- 1Plant Breeding and Genetics Section, School of Integrative Plant Science, Cornell University, Ithaca, NY, United States
- 2Rice Research and Extension Center, University of Arkansas, Stuttgart, AR, United States
- 3Department of Agronomy, Chungnam National University, Daejeon, South Korea
- 4USDA-ARS Dale Bumpers National Rice Research Center, Stuttgart, AR, United States
Rice, Oryza sativa L., is a cultivated, inbreeding species that serves as the staple food for the largest number of people on earth. It has two strongly diverged varietal groups, Indica and Japonica, which result from a combination of natural and human selection. The genetic divergence of these groups reflects the underlying population structure of their wild ancestors, and suggests that a pre-breeding strategy designed to take advantage of existing genetic, geographic and ecological substructure may provide a rational approach to the utilization of crop wild ancestors in plant improvement. Here we describe the coordinated development of six introgression libraries (n = 63 to 81 lines per library) in both Indica (cv. IR64) and Japonica (cv. Cybonnet) backgrounds using three bio-geographically diverse wild donors representing the Oryza rufipogon Species Complex from China, Laos and Indonesia. The final libraries were genotyped using an Infinium 7K rice SNP array (C7AIR) and analyzed under greenhouse conditions for several simply inherited (Mendelian) traits. These six interspecific populations can be used as individual Chromosome Segment Substitution Line libraries and, when considered together, serve as a powerful genetic resource for systematic genetic dissection of agronomic, physiological and developmental traits in rice.
Introduction
Modern-day plant breeders seldom return to wild gene pools to rigorously explore crop wild relatives as a source of variation for plant improvement (Hajjar and Hodgkin, 2007; Warschefsky et al., 2014; Dempewolf et al., 2017). This is because using wild or exotic materials is disruptive to established breeding programs; when they are crossed to elite breeding lines, the influx of new alleles throughout the genome disturbs carefully constructed gene complexes that are the basis of highly valued traits in commercial varieties (Dempewolf et al., 2012). Even as favorable alleles are introduced, they are frequently accompanied by linked deleterious ones that require effort to eliminate. Sterility barriers may also hamper the generation of fertile offspring, adding significant time and cost to variety development (Zamir, 2001; Haussmann et al., 2004). Furthermore, it is difficult to predict the genetic potential of exotic materials because quantitatively inherited phenotypes are often masked until the accessions have been repeatedly backcrossed into well-adapted varieties (Frey et al., 1984; Eshed and Zamir, 1995; Tanksley and McCouch, 1997). Instead, for over half a century, plant breeders have been able to make reliable genetic gain in staple food crops by crossing and recombining variation within elite gene pools, fine-tuning varieties to fit target environments and relevant agricultural practices. Indeed, the combination of improvements in breeding and management has enabled steady increases in global production of staple crops such as maize (Zea mays L.), wheat (Triticum aestivum L.) and rice (Oryza sativa L.) for over 60 years (Food and Agriculture Organization of the United Nations, 2020).
Despite the steady results hitherto achieved with this approach, new challenges lie on the horizon. Paleoclimatic evidence suggests that we are coming to the end of an unprecedented period of global climate stability that ushered in the era of agriculture during the Pleistocene-Holocene transition (Feynman and Ruzmaikin, 2018). Increased variability in temperature and rainfall patterns are forecasted to escalate occurrences of extreme drought, flooding, wind, salt incursion, and pest and disease infestation (Rosenzweig et al., 2014; Fita et al., 2015). By 2050, an additional two billion people will share our planet (United Nations Department of Economic and Social Affairs, 2017), placing even greater pressure on plant-based food, fiber, feed and fuel production (Tilman et al., 2002; Godfray et al., 2010). These upheavals create new impetus for crop improvement programs to respond to a multitude of challenges, and new varieties must address the changing biophysical and economic constraints of growers and producers as well as the evolving demands and aspirations of consumers. Never has there been a more urgent call for plant breeders to take on so many significant challenges at once.
As the far-reaching effects of climate change are increasingly recognized and the need to reconcile short term gains in productivity with the long term viability of agricultural ecosystems becomes more apparent, plant breeders, policy makers and agriculturalists seek new ways of incorporating an expanded palette of genetic variation in farmers’ fields (Dempewolf et al., 2014; Pilling et al., 2020). There is long-standing interest in wild and exotic germplasm as a source of genetic resilience and potentially as a source of novel nutritional and/or quality traits (Redden et al., 2015; Dempewolf et al., 2017; Li et al., 2018; Burgarella et al., 2019; Rosyara et al., 2019). However, efforts to explore the range of genetic variation found in wild and exotic ancestors have been largely ad hoc and are undertaken without a clear path to commercial variety release. This is not to discount the many examples of successful pre-breeding, where wild alleles been shown to confer adaptive advantages in advanced breeding lines (Tanksley and McCouch, 1997; Zamir, 2001; Gur and Zamir, 2004; McCouch et al., 2007; Dwivedi et al., 2008; Mammadov et al., 2018), but rather to point out that these efforts are costly, time consuming and, in a majority of cases, fail to traverse the “last mile” to find their way into farmers’ fields. A more comprehensive understanding of how to systematically explore diverse sources of natural variation, predict genetic value and efficiently incorporate exotic variation into elite cultivated genetic backgrounds is the first step toward enhancing the value of plant genetic resources in the context of crop improvement (Warschefsky et al., 2014; Street et al., 2016; Migicovsky and Myles, 2017; Prohens et al., 2017; Li et al., 2018).
In rice, the Oryza rufipogon Species Complex (ORSC) is the progenitor of O. sativa. It is found dispersed throughout a wide range of habitats across South, Southeast and Eastern Asia (Oka, 1988; Vaughan et al., 2008). Over the last million years, the ORSC has evolved and diversified on both sides of the Himalayan Mountains, successfully adapting to highly variable ecosystems and environmental conditions (Guo and Ge, 2005; Stein et al., 2018). An evaluation of genome-wide variation in a collection of 286 ORSC accessions originating from 15 countries revealed six subpopulations reflecting both perennial and annual types of rice distributed across the geographical range of the species complex. Three of the ORSC subpopulations were found to be genetically related to the three most divergent O. sativa subpopulations, Indica, Aus, and Japonica, raising interesting questions about cross-compatibility, wild-cultivated combining ability, and shared allele complexes. To explore these questions, we undertook the development of a coordinated set of wild × cultivated chromosome segment substitution line (CSSL) libraries by systematically recombining distinct subpopulations of both wild and cultivated forms of rice.
The objective of this study was to develop and make available a suite of wild × cultivated rice CSSLs to stimulate both scientific discovery and translation in breeding, while helping to bridge the gap between genebanks and crop improvement. We provide a resource that can be rigorously evaluated in diverse environments using advanced phenotyping technologies, genetically dissected and/or expanded through crossing and/or genome manipulation, and shared widely with researchers and breeders with a common interest in exploring the genetic potential of crop wild relatives. Toward this goal, we generated six introgression libraries in elite Indica (cv. IR64) and Japonica (cv. Cybonnet) backgrounds using three genetically and bio-geographically diverse ORSC donors from China, Laos and Indonesia. This coordinated set of CSSL libraries is the first of its kind; it will enable investigators to begin to systematically examine the impact of wild introgressions in two very different genetic backgrounds, to mix and match introgressions from the same or different wild donor genomes, and to compare and contrast the phenotypic impact of introgressions in homozygous or heterozygous combinations. These materials provide the basis for predicting whether some wild subpopulations combine more productively with Indica versus Japonica varieties and offer insights about the allelic series in diverse germplasm. Deeper understanding about which combinations of wild by elite parents are most likely to give rise to superior offspring will help provide a roadmap to design pre-breeding programs that enable rice breeders to make more efficient use of wild and exotic plant genetic resources.
Materials and Methods
Parental Selection and Initial Crossing
A total of six libraries of inter-specific CSSLs were constructed in two O. sativa (Indica and Japonica) backgrounds using three wild (ORSC) donor accessions. To help select wild donors for CSSL development, genetic relationships were examined among 286 ORSC accessions and 54 O. sativa varieties initially using 50 Simple Sequence Repeat (SSR) markers and subsequently using a set of 113,739 GBS SNPs (Kim et al., 2016). A rooted Neighbor Joining (NJ) dendrogram using an Oryza officinalis, Wall ex Watt accession set as the outgroup was constructed with 100 bootstrap replicates (Tamura and Nei, 1993) in Geneious v10.0.9. ORSC donors were selected to represent geographical, phenotypic and genomic diversity, and donor selection was also influenced by cross compatibility with the cultivated recurrent parents (RPs). Three donor plants per wild accession were grown out for crossing with multiple individuals from the two RPs in the Guterman Greenhouse at Cornell University (Kim et al., 2016). Efforts to develop purified donor lines from the ORSC individuals used for crossing were not successful, and therefore additional plants were grown from the original donor seed stocks and used as proxies in some of the subsequent analyses.
