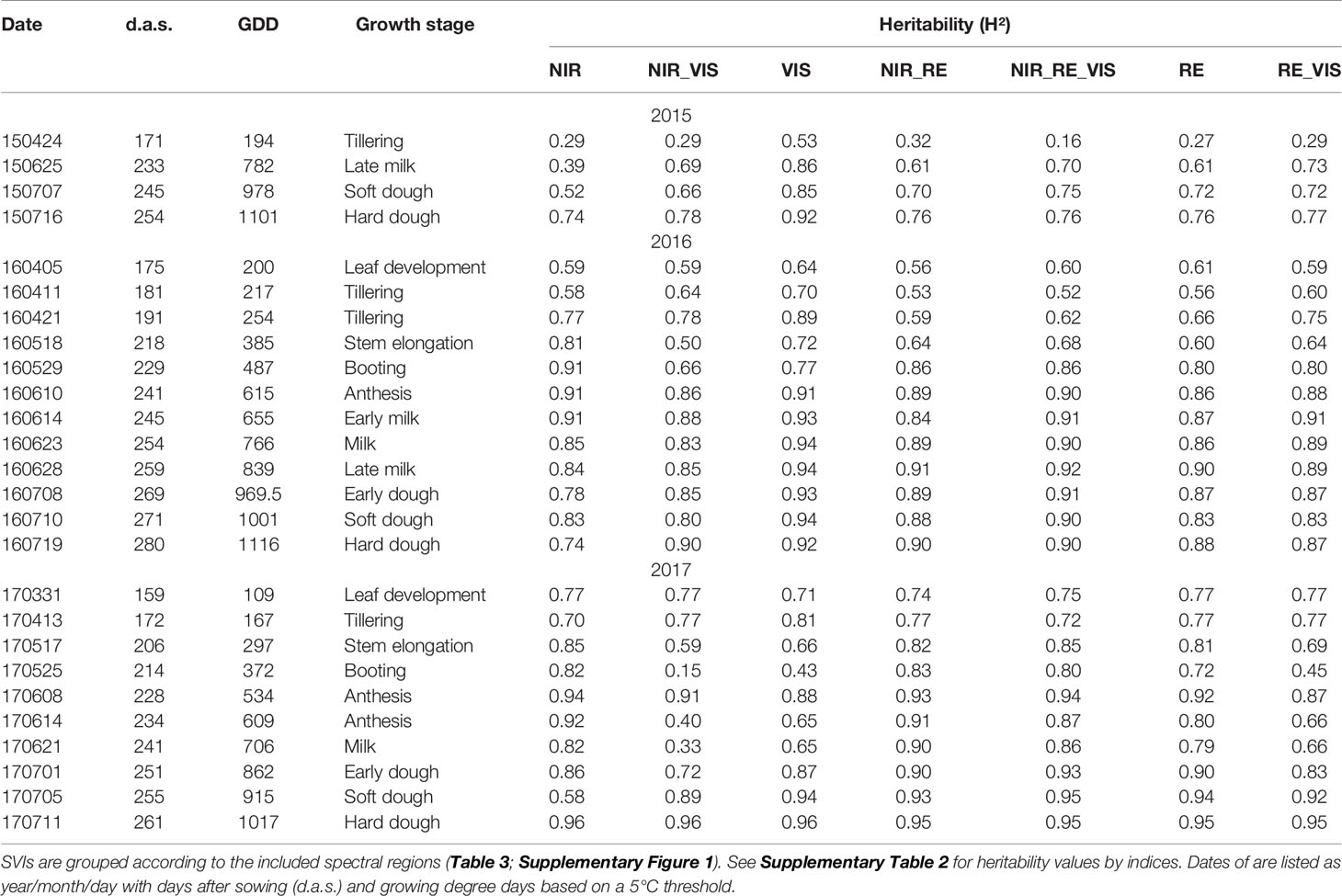
Table 2 Heritability of indices averaged by index groups by measurement dates calculated for all measurement dates.
Selection of Vegetation Indices
SVIs were selected from the literature based on previous work that identified useful applications of the indices, and from an Index-database (https://www.indexdatabase.de; Henrich et al., 2012). The indices were grouped by the included spectral ranges (visible light [VIS], the extended RE, and NIR), with the VIS < 700 nm, RE: 700–765 nm and the NIR > 765 nm (Table 3; Supplementary Figure 1). Prior to index calculation, the spectra were smoothed using a five-band moving average filter (Mistele and Schmidhalter, 2010) in order to remove spectral noise. However, comparisons with unsmoothed data suggested little influence of spectral noise on the trait/index relationships.
Statistical Analysis
For each sampling stage, each SVI was tested in simple linear regressions with DM and N-traits using mean values per genotype as averaged across the replicates. Data analysis was conducted in R (version 3.4.; R Core Team, 2017), using the lm-function. The coefficient of determination (R²) was used to compare the linear relationships. Broad-sense heritability (H²) was calculated for the SVIs for each measurement date using the lmer function as H² = Vg/(Vg+Ve/nR), where V denotes the variance component for the effects of genotype (Vg) and of the residual variance (Ve), and nR the number of replicates (nR = 2 in 2015 and nR = 4 in 2016 and 2017).
In order to overcome the influence of differing growing conditions as well as of the date-specific index rankings, indices were quantitatively ranked by their normalized performance for each trait, adapting the ranking by Frels et al. (2018). Since the coefficient of determination is range-dependent but independent of the level of the trait, it represents a bivariate ranking of the genotypes. Therefore, the R² values were used instead of the RMSE values. For each trait, the seasonally mean (Supplementary Equation 1a) and maximum (Supplementary Equation 1c) R² values of each index were normalized (Supplementary Equation 1e, f) to the trait-specific seasonally mean (Supplementary Equation 1b) and maximum (Supplementary Equation 1d) R² within each year as calculated from the results of all indices, respectively. Thus, a value > 1 indicated a comparative advantage of the index for the trait under consideration. Consequently, both the within-year mean- and maximum-based rankings (Supplementary Figure 9) were summed up across the three years for achieving year-independent rankings (Supplementary Equation 1g, h). These across-years mean- and maximum- based rank sums were combined by summing up both ranking sums for a unique ranking per trait (Supplementary Equation 1i). Considering a selection of indices that is robust towards date-specific effects as more important, the mean-based rank sums were double-weighted. These weighted mean/maximum-rank sums (WMMRS) were used for identifying one trait-specific optimum index, irrespective of the R² level achieved.
The selected indices were validated in test set validations across years by linear regression on the trait-specific WMMRS-indices and on the NDVI (“NDVI2”) and REIP indices, considered as widely used “reference” indices, in comparison to PLSR models. Based on the seasonal evaluation of the SVI-relationships, calibration and validation was conducted using milk ripeness measurements (June 25, 2015, June 28, 2016, and June 21, 2017). Initial PLSR models were fitted on smoothed spectral data for evaluating influential spectral bands. PLSR models used for predictions were based on spectra additionally pretreated by Savitzki-Golay first order derivation due to significant improvements (not shown). Bands below 370 nm and above 990 nm were not included due to spectral noise. PLSR was fitted using the kernel algorithm (Mevik and Wehrens, 2007) in the pls package. The optimum number of components was determined by minimizing the cross validation RMSE with the restriction that an additional component further decreased the RMSE by at least 1%. For both SVIs and PLSR, validation was conducted cross-wise on the data of both other years, resulting into each six validation cases.
Results
Heritability of Vegetation Indices
Heritability (H²) estimated for all SVIs was higher in 2016 and 2017 than in 2015 and generally increased in all index groups with ongoing plant development (Table 3; Supplementary Table 1). For all measurement dates in 2015, the group of VIS indices reached the highest H² values, whereas the NIR/RE/VIS indices yielded similar values in 2016, mostly followed by the group of RE/VIS indices. In 2016, unlike in most other groups, the H² of NIR indices was highest (0.90) already at booting and anthesis, followed by decreasing values until hard dough (0.76). Though moderate H² values (~0.60) were already reached before stem elongation, H² mostly exceeded 0.80 only after booting/anthesis, both in 2016 and 2017. Notably, many indices including VIS bands were not heritable during booting, later anthesis and milk ripeness in 2017, whereas most indices with NIR bands still reached high values (>0.80) on these days.
The Seasonal Trait Assessment
The relationships found between reference traits and SVIs differed between measurement days and between years, so that an identification of optimal measurement dates and SVIs is necessary. Mean and maximum (Supplementary Figure 2) coefficients of determination (R²) peaked during milk ripeness and early dough ripeness at the end of June for most traits in both 2015 and 2016, whereas R² values increased for later measurement dates in 2017 (Figure 3; Figure 4). In all years and for most traits, the steepest R² increase was found between anthesis and milk ripeness, whereas useful relationships were rarely found during the vegetative phase.
For direct DM and Nup traits and for NC, predominantly closer relationships were observed for the maturity traits than for the anthesis traits. Due to the dominant effect of the measurement date, no clear differences in the date suitability by trait were found (Supplementary Figure 2). In all years, the VIS indices represented the weakest index group and their R² values decreased earlier during grain filling than those of most other indices (Supplementary Figure 3). In 2016, several indices with RE bands yielded higher R² values during stem elongation (May 18) than those of the other groups. In 2017, the relationships reached from NIR/VIS indices increased later at anthesis/grain filling than from the other indices.
Seasonal Relationships and Index Rankings
For assessing the trait detection, the trait-specific index suitability, and the stability over time, seasonally maximum (Figure 1; Supplementary Figure 4) and mean (Supplementary Figure 5) R² values were calculated for each SVI–trait combination across measurement dates in the individual years. The group of direct DM traits was relatively best assessed, followed by direct Nup traits, whereas the derived DM traits were the least estimated (Table 4). The relationships differed more strongly between traits and years for the groups of derived DM and N traits. Mean and maximum R² values by traits were closely related for most traits, indicating that the comparison of the trait estimation was not derived from specific dates only. For each trait, the indices were ranked based on weighted mean/maximum-rank sums (WMMRS) achieved over the three years (Figure 2). Seasonal R² values are presented for selected DM and N traits.
