- 1International Institute of Tropical Agriculture (IITA), Ibadan, Nigeria
- 2Biometrics and Statistics Unit, International Maize and Wheat Improvement Center (CIMMYT), Mexico City, Mexico
- 3Department of Plant Science, Institute for Agricultural Research/Ahmadu Bello University, Zaria, Nigeria
- 4Cereals Division, CSIR-Crops Research Institute, Kumasi, Ghana
- 5Maize Improvement Program, Institute de Economic Rurale, Bamako, Mali
- 6Crop Breeding Department, National Institute of Agricultural Research of Benin/CRA, Cotonou, Benin
- 7Department of Agronomy, University of Ilorin, Ilorin, Nigeria
- 8Maize Improvement Program, CSIR-Savanna Agricultural Research Institute, Tamale, Ghana
Maize is a food security crop cultivated in the African savannas that are vulnerable to the occurrence of drought stress and Striga hermonthica infestation. The co-occurrence of these stresses can severely damage crop growth and productivity of maize. Until recently, maize breeding in International Institute of Tropical Agriculture (IITA) has focused on the development of either drought tolerant or S. hermonthica resistant germplasm using independent screening protocols. The present study was therefore conducted to examine the extent to which maize hybrids simultaneously expressing resistance to S. hermonthica and tolerance to drought (DTSTR) could be developed through sequential selection of parental lines using the two screening protocols. Regional trials involving 77 DTSTR and 22 commercial benchmark hybrids (STR and non-DTSTR) were then conducted under Striga-infested and non-infested conditions, managed drought stress and fully irrigated conditions as well as in multiple rainfed environments for 5 years. The observed yield reductions of 61% under managed drought stress and 23% under Striga-infestation created desirable stress levels leading to the detection of significant differences in grain yield among hybrids at individual stress and non-stress conditions. On average, the DTSTR hybrids out-yielded the STR and non-DTSTR commercial hybrids by 13–19% under managed drought stress and fully irrigated conditions and by −4 to 70% under Striga-infested and non-infested conditions. Among the DTSTR hybrids included in the regional trials, 33 were high yielders with better adaptability across environments under all stressful and non-stressful testing conditions. Twenty-four of the 33 DTSTR hybrids also yielded well across diverse rainfed environments. The genetic correlations of grain yield under managed drought stress with yield under Striga-infestation and multiple rainfed environments were 0.51 and 0.57, respectively. Also, a genetic correlation between yields under Striga-infestation with that recorded in multiple rainfed environments was 0.58. These results suggest that the sequential selection scheme offers an opportunity to accumulate desirable stress-related traits in parents contributing to superior agronomic performance in hybrids across stressful and diverse rainfed field environments that are commonly encountered in the tropical savannas of Africa.
Introduction
Maize is a dominant staple food crop with 70% of its grain used directly for human consumption in sub-Saharan Africa (Vogel et al., 2019). Among the 22 countries in the world where maize provides the highest percentage of calorie in the national diet, 16 are in Africa (Nuss and Tanumihardjo, 2011). However, its low average grain yields that are pervasive in farmers' fields pose a serious threat to food security and livelihoods of millions of farmers in Africa. Farmers face many challenges that affect yields, among which drought and a parasitic weed known as Striga hermonthica (Del.) Benth have been recognized as the most widespread stresses limiting the productivity of maize in Africa (Ejeta, 2007; Edmeades, 2013). When drought occurs during flowering of maize, it disrupts pollination and diminishes availability of photosynthate to developing kernels leading to reduction in kernel number and final yield loss of 17 to 60% (Edmeades et al., 1999; Cattivelli et al., 2008; Aslam et al., 2015). Likewise, maize plants with attached Striga plants to their roots exhibit stunted growth resulting from the withdrawal of water, nutrients and assimilate from the host by the parasite (Gurney et al., 1999) causing yield loss of up to 100% in severely infested fields in Africa (Kim et al., 2002; Ejeta, 2007). Moreover, the projected rising temperatures and uncertainties in rainfall patterns associated with climate change will further accentuate the intensity and frequency of drought in many parts of Africa (Masih et al., 2014; Shiferaw et al., 2014) and create ideal conditions for S. hermonthica to thrive and expand its distribution into suitable new habitats (Mohamed et al., 2007).
Cereal crops grown in the tropical savannas of sub-Saharan Africa (SSA) are often exposed to a combination of drought stress and S. hermonthica infestation. When drought occurs in maize fields infested with S. hermonthica, it accentuates parasite damage leading to greater yield loss (Adetimirin et al., 2000). Similarly, maize plants infected with S. hermonthica produce more abscisic acid (ABA) that trigger stomatal closure to minimize water loss and this can exacerbate the negative effect of drought on productivity of maize (Taylor et al., 1996; Taylor et al., 1998). As both drought stress and Striga infection increase ABA synthesis, Ronald et al. (2017) postulates that the ABA-driven increase in stomatal closure under the combined stresses diminishes the uptake of carbon dioxide and the production of photosynthate to sustain plant growth and development. Therefore, approaches that minimize the deleterious effect of the concurrent onslaught of drought and S. hermonthica in maize are needed to improve food security and income of farmers who depend on the crop for their livelihoods.
Over many years, breeders in the International Institute of Tropical Agriculture (IITA) have run independent breeding programs with specific emphasis on developing either drought tolerant or Striga resistant maize germplasm using reliable screening protocols established for each stress. Significant achievements have been recorded in generating maize varieties that are adapted either to drought stress (Edmeades, 2013; Aslam et al., 2015) or S. hermonthica infection (Kim, 1996: Kling et al., 2000; Menkir et al., 2007). Nonetheless, such an approach will be inadequate for areas affected by concurrent presence of drought stress and S. hermonthica infestation (Mittler and Blumwald, 2010; Atkinson and Urwin, 2012). Numerous recent reviews report that abiotic and biotic stress combinations inflict greater damage to plant growth, development, and productivity in comparison to each stress applied separately in different crops (Mittler, 2006; Atkinson and Urwin, 2012; Atkinson et al., 2013; Narsai et al., 2013; Prasch and Sonnewald, 2013; Kissoudis et al., 2014; Rejeb et al., 2014; Sewelam et al., 2014; Suzuki et al., 2014; Pandey et al., 2015; Pandey et al., 2017). Consequently, a breeding approach that enhances tolerance of maize to the co-occurrence of drought and S. hermonthica can reduce risks and bolster on-farm productivity in tropical savannas.
Mittler (2006) considers simultaneous application of abiotic and biotic stresses critical to accurately characterize the response of crop plants to multiple stresses. However, the difficulty in defining the appropriate timing, duration, and intensity of simultaneous application of drought stress and S. hermonthica infestation that mimics the actual conditions occurring in the field has hampered the establishment of a precise screening protocol to select maize germplasm with tolerance to stress combinations (Suzuki et al., 2014; Ramegowda and Senthil-Kumar, 2015). Breeder in IITA have therefore adopted a sequential selection scheme that initially screens early generation lines under artificial S. hermonthica infestation during the main cropping season which is then followed by screening the selected lines under managed drought stress during the dry season to develop maize inbred lines with tolerance to stress combinations. Repeated selection of the lines for several pedigree generations using the sequential selection scheme led to the development of homozygous lines with tolerance to drought and resistance to S. hermonthica (DTSTR).
The DTSTR maize inbred lines have been evaluated in hybrid combinations through successive stages under artificial Striga infested and non-infested conditions as well as under managed drought stress and non-stress conditions until high yielding and multiple stress tolerant elite hybrids (DTSTR) are selected for dissemination to partners for regional testing. Studies are therefore needed to assess the extent to which the sequential selection scheme can stack tolerance to the two stresses in individual maize hybrids. The need for approaches that facilitate the development of crop cultivars with tolerance to abiotic and biotic stresses combinations have become active areas of recent research reviews (Atkinson et al., 2013; Kissoudis et al., 2014; Rejeb et al., 2014; Pandey et al., 2015; Sinha et al., 2016; Pandey et al., 2017). In this context, testing the agronomic performance of elite DTSTR hybrids encapsulating the impacts of the sequential selection scheme relative to commercial hybrids is important to determine the potential that exists in identifying hybrids with tolerance to stress combinations. The present study was therefore conducted to assess the responses of these hybrids to independently applied managed drought stress and artificial Striga infestation and examine to what extent maize hybrids that simultaneously express tolerance to these stresses are developed. We also evaluated the responses of the DTSTR and commercial hybrids to multiple rainfed field environments and investigated the relationships of hybrid performance under the two controlled stress conditions with performance in multiple field environments.
Materials and Methods
Genetic Materials
Five independent regional trials consisting of 40, 42, 48, 48, and 44 hybrids were evaluated under Striga infested and non-infested conditions, managed drought stress (MDS) and full irrigation (WW) as well as in several testing locations under rainfed conditions in 2012, 2013, 2014, 2015, and 2016, respectively. The year-to-year variation in the number of hybrids included in these trials was caused by constant addition of new hybrids and removal of inferior ones. Altogether, 77 three-way cross DTSTR (H01-H77), two Striga resistant (H78-H79), and 19 conventional commercial hybrids (H80-H98) plus a local maize variety (H99) were included in the regional trials (Supplementary Table S1). The 77 three-way cross DTSTR hybrids were formed from inbred lines that had undergone through eight generations (S8) of inbreeding with sequential selection first under artificial Striga infestation at Abuja and Mokwa followed by selection under managed drought stress conditions at Ikenne. The two Striga resistant and 19 conventional commercial hybrids obtained from Premier Seeds Nigeria Ltd and SeedCo represent top-crosses, single-crosses, and three 3-way crosses that are marketed in Nigeria and other countries in Africa. The local maize variety (Local) was a farmer preferred recycled hybrid or an improved open-pollinated maize variety commonly grown around the testing site where the regional trials were conducted. The two Striga resistant commercial hybrids, which are hereafter referred to as STR hybrids, were developed at IITA but were not specifically bred for tolerance to drought stress. The 19 conventional commercial hybrids as well as the local maize variety, which are hereafter referred to as non-DTSTR hybrids, were not specifically bred for tolerance to drought and resistance to Striga. The STR and non-DTSTR hybrids were included in the regional trials as benchmarks against which the performances of the DTSTR hybrids were compared under stressful and non-stressful growing conditions. Among the 99 hybrids included in the regional trials, 17 were tested for 5 years, 17 were tested for 4 years, 20 were tested for 2 years, and the remaining 45 were tested for 1 year only.
The 77 DTSTR hybrids (H01 to H77) were formed from drought tolerant and Striga resistant maize inbred lines (Supplementary Table S1) developed using a sequential selection scheme in which the inbred lines derived from bi-parental crosses between elite drought tolerant and Striga resistant lines as well as narrow and broad-based populations were first screened under artificial Striga infestation followed by evaluation of the selected lines under managed drought stress until homozygous lines were developed. Promising lines were continually selected for synchronous time in pollen shed and silking, low ear placement, and other desirable agronomic features first under artificial Striga infestation and followed by selection under managed drought stress conditions through eight generation (S8) of self-pollination. The genetic backgrounds of the broad-based source populations (TZLComp1 and STRLowEmergPool), synthetics (ACRSYN-W and IWD-SYN-STR), and a backcross containing Zea diploperennis (ZDiploBC4) and their improvements under artificial Striga infestation had been extensively described by Kling et al. (2000). The synthetics and populations were also improved for tolerance to drought under managed drought stress using an S1 recurrent selection scheme.