CSSL Development
Three criteria were used to select lines for generation advance over the course of development: (1) presence of a wild introgression in a target region, i.e., a region that contributed to the goal of providing coverage of the entire donor genome with independent, overlapping substituted segments, (2) minimum number of wild introgressions in non-target regions, contributing to the goal of creating a library consisting of a set of near isogenic lines, each carrying a single wild introgression in an RP genetic background and (3) recovery of a “lost introgression,” in cases where a heterozygous donor segment present in one generation was not recovered in the subsequent generation, requiring the retrieval of a line from an earlier generation. The breeding scheme is presented in Supplementary Figure S1.
Marker-assisted selection (MAS) was carried out at every generation. Foreground selection was performed in the first three generations using 384 Oligo Pool Assays (OPAs) to establish sets of lines that covered the donor genome (Thomson et al., 2012). Subsequent generations utilized 6 K or 7 K SNP arrays (the C6AIR and C7AIR; Thomson et al., 2017; Morales et al., 2020), to eliminate non-target introgressions (negative selection) and increase the proportion of recurrent parent background. Length of each donor segment was determined based on the physical position of the marker genotypes. For a given introgression line, the preferred range for a target donor introgression was 4–5 Mb (13–17 cM), with maximum recurrent parent background. Each introgression line was selected such that there was a 1–2 Mb overlap within its neighboring introgression line on both sides of the introgressed segment. Donor segment selection was given preference over background recovery. Therefore, if donor segments were lost during backcrossing, plants from an earlier generation with less recurrent parent recovery were selected for the final library. For each population, the set of lines representing the final library were analyzed using CSSL Finder v.0.9.7221. The target segment size was calculated in Microsoft Excel, whereas the number of donor and background segments, and the percent recurrent parent genome, were calculated using CSSL finder. The libraries were visualized using the CSSL finder or GGT (van Berloo, 2008) software during population development.
Genotyping
Genomic DNA was extracted from leaf tissue from individual plants in each backcross generation using a modified CTAB protocol (Dellaporta et al., 1983; Fulton et al., 1995). As new technology became available, various genotyping methods were employed for MAS during library development. These platforms were SSRs, 384-OPA, the Cornell_6K_Array_Infinium_Rice (C6AIR) and the C7AIR (McCouch et al., 2002; Orjuela et al., 2010; Thomson et al., 2012, 2017; Morales et al., 2020). Details on each of these genotyping methods are provided in Supplementary Data 1. Genotyping of the final libraries was carried out using the C7AIR SNP array (Morales et al., 2020). The “ACGT” nucleotide file from Genome Studio was exported in the PLINK format and uploaded on TASSEL GUI2. The taxa (samples) were divided into six groups, each representing one of the six CSSL populations. In each library, SNPs were called based on major vs. minor allele, where it was assumed that the minor allele was inherited from the wild donor parent. SNPs with ≥39% missing data or heterozygous calls, monomorphic SNPs, and singleton SNPs were removed from each dataset. Specific SNPs were removed when it was known that one of the parents could carry either the major or the minor allele at that variant due to heterogeneity of the parental accessions. In addition to genome-wide assays, all five parental accessions in addition to CSSLs that had red or red/brown pericarp were analyzed using functional InDel markers for Rc developed by Sweeney et al. (2006) and sequencing primers for Rd described by Furukawa et al. (2007). Likewise, donors and RPs were sequenced at black hull genes, Ph and BH4 using primers described in Yu et al. (2008) and Zhu et al. (2011).
Phenotyping
Morphological variation for 16 traits was documented for donor accessions and RPs based on observations of two to five plants per accession. Parent plants were grown in 4 inch pots in the Guterman greenhouse at Cornell University (Ithaca, NY, United States) during summer 2015 and evaluated as described in Supplementary Table S1. The phenol reaction of the grain was determined on the parents following the method of Chen et al. (2014). CSSLs were also evaluated for pericarp color, hull color, seed shattering, seed set, and delayed flowering (noted as very late flowering under long (∼15 h) days.
CSSL Statistics
Custom R scripts3 were written to calculate per-library and per-line statistics and to make figures of marker distribution and graphical genotypes. Percent donor genome, percent heterozygosity, and percent missingness were computed based on the total number of informative markers per library. To map QTL in the interspecific CSSL population, stepwise regression within IciMapping v4.0 software4 (RSTEP-LRT option) can be implemented as described in Wang et al. (2017) and Balakrishnan et al. (2020).
Germplasm Availability
Seeds from the CSSLs and the RPs, IR64 and Cybonnet, are available from the Genetics Stocks-Oryza (GSOR) center in Stuttgart, AR, United States5. As is standard practice, five seeds per genetic stock will be provided for researchers to amplify. For partially sterile and low-yielding CSSLs, seeds from sibling or progeny lines may be provided in lieu of the original CSSL seed. Seeds from genebank accessions of the three ORSC donors are available as follows: OrA (W1944) from the National Institute of Genetics, Japan; OrB (IRGC106148) and OrC (IRGC105567) from the International Rice Germplasm Collection in the Philippines.
Results and Discussion
Selection of Wild and Cultivated Parents
Genetic relationships among a collection of 286 accessions from the ORSC and a set of 54 O. sativa control samples were examined using a rooted NJ dendrogram and a previously generated set of 113,739 GBS SNPs (Kim et al., 2016; Figure 1). Based on geographical, phenotypic and genomic diversity, as well as cross-compatibility, three ORSC accessions were selected for crossing with both Indica and Japonica elite varieties. They were (1) NIAS W1944 (hereafter referred to as OrA) from China, classified in the Institute of Genetics in Japan as O. rufipogon Griff.; (2) IRGC106148 (hereafter referred to as OrB) from Laos, identified as Oryza nivara Sharma and Shastry; and (3) IRGC105567 (hereafter referred to as OrC) from Indonesia, classified as O. rufipogon Griff (Table 1). These accessions represented wild subpopulations W6, W4/W1 admix, and W1, respectively, as reported by Kim et al. (2016). Genetically, this suggests that OrA is more closely related to the Japonica varietal group, OrB shares ancestry with Aus and Indica, and OrC represents a group of ancestors that do not share close ancestry with any O. sativa subpopulation.
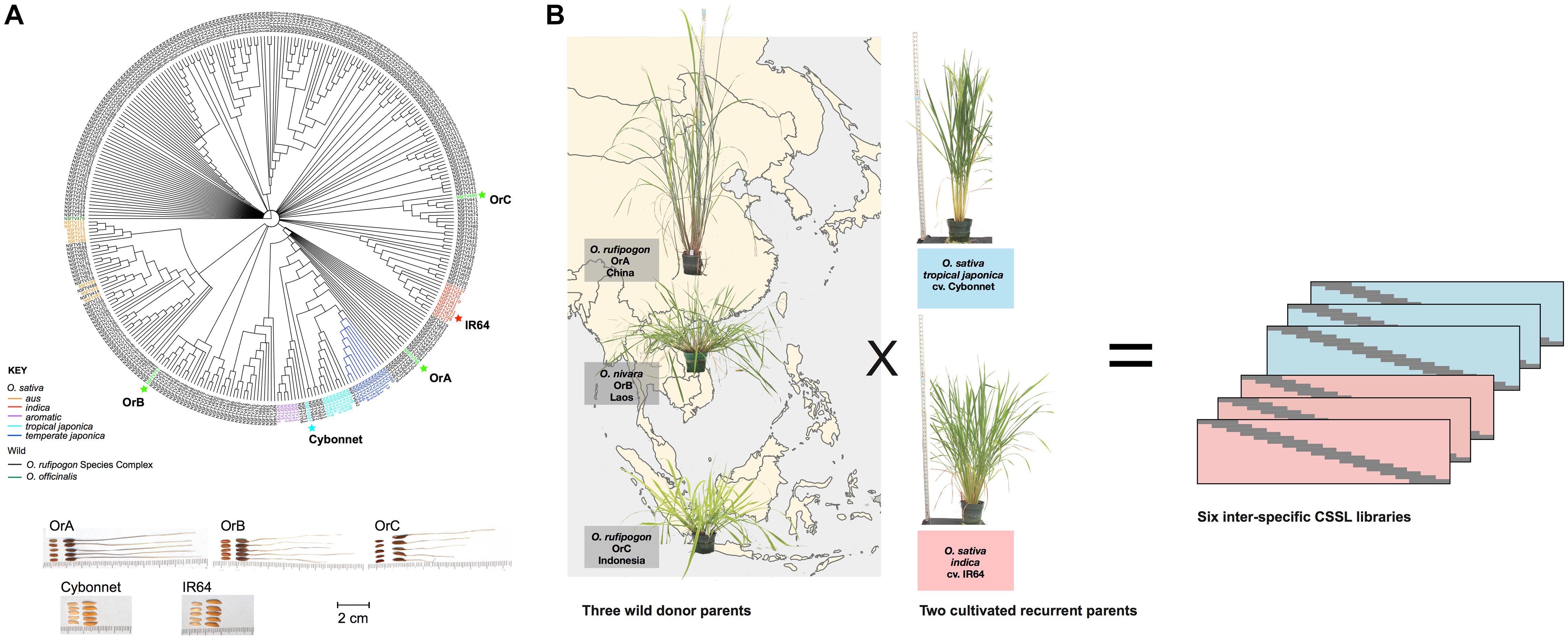
Figure 1. Parental selection of six inter-specific Chromosome Segment Substitution Line (CSSL) libraries. Six libraries of CSSL libraries were constructed in two elite O. sativa backgrounds (cv. Cybonnet and IR64) using three wild Oryza rufipogon species complex (ORSC) donor accessions (OrA, OrB, and OrC). Seed morphology and genetic relationships of parental lines with each other and other wild and cultivated accessions are shown in panel (A). Geographical origin and plant architecture variation of the wild donors are shown in panel (B).