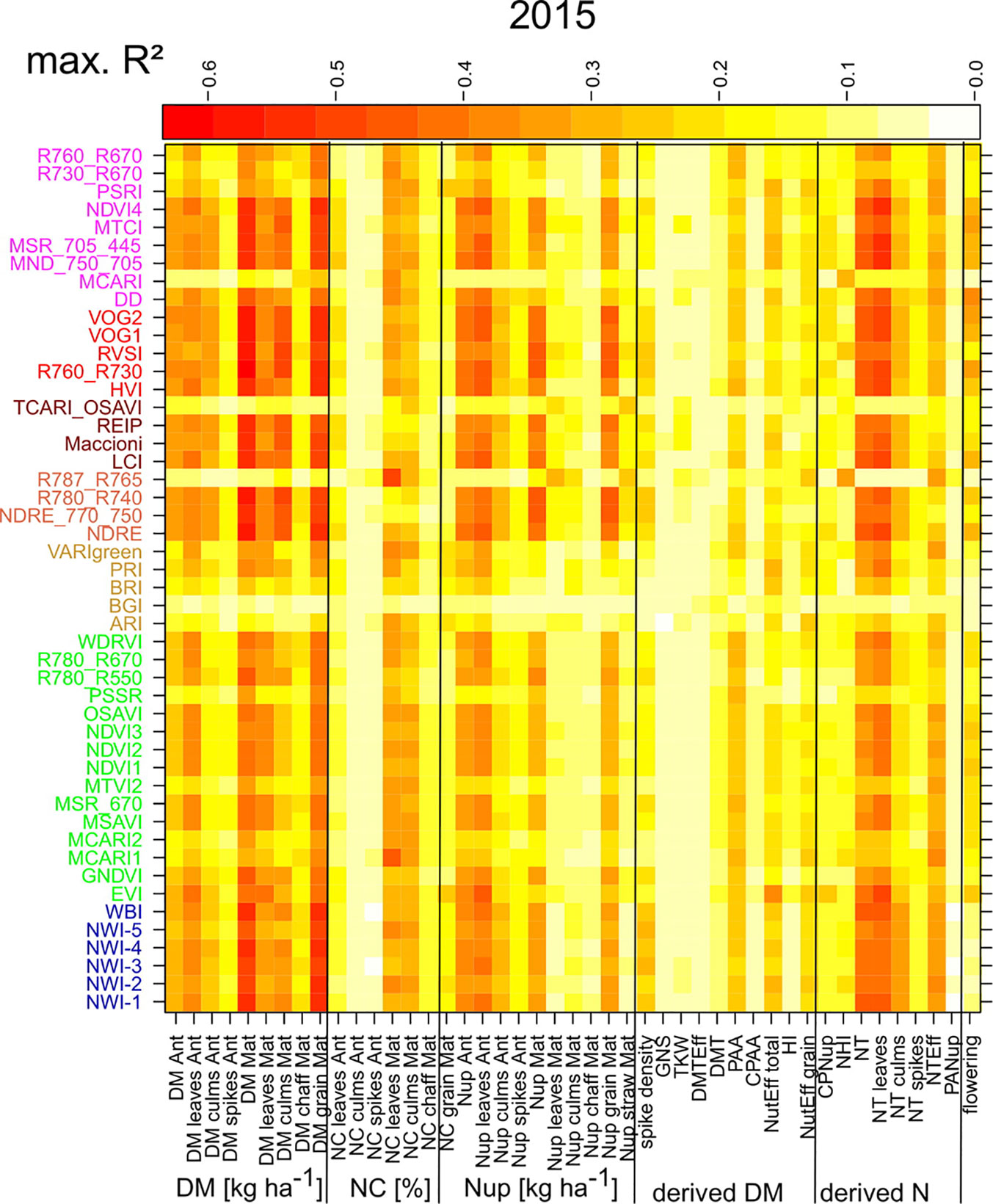
Figure 1 Maximum coefficients of determination (R²) calculated across the R² values of the different measurement dates in 2015 by trait/SVI combinations. Indices are colored according to the included spectral regions (Supplementary Figure 1; from bottom to top): NIR (blue), NIR/VIS (green), VIS (orange), NIR/RE (light red), NIR/RE/VIS (brown), RE (red), RE/VIS (purple). Refer to Supplementary Figure 4 for results of the other years.
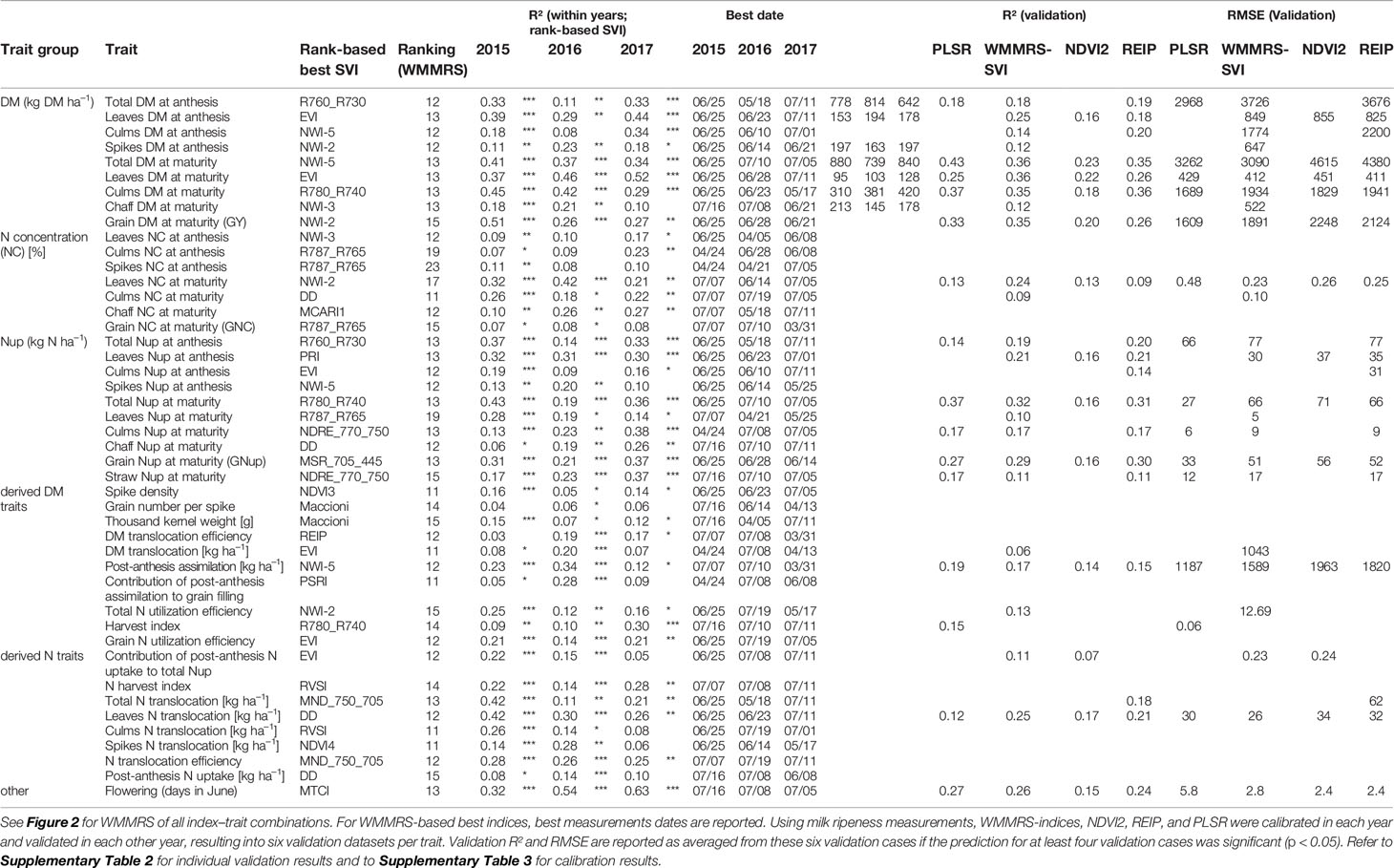
Table 4 Best trait-specific indices identified based on the weighted mean/maximum rank sums (WMMRS) and their coefficients of determination (R²; *: p < 0.05; **: p < 0.01; ***: p < 0.001) on the optimum dates.
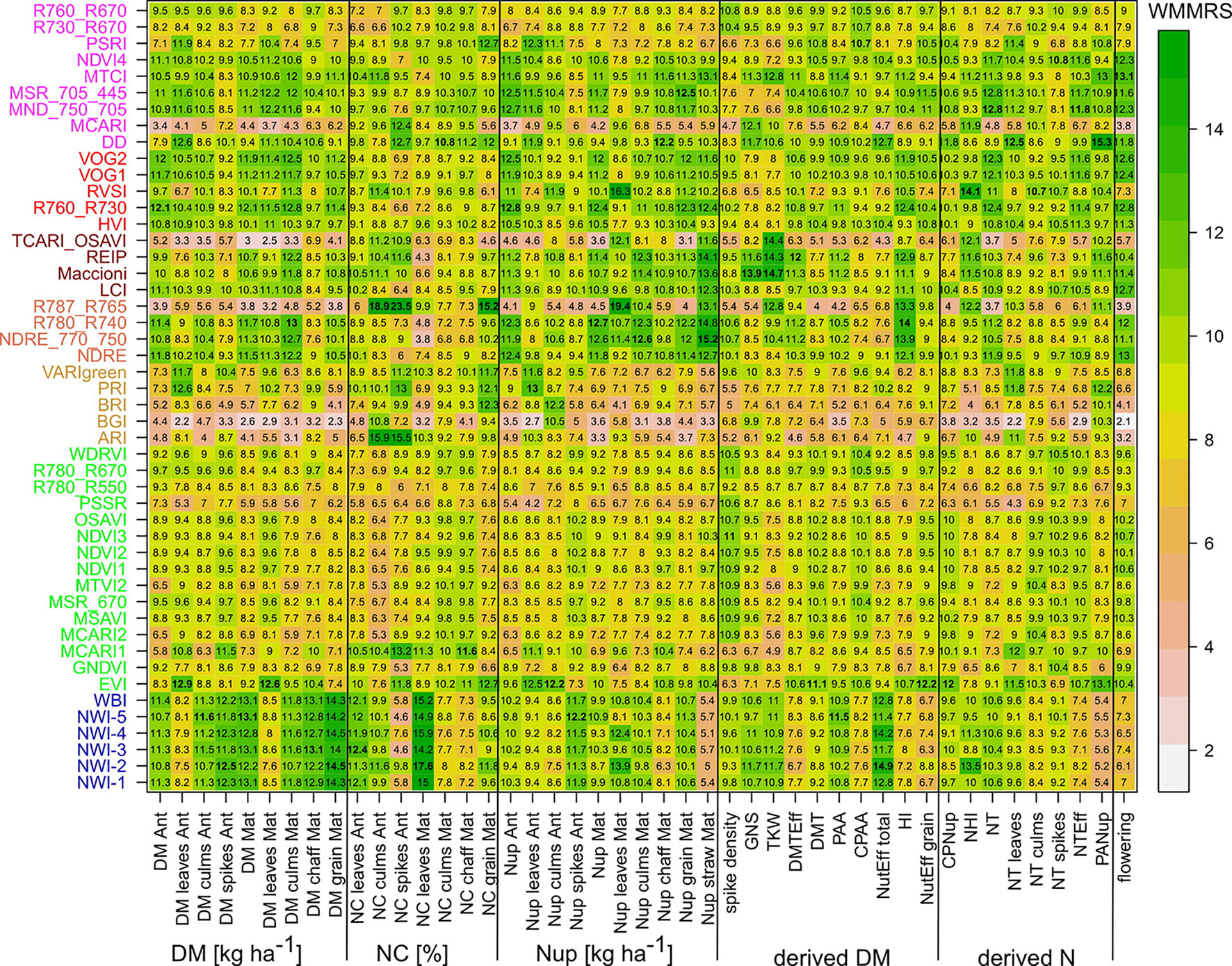
Figure 2 Weighted mean/maximum rank sums (WMMRS) for all SVI/trait combinations. The column-wise and overall WMMRS-mean is 9. A WMMRS > 9 indicates a comparative advantage of an index for a specific trait. WMMRS values are colored from low (white) to high (green) values. For each trait, the highest value is highlighted in bold. For a better comparison in the main range, the color shading for WMMRS > 15 is not differentiated. Indices are colored according to the included spectral regions (Refer to caption of Figure 1 and Supplementary Figure 1).
Direct DM Traits
NIR indices showed a clear advantage for total DM at maturity (Mat; WMMRS > 12; average of all indices = 9; Figure 2) and for grain DM (GY; WMMRS > 14; Figure 2), but performed below-average for DM of leaves both at anthesis and maturity—traits RE-based indices and the EVI (WWMRS > 12) were mostly superior for (Table 4; Figure 3). Noticeably, among the large group of NIR/VIS indices (n = 15), only few indices reached superior WMMRS values. Total DM at anthesis was better estimated in 2015 (WMMRS-index: R760_R730: max. R² = 0.33***; *: p < 0.05; **: p < 0.01; ***: p < 0.001) and 2017 (max. R² = 0.33***) than in 2016 (max. R² = 0.11***; Table 4). A pronounced depression with low R² values is visible for booting in 2016 and for anthesis in 2017 for most traits (Figure 3). Among plant organs at anthesis, DM of leaves was best detected with slightly higher (2015 and 2016) or clearly higher (2017: R² = 0.44; EVI) R² values, as was found for total DM at anthesis (Table 4). While in 2015, indices with RE bands or only NIR bands performed similarly well during milk ripeness for DM traits (Figure 3), all indices with only NIR bands (blue lines) outperformed the other groups on most dates in 2016 and 2017 for total DM and GY. In all years, significant (p < 0.005) relationships were found for GY (grain DM at maturity; Figure 3) although the best R² values of the WMMRS-index NWI-2 (R² = 0.51, 0.26, 0.27) were lower in 2016 and 2017 than those found for total DM at maturity (NWI-5; R² = 0.41, 0.37, 0.34). For both traits, relationships peaked in all years at milk ripeness or early dough ripeness, and the water-related NIR indices (blue lines) excelled the other groups during grain filling and were more consistent over time. In all years, R² values of the related water band indices WBI and NWI-1 were almost identical during grain filling (Supplementary Figure 7). The NIR/RE indices were generally the second best group but failed at the dough stages.