Performance Testing Under Managed Drought Stress and Well-Watered Conditions
The 40, 42, 48, 48, and 44 hybrids included in the five independent regional trials were arranged in 5x8, 7x6, 6x8, 6x8, and 11x4 alpha lattice designs, respectively, and were evaluated with three replications under managed drought stress (MDS) and full irrigation (WW) at the IITA experiment station in Ikenne (6053' N, 3042' E, altitude of 60 m) during the 2011/2012, 2012/2013, 2013/2014, 2014/2015, and 2015/2016 dry seasons. The trials were planted on 21 November in 2011 and on 18 November in 2012, 2013, 2014, and 2015 in two adjacent blocks separated by four ranges each being 4.25 m wide. The four ranges were planted to a commercial hybrid to minimize lateral movement of water from irrigated plots to drought stress plots. The soil at Ikenne is eutric nitosol (FAO classification) and the station has uniform experimental fields. One of the blocks received full irrigation (WW) every week through a sprinkler irrigation system from planting until the hybrids attained physiological maturity. In the second block, drought stress (MDS) was induced by withdrawing irrigation from 35 days after planting to harvesting time of the trials.
Each hybrid was planted in two 4 m long row plots with 0.75 m spacing between rows and 0.25 m spacing between plants within a row. We planted two seeds in each hill and thinned them later to one plant after emergence to attain a population density of 53,333 plants per ha. At the time of sowing, we applied 60 kg N, 60 kg P, and 60 kg K ha−1 fertilizer with an additional 60 kg N ha−1 fertilizer applied 4 weeks later. The trial field was sprayed with gramazone and atrazine as pre-emergence herbicides at the rate of 5 liters ha−1, which was followed by manual weeding to keep the trials weed-free.
Performance Testing Under Artificial Striga Infestation and Non-Infested Conditions
The regional trials were also evaluated under artificial S. hermonthica infestation (STRIN) and non-infested conditions (STRNO) at Kubwa and Mokwa in Nigeria during the main rainy seasons for 5 years using the alpha lattice designs described above with two replications. Kubwa and Mokwa are located 340 km apart and represent different climatic conditions. Kubwa is located near Abuja (9°14'N and 7°35'E, 445 m elevation), has a ferric luvisol Plinthustalf soil containing 81% sand, 12% silt, and 7% clay, receives about 1,389 mm of rainfall, and had average monthly minimum temperatures of 19 to 240 C as well as average monthly maximum temperatures of 26 to 340 C during evaluation of the hybrids for 5 years (Supplementary Table S2). At Kubwa, the growing season starts in May and ends in October. Mokwa is situated in Niger state (9°29'N and 5°05'E, 153 m elevation), has a Tropeptic Haplustox soil that is fine and kaolinitic in nature, receives 1,150 mm of rainfall and had average monthly minimum temperatures of 20 to 230 C as well as average monthly maximum temperatures of 29 to 340 C during evaluation of the hybrids for 5 years (Supplementary Table S2). The growing season at Mokwa starts at the end of June and ends in October.
Each hybrid was planted in adjacent infested and non-infested strips facing opposite to each other and separated by 1.5 m alley. Within each strip, the same hybrid was planted in two infested rows and two non-infested rows that were planted directly opposite to each other to determine precise estimates of yield losses due to S. hermonthica damage (Kling et al., 2000). Each row was 5 m long with spacing of 0.75 m between rows and 0.25 m spacing between plants within a row. The non-infested rows were treated with ethylene, which was a gas injected into the soil from a cylinder to stimulate the germination of Striga seeds 2 weeks before planting, to eliminate any potential S. hermonthica seeds present in the soil. Every year, S. hermonthica seeds were collected from farmers' sorghum fields around Abuja and Mokwa and used for infestation, which was carried out by injecting 8.5 g of sand-mixed S. hermonthica seed inoculum into holes of about 6 cm deep and 10 cm wide. The number of germinable S. hermonthica seeds placed in each hill was estimated at 3,000. We placed two maize seeds into each hole infested with sand-mixed S. hermonthica seeds and covered them with soil. One plant was manually removed from each hill 2 weeks after planting (wks) to attain a population density of 53,333 plants ha−1. As S. hermonthica infection is high under low nitrogen (Kling et al., 2000), nitrogen, phosphorus and potassium were applied at the rate of 30 kg ha−1, 60 kg ha−1, and 60 kg ha−1 at planting, respectively, and additional 30 kg ha−1 nitrogen was applied 4 weeks later. Weeds other than S. hermonthica were removed by hand throughout the cropping season.
Performance Testing in Multi-Environment Trials Under Rainfed Conditions (MET)
The regional elite DTSTR hybrid trials were also arranged in alpha lattice designs described above with three replications and evaluated during the main rainy seasons in collaboration with the national agricultural research systems (NARS) and private seed companies in 35 locations in 2012, 25 locations in 2013, 26 locations in 2014, 23 locations in 2015, and 16 locations in 2016 in nine countries in West Africa (Figure 1). These test locations represent the diverse maize growing environments in this region. Each hybrid was planted in two rows each 5 m long with spacing of 0.75 m between rows and 0.5 m between plants within a row. The collaborators in the NARS and private seed companies used crop management practices, rates of fertilizer application, and weed control methods recommended for each of their testing location when they conducted these trials.
Trait Measurements
Even though several traits were measured in the regional trials evaluated under MDS, WW, STRIN, STRNO, and in MET, grain yield was considered as the primary trait for analyses in the present study because direct selection for this trait under stressful conditions allows identification of promising stress tolerant hybrids (Verulkar et al., 2010). All ears harvested from each plot were shelled to determine percent moisture, which was used to determine grain yield adjusted to 15% moisture under each growing condition. Grain yield was calculated from shelled grain under MDS and WW, and from ear weight and grain moisture under STRIN, STRNO, and in MET assuming a shelling percentage of 80% and final adjusted moisture content of 15% in each testing environment.
Statistical Analysis
The year-to-year variation in the number of hybrids included in the five regional trials created unbalanced data sets for grain yield recorded in each location. Year-location combinations are hereafter referred to as environments. These data sets for MDS, WW, STRIN, STRNO, and MET were subjected to analyses of variance and covariance using a mixed model with the restricted maximum likelihood procedure (REML) of SAS (Vargas et al., 2013). In these analyses, all effects including hybrids were considered random and best linear unbiased predictors (BLUPs) were computed. The hybrids were considered random because they represent the elite DTSTR hybrids developed and disseminated to partners for regional testing in our breeding program. The significance test of the effects of the variance parameters was obtained from the mixed model analysis using PROC Mixed in SAS (SAS Institute, 2016). Variance component estimates from the mixed model were used to calculate repeatability (broad sense heritability) values, the grand means, and the coefficient of variation (CV) (Vargas et al., 2013). Phenotypic and genotypic correlations between yield BLUPs were computed for pairs of growing conditions (MDS, WW, STRIN, STRNO, and MET) to determine the similarity or differences in response patterns of hybrids using the procedure described by Alvarado et al. (2015) in META-R.
Descriptive statistics were computed for yield BLUPs of the DTSTR, STR, and non-DTSTR hybrids recorded under MDS, WW, STRIN, and STRNO as well as in MET using the univariate procedure of SAS (SAS Institute, 2016). Hybrid yield BLUPs of individual environments (years or year-location combinations) calculated for each stressful or non-stressful testing condition were subjected to canonical discriminant analyses to summarize the differentiation between the three hybrid groups using the CANDISC procedure in SAS (SAS Institute, 2016). The pair-wise squared distance between hybrid groups (DTSTR, STR, and non-DTSTR) was tested for significance using Mahalanobis distance statistics (Mahalanobis, 1936).
The unbalanced yield data obtained from the regional trials conducted under MDS, WW, STRIN, STRNO, and in MET for 5 years were then subjected to mixed model analyses using a factor analytic model (FA) with covariance structure. While environments were considered as fixed effects in these analyses, replicates within environments, hybrids and hybrid by environment interaction were regarded as random effects (Piepho, 1998; Smith et al., 2001). This approach has proven to be effective in generating biplots which graphically represent genotype stability and adaptability in MET (Burgueño et al., 2008; Crossa et al., 2010; Crossa et al., 2013; Figueiredo et al., 2015). The two factor analysis (FA(2)) that has become a standard model for MET analysis (Kelly et al., 2007) was used in the analyses of all data sets. The resulting two factors (FA1 and FA2) scores were utilized for assessing the hybrid by environment relationships and the adaptability and stability of hybrids in our study. Hybrids with large positive FA1 scores could be regarded as high yielders with desirable ranks across years (Stefanova and Buirchell, 2010; Smith et al., 2015), whereas those with positive FA1 scores but with close to zero FA2 scores under each growing condition were regarded as productive and stable hybrids (Nuvunga et al., 2015). To discern the adaptability patterns of the DTSTR hybrids relative to the commercial hybrids, yield BLUP estimates from each hybrid was regressed against the first factor (FA1) scores using PROC REG in SAS (Smith et al., 2001).
Among the 99 hybrids, 14 DTSTR and two STR commercial hybrids plus the farmer preferred local maize variety were continuously evaluated for 5 years. These hybrids were used to assess consistency of hybrid yield BLUP estimates across years/environments under controlled stressful (MDS and STRIN) and non-stressful (WW and STRNO) growing conditions as well as in MET. Yield BLUPs of these genotypes were ranked in each year/environment using PROC RANK in SAS (SAS Institute, 2016). The resulting ranks were then analyzed for concordance across years/environments under all testing conditions (Kendall, 1962). To further determine consistency in average performance of the hybrid groups under each testing condition (MDS, WW, STRIN, STRNO, and MET), analysis of the yield BLUPs of the 17 hybrids for each year or environment was carried out using the Statistical Analysis System (SAS) software version 9.4 (SAS Institute, 2016). Analysis of variance (ANOVA) was performed using the general linear procedure (Proc GLM). Following this analysis, the;east squares means/PDIFF (LSMEANS/PDIFF) option at P |t|< 0.05 was used to test the significant differences among the average grain yields of the different hybrid groups (DTSTR, STR, and Local).
Results
Hybrid Performance Under Managed Drought Stress and Fully Irrigated Conditions
The rainfall recorded during the period in which the regional trials were evaluated at Ikenne was very low, except for 2012/2013 that received an appreciable rainfall in November and February (Supplementary Figure S1). As a result, the hybrids were exposed to water deficit for 35 to 40 days prior to flowering with no additional irrigation applied thereafter. Relative to fully irrigated condition, the drought stress attained in this study reduced average hybrid yield by 66% in 2012, 18% in 2013, 62% in 2014, 72% in 2015, and 79% in 2016. As shown in Table 1, significant differences in grain yield were observed among hybrids and their interaction with years but not among testing years under both MDS and WW conditions. Repeatability estimates for grain yield were 0.70 for MDS and 0.82 for WW conditions (Supplementary Table S3). The range of mean grain yields of the DTSTR hybrids was broader than those of the STR and non-DTSTR commercial hybrids under these testing conditions (Table 2). As a group, the DTSTR hybrids produced significantly higher average grain yields than the STR and non-DTSTR hybrids under MDS and WW conditions (Supplementary Table S4). In contrast, the non-DTSTR hybrids had significantly higher average grain yield than the STR hybrids under WW but not under MDS.
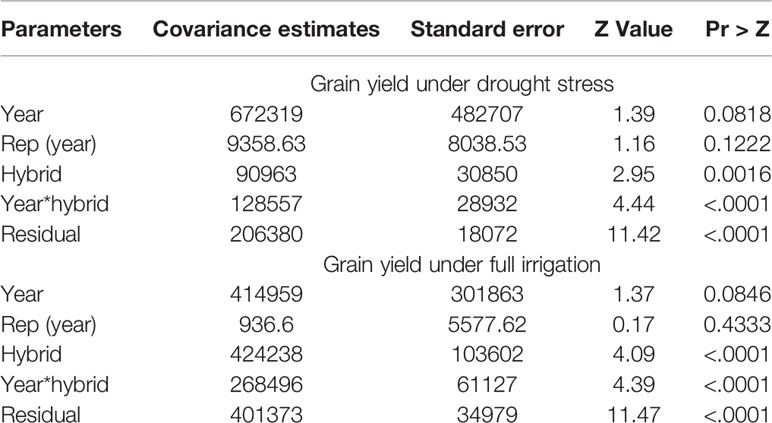
Table 1 Covariance estimates from a mixed model analysis with the restricted maximum likelihood procedure for 5 years.