Recurrent parents were selected to represent both the Japonica and the Indica varietal groups, with emphasis on elite, publicly available breeding material commonly used in public-sector breeding programs and widely grown in different parts of the world. Cultivars Cybonnet (CV-122, PI636726, GSOR301380) and IR64 (IRGC117268, GSOR301401) were selected as the RP (Table 1). Cybonnet is an elite tropical japonica from the United States adapted to the sub-tropics, and was released by the Arkansas Agricultural Experiment Station in 2004. It is early maturing, high-yielding, and semi-dwarf, with long-grain, improved milling yield and good blast resistance (Gibbons et al., 2006). IR64 is an elite Indica developed by the International Rice Research Institute (IRRI) in the Philippines and released in 1985. It is a semi-dwarf variety with high yield potential, short growth duration, enhanced resistance to diseases and insect pests and is well-known for its superior eating quality (Khush, 2005; Mackill and Khush, 2018). IR64 is adapted to the tropics and is widely grown throughout South and SE Asia. It is still commonly used as a parent for breeding of both inbred and hybrid varieties (Virmani and Kumar, 2004; Toriyama and Kazama, 2016).
Phenotypic Diversity of Donor and Recurrent Parents
The wild donors and the elite RPs were evaluated in the greenhouse for 16 characters, with emphasis on plant and seed morphological traits, flowering time, and seed set (Table 2 and Supplementary Table S1). OrA from China was readily differentiated from the other two donors with its tall, upright plant type, compact panicles, lack of shattering, lack of stolons and elbows, as well as its early flowering. OrB from Laos had short stature, abundant stolons and elbows, spreading culm habit, open panicle type and abundant shattering, with intermediate flowering time (Figure 1). OrC from Indonesia was also short, flowered later than the other donors, had open panicles that shattered readily, and very poor seed set. All donors had black hull, long awns and positive phenol reaction. Conversely, neither recurrent parent displayed awns, elbows or a shattering habit during any greenhouse or field observations, and both had a high rate of seed production. Cybonnet had erect culm angle, compact panicles and straw colored grains. IR64 had erect culm angle with intermediate panicle type and golden hull color. Cybonnet seeds showed no reaction to phenol while IR64 seeds had a positive phenol reaction whereby seeds turned a dark purple-black color when exposed to phenol.
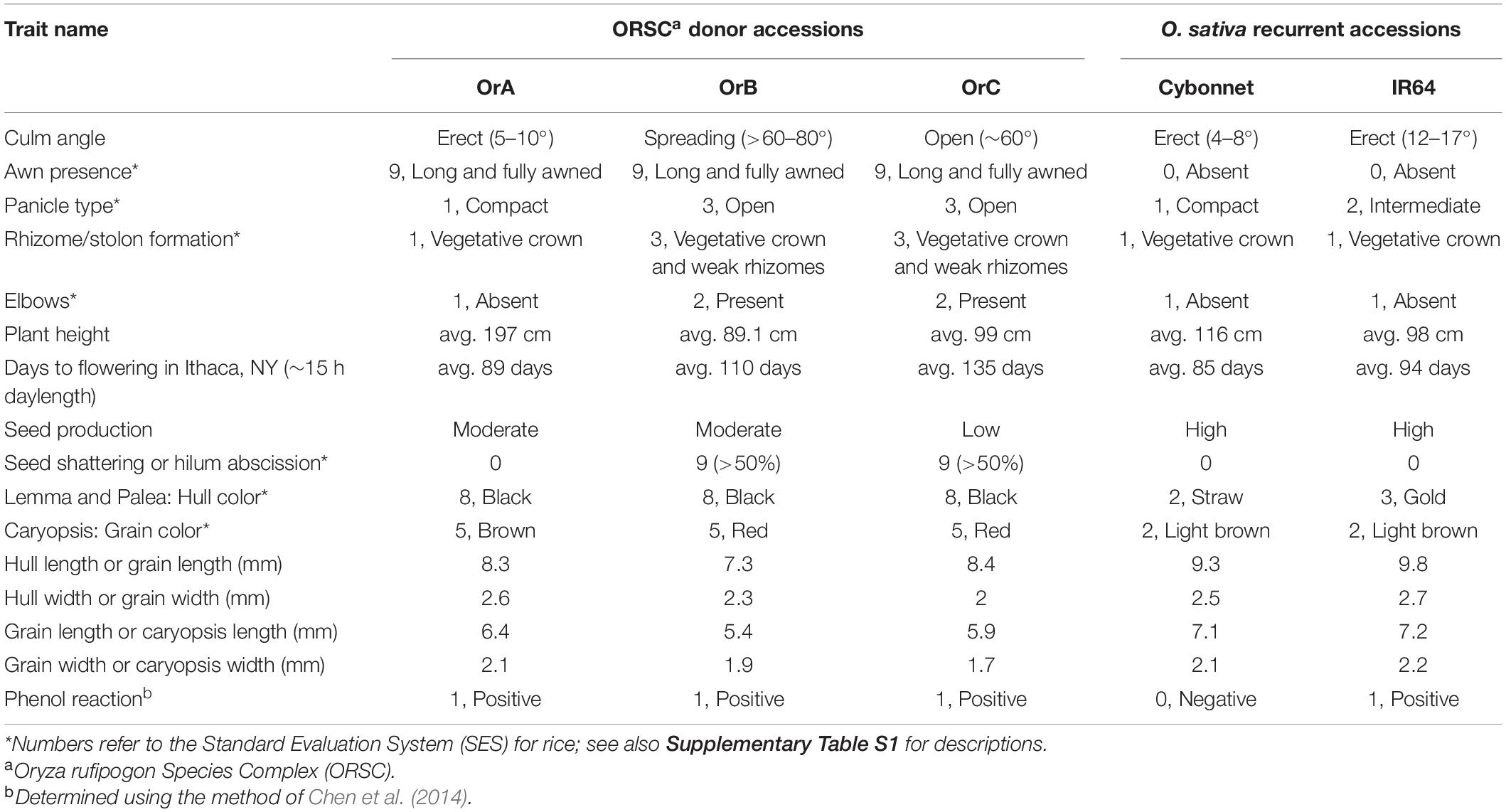
Table 2. List of traits evaluated under greenhouse conditions in the CSSL donors and recurrent parents.
Characterization of CSSL Libraries
Table 3 provides a summary of the six CSSL libraries in terms of number of lines, donor contribution, marker coverage, and percent heterozygosity. Individual libraries are comprised of 63–81 lines each, with an estimated percent donor contribution per line ranging from 2.9 to 6.9%, based on the total number of informative markers per library. Libraries constructed using OrA gave rise to populations with the largest number of spurious background introgressions per line compared to libraries constructed using OrB or OrC, though the reason for this is not clear (Figures 2, 3).