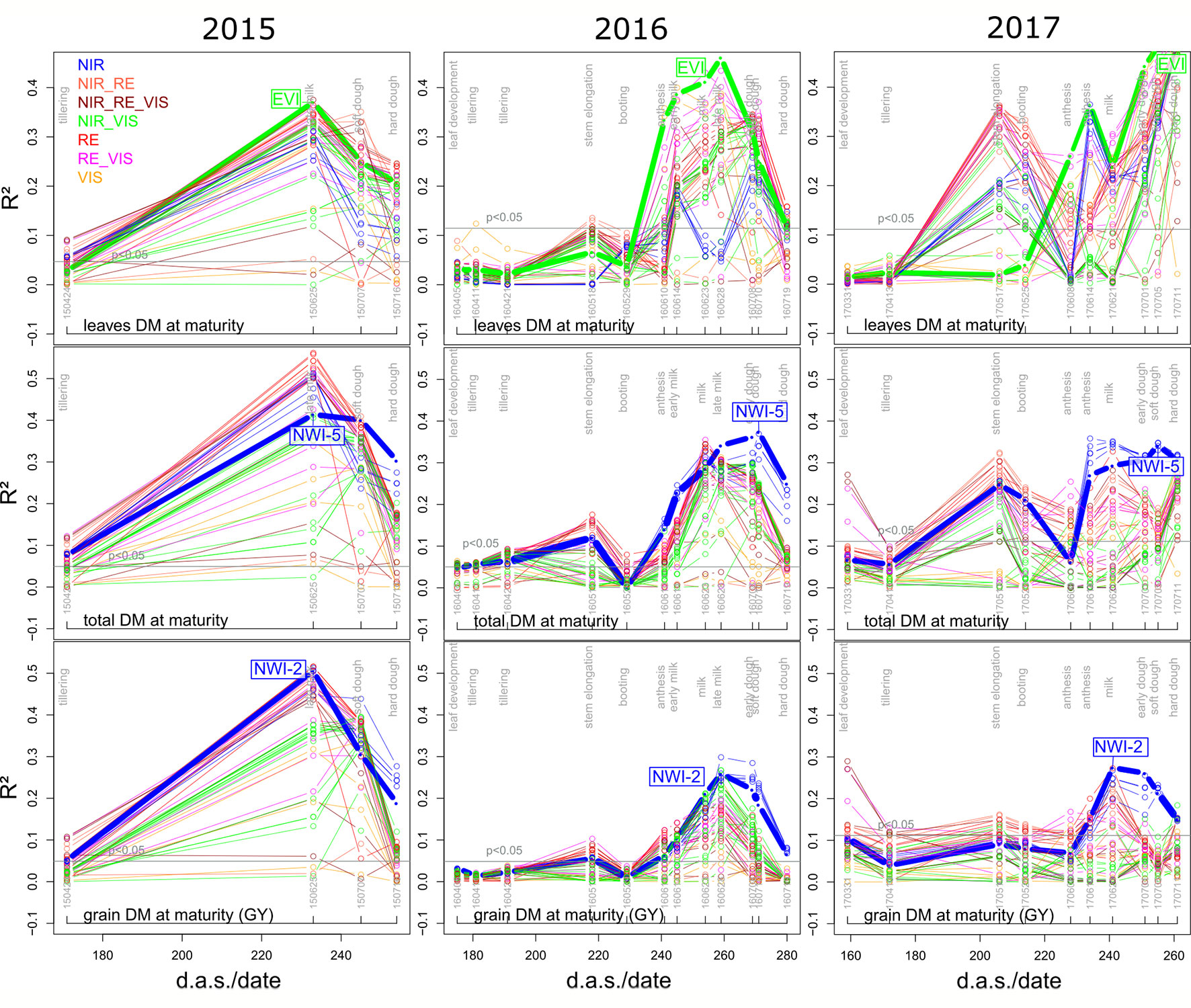
Figure 3 Seasonal coefficients of determination (R²) of selected direct DM traits in the three years for the tested 48 SVI. Index lines are colored according to the included spectral regions (Supplementary Figure 1; Table 3). Horizontal lines indicate the significance thresholds (p < 0.05), differing between years due to the differing number of data points. Thick lines indicate R² values of the labeled rank-based best index per trait (Figure 2; Table 4).
N Concentration Traits
Weak relationships were found for all NC traits, especially at anthesis, and R² values of the WMMRS-indices (Table 4; Figure 1) differed more from the maximum relationships than for other trait groups. The R² values found from the WMMRS-index for grain NC were weak (max. R² = 0.08*) although other SVIs performed clearly better in individual years (Supplementary Figure 4). Relationships with maturity NC traits were closer than with anthesis NC (maximum R² of WMMRS-indices for culms: 0.26*** in 2015, 0.18* in 2016 and 0.22** in 2017; for leaves: 0.32***, 0.42** and 0.21**; Table 4).
N Uptake Traits
N uptake traits were best assessed in 2017, while the relationships were often weaker than for DM traits in the previous years (Table 4).
Leaf and total Nup were better estimated than the Nup of other organs. Both in 2015 and 2017, Nup of leaves at anthesis was best detected from indices of the groups of the RE/VIS, NIR/RE whereas the PRI was identified as best WMMRS index (Figures 4 and 5). As for total DM and grain DM, similar R² curves were observed for total Nup (WMMRS-index R780_740) and GNup (MSR_705_445; Figure 4), but R² values remained higher during dough ripeness for total Nup. For both traits, the group of NIR/RE indices stood out from the others during milk ripeness notably in 2015 and 2017. The detection of the vegetative Nup differed more between years than for DM. Notably, maturity leaf Nup was best detected in 2015 (WMMRS-index R787_R765: R² = 0.28***; Table 4), whereas culm Nup was best detected in 2017 (WMMRS-index NDRE_770_750: R² = 0.38***). Straw Nup is an indicator for the remaining, non-harvested Nup. It was weaker and similarly estimated than total Nup in 2015 and the other years, respectively (Table 4).
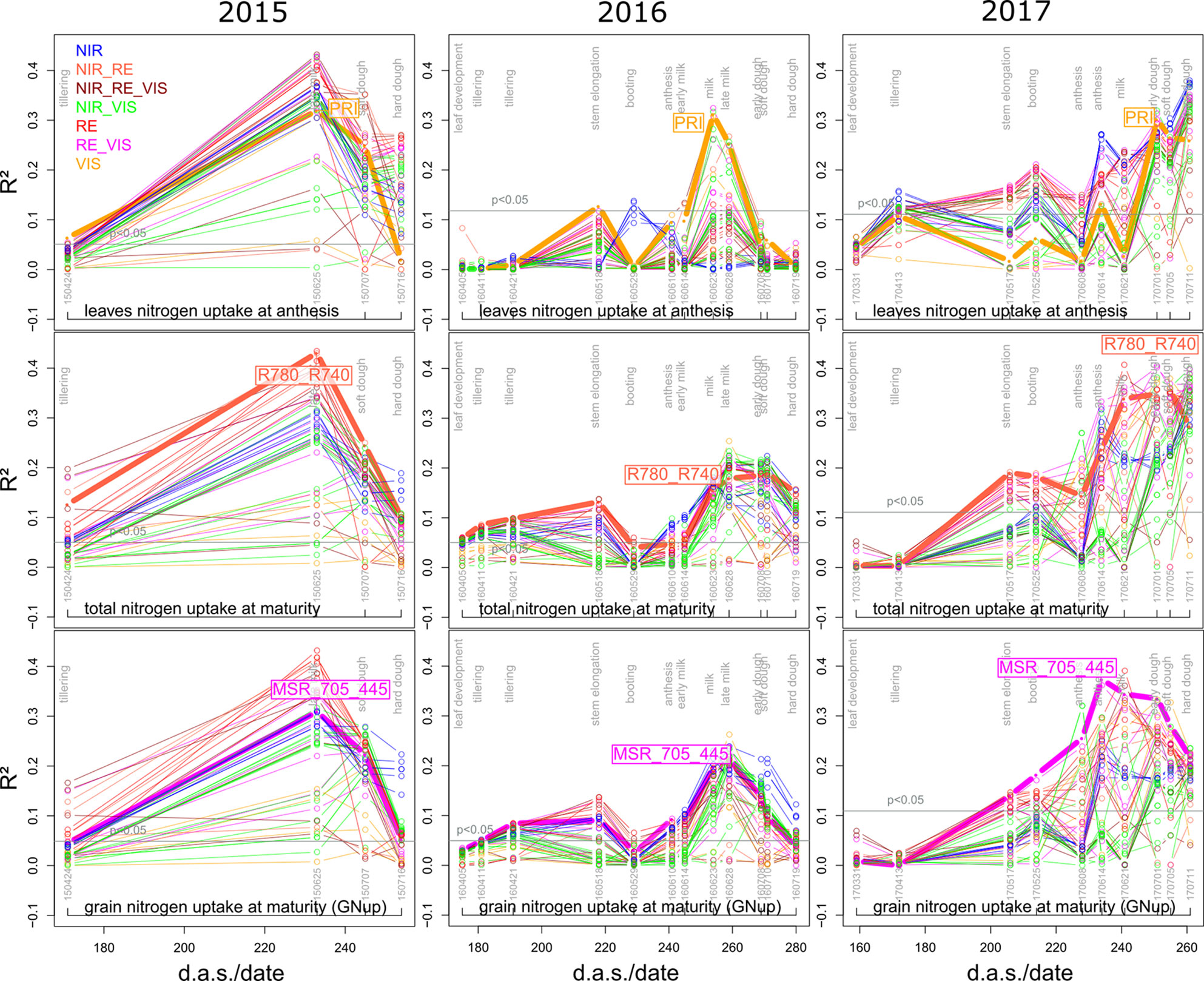
Figure 4 Seasonal coefficients of determination (R²) for selected N uptake (Nup) traits. See the legend of Figure 3 for details.
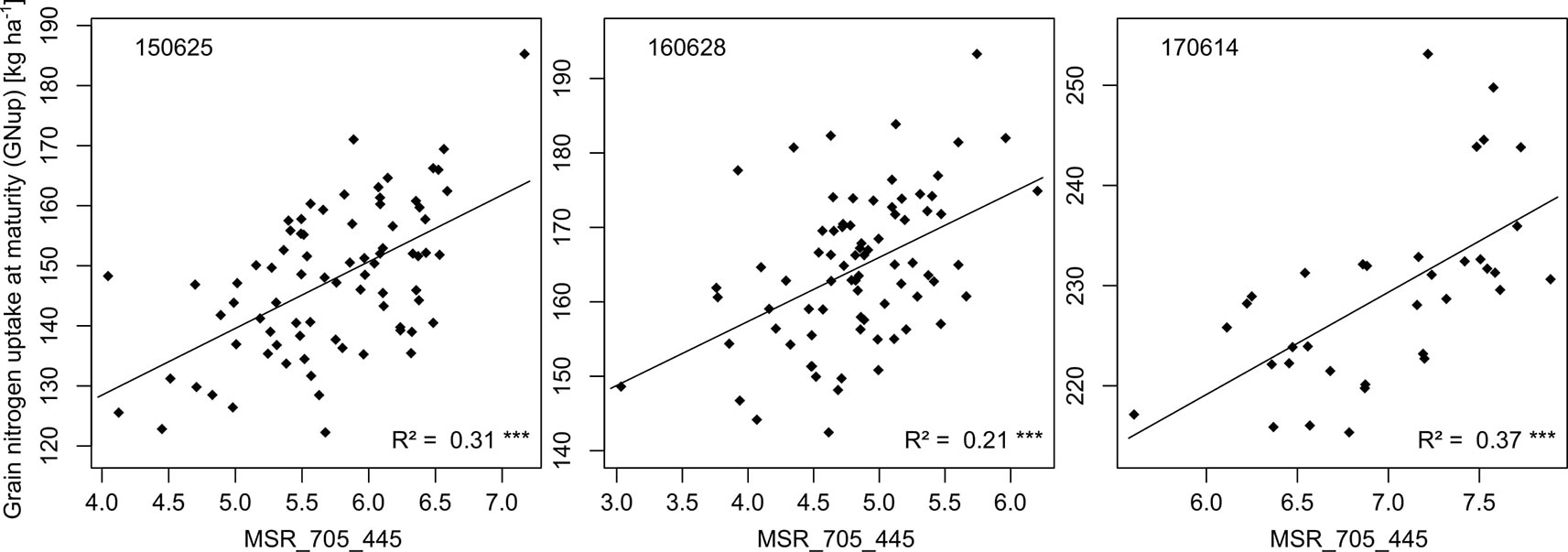
Figure 5 Relationships of the rank-based best index MSR_705_445 (R750-R445)/(R705-R445) with GNup on the most suitable measurements dates.