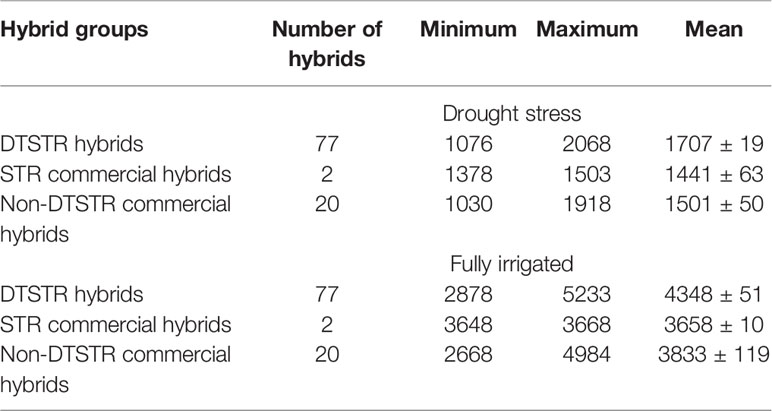
Table 2 Descriptive statistics for yield BLUPs estimated from regional trials evaluated under managed drought stress and full irrigation at Ikenne during the dry season for 5 years.
In the FA(2) model, the first factor (FA1) alone captured a total variation of 52% under MDS and 73% under WW condition, allowing appropriate assessment of adaptability of hybrids. As all the estimated FA1 loadings were positive varying from 0.31 to 1.31 under MDS and from 0.47 to 1.84 under WW, the hybrids with large positive FA1 scores could be regarded as high yielders with desirable ranks across years (Stefanova and Buirchell, 2010; Smith et al., 2015). The results of regression analyses showed that for every unit increase in FA1 score, yield BLUP estimates increased by 456 kg ha−1 under MDS and 800 kg ha−1 under WW conditions (Figure 2). Under MDS, only 26 DTSTR hybrids had negative FA1 scores and relatively lower grain yields (Supplementary Tables S3 and S4). In contrast, 20 of the 22 commercial hybrids had negative FA1 scores and relatively lower grain yields. It is interesting to note that six non-DTSTR hybrids with negative FA1 scores produced grain yields varying from 1629 to 1918 kg ha−1 under MDS, possibly due to the selection of these hybrids based on wide area testing. Under WW condition, only 21 DTSTR hybrids had negative FA1 scores and produced grain yields varying from 2878 to 4237 kg ha−1 whereas 18 commercial hybrids showed negative FA1 scores and produced grain yields varying from 2668 to 4984 kg ha−1. Among the 77 DTSTR hybrids, 44 had close to zero or positive FA1 scores under both MDS and WW condition (Supplementary Table S5). We found a few DTSTR hybrids having positive FA1 scores that also had close to zero FA2 scores under both MDS and WW conditions that could be regarded productive and stable (Supplementary Figure S2). In contrast, there was only one commercial hybrid that had a positive FA1 score combined with close to zero FA2 score under MDS but not under WW conditions.
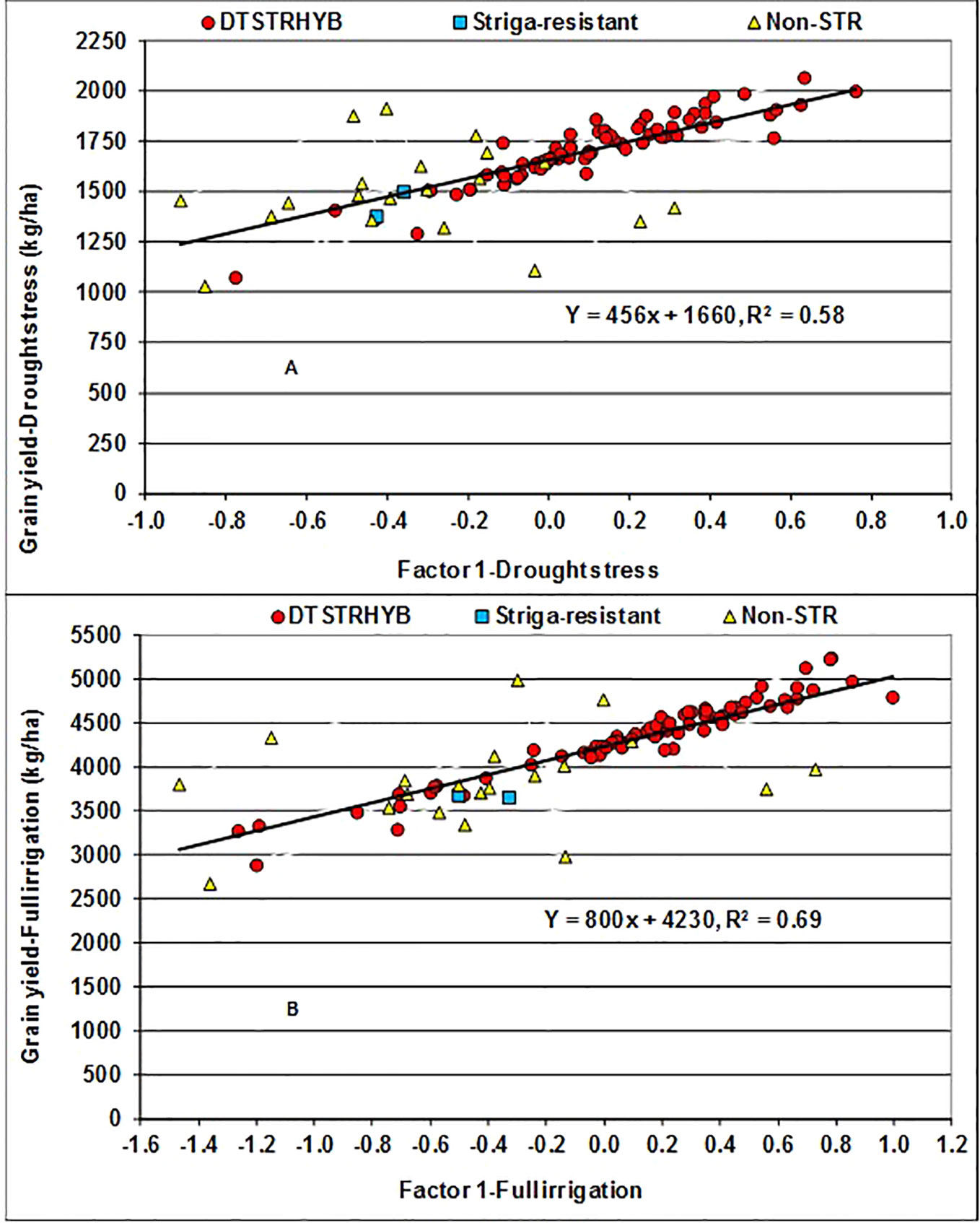
Figure 2 Regression of Best Linear Unbiased Predictors (BLUPs) of grain yield on the first factor (FA1) scores of hybrids evaluated under drought stress (A) and fully irrigated conditions (B) for five years.
Hybrid Performance Under Artificial Striga Infestation and Non-Infested Conditions
The environmental average grain yields for regional trials varied from 1727 kg ha−1 to 4244 kg ha−1 under STRIN and from 2472 kg ha−1 to 5525 kg ha−1 under STRNO conditions. A yield loss of 65% was recorded under STRIN in a commercial hybrid that was not bred for resistance to S. hermonthica, indicating that the level of Striga infection was severe in the present study. Overall yield reductions from Striga damage varied from 3% to 50% for DTSTR hybrids, from 10% to 15% for STR hybrids, and from 29% to 65% for non-DTSTR hybrids (Supplementary Table S3). About 64% of the DTSTR hybrids sustained yield losses of less than 20%. In the analysis of covariance, grain yield was significantly affected by the environment, rep (environment), hybrid, hybrid x environment interaction, and residuals under both STRIN and STRNO conditions (Table 3). Repeatability estimates for grain yield were strikingly high for both STRIN (0.93) and STRNO (0.88) conditions (Supplementary Table S3). Grain yields of the DTSTR hybrids had a wider range as opposed to the STR and non-STR hybrids under both STRIN and STRNO conditions (Table 4). On average, the DTSTR hybrids had significantly higher grain yields in comparison to the non-DTSTR hybrids but not the STR hybrids under both STRIN and STRNO conditions (Supplementary Table S6). Also, the STR hybrids had significantly greater average grain yields than the non-DTSTR hybrids under the two testing conditions.
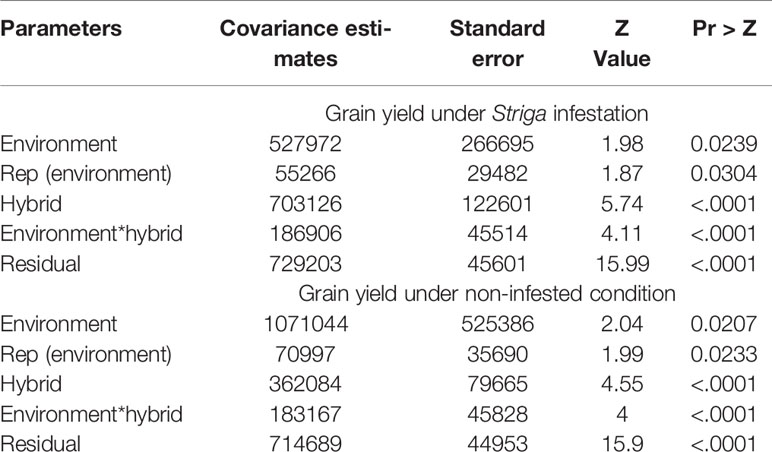
Table 3 Covariance estimates from a mixed model analysis with the restricted maximum likelihood procedure for grain yield of hybrids recorded at two locations under Striga infestation and non-infested conditions for 5 years.
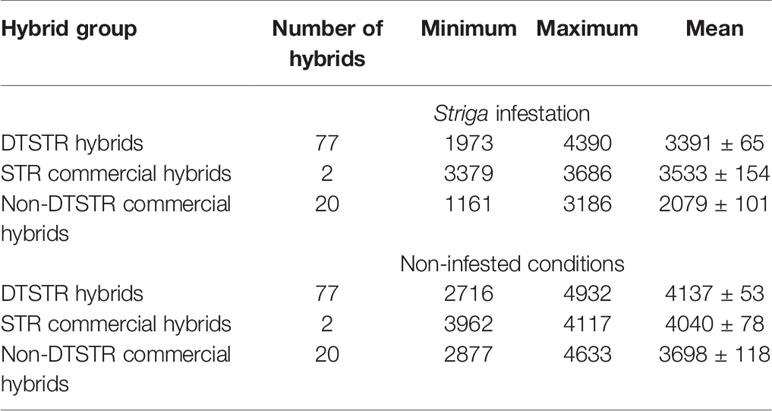
Table 4 Descriptive statistics for yield BLUPs estimated from regional trials evaluated under Striga infestation and non-infested conditions at Abuja and Mokwa in Nigeria during the main cropping season for 5 years.
The FA(2) model accounted for 91% of the total hybrid by environment variation in grain yield recorded under STRIN and 88% under STRNO conditions. FA1 alone represented 82% of the total variation under STRIN and 57% under STRNO conditions. FA1 had positive loadings of 0.60 to 1.39 under STRIN but negative loading of −0.97 to −017 under STRNO conditions. As a result, the hybrids with large positive FA1 scores under STRIN and negative FA1 scores under STRNO condition could be considered as high yielders with favorable ranks across environments (Stefanova and Buirchell, 2010). The regression analyses of yield BLUP estimates on FA1 scores accounted for 84% of the total variation among hybrids under STRIN and 56% under STRNO (Figure 3). In these analyses, every unit increase in FA1 score was associated with 913 kg ha−1 increase in grain yield under STRIN but with 558 kg ha−1 decrease in grain yield under STRNO condition. Among the 99 hybrids, 53 DTSTR and two STR commercial hybrids produced more than 3000 kg ha−1 yield and had close to zero or positive FA1 scores, whereas 19 non-DTSTR hybrids had negative FA1 scores and produced less than 3,000 kg ha−1 grain yields under STRIN (Supplementary Tables S3 and S5). Again, 46 DTSTR, one STR and three non-DTSTR hybrids had negative FA1 scores and produced more than 4,000 kg ha−1 grain yields under STRNO condition. Out of the 77 DTSTR hybrids, 42 had close to zero or positive FA1 scores under STRIN and negative FA1 scores under STRNO conditions (Supplementary Tables S3 and S5). Furthermore, a few DTSTR hybrids that could be considered high yielding and stable combined close to zero FA2 scores with positive FA1 scores under STRIN while at the same time they had close to zero FA2 scores with negative FA1 scores under STRNO condition (Supplementary Figure S3). However, none of the non-DTSTR hybrids had a combination of positive FA1 scores and close to zero FA2 scores under both STRIN and STRNO conditions.