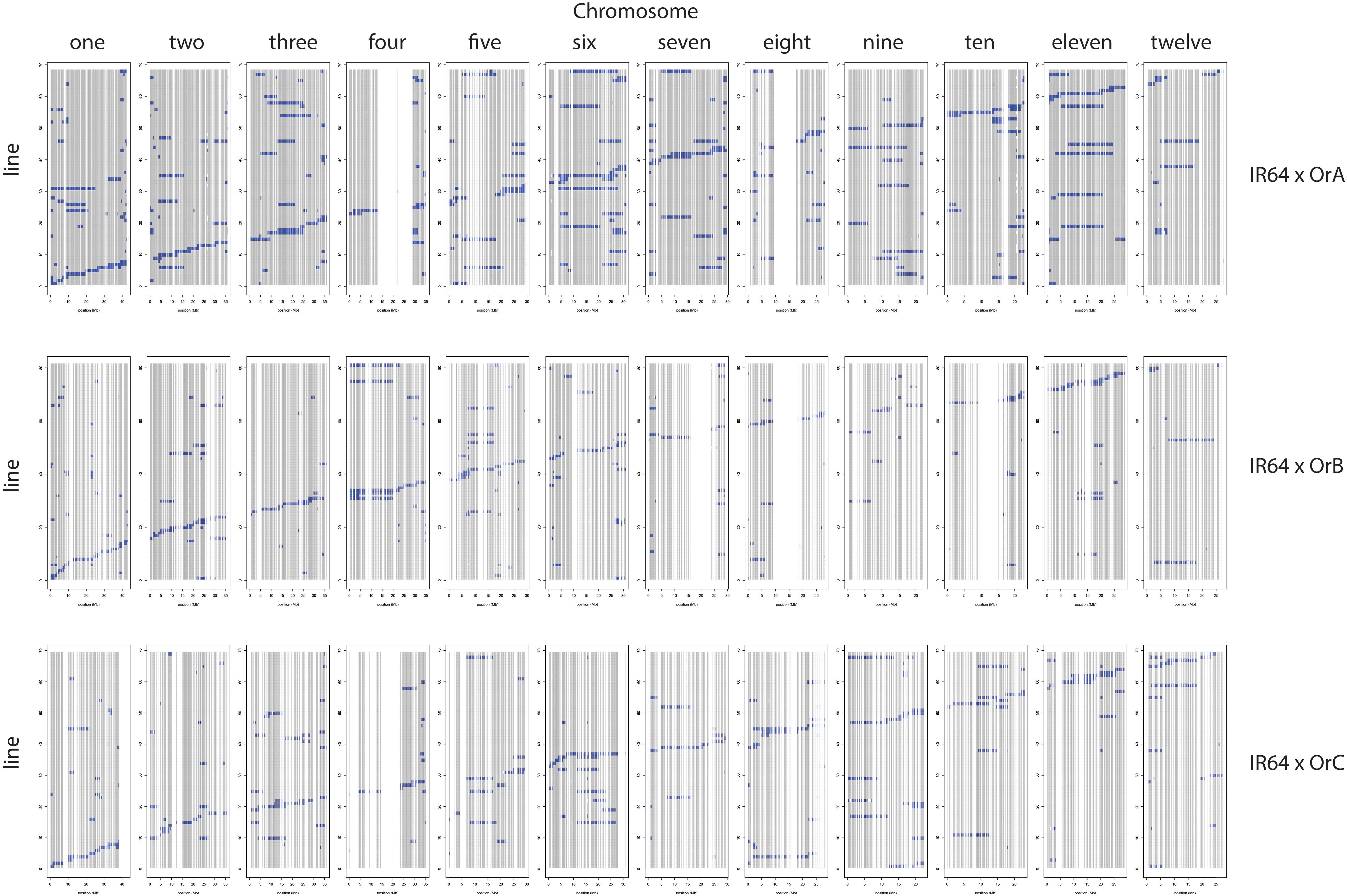
Figure 2. IR64 CSSL libraries using OrA (top), OrB (middle), and OrC (bottom) donors. The 12 chromosomes are identified along the top and the markers for each of the 12 chromosomes are represented as a “column” based on physical distance. The genotype of each CSSL (line) is represented as a “row” within the given library identified on the left side.
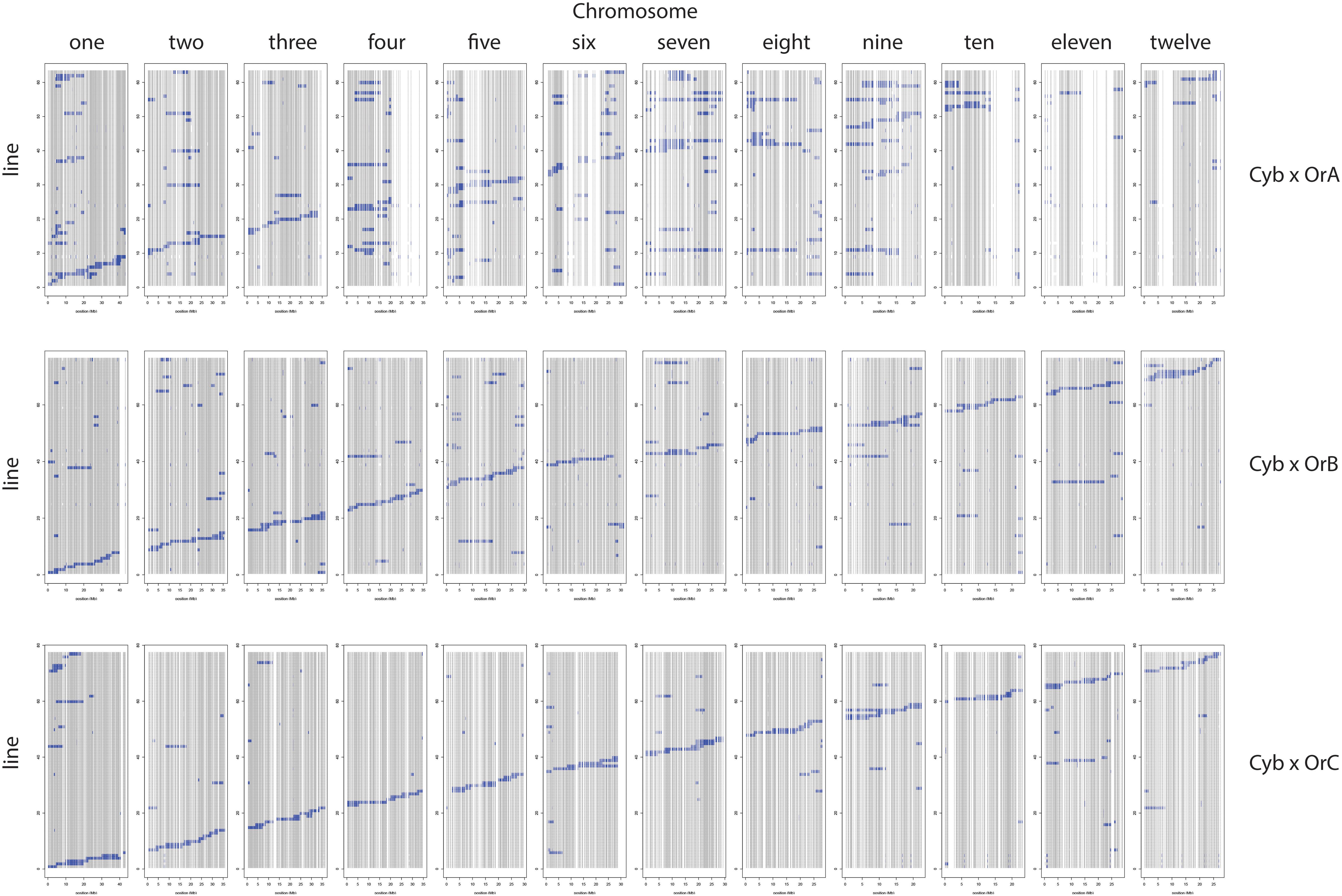
Figure 3. Cybonnet CSSL libraries using OrA (top), OrB (middle), and OrC (bottom) donors. The 12 chromosomes are identified along the top and the markers for each of the 12 chromosomes are represented as a “column” based on physical distance. The genotype of each CSSL (line) is represented as a “row” within the given library identified on the left side.