Derived DM Traits
Among the three yield components spike density, thousand kernel weight and grain number per spike, no consistent relationships were found with SVIs (Table 4). For the DM translocation (DMT; R² = 0.20***) and its efficiency (DMTEff; R² = 0.19***), moderate R² values were found only in 2016. For post-anthesis assimilation (PAA), the WMMRS-index NWI-5 revealed useful relationships during dough ripeness in 2015 (max. R² = 0.23***) and 2016 (R² = 0.34***), but not in 2017. In contrast, the harvest index (HI) was spectrally detected only in 2017 from NIR indices from anthesis on (R² = 0.30***). The total N utilization efficiency (NutEff_total) was best detected by the NWI-2, however with substantially different seasonal curves in the three years (not shown). For grain N utilization efficiency (NutEff_grain), the WMMRS-index EVI provided moderate relationships (R² = 0.21***, 0.14***, 0.21*** in 2015, 2016, and 2017 respectively), which however turned from positive sign in 2015 to negative in the other years (Supplementary Figure 6).
Derived N Traits
As for the DM harvest index, the best relationships for the N harvest index (NHI) were found in 2017, but just like for NutEff_grain, the direction of the relationship was not consistent (Supplementary Figure 6). In 2015 (WMMRS-index MND_750_705: R² = 0.42***) and 2017 (0.21***), N translocation (NT) was better detected than DMT. On the organ-level, NT of leaves was detected best (DD: R² = 0.42***, 0.30***, 0.26***). Unlike post-anthesis N uptake and its contribution to total Nup, and in contrast to DMTEff, N translocation efficiency (NTEff) also yielded moderate relationships, but the direction of the regression line was not consistent (Table 4; Supplementary Figure 6; Supplementary Figure 8). Relations for NTEff peaked later at dough ripeness than for NT. With NT being in close relationship to total Nup at anthesis (r > 0.93 in all years; not shown), the seasonal R² values were similar as for for both traits (Supplementary Figure 8).
Validation of Index and PLSR Models
PLSR models were compared to the WMMRS-based selected index, the NDVI2 and the REIP. Due to the year-specific shifts in the spectral data and the differing seasonality, GY was substantially overestimated in 2015 (Figure 6). In 2017, GY predicted from PLSR models was relatively close at the 1:1 line whereas the index models resulted in substantial underestimations and low slope values. Models calibrated in 2017 overestimated GY in the other years, whereas models calibrated in 2016 over- and underestimated GY in 2015 and 2017, respectively. For GY, the WMMRS-index NWI-2 achieved similar R² values of validation but on average slightly higher RMSE values (RMSE = 1891 kg ha−1; Table 4; Supplementary Table 2) than the PLSR (RMSE = 1609 kg ha−1), while R² values were higher and RMSE values lower than from the NDVI and REIP models in all cases. Refer to Supplementary Table 2 for all validation results and to Supplementary Table 3 for calibration results.
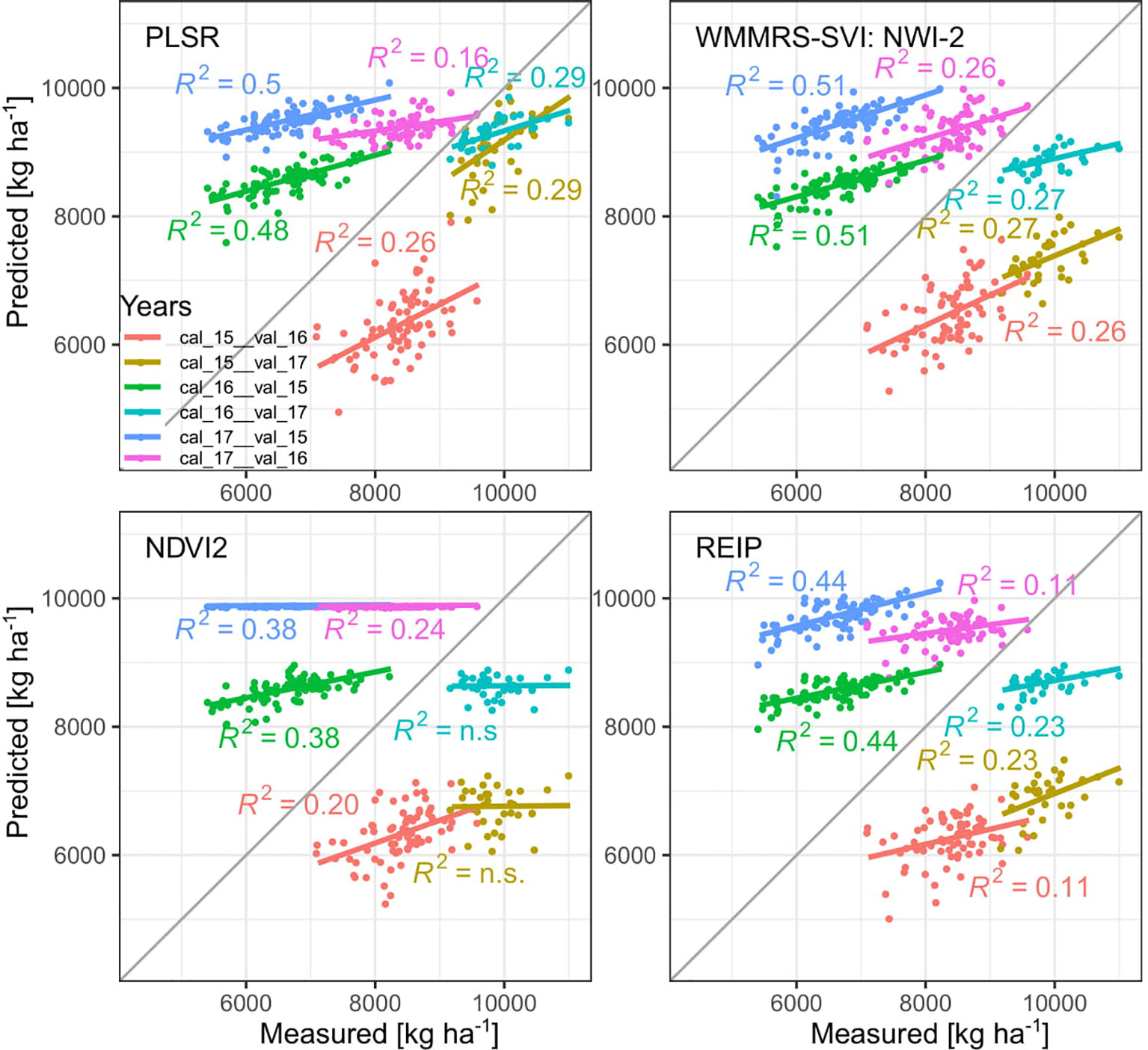
Figure 6 Test set validation results (p < 0.05) across years for GY for PLSR and index models. Cal and val indicate years of calibration and validation, respectively: 2015 (15), 2016 (16) and 2017 (17).
For GNup, the advantage of the WMMRS-index MSR_705_445 (average R² from the six test cases = 0.29; average RMSE = 51 kg N ha−1) was relatively stronger with respect to the NDVI (R² = 0.20; RMSE = 56 kg N ha−1) but less evident with respect to the REIP (R² = 0.30; RMSE = 52 kg N ha−1, Table 4). PLSR reached lower average prediction errors (RMSE = 33 kg N ha−1) but not higher R² values (R² = 0.27). Compared for the averaged validation results (n = 6; Table 4), the WMMRS-indices achieved higher R² values and lower RMSE values than the PLSR models for 29 and 22 of the investigated 45 traits, for 32 and 27 traits compared to the REIP, and for 41 and 35 traits compared to the NDVI, respectively. The strongest improvement over the PLSR models was found for leaf DM at anthesis and maturity (ΔR² = +0.09 and +0.11, respectively; Table 4), leaf NC at maturity (ΔR² = +0.10) as well as total and leaf NT (ΔR² = +0.11 and +0.13). In contrast, PLSR was superior notably for total DM at maturity (ΔR² = +0.07), harvest index (ΔR² = +0.09) and several traits of Nup at maturity.
In addition to optimized PLSR models on derivated spectra, PLSR models were fitted on non-derivated spectra due to the shift through derivation for identifying influential wavebands. For GY, the RE region and the water band beyond about 950 nm showed highest Variable Importance in Projection (VIP) values (VIP > 1; Figure 7), whereas the VIS range was not particularly relevant. A similar pattern was observed for GNup, yet with a higher importance of the RE and a weaker peak at the water band. However, no pronounced RE-peak was observed for GNup in 2016.
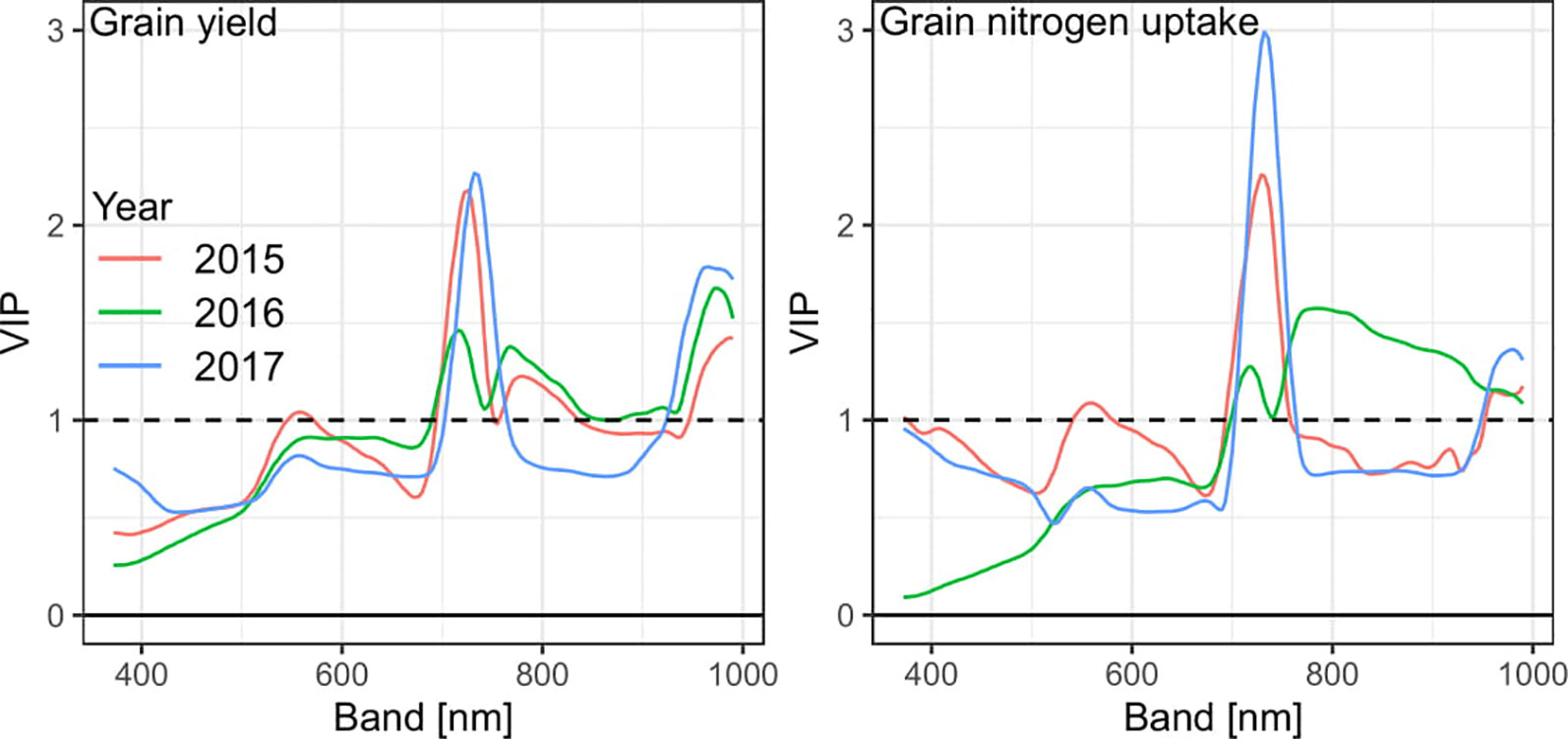
Figure 7 Variable Importance in Projection (VIP) of PLSR models for grain yield and grain nitrogen uptake. See Supplementary Figure 11 for VIP values of all traits.