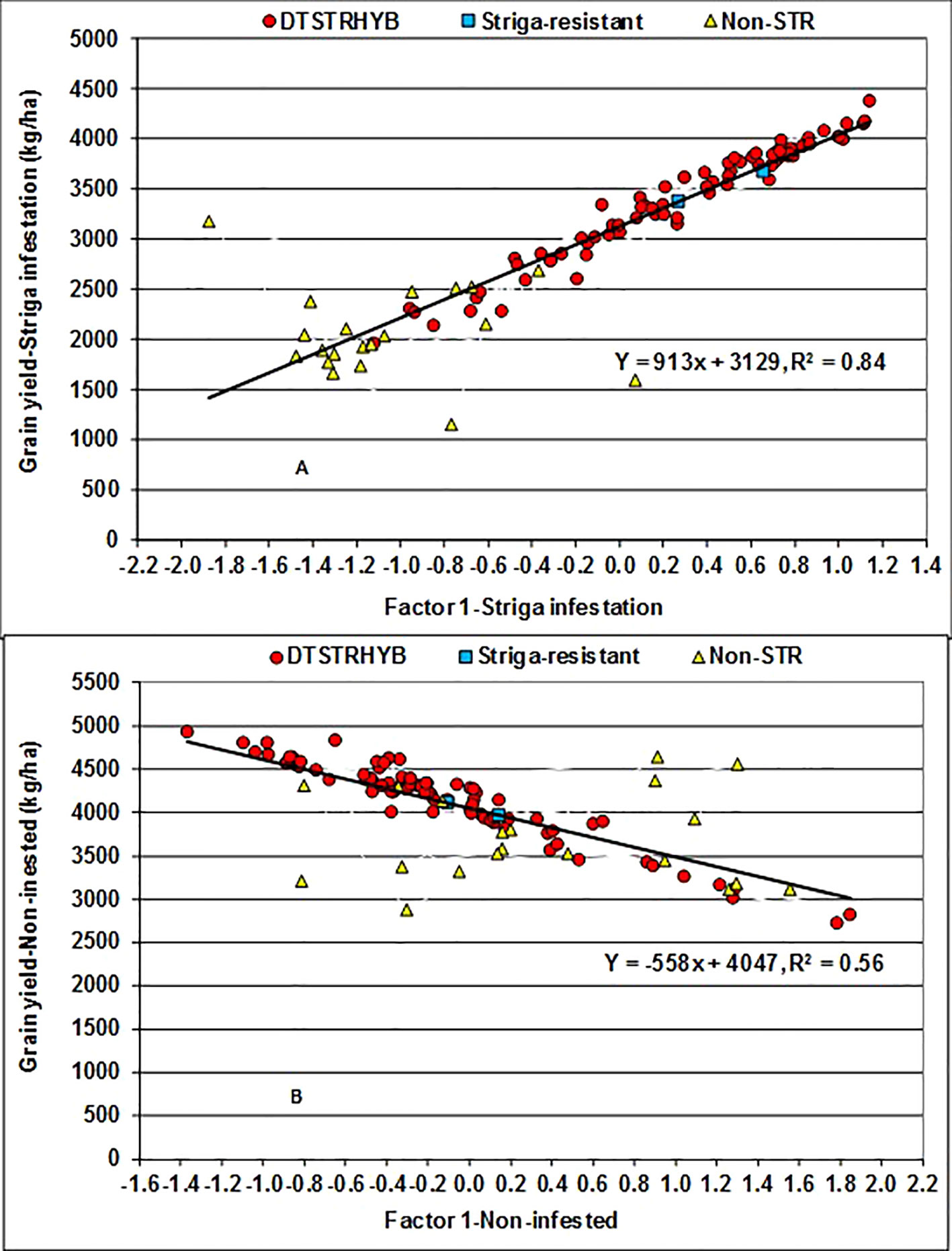
Figure 3 Regression of Best Linear Unbiased Predictors (BLUPs) of grain yield on the first factor (FA1) scores of hybrids evaluated under artificial Striga infested (A) and non-infested (B) conditions at two locations for five years.
Relationship Between Performances Under Managed Drought Stress and Artificial Striga Infestation
We examined the similarity in response patterns of elite hybrids to MDS and STRIN relative to their response to WW and STRNO using phenotypic and genetic correlations. The phenotypic correlations between grain yields measured under MDS and WW (rp = 0.74) and between those recorded under STRIN and STRNO (rp = 0.73) were significant (p < 0.0001) and strong. We also found significant (p < 0.0001) phenotypic correlations between grain yields of MDS and STRIN (rp = 0.42) and those of WW and STRNO (rp = 0.67). In addition, significant (p < 0.01) and strong genetic correlations were found between MDS and WW (rg = 0.91) and between STRIN and STRNO (rg = 0.88). The genetic correlations of yields under MDS with STRIN was moderate (rg = 0.51) but was not significant. As FA1 accounted for the largest proportion of the variation in yield BLUPs in the FA(2) models, the correlation between FA1 scores of MDS and STRIN and between WW and STRNO were used as the bases to further assess the similarity in adaptability of hybrids across different growing environments. The correlations of FA1 scores of MDS with WW (r = 0.74) and those of STRIN with STRNO (r = −0.67) were significant (p < 0.0001). Also, there were significant (p < 0.0001) correlations between FA1 scores of MDS and STRIN (r = 0.51) and those of WW and STRNO (r = −0.74). Out of the 77 DTSTR hybrids evaluated in the present study, 39 had close to zero or positive FA1 scores under both MDS and STRIN (Supplementary Table S3). Thirty-three of 39 DTSTR hybrids also had close to zero or positive FA1 scores under WW and close to zero or negative FA1 scores under STRNO conditions.
Hybrid Performance in Multi-Environment Trials Under Rainfed Conditions
The regional trials evaluated under rainfed environments had mean grain yields varying from 460 kg ha−1 to 7,993 kg ha−1. Overall, grain yield was affected by the environment, rep (environment), hybrid, hybrid x environment interaction, and the residuals (Table 5). The repeatability estimate for yield was very high (0.98) for MET (Supplementary Table S3). As a group, the DTSTR hybrids exhibited a wider range in yield BLUP estimates in comparison to the STR and non-DTSTR hybrids (Table 6). Also, the DTSTR hybrids produced significantly higher average yield as opposed to the non-DTSTR hybrids but not the STR hybrids in MET (Supplementary Table S7).
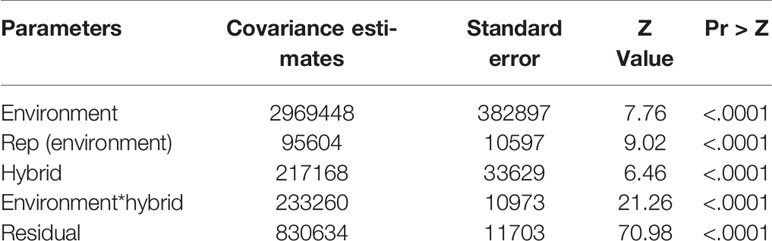
Table 5 Covariance estimates from a mixed model analysis with the restricted maximum likelihood procedure for grain yield of hybrids recorded in diverse rainfed field environments in 2012, 2013, 2014, 2015, and 2016.
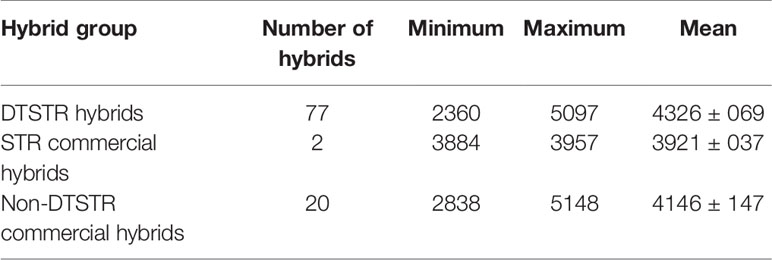
Table 6 Descriptive statistics for yield BLUPs estimated from regional trials evaluated across diverse rainfed field environments (MET) in 2012, 2013, 2014, 2015, and 2016.
The FA(2) model accounted for 77% of the total yield variation in MET. In this model, FA1 represented 53% of the total hybrid x environment variation in grain yield and had positive loadings varying from 0.31 to 1.31. Many hybrids that had large positive FA1 scores in MET were then regarded as high yielding hybrids with better ranks across environments. In the regression analyses of yield BLUP estimates on FA1 scores, the model accounted for 64% of the total variation among hybrids. This analysis showed that for every unit increase in FA1 score grain yield increased by 541 kg ha−1 (Figure 4). Among the 99 hybrids evaluated in our study, 48 DTSTR and three non-DTSTR hybrids had close to zero or positive FA1 scores and produced above 3,500 kg ha−1 yield in MET (Supplementary Tables S2 and S4). We also found some DTSTR hybrids with close to zero FA2 scores and positive FA1 scores (Supplementary Figure S4) that could be regarded as high yielding and stable hybrids across diverse rainfed environments. However, none of the non-DTSTR hybrids combined a positive FA1 score with close to zero FA2 score in the MET.
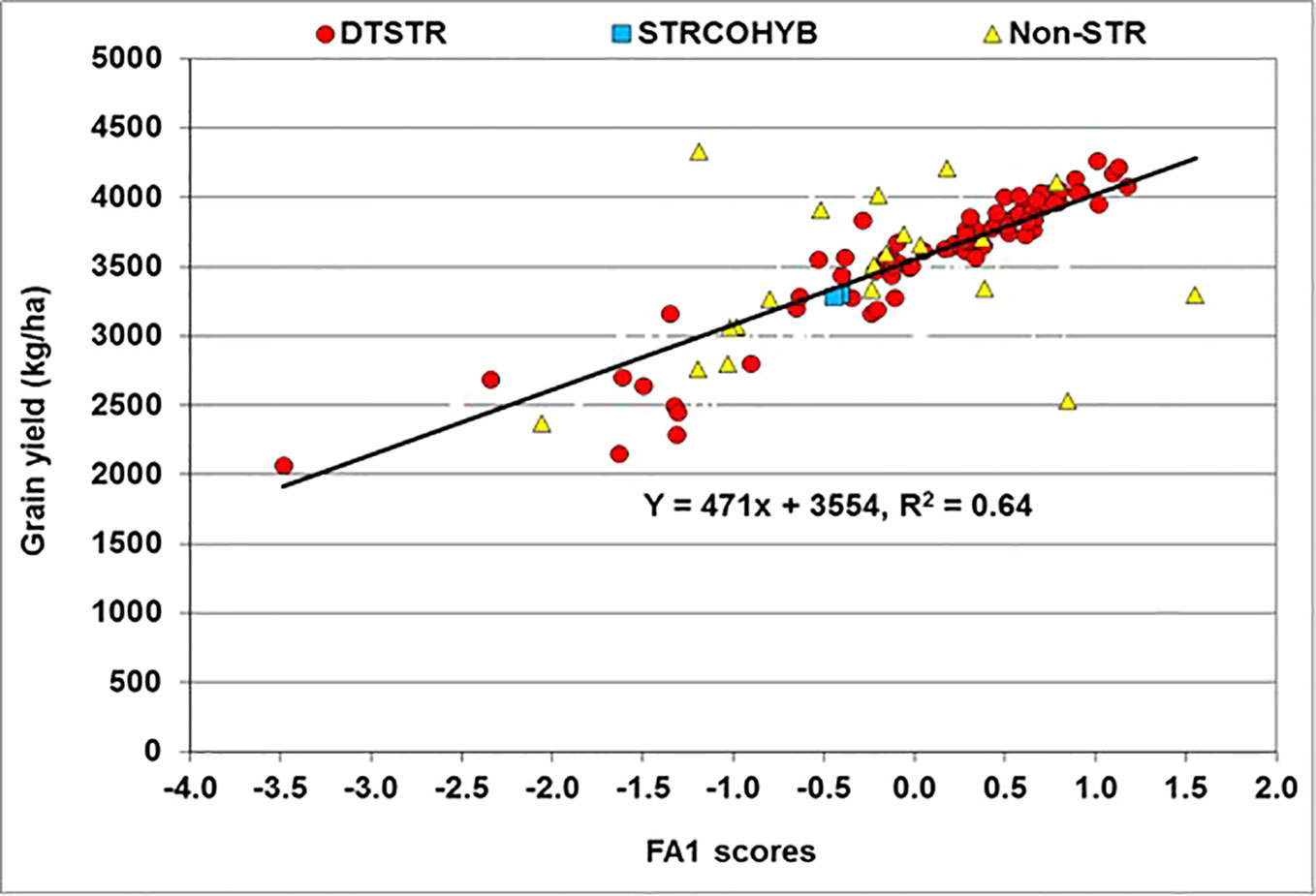
Figure 4 Regression of Best Linear Unbiased Predictors (BLUPs) of grain yield on the first factor (FA1) scores of hybrids obtained from multi-environment trails conducted under rainfed conditions.