The number of informative SNPs per population ranged from 1,496 to 2,962, and was correlated with the degree of genetic relatedness between the parents. Thus, in the Cybonnet × OrA library, the number of SNPs detected was lower than in the Cybonnet × OrB or OrC libraries, and the reverse was true for the IR64 libraries. This was consistent with the ORSC subpopulation relationships reported by Kim et al. (2016) and with the phylogenetic analysis summarized in Figure 1. The average inter-marker distance is negatively correlated with marker number, but does not adequately portray the patterns of marker distribution observed in the different libraries; these are summarized in Figure 4. The large gaps in marker coverage are most pronounced in the IR64 populations (with maximum sizes of 8.3, 9.3, and 8.8 Mb in the OrA, OrB, and OrC populations, respectively), but are also apparent in the Cybonnet populations (with maximum sizes of 4.8, 3.3, and 1.9 Mb in the OrA, OrB, and OrC populations, respectively) (regions highlighted in green in Figure 4). On chromosome 4, a marker gap at 12,954,360–21,271,163 bp in the IR64 × OrA population was found to be partially shared with a gap at 14,482,427–23,294,656 bp in the IR64 × OrC and also with a gap from 20,352,551 bp until the end of the chromosome at 35,502,694 bp in the Cybonnet × OrA population. Other examples include the gaps on chromosome 8 observed from 9,289,473 to 17,516,403 bp in IR64 × OrA, 8,897,653–18,185,078 bp in IR64 × OrB, and 15,439,243–18,108,496 bp in IR64 × OrC. These regions that are devoid of informative markers are referred to as “SNP deserts,” and are not believed to be the result of major bias in the SNP assays, based on the fact that marker coverage in the Cybonnet × OrB and Cybonnet × OrC populations is fairly uniform and that apparent gaps in one population are invariably well covered regions in another. They are indicated as white spaces in the CSSL maps in Figures 2, 3. Overall, the amount of missing data after SNP filtering was very low, ranging from 0.03 to 0.32%, and the level of heterozygosity is <1% in all libraries.
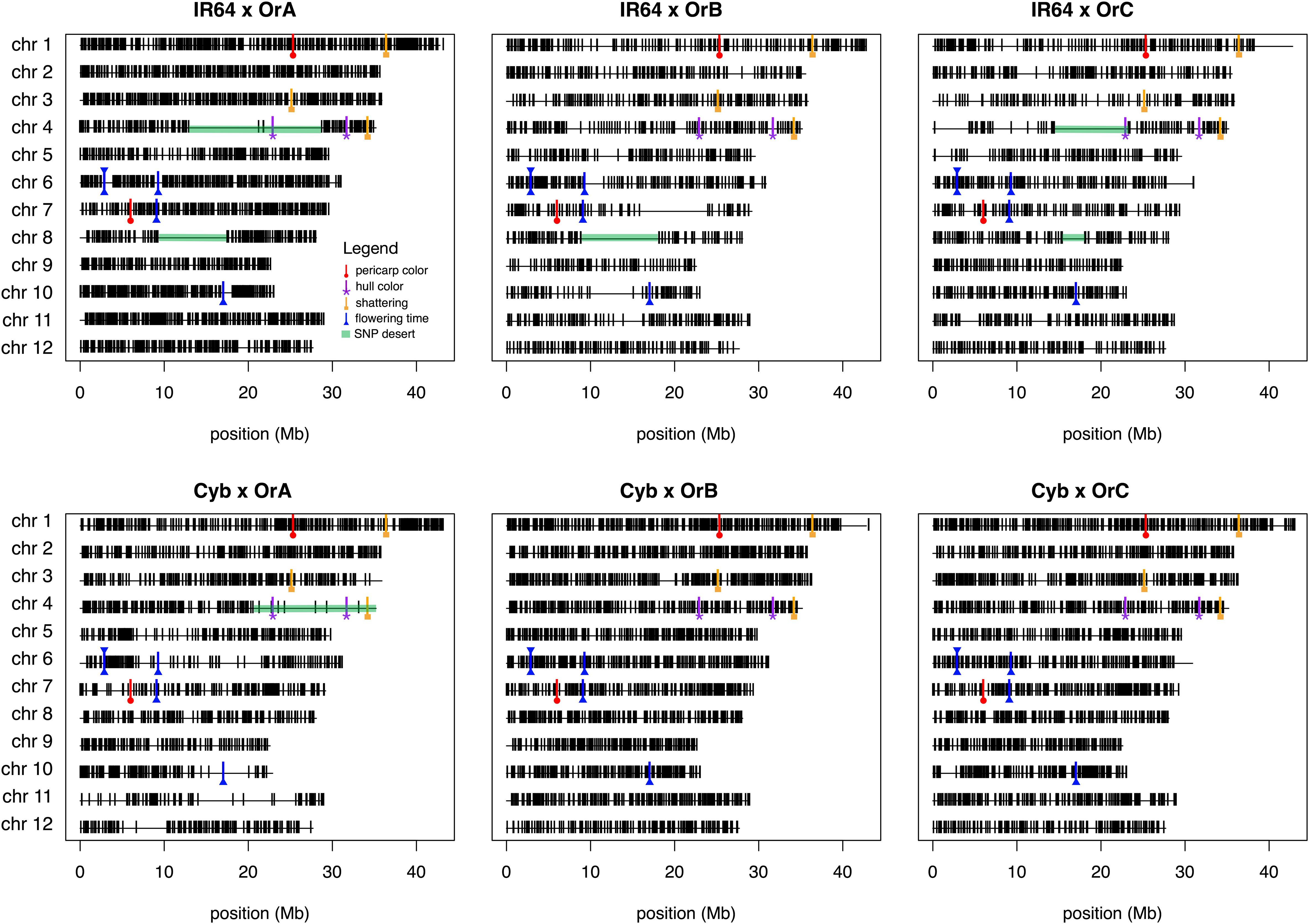
Figure 4. Physical distribution of markers using the C7AIR across the six finalized CSSL libraries. Total number of polymorphic markers in the populations ranged from 1496 (IR64 × OrB) to 2962 (IR64 × OrA). Visual gaps in marker distribution are due to either lack of polymorphism or poor SNP calling for which markers were filtered. Genes discussed in this manuscript are annotated using symbols shown in the legend: red line with circle for pericarp color genes (Rc, Rd); purple line with asterisk for hull color genes (Ph, BH4); orange line with square for shattering genes (SH4, qSH1, Sh1); and blue line with triangle flowering time genes (RFT, Hd1, Hd3a, Ehd1, Ghd7). Also indicated are marker gaps in green highlight that are discussed in the text.
We hypothesize that the “SNP deserts” may be due to one or more of the following: (1) these regions are common by state (cbs) and inferred to be common by descent (cbd) in the different cross combinations, thereby resulting in swaths of monomorphic SNPs; (2) sterility challenges led to irrecoverable introgressions, whereby target donor segments in one generation could not be propagated into future generations, resulting in an apparent lack of polymorphism in these regions in the final library dataset; and/or (3) heterogeneity in recurrent parent accessions used for crossing gave rise to polymorphic regions in final libraries that were difficult to attribute to either donor or recurrent parent and thus markers in these regions were dropped during SNP filtering (see section “Materials and Methods”). It is difficult to explicitly attribute marker gaps to cbd (hypothesis one) because the exact individuals used for crossing may not have been the same ones used for genotyping; however, given our current understanding of the genetic relationships between wild and cultivated rice subpopulations, we postulate that it is unlikely the gap on chromosome 4 shared between IR64 × OrA, IR64 × OrC, and Cybonnet × OrA libraries is a result of cbd. As an example of hypothesis two, we report efforts to maintain a line containing an introgression covering the region on chromosome 11 from 14 to 25.7 Mb in the Cybonnet × OrA population. The line was eventually lost due to sterility, and its absence from the final library led to apparent monomorphism in the genotyping dataset, explaining the marker gap in this region for that library (Figure 4). As for hypothesis three, within-line genetic heterogeneity is common in many improved varieties of rice, and is an essential characteristic of landrace and wild accessions found in genebanks (Olufowote et al., 1997). In this study, three different individuals per wild accession were used as pollen donors in crosses with multiple individuals from the two RPs. Regions in the genome where heterogenity is prevalent among individuals of an accession pose difficulties downstream in determining whether donor introgressions are present. Future attempts to carry out deep sequencing on these lines may likewise run into similar challenges in attributing alleles to donor or recurrent parent.
Wild Phenotypes Displayed in CSSL Lines
Depending on the specific donor segments carried by each of the CSSLs, wild characteristics are sometimes observed in the final lines. Classic domestication traits that help differentiate wild and cultivated rice include pericarp color, hull color, degree of seed shattering and flowering time. Some of these characteristics make the CSSLs difficult to grow and/or subject them to regulatory oversight, and thus knowing which lines carry “wild” traits is helpful in designing experiments to further evaluate the lines.