Discussion
The findings corroborate the possibility of early estimation of GY and GNup in optimum growth stages. Substantial variation in most traits was identified (Prey et al., 2019b), and many traits were significant for explaining plant breeders' main target traits GY, GNup, and grain N concentration (GNC), or are of direct interest. Sufficient heritability (H²) is essential for using SVIs as indirect selection tool. Increasing H² values between the vegetative and grain filling phases are in line with Frels et al. (2018) and may be due to a stronger genetic determination of the senescence process compared to the vegetative growth. The lower H² values in 2015 may be due to the only two replicates in that year, whereas the overall higher values than those found in a nearby experiment (Becker and Schmidhalter, 2017) may be associated with the larger plot size in the present experiment. The lower H² of NIR/VIS indices compared to the water (NIR) indices is in line with Babar et al. (2007) and Becker and Schmidhalter (2017).
Optimum Growth Stages
More measurement dates than in most previous studies were tested in order to identify reliable growth stages. Overall, the grain filling phase was found to be relatively most suitable for all traits, including “post-dictions” of traits related to the anthesis canopy status. The suitability of the milk ripeness stage is in line with previous results (Freeman et al., 2003; Babar et al. 2006a; Gutierrez et al. 2010a; Christopher et al., 2014; Zhang et al., 2019). In contrast, under conditions of drought/heat-induced rapid senescence, post-anthesis assimilation is reduced and early flowering and the translocation of vegetative DM may be an escape-strategy rather than the stay-green trait (Van Herwaarden et al., 1998; Inoue et al., 2004). The canopy status at anthesis may then be more indicative than under prolonged maturation, possibly explaining the relatively better relationships of earlier dates in drier environments (Babar et al. 2006a; Prasad et al. 2007b; Becker and Schmidhalter, 2017), and the contrasting late R²-peaks in 2017, the year with the most favorable ripening conditions. The weak relationships at heading-anthesis are in line with the sensitivity of the spectral signal to the ear emergence (Pimstein et al., 2009).
The Potential of Early Estimation of DM and GY
GY can only be predicted indirectly from spectral readings, which dominantly detect the leaf area, vegetative biomass, chlorophyll, and senescence status (Jacquemoud et al., 2009). The interrelationships of the traits contributing to GY indicated that the major fraction (56–69% in the three years) of GY was formed post-anthesis (Prey et al., 2019b). Moderate correlations were found between GY and total anthesis DM (r = 0.35, 0.43, 0.57***; not shown) in all three years, as well as with most organ-level DM traits at anthesis. In contrast to DM translocation and its efficiency, post-anthesis assimilation correlated with GY (r = 0.71***, 0.42**, and 0.69***) in all three years, explaining the better spectral relationships during grain filling.
The lower R² values in 2016 and 2017 indicate saturation of the spectral signal in dense canopies (Prasad et al., 2007b; Pavuluri et al., 2015; Frels et al., 2018). In the present dataset, the only moderate relationships in 2016 and 2017 still enable to “half” the population without losing the best-yielding genotypes—a “culling tool” strategy that would be relevant to plant breeders (Garriga et al., 2017; Frels et al., 2018) aiming at a visual evaluation of only relevant genotypes or even non-harvesting the others. The relationships were in the same range or closer than in similar studies (Pavuluri et al., 2015; Frels et al., 2018), even though the levels of DM and GY were substantially higher in the present study. With regard to plant organs, the best assessment of leaves is in line with Barmeier and Schmidhalter (2017), which was ascribed to the nadir position of the sensor, since leaves dominate the spectral signal.
Water Band and NIR/VIS Indices for DM and GY
In all years, the water band indices were among the best indices for GY and mostly for total DM but performed less well than most RE-based and NIR/VIS indices for leaf DM. It may be possible that the reflection in the water absorption band is influenced by the water, which is mainly located in culms and—with ongoing grain filling—in kernels, whereas the leaves' appearance dominantly impact the VIS and NIR reflection outside the water absorption band (Haboudane et al., 2004). Given that water band indices ranked relatively high during the late grain filling stages, it is also conceivable that there is a better detection of senescence traits. Total DM (r = 0.90, 0.75, 0.82 in 2015, 2016 and 2017 respectively; not shown) was dominant for explaining GY, whereas the variation in the harvest index was significant (r = 0.56***) only in 2016, explaining the similar index rankings and seasonal patterns for total DM and GY. In all years, GY correlated closer with total DM than with the DM of vegetative organs (r = 0.75 in 2016 and r > 0.82 in the other years; not shown), possibly explaining that the indirect prediction of GY from indices optimized for LAI was less successful.
The constant direction (Figure 8) of the relationships indicates that genotypes keeping canopy water later in the season also reached higher DM formation (Gutierrez et al. 2010a). This “stay-moist” trait was relatively better detected than the stay-green trait, especially in 2017, as seen from the poor performance of the VIS and NIR/VIS indices this year. In addition, water band indices were reported to be less prone to saturation than the NDVI (Sims and Gamon, 2003), corresponding to their stronger relative advantage in the highest-yielding year, 2017. The lower ranking of NIR/VIS indices optimized for LAI (EVI; MCARI1, MCARI2, MTVI2) for GY suggests that structural information that they are able to detect is less relevant for GY than the canopy water status. The present breeding population was morphologically and phenologically diverse—characteristics known to influence the spectral signal (Gutierrez et al., 2015) without direct influence on GY (Prey et al., 2019b). Among NIR/VIS and VIS indices, only the EVI ranked among the best indices for leaf DM traits, but failed for GY. It was reported to saturate less for canopies beyond NDVI values of about 0.80 (Huete et al., 2002), which were clearly exceeded from tillering to milk ripeness. The group of VIS indices ranked clearly below the other groups. Only the VARIgreen reached similar rankings as the NIR/VIS indices, as previously found for DM traits (Erdle et al., 2011).
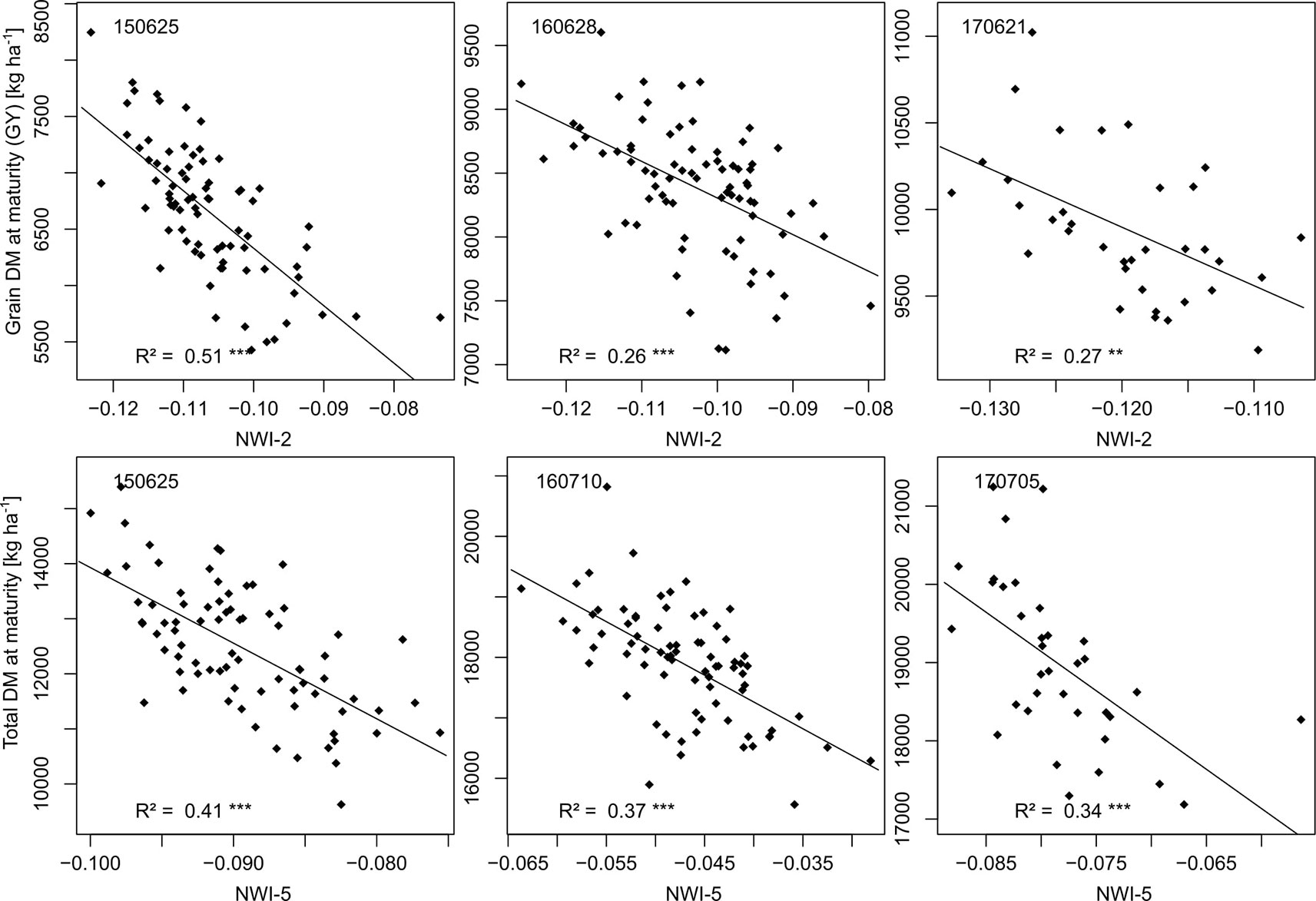
Figure 8 Relationships of the rank-based best indices with GY and total DM at maturity on the most suitable measurements dates (year/month/day). The index equations are (R970-R850)/(R970+R850) [NWI-2] for GY and (R970-R930)/(R970+R930) [NWI-5] for total DM at maturity.
Normalized Difference Versus Simple Ratio Equations
For three pairs of spectral bands, a normalized difference index and a simple ratio version were included each, namely WBI and NWI-1, GNDVI and R780_R550, as well as NDVI2 and R780_R670. For most direct and indirect DM traits, the R780_R550 (on average WMMRS Δ +0.2) and the R780_R670 (on average Δ +0.2), ranked slightly higher than the normalized difference index versions, confirming previous results (Nguy-Robertson et al., 2012; Yu et al., 2012; Barmeier and Schmidhalter, 2017).
RE Indices for DM and GY
Most RE-indices ranked higher than the NIR/VIS indices for most DM traits. The advantage of using wavelengths at the RE, was attributed to increased sensitivities in dense canopies (Nguy-Robertson et al., 2012; Prey and Schmidhalter, 2019a). The RE-indices were also suggested for GY (Pavuluri et al., 2015; Barmeier et al., 2017; Frels et al., 2018). For most dates, the red band used by many NIR/VIS indices was left to the position, where the reflectance difference between plots of maximum and minimum GY was most negative (Supplementary Figure 10). It is possible that most NIR/RE and RE indices reached higher sensitivity by positioning their lower band in this range, whereas their NIR bands were similarly positioned as those of the NIR/VIS indices, beyond approximately 760 nm at the “NIR-plateau” with similar reflectance differences, as supported by the influential bands in the PLSR models.