Relationship of Performances Under Drought Stress and Striga Infestation With Performance in Multi-Environment Trials
Yield BLUP estimates for MET had significant (p <0.0001) phenotypic correlation with those of MDS (rp = 0.49), STRIN (rp = 0.51), WW (rp = 0.66), and STRNO (pr = 0.81) conditions. The genetic correlations of BLUP estimates for MET with those of WW (rg = 0.76) and STRNO (rg = 0.92) were significant (p < 0.0001) but not with those of MDS (rg = 0.57) and STRIN (rg = 0.58). The correlation between FA1 scores of MET with MDS (r = 0.37), MET with STRIN (r = 0.50), MET with WW (r = 0.58), and MET with STRNO (r =−0.63) were significant (p <0.0001), highlighting the presence of varying levels of similarity in adaptability of hybrids under the presence or absence of specific stress relative to those in multiple field environments. Among the 33 DTSTR hybrids with close to zero or positive FA1 scores under MDS, WW, STRIN, and STRNO, 24 also had close to zero or positive FA1 scores in MET (Supplementary Table S4).
Performance of Selected Hybrids Under Controlled Stresses and in Multi-Environment Trials
Smith et al. (2005) recommend use of 5 to 10 years data to obtain a representative sample of seasons for accurate assessment of varietal performance in diverse growing environments. In our study, 14 DTSTR, two STR commercial hybrids and “a local maize variety”, which were tested for 5 years, were then chosen for assessing yield potential across years/environments under MDS, WW, STRIN, STRNO, and in MET. The average grain yields of the DTSTR hybrids were consistently higher than those of the STR hybrids and the Local maize variety across years under both MDS and WW conditions (Figure 5 and Supplementary Tables S8 and S9). Also, the DTSTR hybrids on average produced consistently higher grain yields than the Local maize variety but were competitive to the STR hybrids in all environments under both STRIN and STRNO conditions (Figure 6 and Supplementary Tables S10 and S11). In MET, the average yield of the DTSTR hybrids was always higher than those of the STR hybrids and the Local maize variety across 5 years (Figure 7 and Supplementary Tables S12 and S13). Further assessment of the coefficients of concordance (W) of the ranks of the 17 hybrids were found to be significant (p < 0.0001) across years under MDS (W = 0.51) and WW (W = 0.84) and across environments under STRIN (W = 0.60) and STRNO (W = 0.62) as well as in MET (W = 0.36). As shown in Table 7, the average yield advantages of each of the best seven DTSTR hybrids (H01, H06, H07, H09, H11, H15, and H17) over the best STR commercial hybrid (H79) varied from 21 to 38% under MDS, from 15 to 36% under WW, from 11 to 30% under STRIN, from 7 to 24% under STRNO, and from 12 to 23% in MET (Table 7). The best DTSTR hybrids also produced 32 to 115% more grain yields than the Local maize variety under all stressful and non-stressful testing conditions.
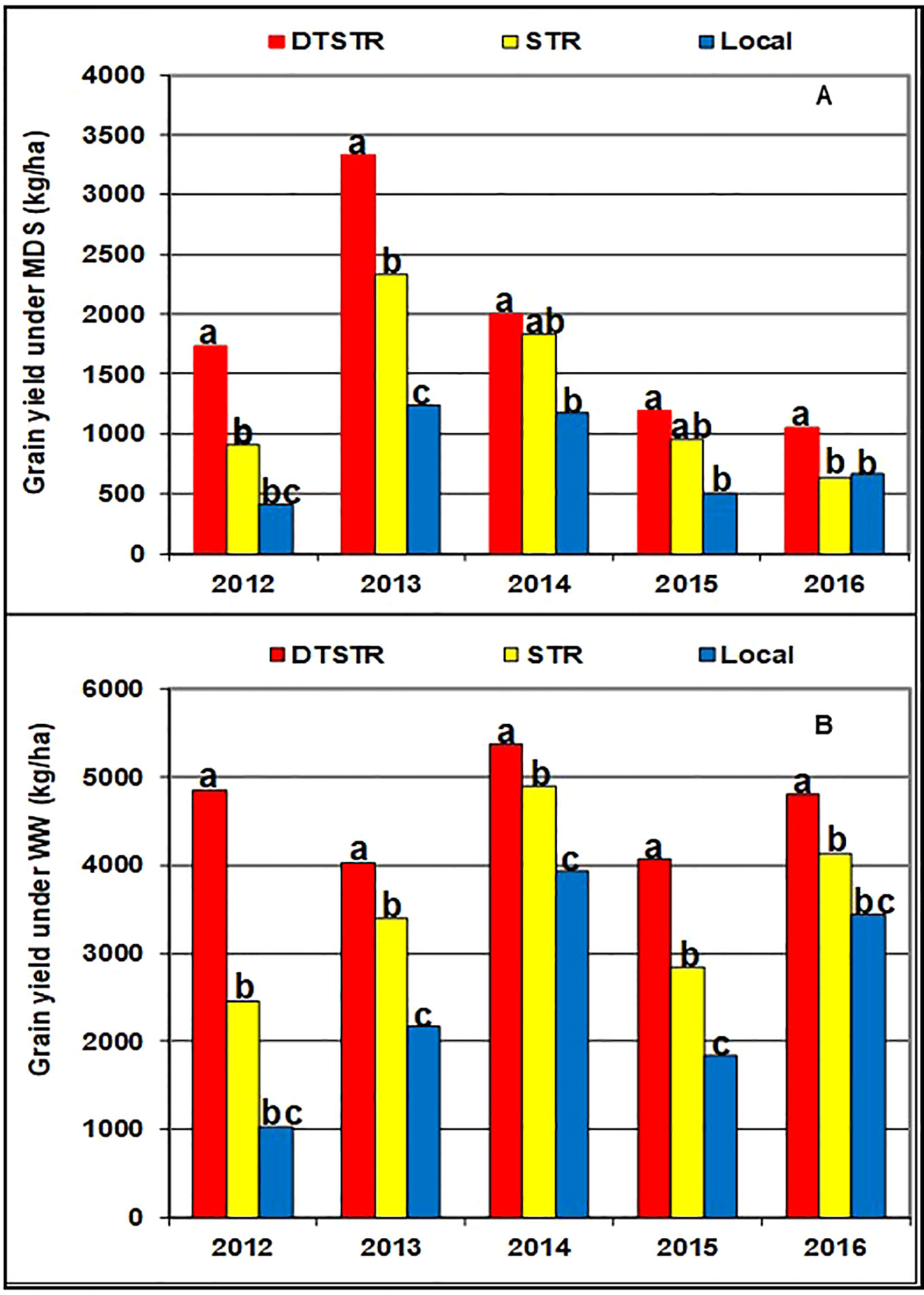
Figure 5 Overall mean grain yields of three sets of hybrids tested under managed drought stress (MDS-A) and fully irrigated (WW-B) conditions in each of the five years (2012–2016). Average grain yields of the different hybrid groups showing the same lower-case letters were not significantly different from each other at P |t|< 0.05 based on the Proc GML and LSMEANS/PDIFF procedures of SAS (SAS Institute, 2016).
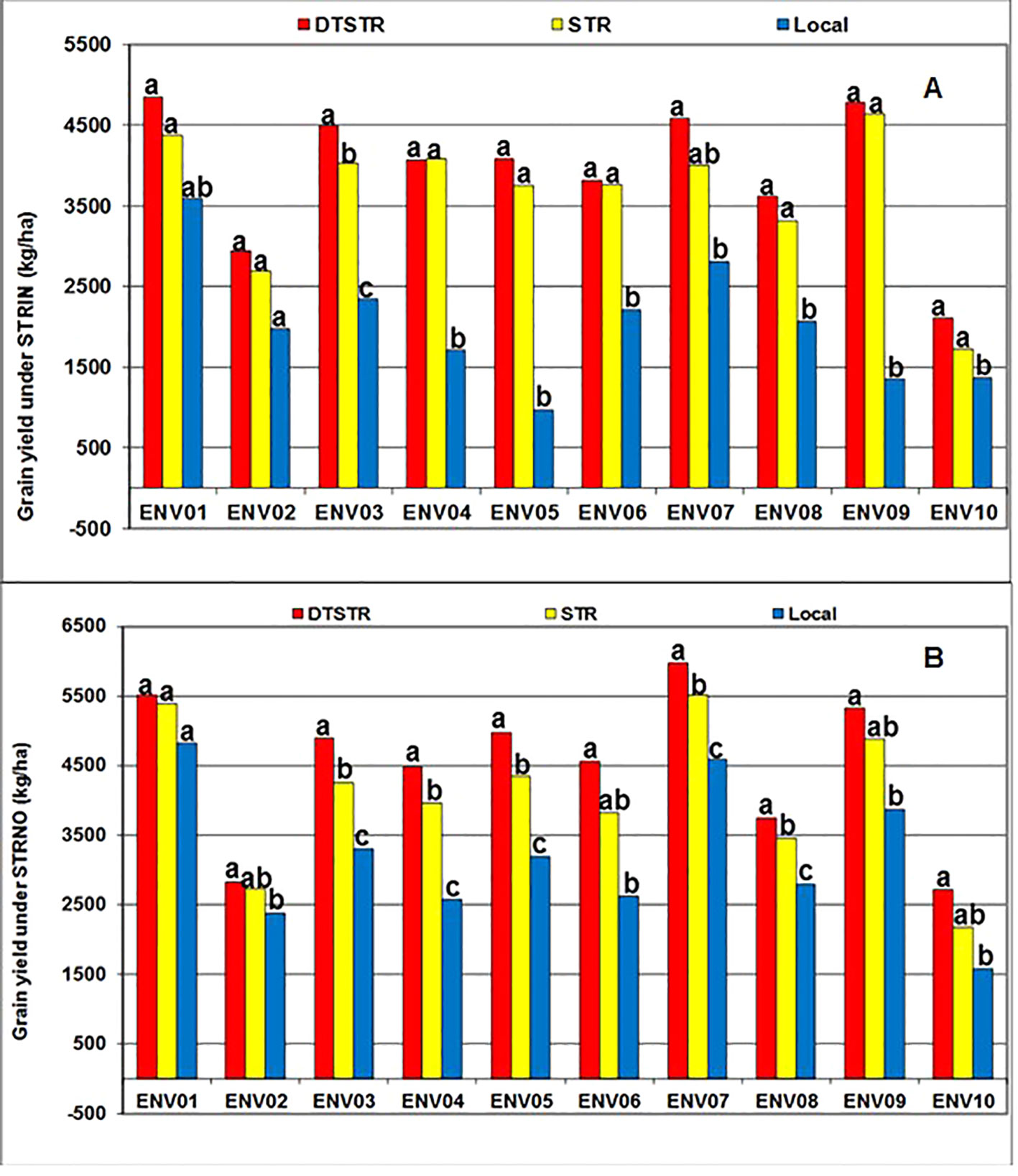
Figure 6 Overall mean grain yields of three sets of hybrids tested under artificial Striga infested (STRIN-A) and non-infested (STRNO-B) conditions in 10 environments (ENVO1-ENVO10). Average grain yields of the different from each other at P |t|<0.05 based on the Proc GLM and LSMEANS/PDIFF procedures of SAS (SAS Institute, 2016).