Pericarp Color
Out of the total 434 CSSLs comprising the six introgression libraries, 16 had pericarp color typically associated with wild rice (i.e., red or red/brown) (Supplementary Figure S2); 10 of these were lines in the Cybonnet background, and six were in the IR64 background. Five of the six libraries presented ILs with colored pericarp; the Cybonnet × OrC library was comprised only of white pericarp lines. Pericarp color is dependent upon the combination of alleles at the Rc (Os07g0211500) and Rd (Os01g0633500) loci, located on rice chromosomes 7 and 1, respectively (Table 4 and Figure 4). Both Rc and Rd must carry a dominant allele to produce red pericarp, whereas a dominant Rc allele and a recessive rd allele will produce brown pericarp. Any other combination (rc/rd, rc/Rd) will produce rice with white pericarp. All three donor accessions in these libraries had red pericarp and both RPs had white pericarp (Supplementary Figure S2). PCR results showed that both cultivated parents were homozygous recessive at the Rc locus; both carried the 14 bp deletion in exon 2 associated with lack of function for Rc, while all three wild parents carried the dominant (functional) allele at Rc. We next examined the Rd gene and found that Cybonnet carried the recessive (non-functional) allele but IR64 had the dominant Rd allele. OrB and OrC both carried the dominant (functional) allele at the Rd locus, but OrA was heterogeneous; some individuals carried the dominant allele and had red seeds, and others had brown seeds due to a recessive allele at Rd.
Supplementary Figure S2 shows CSSLs that have donor introgressions at the Rc and/or Rd genes, and indicates the pericarp color observed in the grain. Of the 16 CSSLs that carried pigmented pericarp, eight were from the Cyb × OrA library, and none were from the Cyb × OrC library. The lines were genotyped using the methods described above and the observed pericarp color was consistent with genetic predictions in the libraries derived from OrB and OrC in both the Cybonnet and the IR64 backgrounds. On the other hand, sequencing of the Rd gene in the CSSLs derived from OrA revealed two separate single nucleotide substitutions (mut#1 = A and mut#2 = G) in exon 2, each of which have previously been shown to render the gene non-functional (Sun et al., 2018). In the Cyb × OrA library, some CSSLs carried the wild type allele at Rd while others carried a non-functional rd allele; all lines with a non-functional allele carried mut#2 in exon2, while a few also carried mut#1. We observed a single line, Cyb_ OrA_4, with brown pericarp due to the fact that it carried a donor introgression at Rc on chromosome 7 and a second donor introgression on chromosome 1 carrying the rd allele with both mut#1 and mut#2. Other lines (Cyb_OrA_6, IR64_OrA_5) carrying the same donor allele at rd (with the two mutations) had white pericarp because they carried rc alleles from their respective RPs on chromosome 7 (Supplementary Figure S2).
Hull Color
The black hull phenotype is controlled by at least two complementary genes that are linked in a cluster of domestication-related genes on rice chromosome 4 (Fukuda et al., 2012). The gene Black Hull 4 (BH4) encodes a tyrosine transporter (Zhu et al., 2011), and Phenol Reaction (Ph) encodes the polyphenol oxidase (PPO) enzyme which uses tyrosine as a substrate (Yu et al., 2008; Table 4 and Figure 4). A third gene identified as OsbHLH016 is located between the other two genes on chromosome 4, and encodes a bHLH transcription factor. It is known to positively regulate the biosynthesis of anthocyanin and has been tentatively associated with black hull (Sun et al., 2018). Other genes for black hull have been reported on chromosomes 4, 5, and 7 (Morishima and Oka, 1981; Maekawa, 1984; Gu et al., 2005). Functional alleles at both BH4 and Ph are necessary to generate the black hull phenotype, but straw-white hull color has been selected under domestication. Multiple functional mutations have been documented in both genes, with clear divergence between the Indica and Japonica gene pools, resulting in straw-gold hull color in a majority of cultivated germplasm (Yu et al., 2008; Zhu et al., 2011; Fukuda et al., 2012).
In this study, the three donor parents all had black hulls, while both RPs had straw-gold hulls (Figure 1 and Table 2). To confirm which of the parental lines carried a functional Ph gene, seeds of the donors and RPs were tested for phenol reaction. All three wild donors and the IR64 RP displayed positive phenol reaction, while Cybonnet seeds showed no discoloration. Based on this evidence, we determined that Cybonnet carried the non-functional ph allele, while IR64 carried the functional Ph allele, consistent with a majority of Indica varieties. We further confirmed this result by sequencing the Ph locus. Sequencing demonstrated that Cybonnet carried a 1-bp insertion in exon 1 (chr 4: 31,749,302 bp) causing a frameshift that rendered the protein non-functional. This was somewhat unexpected, as a well known 18 bp loss-of-function deletion in exon 3 is more characteristic of japonica varieties (Yu et al., 2008). Sequencing of the BH4 gene confirmed that all three wild donor parents carried functional BH4 alleles, while Cybonnet and IR64 both carried non-functional alleles caused by a common 22 bp deletion in exon 3 (Zhu et al., 2011). We next assessed hull color in the CSSLs and found that only three lines, all from the IR64 × OrB library, carried the black hull phenotype (individuals IR64_OrB_34, IR64_OrB_35, and IR64_OrB_81). Each of the black hulled lines contained a donor introgression at the BH4 locus, but the introgression alone was not predictive of the phenotype; two lines from the same library carried donor introgressions at BH4 (individuals IR64_OrB_15 and IR64_OrB_21), but did not have black hulls (Supplementary Table S2). These results suggest that if functional alleles at Ph and BH4 alone were sufficient to cause black hull, any CSSL in the IR64 background (Ph/bh4) would need only a single introgression carrying a functional copy of Bh4 to manifest the black hull phenotype, while a CSSL in the Cybonnet background (ph/bh4) would require an extended donor introgression covering both genes (∼8.8 Mb region). As summarized in Supplementary Table S2, we identified four CSSLs in the Cybonnet × OrA population carrying an introgression that potentially encompasses both Ph and BH4; however, these lines did not have black hulls, suggesting that none of them carried both genes. The paucity of markers in the region (Figure 4) precludes explicit definition of the introgression breakpoints. Sequencing the Ph and BH4 regions of the five previously mentioned IR64 × OrB CSSLs potentially carrying the BH4 introgression would further elucidate the mechanism underlying the black hull phenotype.
Shattering
Several major shattering genes have been identified in rice (Cai and Morishima, 2000; Onishi et al., 2007). Shattering 4 (SH4) is a member of the trihelix family of transcription factors and lies near the end of the long arm of chromosome 4, linked to the two major black hull loci described above (Li et al., 2006; Lin et al., 2007). qSH1 is a BEL1-type homeobox-containing protein on chromosome 1 (Konishi et al., 2006). OsShattering 1 (OsSh1) is a YABBY transcription factor that was originally mapped as an ortholog of the Shattering1 gene in sorghum (Lin et al., 2012); it is co-located with qSH3, a shattering QTL on chromosome 3 (Inoue et al., 2015; Ishikawa et al., 2017; Table 4 and Figure 4).
While shattering was not measured quantitatively in this study, 14 CSSLs were noted to have an unusually high degree of shattering such that they required bagging of panicles to ensure seed harvest (Supplementary Table S2). Thirteen of the 14 shattering CSSLs carried a donor introgression at SH4 (Supplementary Table S2). The exception was CSSL “IR64_OrB_29” which had a shattering phenotype but did not carry donor alleles at SH4; however there was a donor introgression at the OsSh1 locus on chromosome 3. Nine CSSLs from three different libraries (five from the IR64 × OrA library, two from the IR64 × OrC library, and two from the Cybonnet × OrC library) carried donor introgressions at SH4 but were not observed to be shattering, and between 3 and 6 lines from each of the six libraries carried introgressions at OsSh1 and/or qSH1 with no obvious effect on the phenotype (Supplementary Table S2). We found no highly shattering phenotypes in the Cybonnet × OrA library, nor did we find any black hull phenotypes. This may be due to the fact that there was a paucity of SNP markers across the BH4–SH4 region of chromosome 4 in this library, relative to all other libraries, which made it virtually impossible to select for the presence of donor introgressions in that region during CSSL development (Figure 4).
Seed shattering is the result of a developmentally programmed weakening or degradation of the abscission cell layer at the base of the grain where it attaches to the pedicel, and abscission layer formation is known to be regulated by multiple genes that control the timing and degree of seed shattering (Inoue et al., 2015). Large variation in the degree of seed shattering is observed in rice cultivars, with Indica varieties typically exhibiting higher degrees of shattering than Japonica. Consistent with this observation, 12 of the shattering lines in this study were from the IR64 libraries while only two were from the Cybonnet libraries. These results suggest that the major-effect genes described above interact with other genes in the genetic background to determine the extent and degree of shattering, and that the pattern of interacting background alleles in IR64 (Indica) differs from Cybonnet (Japonica). Thus, despite the presence of donor introgressions at OsSh1/qSH3, qSH1 and SH4 in many of our shattering CSSLs, it is not surprising that these loci, alone, are not predictive of the phenotype. These results open the door to further research using these materials to gain a deeper understanding of the genetic determinants of seed shattering in rice.