Derived DM Traits
No consistently useful estimations were achieved for the yield components, possibly because these traits were rarely correlated to GY (Prey et al., 2019b). The mostly lower coefficients of determination found for DMT compared to NT may be explained by the stronger variation in DMTEff than in NTEff. The HI was only well discriminated (R² = 0.30***) in 2017, the year when HI and total DM correlated negatively, thus indicating only indirect relationships through the detection of DM. Similarly, NutEff_grain showed negative relationships with the EVI greenness index in 2017 and 2016, but a positive relationship in 2015. In contrast, the regression of NutEff_total with its best index NWI-2 did not turn in direction, which is in line with the NutEff_total's positive correlations with total DM in all years. In contrast, Erdle et al. (2013a) found good relationships for the HI at milk ripeness, however for fewer cultivars. For NutEff_grain, Frels et al. (2018) found good relationships (max. R² = 0.41) already at heading in one year, but weaker relationships than in the present study in another year.
The Estimation of N Traits
The usefulness of RE bands for N-related traits is well established and was related to the rightward-shift of the RE position with increasing N status (Guyot et al., 1988; Boochs et al., 1990; Guo et al., 2017) and—as for GY—the higher sensitivity in dense canopies (Erdle et al., 2011; Zhang et al., 2019). The higher ranking of most RE, NIR/RE, and NIR/RE/VIS indices may be associated with the placement of the lower band in the lower RE at 700–750 nm (Supplementary Figure 10) (Datt, 1999), whereas the RE/VIS indices use mostly similar red bands as the NIR/VIS indices. The results are in line with the Maccioni index that was suggested for GNup and total Nup efficiency (Frels et al., 2018), the R780_740 for detecting total Nup and for NUE (Pavuluri et al., 2015), the R760_R730 for spike Nup (Erdle et al., 2013a), as well as the NDRE_770_750 (Prey and Schmidhalter, 2019b) and the REIP (Prey et al., 2018) for GNup—all indices that ranked high for many Nup traits.
The similar best growth stages for predicting GNup just like for GY is in line with the coupling of both traits (r = 0.86, 0.66 and 0.64 in 2015, 2016 and 2017, respectively; not shown). The only date- and SVI-specific relationships found within years for GNC indicate that the formation of GNC was highly influenced by the year-specific growing conditions. Thus, the negative relationship (R² = 0.24***) found between the EVI index and GNC at milk ripeness in 2015 and the positive relationship between the senescence index PSRI and GNC in 2017 (R² = 0.18***) indicate that due to the GY/GNC antagonism, late canopy greenness was promoting GY (positive relationship with EVI, R² = 0.34***), but reducing GNC.
As the HI for GY, the NHI was secondary for explaining GNup (Prey et al., 2019b), and GNup was therefore closely correlated with total Nup (r > 0.93 in all years). This explains that the seasonal R² patterns and the index rankings were comparable, similarly as reported by Frels et al. (2018). In contrast to Frels et al. (2018), post-anthesis Nup was not sufficiently estimated (max. R² = 0.16***), even if it correlated positively with GNup. However, total N translocation, which was the dominating fraction for GNup in all years, revealed useful relationships during grain filling in 2015 and 2017 due to its close correlations with total Nup at anthesis. The weaker detection of vegetative Nup at maturity in organs and in the straw than of total and grain Nup may be due to the low absolute residual Nup, as well as the differing influence of the organ-level NTEff. The weak detection of N concentration (NC) traits at anthesis does not allow the recommendation of optimum indices. At maturity, moderate NC estimations were possible only for the vegetative organs but the indices previously optimized for leaf chlorophyll, TCARI_OSAVI (Haboudane et al., 2002; Huang et al., 2011), and MCARI (Daughtry et al., 2000), or for NC (R787_R765), ranked never among the best indices, thus indicating rather indirect relationships.
Index Validation and PLSR
The comparison of the WMMRS-SVIs to the “reference” SVIs NDVIs and REIP in the year-to year test set validation models supports the usefulness of the seasonal rank-based SVI selection. The NDVI, which, despite its known limitations, is still widely used, was clearly outperformed for the vast majority of the traits by the REIP, the PLSR models, and the WMMRS-indices, confirming the results observed in the individual years. The relative advantage of the WMMRS-indices over the REIP was confirmed for GY and other DM traits, but was less pronounced for most N traits.
The often lacking or weak R²-improvements from PLSR models indicate that optimized selection of SVIs can compete with multivariate models and may be preferred in terms of calibration effort, the transferability to simpler, multispectral sensors and applicability by breeders. Thus, PLSR suggested substantial improvements in the calibration (Supplementary Table 3), which however largely dwindled in the validation (Table 4). While the relative discrimination will often be sufficient in phenotyping (Garriga et al., 2017), lower RMSE values of PLSR for several traits indicate a higher robustness over year- and growth stage-specific shifts in the spectral data, being in line with results on barley (Barmeier et al., 2017; Barmeier and Schmidhalter, 2017). Unlike to the latter study, the year-based calibrations in the present study were relatively more useful than pre-evaluated across-years models (not shown), but the validation results were generally weaker due to testing only on individual years' data. The influential bands in the PLSR confirm the RE and water bands to be most indicative.
Conclusions
For most plant traits including GY and GNup, the milk ripeness stage was the most reliable under conditions of moderate terminal heat/drought or pathogen stress, whereas the relationships were more stable during dough ripeness in the year with favorable senescing conditions (2017). In contrast, phenological shifts at heading/anthesis appeared to decrease the relationship in this phase. NIR-combinations exploiting the water absorption band at 970 nm were found to be indispensable to achieve a useful discrimination in GY in dense canopies, followed by NIR/RE combinations, which mostly outperformed the NIR/VIS indices including the NDVI. For GNup, simple NIR/RE indices ranked high and clearly better than the NDVI. Relationships of indices with GY and GNup were explained by the detection of total DM and Nup, respectively, rather than by that of the relative allocation (harvest index) to the grain. The validation of the selected indices confirms the usefulness of the rank-based index selection notably for overcoming limitations of the NDVI. The PLSR did not achieve clearly higher R² values, but often lower estimation errors, thus that it should be preferred for improving prediction accuracies, whereas optimized SVIs appear sufficient for a relative discrimination of important traits. GNC was not reliably predicted. DM and N traits related to maturity canopy status were detected better than anthesis traits. The screening for useful band combinations can be used for optimizing sensor configurations. The results could also be transferred to multispectral sensors, thus improving the transfer of the evaluated methods to the application in breeding nurseries.
Data Availability Statement
The datasets generated for this study are available on request to the corresponding author.
Author Contributions
YH, LP, and US conceived and designed the experiments. LP performed the experiments. LP analyzed the data. LP and US wrote the paper.
Funding
This research was funded by the DFG (German Research Foundation)-funded project SCHM 1456/6-1 and was partly supported by funds of the Federal Ministry of Food and Agriculture (BMEL) under the innovation support program for the project 28-1-B3.030-16.
Conflict of Interest
The authors declare that the research was conducted in the absence of any commercial or financial relationships that could be construed as a potential conflict of interest.
Acknowledgments
The authors are grateful to Lorenz Hartl for providing the genotypes and to Jürgen Plass for technical support with spectral measurements. The authors gratefully acknowledge valuable suggestions by both reviewers.
Supplementary Material
The Supplementary Material for this article can be found online at: https://www.frontiersin.org/articles/10.3389/fpls.2019.01672/full#supplementary-material
References
Acquaah, G. (2007). Principles of plant genetics and breeding (Chichester: John Wiley & Sons). doi: 10.1016/S0034-4257(96)00072-7
Aparicio, N., Villegas, D., Casadesus, J., Araus, J. L., Royo, C. (2000). Spectral vegetation indices as nondestructive tools for determining durum wheat yield. Agron. J. 92, 83. doi: 10.2134/agronj2000.92183x
Araus, J. L., Cairns, J. E. (2014). Field high-throughput phenotyping: the new crop breeding frontier. Trends Plant Sci. 19, 52–61. doi: 10.1016/j.tplants.2013.09.008
Babar, M. A., Reynolds, M. P., Van Ginkel, M., Klatt, A. R., Raun, W. R., Stone, M. L. (2006a). Spectral reflectance indices as a potential indirect selection criteria for wheat yield under irrigation. Crop Sci. 46, 578–588. doi: 10.2135/cropsci2005.0059
Babar, M. A., van Ginkel, M., Klatt, A. R., Prasad, B., Reynolds, M. P. (2006b). The potential of using spectral reflectance indices to estimate yield in wheat grown under reduced irrigation. Euphytica 150, 155–172. doi: 10.1007/s10681-006-9104-9
Babar, M. A., Van Ginkel, M., Reynolds, M. P., Prasad, B., Klatt, A. R. (2007). Heritability, correlated response, and indirect selection involving spectral reflectance indices and grain yield in wheat. Aust. J. Agric. Res. 58, 432–442. doi: 10.1071/AR06270
Barmeier, G., Schmidhalter, U. (2017). high-throughput field phenotyping of leaves, leaf sheaths, culms and ears of spring barley cultivars at anthesis and dough ripeness. Front. Plant Sci. 8, 1–16. doi: 10.3389/fpls.2017.01920
Barmeier, G., Mistele, B., Schmidhalter, U. (2016). Referencing laser and ultrasonic height measurements of barley cultivars by using a herbometre as standard. Crop Pasture Sci. 67, 1215. doi: 10.1071/CP16238
Barmeier, G., Hofer, K., Schmidhalter, U. (2017). Mid-season prediction of grain yield and protein content of spring barley cultivars using high-throughput spectral sensing. Eur. J. Agron. 90, 108–116. doi: 10.1016/j.eja.2017.07.005
Barnes, E. M., Clarke, T. R., Richards, S. E., Colaizzi, P. D., Haberland, J., Kostrzewski, M., et al. (2000). “Coincident detection of crop water stress, nitrogen status and canopy density using ground based multispectral data,” in Proceedings of the Fifth International Conference on Precision Agriculture, Bloomington, MN, USA, 16–19.
Becker, E., Schmidhalter, U. (2017). Evaluation of yield and drought using active and passive spectral sensing systems at the reproductive stage in wheat. Front. Plant Sci. 8, 379. doi: 10.3389/fpls.2017.00379
Boochs, F., Kupfer, G., Dockter, K., Kühbauch, W. (1990). Shape of the red edge as vitality indicator for plants. Int. J. Remote Sens. 11, 1741–1753. doi: 10.1080/01431169008955127
Broge, N. H., Leblanc, E. (2001). Comparing prediction power and stability of broadband and hyperspectral vegetation indices for estimation of green leaf area index and canopy chlorophyll density. Remote Sens. Environ. 76, 156–172. doi: 10.1016/S0034-4257(00)00197-8
Chen, J. M. (1996). Evaluation of vegetation indices and a modified simple ratio for boreal applications. Can. J. Remote Sens. 22, 229–242. doi: 10.1080/07038992.1996.10855178
Christopher, J. T., Veyradier, M., Borrell, A. K., Harvey, G., Fletcher, S., Chenu, K. (2014). Phenotyping novel stay-green traits to capture genetic variation in senescence dynamics. Funct. Plant Biol. 41, 1035–1048. doi: 10.1071/FP14052
Cristiano, P. M., Posse, G., Di Bella, C. M., Jaimes, F. R. (2010). Uncertainties in fPAR estimation of grass canopies under different stress situations and differences in architecture. Int. J. Remote Sens. 31, 4095–4109. doi: 10.1080/01431160903229192
Dash, J., Curran, P. J. (2003). “The MERIS terrestrial chlorophyll index” in Proceedings of MERIS User Workshop (Frascati, Italy).