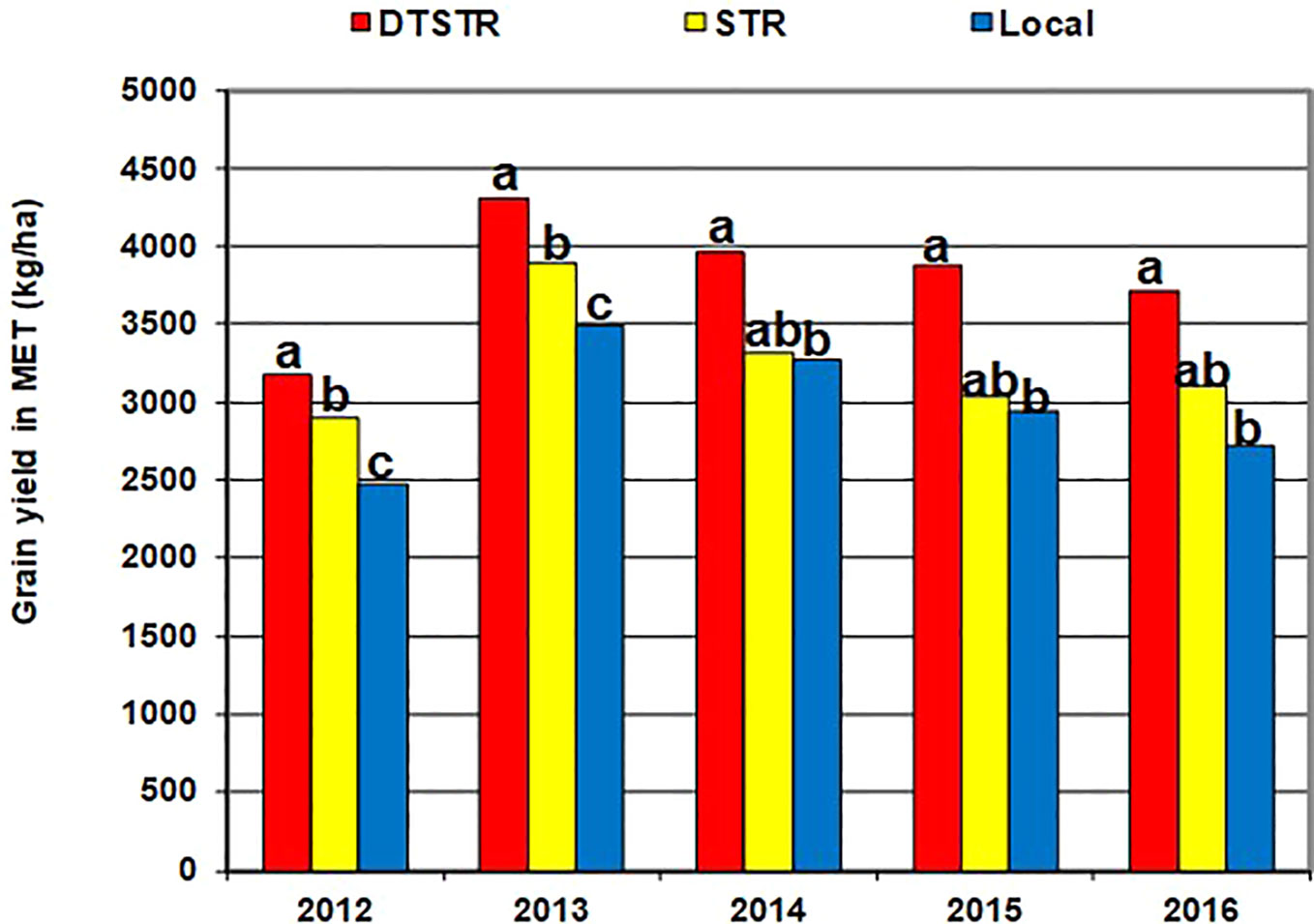
Figure 7 Overall mean grain yields of the different sets of hybrids tested in diverse rainfed field environments (MET) for each of the five years (2012–2016). Average grain yields of the different hybrid groups showing the same lower-case letters were not significantly different from each other at P |t| < 0.05 based on the Proc GML and LSMEANS/PDIFF procedures of SAS (SAS Institute, 2016).
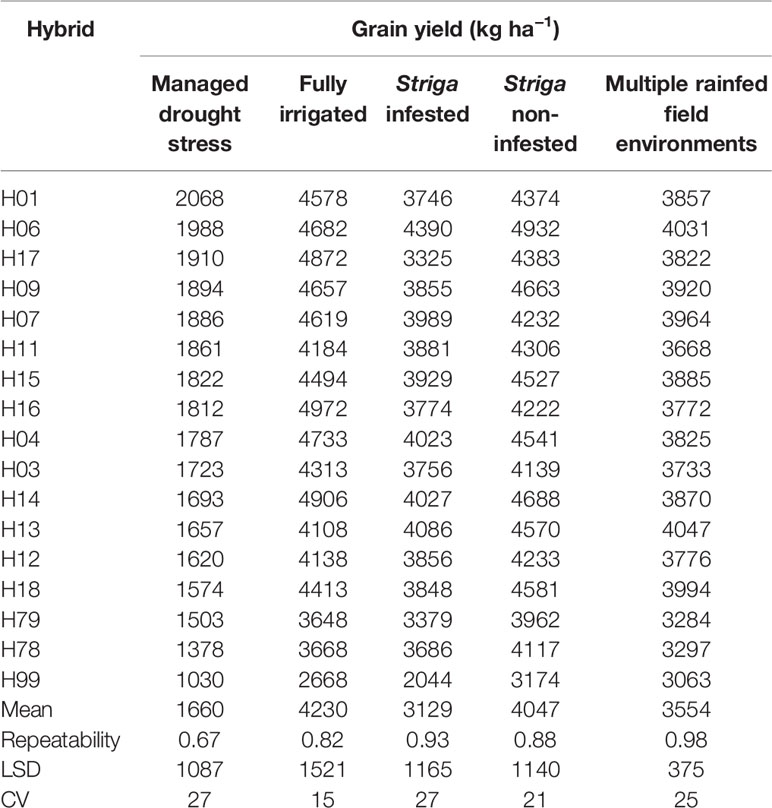
Table 7 Grain yield BLUPs of selected hybrids constantly evaluated under managed drought stress and fully irrigated conditions, artificial Striga infestation and non-infested conditions as well as in diverse rainfed field environments for 5 years.
Discussion
When drought and S. hermonthica occur simultaneously in production fields, they inflict sever damage to growth and productivity of maize. Breeding maize in IITA for many year employed independent screening protocols to develop maize germplasm with either tolerance to drought (Campos et al., 2006: Cooper et al., 2014: Edmeades, 2013) or resistance to S. hermonthica (Kim, 1996; Kling et al., 2000), but not with tolerance to the combination of the two stresses. The present study was therefore conducted to examine the extent to which maize hybrids that simultaneously express tolerance to drought and resistance to S. hermonthica could be developed through sequential selection using reliable screening protocols established for the two stresses. The results of regional trials evaluated under MDS, WW, STRIN, and STRNO showed yield reductions of more than 60% under MDS for 4 years and 65% in a susceptible commercial hybrid under STRIN, indicating the adequacy of stress levels imposed during evaluation of hybrids for 5 years. Similarly, Bänziger et al. (2000) considers managed drought stress levels leading to 40 to 60% yield reductions appropriate to distinguish maize genotypes with tolerance genes. Moreover, the high repeatability estimates for grain yield recorded under MDS, WW, STRIN, and STRNO conditions demonstrated that the managements of both stressful and non-stressful growing conditions were adequate in eliciting consistent genetic differences among the DTSTR and benchmark hybrids across years and environments.
The success in breeding for tolerance to stress combinations was assessed in the present study by measuring improvement in grain yield of the DTSTR hybrids achieved under each stress condition relative to the yield potential of the commercial benchmark hybrids. The DTSTR hybrids produced higher average grain yields than the STR hybrids under MDS and WW and the non-DTSTR hybrids under MDS, WW, STRIN, and STRNO conditions, indicating that use of the sequential selection scheme can generate hybrids combining tolerance to drought with resistance to S. hermonthica without compromising yields under non-stressful growing conditions. These results are consistent with approaches advocated by other workers who promote the development of crop varieties with tolerance to multiple stresses that also have inherent capacity to maintain high rates of photosynthesis, growth rates, and yield under non-stressful production conditions (Condon et al., 2004; Morison et al., 2008; Bechtold et al., 2010). Such crop varieties allow farmers to attain high level of productivity in years when the occurrence of drought stress and S. hermonthica infestation are severe in their fields while optimizing harvested grains during favorable growing conditions.
In the current study, 60 to 71% of the DTSTR hybrids were high yielding and had greater adaptability across environments under each of MDS, WW, STRIN, and STRNO conditions. In contrast, most of the non-DTSTR hybrids produced low grain yields and were poorly adapted to environments particularly under stressful testing conditions. These findings signify the importance of fixing desirable stress-tolerant traits in parental lines through sequential selections using carefully controlled screening protocols to productivity increases expressed in hybrids under both parasite pressure and drought stress. In this selection scheme, the inbred lines were first selected under artificial Striga infestation based on less visible reduction in plant height, stem diameter, leaf chlorosis, leaf scorching, ear size, and tassel size and fewer emerged parasites counted from maize roots (Kim, 1994; Kling et al., 2000; Menkir et al., 2007). The resulting improvements in the overall plant growth and reductions in the number of emerged parasites may lead to reduced parasite damage on host photosynthesis (Graves et al., 1989; Gurney et al., 1995; Smith et al., 1995; Watling and Press, 2001). Gurney et al. (2002) found that a tolerant maize cultivar that produced high grain yield in the presence of Striga asiatica infection maintained higher rates of photosynthesis in the field in comparison to a susceptible cultivar. As drought stress reduces leaf area, accelerates leaf senescence, decreases leaf source activity, and changes numerous physiological and biochemical processes (Zaidi et al., 2004; Wahid et al., 2005; Li et al., 2018), the retention of green leaf area in the DTSTR hybrids under parasite infection may promote high rates of photosynthesis leading to increased kernel setting and grain yield under drought stress (Li et al., 2018; Tani et al., 2019). At the same time, the emphasis on enhanced vegetative growth, less visible leaf senescence, and synchronized male-female flowering of lines during the second stage of selection under managed drought stress may further accentuate photosynthetic activity to boost performance of the DTSTR hybrids under both drought stress and Striga infestation. Taylor et al. (1996) found that drought tolerant maize genotypes accumulate less ABA in their leaves after receiving the signal from the roots infected with Striga and their photosynthetic rate is therefore not reduced. Taking these findings together, pyramiding stress-tolerant traits through several cycles of sequential selection during inbreeding until homozygous lines are developed can increase the frequency of favorable dominant alleles for grain yield that confer superior performance in the DTSTR hybrids under both drought stress and parasite infection.