Flowering Time
Eight CSSLs in the Cybonnet background were observed to have modified phenology and displayed later-than-expected flowering under the long days of summer in the greenhouse in Ithaca, NY (12–16 h of daylight). Four of the lines derived from crosses with OrA, which itself had the earliest flowering of the three wild donors, and two lines each from the other Cybonnet libraries. Data on flowering time under long day conditions is not available for the IR64 libraries. Studies of flowering time in rice have demonstrated that a combination of large- and small-effect genes define the genetic architecture of the trait, and adaptation to a wide range of daylength and temperature regimes is mediated by complex gene × gene interactions in both wild and cultivated populations. To date, more than a dozen flowering time genes have been cloned and characterized (OGRO database)6 (Yamamoto et al., 2012) and numerous additional small-effect QTLs have been extensively documented (Bentley et al., 2013; Itoh and Izawa, 2013; Hori et al., 2015).
In this study we identified lines carrying donor introgressions at five major flowering time loci located on chromosomes 6, 7, and 10 (Figure 4; Table 4; and Supplementary Table S2). The genes belong to two independent signaling pathways, as summarized in Hori et al. (2015). The first pathway, referred to as the OsGI-Hd1-Hd3a (rice GIGANTEA, Heading data 1, Heading date 3a) pathway, corresponds to the GI-CO-FT (GIGANTEA, CONSTANS, FLOWERING LOCUS T) pathway in Arabidopsis. Hd1 is a homolog of Arabidopsis CO and it promotes heading under short-day and represses it under long-day conditions (Yano et al., 2000). HD1 promotes the expression of both Hd3a and its tandemly duplicated paralog, Rice flowering locus T 1 (RFT1), under short-day conditions, but inhibits Hd3a and RFT1 under long-day conditions (Kojima et al., 2002). Hd3a and RFT1 function as florigens, or floral inducers (Tamaki et al., 2007; Ogiso-Tanaka et al., 2013). These three genes are all located on chromosome 6 in rice. The other pathway represents an independent signaling cascade that also regulates the florigens but is unique to rice. It includes Early heading date 1 (Ehd1) and Grain number, plant height and heading date 7 (Ghd7). Ehd1 is expressed only under short-days and it promotes flowering by inducing transcription of Hd3a and RFT1, functioning independently of Hd1 (Doi et al., 2004). Ghd7 represses Ehd1, Hd3a, and RFT1 under LD conditions, and does not affect Hd1 mRNA levels (Xue et al., 2008). Ehd1 is located on chromosome 10 and Ghd7 on chromosome 7 (Figure 4).
Chromosome segment substitution lines containing introgressions at these five loci are summarized in Supplementary Table S2. For the four late-flowering lines from the Cyb × OrA library, we observed no relationship between the phenotype and the presence of donor introgressions at any of the above-mentioned flowering time loci. However, for the Cyb × OrB and Cyb × OrC libraries, the presence of a donor introgression at the Hd1 locus was perfectly predictive of late flowering. In the case of Cyb_OrB_39, which was also late flowering, we see that the line carries an introgression across the Hd3a and RFT1 genes, but does not include Hd1. This suggests that donor alleles at other genes are responsible for the phenotype in this line.
Seed Production in CSSLs
During development of the CSSL libraries, we observed instances of low seed set (less than 200 seeds per plant under greenhouse or field conditions) in several lines per population (Supplementary Table S2). Overall, 28 lines were noted to have low levels of fertility (four in the Cyb × OrA, six in the Cyb × OrB, four in the Cyb × OrC, four in the IR64 × OrA, six in the IR64 × OrB, and four lines in the IR64 × OrC populations). Six lines in the Cybonnet × OrB and OrC libraries had low or no seed set in the field (Lafayette, LA, United States, 2019) but it was possible to obtain some seed set from the same plants grown under greenhouse conditions in Ithaca, NY, United States. Over the course of development, we also noticed that some lines were fertile in the heterozygous condition, but we were unable to harvest seed from homozygous offspring. In some of these cases, we were able to select sib lines that contained segment(s) of interest and produced seed when selfed, but over time, it became more difficult to find lines containing specific regions of introgression; in some cases, we lost segments entirely due to sterility (Supplementary Table S3). For example, in both the Cyb × OrB and IR64 × OrB libraries, a ∼650 kb region on chromosome 1 (41,985,829–42,630,129 bp) was only maintained as a heterozygous region and the seed set was always very low in the CSSLs carrying this introgression, indicative of the presence of a sterility gene(s). This region was implicated only in the OrB libraries, as sterility was not a problem in the corresponding lines developed from the OrA or the OrC donors. At this time, we have chosen to maintain some lines as backcross populations as an insurance policy to avoid losing additional segments. A case in point is Line Cyb_OrB_4 which contains six introgressions in chromosomes 1, 3, and 11, and is currently propagated by backcrossing to Cybonnet.
There are hundreds of loci known to contribute to varying levels of gametic and zygotic incompatibilities distributed throughout the 12 chromosomes of rice as noted in Oryzabase7 (Kurata and Yamazaki, 2006) and the OGRO database6; (Yamamoto et al., 2012). Most are population-specific, and this makes it difficult to associate the low fertility phenotypes with particular genes or introgressions in the CSSLs. Our objective in documenting CSSLs with low levels of seed set (as well as very late flowering) is to alert researchers which lines may be difficult to propagate and therefore require special attention. In some cases, we also alert users of these genetic resources that seeds of heterozygous backcross progeny or bulked seeds harvested from multiple generations of pure-line sibs may be distributed for particular lines.
Functional SNPs on the C7AIR and Predicted Phenotypes of the CSSLs
The C7AIR includes eight functional SNPs in its design that target important agronomic or physiological traits (Morales et al., 2020). They tag causal variants in genes conferring blast disease resistance (Pi-ta; Bryan et al., 2000), grain size (GS3; Fan et al., 2006; Takano-Kai et al., 2009), and eating and cooking quality (ALK, WAXY; Okagaki and Wessler, 1988; Wang et al., 1995; Gao et al., 2003). The parents of all six libraries were polymorphic for these variants, and CSSLs carrying donor SNPs at Pi-ta, GS3, ALK, and WAXY were documented (Supplementary Table S2). At GS3, both Cybonnet and IR64 carried the allele for long grain, while the three wild parents carried the wild type allele conferring short-medium grain length. At Pi-ta, the two RPs carried the allele for resistance while the wild parents carried the ancestral pi-ta allele conferring blast susceptibility (Huang et al., 2008). The evolution of resistance at the Pi-ta locus is believed to have been the result of a mutation that coincided with domestication, so it is not entirely surprising that none of the wild donors used in this study were found to carry the resistant allele (Lee et al., 2009; Yoshida and Miyashita, 2009). We were unable to predict grain quality phenotypes in the CSSLs because the traits determined by ALK and WAXY are quantitative, epistatic, and post-transcriptionally regulated (Bligh et al., 1998; Tian et al., 2009; Huggins et al., 2019). Evaluating these lines for novel grain and nutritional quality attributes is an exciting area for future research.
CSSL Identifier
To facilitate downstream use and evaluation of our six libraries by the greater rice genetics and breeding community, we developed a simple, user-friendly R Shiny Application that enables searching for lines of interest across user-selected libraries by inputting a genomic region of interest. The web application returns a comprehensive list of individual lines across the selected libraries that harbor introgressions either fully or partially across the region of interest from the donor accessions so that users may shortlist lines that they may potentially want to evaluate. Users may access the C7Air genotype data in Supplementary Dataset 2 or may also download C7AIR genotype data directly from the application for their filtered list of lines. This application is available at http://cssl-identifier.rcac.purdue.edu/ and is hosted by Purdue University Research Computing.
Utility
The design and development of these CSSLs represents only the first step in an ambitious conceptualization of a public pre-breeding program that serves to develop, test, and deploy novel variation, with the long-term goal of enabling breeders to more readily tap into the hidden potential of wild and exotic plant genetic resources. The materials developed here are available for distribution and offer researchers new opportunities to undertake complementary multi-year, multi-location trials for yield and agronomic performance, response to abiotic and biotic stresses, and quality traits important to the rice community. To maximize the potential for wide utility and interpretation, phenotypes must be collected using controlled vocabularies, with accompanying climatic metadata and management details to provide environmental context. Further, experimental data describing phenotypic, genotypic and environmental variation should be deposited into a common database or data management system to make those data findable, accessible, interoperable and re-usable (Wilkinson et al., 2016) so that benefits can be collectively realized.