Datt, B. (1999). A new reflectance index for remote sensing of chlorophyll content in higher plants: tests using Eucalyptus leaves. J. Plant Physiol. 154, 30–36. doi: 10.1016/S0176-1617(99)80314-9
Daughtry, C. S. T., Walthall, C. L., Kim, M. S., Brown de Colstoun, E., McMurtrey, J. (2000). Estimating Corn Leaf Chlorophyll Concentration from Leaf and Canopy Reflectance. Remote Sens. Environ 74, 229–239. doi: 10.1016/S0034-4257(00)00113-9
Erdle, K., Mistele, B., Schmidhalter, U. (2011). Comparison of active and passive spectral sensors in discriminating biomass parameters and nitrogen status in wheat cultivars. F. Crop Res. 124, 74–84. doi: 10.1016/j.fcr.2011.06.007
Erdle, K., Mistele, B., Schmidhalter, U. (2013a). Spectral high-throughput assessments of phenotypic differences in biomass and nitrogen partitioning during grain filling of wheat under high yielding Western European conditions. F. Crop Res. 141, 16–26. doi: 10.1016/j.fcr.2012.10.018
Erdle, K., Mistele, B., Schmidhalter, U. (2013b). Spectral assessments of phenotypic differences in spike development during grain filling affected by varying N supply in wheat. J. Plant Nutr. Soil Sci. 176, 952–963. doi: 10.1002/jpln.201300247
Fava, F., Colombo, R., Bocchi, S., Meroni, M., Sitzia, M., Fois, N., et al. (2009). Identification of hyperspectral vegetation indices for mediterranean pasture characterization. Int. J. Appl. Earth Obs. Geoinf. 11, 233–243. doi: 10.1016/j.jag.2009.02.003
Feng, W., Zhang, H.-Y., Zhang, Y.-S., Qi, S.-L., Heng, Y.-R., Guo, B.-B., et al. (2016). Remote detection of canopy leaf nitrogen concentration in winter wheat by using water resistance vegetation indices from in-situ hyperspectral data. F. Crop Res. 198, 238–246. doi: 10.1016/j.fcr.2016.08.023
Freeman, K. W., Raun, W. R., Johnson, G. V., Mullen, R. W., Stone, M. L., Solie, J. B. (2003). Late-season prediction of wheat grain yield and grain protein. Commun. Soil Sci. Plant Anal. 34, 1837–1852. doi: 10.1081/CSS-120023219
Frels, K., Guttieri, M., Joyce, B., Leavitt, B., Baenziger, P. S. (2018). Evaluating canopy spectral reflectance vegetation indices to estimate nitrogen use traits in hard winter wheat. F. Crop Res. 217, 82–92. doi: 10.1016/j.fcr.2017.12.004
Furbank, R. T., Tester, M. (2011). Phenomics – technologies to relieve the phenotyping bottleneck. Trends Plant Sci. 16, 635–644. doi: 10.1016/j.tplants.2011.09.005
Garriga, M., Romero-bravo, S., Estrada, F., Escobar, A., Matus, I. A., del Pozo, A., et al. (2017). Assessing Wheat Traits by Spectral Reflectance: Do We Really Need to Focus on Predicted Trait-Values or Directly Identify the Elite Genotypes? Front. Plant Sci. 8, 280. doi: 10.3389/fpls.2017.00280
Gitelson, A., Merzlyak, M. N. (1994). Quantitative estimation of chlorophyll-a using reflectance spectra: Experiments with autumn chestnut and maple leaves. J. Photochem. 22, 247–252. doi: 10.1016/1011-1344(93)06963-4
Gitelson, A., Kaufman, Y. J., Merzlyak, M. N. (1996a). Use of a green channel in remote sensing of global vegetation from EOS-MODIS. Remote Sens. Environ. 58, 289–298. doi: 10.1016/S0034-4257(96)00072-7
Gitelson, A., Merzlyak, M., Lichtenthaler, H. (1996b). Detection of Red Edge Position and Chlorophyll Content by Reflectance Measurements Near 700 nm. J. Plant Physiol. 148, 501–508. doi: 10.1016/S0176-1617(96)80285-9
Gitelson, A. A., Merzlyak, M. N., Chivkunova, O. B. (2001). Optical properties and nondestructive estimation of anthocyanin content in plant leaves. Photochem. Photobiol. 74, 38–45. doi: 10.1562/0031-8655
Gitelson, A. A., Kaufman, Y. J., Stark, R., Rundquist, D. (2002). Novel algorithms for remote estimation of vegetation fraction. Remote Sens. Environ. 80, 76–87. doi: 10.1016/S0034-4257(01)00289-9
Gitelson, A. A. (2004). Wide dynamic range vegetation index for remote quantification of biophysical characteristics of vegetation. J. Plant Physiol. 161, 165–173. doi: 10.1078/0176-1617-01176
Gizaw, S. A., Garland-Campbell, K., Carter, A. H. (2016a). Evaluation of agronomic traits and spectral reflectance in Pacific Northwest winter wheat under rain-fed and irrigated conditions. F. Crop Res. 196, 168–179. doi: 10.1016/j.fcr.2016.06.018
Gizaw, S. A., Garland-Campbell, K., Carter, A. H. (2016b). Use of spectral reflectance for indirect selection of yield potential and stability in Pacific Northwest winter wheat. F. Crop Res. 196, 199–206. doi: 10.1016/j.fcr.2016.06.022
Guo, B. B., Qi, S. L., Heng, Y. R., Duan, J. Z., Zhang, H. Y., Wu, Y. P., et al. (2017). Remotely assessing leaf N uptake in winter wheat based on canopy hyperspectral red-edge absorption. Eur. J. Agron. 82, 113–124. doi: 10.1016/j.eja.2016.10.009
Gutierrez, M., Reynolds, M., Klatt, A. (2010a). Association of water spectral indices with plant and soil water relations in contrasting wheat genotypes. J. Exp. Bot. 61, 3291–3303. doi: 10.1093/jxb/erq156
Gutierrez, M., Reynolds, M. P., Raun, W. R., Stone, M. L., Klatt, A. R. (2010b). Spectral water indices for assessing yield in elite bread wheat genotypes under well-irrigated, water-stressed, and high-temperature conditions. Crop Sci. 50, 197–214. doi: 10.2135/cropsci2009.07.0381
Gutierrez, M., Reynolds, M. P., Klatt, A. R. (2015). Effect of leaf and spike morphological traits on the relationship between spectral reflectance indices and yield in wheat. Int. J. Remote Sens. 36, 701–718. doi: 10.1080/01431161.2014.999878
Guyot, G., Baret, F., Major, D. J. (1988). High spectral resolution: Determination of spectral shifts between the red and infrared. Int. Arch. Photogramm. Remote Sens. 11, 750–760.
Haboudane, D., Miller, J. R., Tremblay, N., Zarco-Tejada, P. J., Dextraze, L. (2002). Integrated narrow-band vegetation indices for prediction of crop chlorophyll content for application to precision agriculture. Remote Sens. Environ. 81, 416–426. doi: 10.1016/S0034-4257(02)00018-4
Haboudane, D., Miller, J. R., Pattey, E., Zarco-Tejada, P. J., Strachan, I. B. (2004). Hyperspectral vegetation indices and novel algorithms for predicting green LAI of crop canopies: modeling and validation in the context of precision agriculture. Remote Sens. Environ. 90, 337–352. doi: 10.1016/j.rse.2003.12.013
Hansen, P. M., Schjoerring, J. K. (2003). Reflectance measurement of canopy biomass and nitrogen status in wheat crops using normalized difference vegetation indices and partial least squares regression. Remote Sens. Environ. 86, 542–553. doi: 10.1016/S0034-4257(03)00131-7
Henrich, V., Krauss, G., Götze, C., Sandow, C. (2012). “Entwicklung einer Datenbank für Fernerkundungsindizes Einleitung und Methode Arbeiten mit der IDB,” in AK Fernerkundung, 4.-5., 10 2012. Bochum.
Huang, W., Wang, Z., Huang, L., Lamb, D. W., Ma, Z., Zhang, J., et al (2011). Estimation of vertical distribution of chlorophyll concentration by bi-directional canopy reflectance spectra in winter wheat. Precis. Agric. 12, 165–178. doi: 10.1007/s11119-010-9166-5
Huete, A., Didan, K., Miura, T., Rodriguez, E. P., Gao, X., Ferreira, L. G. (2002). Overview of the radiometric and biophysical performance of the MODIS vegetation indices. Remote Sens. Environ. 83, 195–213. doi: 10.1016/S0034-4257(02)00096-2
Inoue, T., Inanaga, S., Sugimoto, Y., El Siddig, K. (2004). Contribution of pre-anthesis assimilates and current photosynthesis to grain yield, and their relationships to drought resistance in wheat cultivars grown under different soil moisture. Photosynthetica 42, 99–104. doi: 10.1023/B:PHOT.0000040576.52128.ed
Jacquemoud, S., Verhoef, W., Baret, F., Bacour, C., Zarco-Tejada, P. J., Asner, G. P., et al. (2009). Prospect + sail models: a review of use for vegetation characterization. Remote Sens. Environ. 113, S56–S66. doi: 10.1016/j.rse.2008.01.026
Jasper, J., Reusch, S., Link, A. (2009). “Active sensing of the N status of wheat using optimized wavelength combination: impact of seed rate, variety and growth stage,” in Precision Agriculture. Seventh European Conference on Precision Agriculture, (Wageningen, the Netherlands: Wageningen Academic Publishers) 23–30.
Kipp, S., Mistele, B., Schmidhalter, U. (2014). The performance of active spectral reflectance sensors as influenced by measuring distance, device temperature and light intensity. Comput. Electron. Agric. 100, 24–33. doi: 10.1016/j.compag.2013.10.007
Le Maire, G., Francois, C., Dufrene, E., Maire, G., Franc, C., Dufre, E., et al (2004). Towards universal broad leaf chlorophyll indices using PROSPECT simulated database and hyperspectral reflectance measurements. Remote Sens. Environ. 89, 1–28. doi: 10.1016/j.rse.2003.09.004
Li, F., Miao, Y., Hennig, S. D., Gnyp, M. L., Chen, X., Jia, L., et al (2010). Evaluating hyperspectral vegetation indices for estimating nitrogen concentration of winter wheat at different growth stages. Precis. Agric. 11, 335–357. doi: 10.1007/s11119-010-9165-6
Li, F., Mistele, B., Hu, Y., Chen, X., Schmidhalter, U. (2013). Comparing hyperspectral index optimization algorithms to estimate aerial N uptake using multi-temporal winter wheat datasets from contrasting climatic and geographic zones in China and Germany. Agric. For. Meteorol. 180, 44–57. doi: 10.1016/j.agrformet.2013.05.003
Li, F., Mistele, B., Hu, Y., Chen, X., Schmidhalter, U. (2014). Optimising three-band spectral indices to assess aerial N concentration, N uptake and aboveground biomass of winter wheat remotely in China and Germany. ISPRS J. Photogramm. Remote Sens 92, 112–123. doi: 10.1016/j.isprsjprs.2014.03.006
Maccioni, A., Agati, G., Mazzinghi, P. (2001). New vegetation indices for remote measurement of chlorophylls based on leaf directional reflectance spectra. J. Photochem. Photobiol. 61, 52–61. doi: 10.1016/S1011-1344(01)00145-2
Mehmood, T., Liland, K. H., Snipen, L., Sæbø, S. (2012). A review of variable selection methods in Partial Least Squares Regression. Chemom. Intell. Lab. Syst. 118, 62–69. doi: 10.1016/j.chemolab.2012.07.010
Merton, R. N. (1998). “Monitoring community hysteresis using spectral shift analysis and the red-edge vegetation stress index,” in Proceedings of the Seventh Annual JPL Airborne Earth Science Workshop (Pasadena, California, USA), 12–16.