The significant phenotypic and modest positive genetic correlations between grain yields recorded under MDS and STRIN in our study suggest that yield was mediated mainly by the common set of genes that regulated similar responses in hybrids under both drought stress and parasite pressure (Lorenzana and Bernardo, 2008). Sun et al. (2015) identified some stress-responsive genes showing overlapping expression patterns in rice with pleiotropic effects on adaptive responses to drought stress and S. hermonthica infection. Several other studies also found many unique genes up-regulated under combined drought stress and plant pathogen infection (Narsai et al., 2013; Kissoudis et al., 2014; Rejeb et al., 2014; Pandey et al., 2015; Ramegowda and Senthil-Kumar, 2015), which could be involved in regulating common physiological and biochemical mechanisms that modulate defense responses of crop plants to multiple biotic and abiotic stresses (Kissoudis et al., 2014; Pandey et al., 2015; Ramegowda and Senthil-Kumar, 2015).
As the common maize production system in tropical Africa is rainfed, yield trials conducted across diverse field environments permit reliable assessment of the responses of hybrids to numerous factors occurring in production fields and identify adapted hybrids for cultivation. The high repeatability value observed in MET in our study suggest that trait expression was mainly determined by differences in the genetic makeup of the hybrids rather than by differences in precipitation, temperature, soil properties, incidence of diseases and pests, and crop management practices encountered during field testing. Most of the DTSTR hybrids evaluated in MET had favorable ranks across environments and produced higher mean grain yields in comparison to the STR and non-DTSTR hybrids. Nearly 38% of the DTSTR hybrids were found to be high yielding and had better adaptability under MDS and STRIN conditions as well as in MET. The observed significant phenotypic and modest genetic correlations of yield measured in MET with yields recorded under MDS, WW, STRIN, and STRNO conditions suggest that pyramiding of stress-tolerant traits and allelic combinations in parental lines through sequential selection can also impart a broad-spectrum of plasticity in hybrids allowing them to thrive and maintain consistently high grain yields in diverse field growing environments.
Half of the DTSTR hybrids evaluated in the current study simultaneously expressed tolerance to drought and resistance to S. hermonthica, highlighting that these traits were successfully combined to increase productivity in areas where they co-occur. The best seven DTSTR hybrids produced consistently higher grain yields than the STR commercial hybrids under MDS, WW, and in MET but were competitive to the latter under STRIN and STRNO conditions, indicating the potential that exists in achieving high grain yields across diverse production conditions possibly due to the absence of physiological tradeoffs between optimal and stressful environments. The best seven DTSTR hybrids can be recommended as potential candidates for further evaluation, registration, and release in tropical savannas where their predictable and consistent yields will be valuable. Furthermore, parents of the DTSTR hybrids offer breeders with unique traits and novel alleles to further improve maize inbred lines for developing new hybrids that combine much higher levels of resistance to S. hermonthica with tolerance to drought. We believe that the sequential selection scheme can help in the development of more stress resilient maize hybrids that sustain less damage in areas affected by the co-occurrence of drought stress and S. hermonthica infestation.
In summary, the sequential selection of inbred lines for desirable traits under Striga infestation and managed drought stress had frequently led to improvements in yield potential and adaptability of hybrids across stressful and non-stressful growing conditions. Among the 38 DTSTR hybrids that simultaneously expressing tolerance to drought with resistance to S. hermonthica, 14 yielded well in MET indicating that this selection scheme was effective in pyramiding stress-tolerant traits that are also beneficial across a range of rainfed field environments. Our study thus presents a clear case for breeders to use the sequential selection scheme to underpin productivity in areas affected by co-occurrence of recurrent drought and Striga infestation. Since little is known about the response of maize genotypes to the simultaneous presence of drought stress and Striga infestation that may not always be additive (Pandey et al., 2015; Ramegowda and Senthil-Kumar, 2015), the DTSTR hybrids and their parental lines can be suitable candidates for testing under combined stress conditions to unravel the physiological, biochemical, and molecular components contributing to productivity that can be targeted for breeding to develop more resilient maize cultivars with better adaptation to production conditions in farmers' fields. In addition, the DTSTR inbred lines can be used as elite gene pools for breeders to further improve resilience in hybrids for areas affected by the co-occurrence of drought stress and S. hermonthica infestation.
Data Availability Statement
All yield data generated for this study are included in the article/Supplementary Material.
Author Contributions
AM drafted the manuscript. JC conducted analyses and contributed to write-up of the different sections of the manuscript. SM coordinated conduct of regional trials and collection of data under managed drought stress and artificial Striga infestation. BB assisted in organizing the data for analyses and preparation of figures and tables. OM, PR, MC, A-MY, GO, and HA conducted regional trials in several locations in their respective countries, collected data and provided for analyses.
Funding
This study was financed by the Drought Tolerant Maize for Africa and Stress Tolerant Maize for Africa Projects with funding from the Bill and Melinda Gates Foundation (BMGF).
Conflict of Interest
The authors declare that the research was conducted in the absence of any commercial or financial relationships that could be construed as a potential conflict of interest.
Supplementary Material
The Supplementary Material for this article can be found online at: https://www.frontiersin.org/articles/10.3389/fpls.2020.00166/full#supplementary-material
Abbreviations
DTSTR, Drought tolerant and Striga resistant; STR, Striga resistant commercial hybrids; non-DTSTR, Conventional commercial hybrids and a farmer preferred local maize variety; MDS, Managed drought stress; WW, Fully irrigated condition; STRIN, Striga infested condition; STRNO, Striga non-infested condition; MET, Multi-environment trial.
References
Adetimirin, V. O., Kim, S. K., Aken'ova, M. E. (2000). Effects of Striga hermonthica on yield components in maize. J. Agric. Sci. 135 (2), 185–191. doi: 10.1017/S0021859699008217
Alvarado, G., López, M., Vargas, M., Pacheco, Á., Rodríguez, F., Burgueño, J., et al. (2015). META-R (Multi Environment Trial Analysis with R), Version 5.0, http://hdl.handle.net/11529/10201.
Aslam, M., Amir, M., Cengiz, M. R. (2015). Drought stress in maize (Zea mays L.): effects, resistance mechanism, global achievements and biological strategies for improvement. Springer Briefs Agric. 5–17. doi: 10.1007/978-3-319-25442-5
Atkinson, N. J., Urwin, P. E. (2012). The interaction of plant biotic and abiotic stresses: from genes to the field. J. Exp. Bot. 63, 3523–3544. doi: 10.1093/jxb/ers100
Atkinson, N. J., Lilley, C. J., Urwin, P. E. (2013). Identification of genes involved in the response to simultaneous biotic and abiotic stress. Plant Physiol. 162, 2028–2041. doi: 10.1104/pp.113.222372
Bänziger, M., Edmeades, G. O., Beck, D., Bellon, M. (2000). Breeding for drought and nitrogen stress tolerance in maize: from theory to practice (México: CIMMYT).
Bechtold, U., Lawson, T., Meyer, R. C., Mejia-Carranza, J., Brown, I. R., Altmann, T., et al. (2010). Constitutive salicylic acid defences do not compromise seed yield, drought tolerance and water productivity in the Arabidopsis accession C24. Plant Cell Environ. 33, 1959–1973. doi: 10.1111/j.1365-3040.2010.02198.x
Burgueño, J., Crossa, J., Cornelius, P. L., Yang, R. C. (2008). Using factor analytic models for joining environments and genotypes without crossover genotype × environment interaction. Crop Sci. 48, 1291–1305. doi: 10.2135/cropsci2007.11.0632
Campos, H., Cooper, M., Edmeades, G. O., Loffler, C., Schussler, J. R., Ibañez, M. (2006). Changes in drought tolerance in maize associated with fifty years of breeding for yield in the U.S. Maize belt. Maydica 51, 369–381.
Cattivelli, L., Rizza, F., Badeck, F. W., Mazzucotelli, E., Mastrangelo, A. M., Francia, E., et al. (2008). Drought tolerance improvement in crop plants: an integrated view from breeding to genomics. Field Crops Res. 105, 1–14. doi: 10.1016/j.fcr.2007.07.004
Condon, A. G., Richards, R. A., Rebetzke, G. J., Farquhar, G. D. (2004). Breeding for high water-use efficiency. J. Exp. Bot. 55 (407), 2447–2460. doi: 10.1093/jxb/erh277
Cooper, M., Gho, C., Leafgren, R., Tang, T., et al. (2014). Breeding drought-tolerant maize hybrids for the US corn-belt: discovery to product. J. Exp. Bot. 65, 6191–6204. doi: 10.1093/jxb/eru064
Crossa, J., Vargas, M., Joshi, A. K. (2010). Linear, bilinear, and linear–bilinear fixed and mixed models for analyzing genotype × environment interaction in plant breeding and agronomy. Can. J. Plant Sci. 90, 561–574. doi: 10.4141/CJPS10003
Crossa, J., Vargas, M., Cossani, C. M., Alvarado, G., Burgueño, J., Mathews, K. L., et al. (2013). Evaluation and interpretation of interactions. Agron. J. 105, 1–12. doi: 10.2134/agronj2012.0491
Edmeades, G. O., Bolaños, J., Chapman, S. C., Lafitte, H. R., Bänziger, M. (1999). Selection improves drought tolerance in tropical maize populations: I. Gains in biomass, grain yield, and harvest index. Crop Sci. 39, 1306–1315. doi: 10.2135/cropsci1999.3951306x
Edmeades, G. O. (2013). Progress in Achieving and Delivering Drought Tolerance in Maize-An Update (Ithaca, NY: International Service for the Acquisition of Agri-Biotech Applications).
Ejeta, G. (2007). “The Striga scourge in Africa: A growing pandemic,” in Integrating New Technologies for Striga Control: Towards ending the witch-hunt. Eds. Ejeta, G., Gressel, J. (Singapore: World Scientific Publishing Co., Pte, Ltd), 3–16.
Figueiredo, A. G., VonPinho, R. G., Silva, H. D., Balestre, M. (2015). Application of mixed models for evaluating stability and adaptability of maize using unbalanced data. Euphytica 202, 1–17. doi: 10.1007/s10681-014-1301-3
Graves, J. D., Press, M. C., Stewart, G. R. (1989). A carbon balance model of the sorghum-Striga hermonthica host-parasite association. Plant Cell Environ. 12, 101–107. doi: 10.1111/j.1365-3040.1989.tb01921.x
Gurney, A. L., Press, M. C., Ransom, J. K. (1995). The parasitic angiosperm Striga hermonthica can reduce photosynthesis of its sorghum and maize hosts in the field. J. Exp. Bot. 46, 1817–1823. doi: 10.1093/jxb/46.12.1817
Gurney, A. L., Press, M. C., Scholes, J. D. (1999). Infection time and density influence the response of sorghum to the parasitic angiosperm Striga hermonthica. New Phytol. 143, 573–580. doi: 10.1046/j.1469-8137.1999.00467.x
Gurney, A. L., Taylor, A., Mbwaga, A., Press, M. C. (2002). Do maize cultivars demonstrate tolerance to the parasitic weed striga asiatica? Weed Res. 42, 299–306. doi: 10.1046/j.1365-3180.2002.00287
Kelly, A., Smith, A., Eccleston, J., Cullis, B. (2007). The accuracy of varietal selection using factor analytic models for multi-environment plant breeding trials. Crop Sci. 47, 1063–1070. doi: 10.2135/cropsci2006.08.0540
Kim, S. K., Adetimirin, V. O., The, C., Dossou, R. (2002). Yield losses in maize due to Striga hermonthica in West and Central Africa . Int. J. Pest Managt. 48, 211–217. doi: 10.1080/09670870110117408
Kim, S. K. (1994). Genetics of maize tolerance of Striga hermonthica. Crop Sci. 34, 900–907. doi: 10.2135/cropsci1994.0011183X003400040012x
Kim, S. K. (1996). Horizontal resistance: core to a research breakthrough to combat Striga in Africa. Int. Pest Mngt. Rev. 1, 229–249. doi: 10.1007/BF00139766
Kissoudis, C., van de Wiel, C., Visser, R. G. F., van der Linden, G. (2014). Enhancing crop resilience to combined abiotic and biotic stress through the dissection of physiological and molecular cross talk. Front. Plant Sci. 5, 207. doi: 10.3389/fpls.2014.00207
Kling, J. G., Fajemisin, J. M., Badu-Apraku, B., Diallo, A., Menkir, A., Melake-Berhan, A. (2000). “Striga Resistance Breeding in Maize,” in Breeding for Striga Resistance in Cereals. Proceedings of a Workshop held at IITA, Ibadan, Nigeria from 16-20 August 1999. Eds. Haussmann, B. I. G., Hess, D. E., Koyama, M. L., Grivet, L., Rattunde, H. F. W., Geiger, H. H. (Weikersheim, Germany: Margraf Verlag), 103–118.
Li, Y., Tao, H., Zhang, B., Huang, S., Wang, P. (2018). Timing of water deficit limits maize kernel setting in association with changes in the source-flow-sink relationship. Front. Plant Sci. 9, 1326. doi: 10.3389/fpls.2018.01326
Lorenzana, R. E., Bernardo, R. (2008). Genetic correlation between corn performance in organic and conventional production systems. Crop Sci. 48, 903–910. doi: 10.2135/cropsci2007.08.0465
Mahalanobis, P. C. (1936). On the generalised distance in statistics. Proc. Natl. Ins. Sci. India 2 (1), 49–55.
Masih, I., Maskey, S., Mussá, F. E. F., Trambauer, P. (2014). A review of droughts on the African continent: a geospatial and long-term perspective Hydrol. Earth Syst. Sci. 18, 3635–3649. doi: 10.5194/hess-18-3635-2014
Menkir, A., Badu-Apraku, B., Yallou, C. G., Kamara, A. Y., Ejeta, G. (2007). “Breeding maize for broad-based resistance to Striga hermonthica,” in Integrating New Technologies for Striga Control: Towards ending the witch-hunt. Eds. Ejeta, G., Gressel, J. (Singapore: World Scientific Publishing Co., Pte, Ltd), 99–114.
Mittler, R., Blumwald, E. (2010). Genetic engineering for modern agriculture: challenges and perspectives. Annu. Rev. Plant Biol. 61, 443–462. doi: 10.1146/annurev-arplant-042809-112116
Mittler, R. (2006). Abiotic stress, the field environment and stress combination. Trends Plant Sci. 11, 15–19. doi: 10.1016/j.tplants.2005.11.002
Mohamed, K., Bolin, J., Musselman, L., Peterson, A. (2007). Genetic diversity of Striga and implications for control and modeling future distributions. Eds. Ejeta, G., Gressel, J., (Singapore: World Scientific Publishing Co., Pte, Ltd) 71–84.
Morison, J. I. L., Baker, N. R., Mullineaux, P. M., Davies, W. J. (2008). Improving water use in crop production. Philosophical transactions of the royal society B. Bio. Sci. 363, 639–658. doi: 10.1098/rstb.2007.2175
Narsai, R., Wang, C., Chen, J., Wu, J., Shou, H., Whelan, J. (2013). Antagonistic, overlapping and distinct responses to biotic stress in rice (Oryza sativa) and interactions with abiotic stress. BMC Genomics 14, 93. doi: 10.1186/1471-2164-14-93
Nuss, E. T., Tanumihardjo, S. A. (2011). Maize: a paramount staple crop in the context of global nutrition. Comp. Rev. Food Sci. Food Saf. 9 (4), 417–436. doi: 10.1111/j.1541-4337.2010.00117
Nuvunga, J. J., Oliveira, L. A., Pamplona, A. K. A., Silva, C. P., et al. (2015). Factor analysis using mixed models of multi-environment trials with different levels of unbalancing. Genet. Mol. Res. 14, 14262–14278. doi: 10.4238/2015
Pandey, P., Ramegowda, V., Senthil-Kumar, M. (2015). Shared and unique responses of plants to multiple individual stresses and stress combinations: physiological and molecular mechanisms. Front. Plant Sci. 6, 723. doi: 10.3389/fpls.2015.00723
Pandey, P., Irulappan, V., Bagavathiannan, M. V., Senthil-Kumar, M. (2017). Impact of combined abiotic and biotic stresses on plant growth and avenues for crop improvement by exploiting physio-morphological traits. Front. Plant Sci. 6, 537. doi: 10.3389/fpls.2017.00537
Piepho, H. P. (1998). Empirical best linear unbiased prediction in cultivar trials using factor-analytic variance-covariance structures. Theor. Appl. Genet. 97, 195–201. doi: 10.1007/s001220050885
Prasch, C. M., Sonnewald, U. (2013). Simultaneous application of heat, drought, and virus to Arabidopsis plants reveals significant shifts in signaling networks. Plant Physiol. 162, 1849–1866. doi: 10.1104/pp.113.221044
Ramegowda, V., Senthil-Kumar, M. (2015). The interactive effects of simultaneous biotic and abiotic stresses on plants: mechanistic understanding from drought and pathogen combination. J. Plant Phys. 176, 47–54. doi: 10.1016/j.jplph.2014.11.008
Rejeb, I. B., Pastor, V., Mauch-Mani, B. (2014). Plant responses to simultaneous biotic and abiotic stress: molecular mechanisms. Plants (Basel) 3 (4), 458–475. doi: 10.3390/plants3040458
Ronald, M., Charles, M., Stanford, M., Eddie, M. (2017). Effect of witchweed (Striga asiatica L. Kuntze) infestation and moisture stress on selected morpho-physiological traits of sorghum (Sorghum bicolor L. Moench) genotypes in zimbabwe. Journal of Agronomy 16 (2), 65–75. doi: 10.3923/ja.2017.65.75
Sewelam, N., Oshima, Y., Mitsuda, N., Ohme-Takagi, M. (2014). A step towards understanding plant responses to multiple environmental stresses: a genome-wide study. Plant Cell Environ. 37, 2024–2035. doi: 10.1111/pce.12274
Shiferaw, B., Tesfaye, K., Kassie, M., Abate, T., Prasanna, B. M., Menkir, A. (2014). Managing vulnerability to drought and enhancing livelihood resilience in sub-Saharan Africa: technological, institutional and policy options. Weather Climate Extremes 3, 67–79. doi: 10.1016/j.wace.2014.04.004
Sinha, R., Gupta, A., Senthil-Kumar, M. (2016). Understanding the impact of drought on foliar and xylem invading bacterial pathogen stress in chickpea. Front. Plant Sci. 7, 902. doi: 10.3389/fpls.2016.00902
Smith, L. H., Keys, A. J., Michael, C. E. W. (1995). Striga hermonthica decreases photosynthesis in Zea mays through effects on leaf cell structure. J. Exp. Bot. 46, 759–765. doi: 10.1093/jxb/46.7.759
Smith, A. B., Cullis, B. R., Gilmour, A. (2001). The analysis of crop variety evaluation data in Australia. Aust. N. Z. J. Stat. 43, 129–145. doi: 10.1111/1467-842X.00163
Smith, A. B., Cullis, B. R., Thompson, R. (2005) The analysis of crop cultivar breeding and evaluation trials: an overview of current mixed model approaches. J. Agric. Sci. Camb. 143, 449–462. doi: 10.1017/S0021859605005587
Smith, A. B., Ganesalingam, A., Kuchel, H., Cullis, B. R. (2015). Factor analytic mixed models for the provision of grower information from national crop variety testing programs. Theor. Appl. Genet. 128, 55–72. doi: 10.1007/s00122-014-2412-x
Stefanova, K. T., Buirchell, B. (2010). Multiplicative mixed models for genetic gain assessment in lupin breeding. Crop Sci. 50, 880–891. doi: 10.2135/cropsci2009.07.0402
Sun, L., Huang, L., Hong, Y., Zhang, H., Song, F., Li1, D. (2015). Comprehensive analysis suggests overlapping expression of rice ONAC transcription factors in abiotic and biotic stress responses. Int. J. Mol. Sci. 16, 4306–4326. doi: 10.3390/ijms16024306
Suzuki, N., Rivero, R. M., Shulaev, V., Blumwald, E., Mittler, R. (2014). Abiotic and biotic stress combinations. New Phytol. 203, 32–43. doi: 10.1111/nph.12797
Tani, E., Chronopoulou, E. G., Labrou, N. E., Sarri, E., Goufa, M., Vaharide, X., et al. (2019). Growth, physiological, biochemical, and transcriptional responses to drought stress in seedlings of Medicago sativa L., Medicao arborea L. and their hybrids. Agronomy 9, 38. doi: 10.3390/agronomy9010038
Taylor, A., Martin, J., Seel, W. E. (1996). Physiology of the parasitic association between maize and witchweed (Striga hermonthica): is ABA involved? J. Exp. Bot. 47, 1057–1065. doi: 10.1093/jxb/47.8.1057
Taylor, A., Martin, J., Seel, W. E. (1998). Do Striga hermonthica-induced changes in soil metric potential cause the reduction in stomatal conductance and growth in infected maize plants? New Phytol. 138, 67–73. doi: 10.1046/j.1469-8137.1998.00895
Vargas, M., Combs, E., Alvarado, G., Atlin, G., Mathews, K., Crossa, J. (2013). META: a suite of SAS programs to analyze multi-environment breeding trials. Agron. J. 105, 11–19. doi: 10.2134/agronj2012.0016
Verulkar, S. B., Mandal, N. P., Dwivedi, J. L., Singhd, B. N., Sinhab, P. K., Mahatod, R. N., et al. (2010). Breeding resilient and productive genotypes adapted to drought-prone rainfed ecosystem of India. Field Crops Res. 117, 197–208. doi: 10.1016/j.fcr.2010.03.005
Vogel, E., Donat, M. G., Alexander, L. V., Meinshausen, M., Ray, D. K., Karoly, D., et al. (2019). The effects of climate extremes on global agricultural yields. Environ. Res. Lett. 14, 054010. doi: 10.1088/1748-9326/ab154b
Wahid, A., Rasul, E., Rao, R., Iqbal, R. (2005). “Photosynthesis in Leaf, Stem, Flower and Fruit,” in Handbook of Photosynthesis, 2nd Ed. Pessarakli, M. (Florida: CRC Press), 479–497.
Watling, J. R., Press, M. C. (2001). Impacts of infection by parasitic angiosperms on host photosynthesis. Plant Biol. 3, 244–250. doi: 10.1055/s-2001-15195
Keywords: stress combination, tolerance to drought, resistance to Striga hermonthica, Managed drought stress, artificial infestation, multi-environment trial
Citation: Menkir A, Crossa J, Meseka S, Bossey B, Muhyideen O, Riberio PF, Coulibaly M, Yacoubou A-M, Olaoye G and Haruna A (2020) Stacking Tolerance to Drought and Resistance to a Parasitic Weed in Tropical Hybrid Maize for Enhancing Resilience to Stress Combinations. Front. Plant Sci. 11:166. doi: 10.3389/fpls.2020.00166
Received: 15 July 2019; Accepted: 04 February 2020;
Published: 28 February 2020.
Edited by:
Takaki Yamauchi, JST PRESTO, JapanReviewed by:
Nimai Prasad Mandal, Indian Council of Agricultural Research, IndiaPangirayi Tongoona, University of KwaZulu-Natal, South Africa
Copyright © 2020 Menkir, Crossa, Meseka, Bossey, Muhyideen, Riberio, Coulibaly, Yacoubou, Olaoye and Haruna. This is an open-access article distributed under the terms of the Creative Commons Attribution License (CC BY). The use, distribution or reproduction in other forums is permitted, provided the original author(s) and the copyright owner(s) are credited and that the original publication in this journal is cited, in accordance with accepted academic practice. No use, distribution or reproduction is permitted which does not comply with these terms.
*Correspondence: Abebe Menkir, YS5tZW5raXJAY2dpYXIub3Jn