Mevik, B. H., Wehrens, R. (2007). The pls package: Principal component and partial least squares regression in R. J. Stat. Softw. 18, 1–23. doi: 10.1159/000323281
Mistele, B., Schmidhalter, U. (2008a). Estimating the nitrogen nutrition index using spectral canopy reflectance measurements. Eur. J. Agron. 29, 184–190. doi: 10.1016/j.eja.2008.05.007
Mistele, B., Schmidhalter, U. (2008b). Spectral measurements of the total aerial N and biomass dry weight in maize using a quadrilateral-view optic. F. Crop Res. 106, 94–103. doi: 10.1016/j.fcr.2007.11.002
Mistele, B., Schmidhalter, U. (2010). Tractor-based quadrilateral spectral reflectance measurements to detect biomass and total aerial nitrogen in winter wheat. Agron. J. 102, 499–506. doi: 10.2134/agronj2009.0282
Mistele, B., R., G., U., S. (2004). “Validation of field-scales spectral measurements of the nitrogen status in winter wheat,” in Program Book of the Joint Conference ICPA (Minneapolis, USA), 1187–1195.
Moges, S. M., Raun, W. R., Mullen, R. W., Freeman, K. W., Johnson, G. V., Solie, J. B. (2004). Evaluation of green, red, and near infrared bands for predicting winter wheat biomass, nitrogen uptake, and final grain yield. J. Plant Nutr. 27, 1431–1441. doi: 10.1081/PLN-200025858
Nguyen, G. N., Kant, S. (2018). Improving nitrogen use efficiency in plants: effective phenotyping in conjunction with agronomic and genetic approaches. Funct. Plant Biol. 45, 606–619. doi: 10.1071/FP17266
Nguy-Robertson, A., Gitelson, A., Peng, Y., Viña, A., Arkebauer, T., Rundquist, D. (2012). Green leaf area index estimation in maize and soybean: Combining vegetation indices to achieve maximal sensitivity. Agron. J. 104, 1336–1347. doi: 10.2134/agronj2012.0065
Oehlschläger, J., Schmidhalter, U., Noack, P. O. (2018). “UAV-based hyperspectral sensing for yield prediction in winter barley”, in Ninth Workshop on Hyperspectral Image and Signal Processing: Evolution in Remote Sensing (WHISPERS), (IEEE), 1–4.
Overgaard, S. I., Isaksson, T., Korsaeth, A. (2013). Prediction of wheat yield and protein using remote sensors on plots - Part II: Improving prediction ability using data fusion. J. Near Infrared Spectrosc. 21, 133–140. doi: 10.1255/jnirs.1044
Pavuluri, K., Chim, B. K., Griffey, C. A., Reiter, M. S., Balota, M., Thomason, W. E. (2015). Canopy spectral reflectance can predict grain nitrogen use efficiency in soft red winter wheat. Precis. Agric. 16, 405–424. doi: 10.1007/s11119-014-9385-2
Peñuelas, J., Filella, I., Biel, C., Serrano, L., Savé, R., Save, R. (1993). The reflectance at the 950–970 nm region as an indicator of plant water status. Int. J. Remote Sens. 14, 1887–1905. doi: 10.1080/01431169308954010
Peñuelas, J., Filella, I., Gamon, J. A. (1995). Assessment of photosynthetic radiation-use efficiency with spectral reflectance. New Phytol. 131, 291–296. doi: 10.1111/j.1469-8137.1995.tb03064.x
Pearson, R. L., Miller, L. D. (1972). Remote mapping of standing crop biomass for estimation of the productivity of the shortgrass prairie, in Remote Sensing of Environment, VIII, 1355.
Pimstein, A., Eitel, J. U. H., Long, D. S., Mufradi, I., Karnieli, A., Bonfil, D. J. (2009). A spectral index to monitor the head-emergence of wheat in semi-arid conditions. F. Crop Res. 111, 218–225. doi: 10.1016/j.fcr.2008.12.009
Prasad, B., Carver, B. F., Stone, M. L., Babar, M. A., Raun, W. R., Klatt, A. R. (2007a). Genetic Analysis of Indirect Selection for Winter Wheat Grain Yield Using Spectral Reflectance Indices. Crop Sci. 47, 1416. doi: 10.2135/cropsci2006.08.0546
Prasad, B., Carver, B. F., Stone, M. L., Babar, M. A., Raun, W. R., Klatt, A. R. (2007b). Potential use of spectral reflectance indices as a selection tool for grain yield in winter wheat under great plains conditions. Crop Sci. 47, 1426–1440. doi: 10.2135/cropsci2006.07.0492
Prey, L., Schmidhalter, U. (2019a). Sensitivity of Vegetation Indices for estimating vegetative n status in winter wheat. Sensors 19, 3712. doi: 10.3390/s19173712
Prey, L., Schmidhalter, U. (2019b). Simulation of satellite reflectance data using high-frequency ground based hyperspectral canopy measurements for in-season estimation of grain yield and grain nitrogen status in winter wheat. ISPRS J. Photogramm. Remote Sens 149, 176–187. doi: 10.1016/j.isprsjprs.2019.01.023
Prey, L., Hu, Y., Schmidhalter, U. (2018). “Spectral sensing traits of nitrogen use efficiency in hybrid and line wheat cultivars,” in Tagungsband Der 68. Jahrestagung Der Vereinigung Der Pflanzenzüchter Und Saatgutkaufleute Österreichs, 20.-22. November 2017. Eds. Brandstetter, A., Geppner, M. (Vienna: Universität für Bodenkultur Wien), 59–62.
Prey, L., Germer, M., Schmidhalter, U. (2019a). Temporal and Organ-specific Responses in NUE Traits to N Fertilization, Fungicide Intensity and Early Sowing in Winter Wheat Cultivars. Agronomy 9, 313. doi: 10.3390/agronomy9060313
Prey, L., Hu, Y., Schmidhalter, U. (2019b). Temporal dynamics and the contribution of plant organs in a phenotypically diverse population of high-yielding winter wheat: Evaluating concepts for disentangling yield formation and nitrogen use efficiency. Front. Plant Sci. 10, 1295. doi: 10.3389/fpls.2019.01295
Prey, L., Kipp, S., Hu, Y., Schmidhalter, U. (2019c). Nitrogen use efficiency and carbon traits of high-yielding european hybrid vs. line winter wheat cultivars: potentials and limitations. Front. Plant Sci. 9, 1988. doi: 10.3389/fpls.2018.01988
R core Team (2017). A Language and Environment for Statistical Computing, (Vienna, Austria: R Foundation for Statistical Computing).
Raun, W. R., Solie, J. B., Johnson, G. V., Stone, M. L., Lukina, E. V., Thomason, W. E., et al (2001). In-Season Prediction of Potential Grain Yield in Winter Wheat Using Canopy Reflectance. Agron. J. 93, 131. doi: 10.2134/agronj2001.931131x
Rischbeck, P., Elsayed, S., Mistele, B., Barmeier, G., Heil, K., Schmidhalter, U. (2016). Data fusion of spectral, thermal and canopy height parameters for improved yield prediction of drought stressed spring barley. Eur. J. Agron. 78, 44–59. doi: 10.1016/j.eja.2016.04.013
Royo, C., Aparicio, N., Villegas, D., Casadesus, J., Monneveux, P., Araus, J. L. (2003). Usefulness of spectral reflectance indices as durum wheat yield predictors under contrasting Mediterranean conditions. Int. J. Remote Sens 24, 4403–4419. doi: 10.1080/0143116031000150059
Schnyder, H. (1993). The role of carbonhydrate storage and redistribution in the source-sink relations of wheat and barley during grain filling-a review. New Phytol. 123, 233–245. doi: 10.1111/j.1469-8137.1993.tb03731.x
Sims, D. A., Gamon, J. A. (2002). Relationships between leaf pigment content and spectral reflectance across a wide range of species, leaf structures and developmental stages. Remote Sens. Environ. 81, 337–354. doi: 10.1016/S0034-4257(02)00010-X
Sims, D. A., Gamon, J. A. (2003). Estimation of vegetation water content and photosynthetic tissue area from spectral reflectance: A comparison of indices based on liquid water and chlorophyll absorption features. Remote Sens. Environ. 84, 526–537. doi: 10.1016/S0034-4257(02)00151-7
Slimane, B. R., Bancal, P., Bancal, M.-O. (2013). Down-regulation by stems and sheaths of grain filling with mobilized nitrogen in wheat. F. Crop Res. 140, 59–68. doi: 10.1016/j.fcr.2012.10.006
Sultana, S. R., Ali, A., Ahmad, A., Mubeen, M., Zia-Ul-Haq, M., Ahmad, S., et al (2014). Normalized Difference Vegetation Index as a Tool for Wheat Yield Estimation: A Case Study from Faisalabad, Pakistan. Sci. World J. 2014, 1–8. doi: 10.1155/2014/725326
Takebe, M., Yoneyama, T., Inada, K., Murakami, T. (1990). Spectral reflectance ratio of rice canopy for estimating crop nitrogen status. Plant Soil 122, 295–297. doi: 10.1007/BF02851988
Thenkabail, P. S., Smith, R. B., De Pauw, E. (2000). Hyperspectral vegetation indices and their relationships with agricultural crop characteristics. Remote Sens. Environ. 71, 158–182. doi: 10.1016/S0034-4257(99)00067-X
Tucker, C. J., Holben, B. N., Elgin, J. H., Jr., McMurtrey, J. E., III (1980). Relationship of spectral data to grain yield variation. Photogramm. Eng. Remote Sens. 46, 657–666.
Ustin, S. L., Gitelson, A. A., Jacquemoud, S., Schaepman, M., Asner, G. P., Gamon, J. A., et al (2009). Remote Sensing of Environment Retrieval of foliar information about plant pigment systems from high resolution spectroscopy. Remote Sens. Environ. 113, S67–S77. doi: 10.1016/j.rse.2008.10.019
Van Herwaarden, A. F., Farquhar, G. D., Angus, J. F., Richards, R. A., Howe, G. N. (1998). “Haying-off”, the negative grain yield response of dryland wheat to nitrogen fertiliser. I. Biomass, grain yield, and water use. Aust. J. Agric. Res. 49, 1067–1082. doi: 10.1071/PP97167
Vogelmann, J. E., Rock, B. N., Moss, D. M., Rock, B. N., Red, D. M. M. (1993). Red edge spectral measurements from sugar maple leaves. Remote Sens. 14, 1563–1575. doi: 10.1080/01431169308953986
Yu, K., Lenz-Wiedemann, V., Leufen, G., Hunsche, M., Noga, G., Chen, X., et al (2012). Assessing hyperspectral vegetation indices for estimating leaf chlorophyll concentration of summer barley. isprs ann. photogramm. remote sens. spat. Inf. Sci. I-7, 89–94. doi: 10.5194/isprsannals-I-7-89-2012
Zarco-Tejada, P. J., Berjón, A., López-Lozano, R., Miller, J. R., Martín, P., Cachorro, V., et al (2005). Assessing vineyard condition with hyperspectral indices: Leaf and canopy reflectance simulation in a row-structured discontinuous canopy. Remote Sens. Environ. 99, 271–287. doi: 10.1016/j.rse.2005.09.002
Zhang, K., Ge, X., Shen, P., Li, W., Liu, X., Cao, Q., et al (2019). Predicting Rice Grain Yield Based on Dynamic Changes in Vegetation Indexes during Early to Mid-Growth Stages. Remote Sens. 11, 387. doi: 10.3390/rs11040387
Keywords: phenomics, smart farming, remote sensing, nitrogen use efficiency, yield prediction, red edge, water band indices, breeding
Citation: Prey L, Hu Y and Schmidhalter U (2020) High-Throughput Field Phenotyping Traits of Grain Yield Formation and Nitrogen Use Efficiency: Optimizing the Selection of Vegetation Indices and Growth Stages. Front. Plant Sci. 10:1672. doi: 10.3389/fpls.2019.01672
Received: 17 July 2019; Accepted: 28 November 2019;
Published: 17 January 2020.
Edited by:
Ashraf El-kereamy, University of California, Riverside, United StatesCopyright © 2020 Prey, Hu and Schmidhalter. This is an open-access article distributed under the terms of the Creative Commons Attribution License (CC BY). The use, distribution or reproduction in other forums is permitted, provided the original author(s) and the copyright owner(s) are credited and that the original publication in this journal is cited, in accordance with accepted academic practice. No use, distribution or reproduction is permitted which does not comply with these terms.
*Correspondence: Urs Schmidhalter, c2NobWlkaGFsdGVyQHd6dy50dW0uZGU=