Pre-breeding is inevitably a long-term value proposition. Benefits will continue to accrue over many years if efforts are carried out in partnership with applied breeding programs dedicated to finished variety development. A partnership model that leverages public and private sector resources would enable the large scale experiments that are needed to drive recombination via rapid-cycle recurrent selection in order to overcome linkage drag and give rise to novel sources of useful variation. Favorable new materials could then be extracted by breeders periodically for crossing with elite lines, while the essential cauldron of diverse alleles were continuously managed, simmered and stirred. The potential of a well-designed, thoughtfully implemented pre-breeding program that is fueled by complementary partnerships, creative benefit-sharing agreements, and strategic deployment of resources has far-reaching consequences for plant breeders and for agricultural communities world-wide. Such a pre-breeding program can drive innovation, motivate new thinking, and unleash strategies that iteratively create value by reinvesting in the deployment of natural variation across the landscape, bringing rewards for decades to come.
Data Availability Statement
The genotypic datasets presented in this study can be found in Supplementary Data 2—Genotype information (C7AIR) for CSSL populations. Sequencing data for grain and hull color genes from the wild and cultivated parents and selected CSSLs is available in GenBank with the following Accession IDs: Bh4 (MW310629 - MW310643), Rd (MW310644 - MW310658), Rc (MW310659 - MW310674), Ph (MW310675 - MW310688).
Author Contributions
NS, LA, HK, KA, SH, J-WK, ES, BM, S-NA, and GE contributed to the development of CSSL populations. NS, SH, GE, and HK phenotyped the plants. NS, KA, and SH genotyped the plants. YS and GD developed data management tools. VG and DW developed the CSSL Identifier R Shiny App. NS, DW, SH, GE, and SM analyzed the data and wrote the manuscript. SM conceptualized the project. All authors contributed to the article and approved the submitted version.
Funding
This project was funded by the National Science Foundation with grants to SM and GE (NSF-PGRP #0606461 and #1026555) and a Ph.D. fellowship award to DW (DGE-1144153), by USDA-CREES-AFRI Proposal 2009-01881 to SM, and by the Rural Development Administration, South Korea with a grant to S-NA “Cooperative Research Program for Agriculture Science and Technology Development (Project No. PJ01321401).”
Conflict of Interest
HK is currently employed by the company LG Chemical, Ltd.
The remaining authors declare that the research was conducted in the absence of any commercial or financial relationships that could be construed as a potential conflict of interest.
Acknowledgments
We mourn the passing of first author Namrata Singh from acute myeloid leukemia. We gratefully acknowledge Fumio Onishii, Lisa Harper, Janelle Jung, Jean Koski, and Greg Inzinna for their dedication and expert assistance managing rice plants in the greenhouse in Ithaca, NY, United States; Daniel E. Wood, Quynh P.-H. Grunden, and Erin N. Simpson for their dedication and expert technical assistance crossing and managing the rice plants, especially the IR64 populations, in Stuttgart, AR, United States; Francisco Agosto Perez for advice and consultation regarding development of the CSSL Identifier R Shiny App; members of the Eurofins Agrigenomics team for their technical support and expert genotyping services; Mark Wright from Cornell and Cindy Lawley and the team at Illumina for aid in design and production of the C6AIR and the C7AIR custom genotyping arrays, and Adam Famoso, Louisiana State University, Lafayette, LA for increasing seed of the Cybonnet CSSLs during summer 2019.
Supplementary Material
The Supplementary Material for this article can be found online at: https://www.frontiersin.org/articles/10.3389/fpls.2020.564824/full#supplementary-material
Footnotes
- ^ http://mapdisto.free.fr/CSSLFinder/
- ^ https://tassel.bitbucket.io/
- ^ https://www.R-project.org/
- ^ http://www.isbreeding.net/software/?type=detail&id=14
- ^ https://www.ars.usda.gov/GSOR
- ^ http://qtaro.abr.affrc.go.jp/ogro
- ^ https://shigen.nig.ac.jp/rice/oryzabase/
References
Balakrishnan, D., Surapaneni, M., Yadavalli, V. R., Addanki, K. R., Mesapogu, S., Beerelli, K., et al. (2020). Detecting CSSLs and yield QTLs with additive, epistatic and QTL×environment interaction effects from Oryza sativa×O. nivara IRGC81832 cross. Sci. Rep. 10:7766. doi: 10.1038/s41598-020-64300-0
Bentley, A. R., Jensen, E. F., Mackay, I. J., Honicka, H., Fladung, M., Hori, K., et al. (2013). “Flowering time,” in Genomics and Breeding for Climate-Resilient Crops, ed. C. Kole (Berling: Springer), 1–66. doi: 10.1007/978-3-642-37048-9_1
Bligh, H. F. J., Larkin, P. D., Roach, P. S., Jones, C. A., Fu, H., and Park, W. D. (1998). Use of alternate splice sites in granule-bound starch synthase mRNA from low-amylose rice varieties. Plant Mol. Biol. 38, 407–415. doi: 10.1023/A:1006021807799
Bryan, G. T., Wu, K. S., Farrall, L., Jia, Y., Hershey, H. P., McAdams, S. A., et al. (2000). tA single amino acid difference distinguishes resistant and susceptible alleles of the rice blast resistance gene Pi-ta. Plant Cell 12, 2033–2046. doi: 10.1105/tpc.12.11.2033
Burgarella, C., Barnaud, A., Kane, N. A., Jankowski, F., Scarcelli, N., Billot, C., et al. (2019). Adaptive introgression: an untapped evolutionary mechanism for crop adaptation. Front. Plant Sci. 10:4. doi: 10.3389/fpls.2019.00004
Cai, H., and Morishima, H. O. (2000). Genomic regions affecting seed shattering and seed dormancy in rice. Theor. Appl. Genet. 100, 840–846. doi: 10.1007/s001220051360
Chen, C., Wu, W., Xingming, S., Li, B., Hu, G., Zhang, Q., et al. (2014). Fine-mapping and candidate gene analysis of Black Hull 1 in rice (Oryza sativa L.). Plant Omics 7, 12–18.
Dellaporta, S. L., Wood, H., and Hicks, J. B. (1983). A plant DNA minipreparation. Plant Mol. Biol. Report. 1, 19–21. doi: 10.1007/bf02712670
Dempewolf, H., Baute, G. J., Anderson, J., Kilian, B., Smith, C., and Guarino, L. (2017). Past and future use of wild relatives in crop breeding. Crop Sci. 57, 1070–1082. doi: 10.2135/cropsci2016.10.0885
Dempewolf, H., Eastwood, R. J., Guarino, L., Khoury, C. K., Muller, J. V., and Toll, J. (2014). Adapting agriculture to climate change: a global initiative to collect, conserve, and use crop wild relatives. Agroecol. Sustain. Food Syst. 38, 369–377. doi: 10.1080/21683565.2013.870629
Dempewolf, H., Hodgins, K. A., Rummell, S. E., Ellstrand, N. C., and Rieseberg, L. H. (2012). Reproductive isolation during domestication. Plant Cell 24, 2710–2717. doi: 10.1105/tpc.112.100115
Doi, K., Izawa, T., Fuse, T., Yamanouchi, U., Kubo, T., Shimatani, Z., et al. (2004). Ehd1, a B-type response regulator in rice, confers short-day promotion of flowering and controls FT-like gene expression independently of Hd1. Genes Dev. 18, 926–936. doi: 10.1101/gad.1189604
Dwivedi, S., Upadhyaya, H., Stalker, H., Blair, M., Berioli, D., Nielen, S., et al. (2008). “Enhancing crop gene pools with beneficial traits using wild relatives,” in Plant Breeding Reviews, ed. J. Janick (Hoboken, NJ: John Wiley & Sons, Inc). doi: 10.1002/9780470380130.ch3
Eshed, Y., and Zamir, D. (1995). An introgression line population of Lycopersicon pennellii in the cultivated tomato enables the identification and fine mapping of yield-associated QTL. Genetics 141, 1147–1162.
Fan, C., Xing, Y. Z., Mao, H. L., Lu, T. T., Han, B., Xu, C., et al. (2006). GS3, a major QTL for grain length and weight and minor QTL for grain width and thickness in rice, encodes a putative transmembrane protein. Theor. Appl. Genet. 112, 1164–1171. doi: 10.1007/s00122-006-0218-1
Feynman, J., and Ruzmaikin, R. (2018). “Climate stability and the origin of agriculture,” in Climate Change and Agriculture, ed. S. Hussain (London: IntechOpen). doi: 10.5772/intechopen.83344
Fita, A., Rodriguez-Burruezo, A., Boscaiu, M., Prohens, J., and Vicente, O. (2015). Breeding and domesticating crops adapted to drought and salinity: a new paradigm for increasing food production. Front. Plant Sci. 6:978. doi: 10.3389/fpls.2015.00978
Food and Agriculture Organization of the United Nations (2020). FAOSTAT Database. Rome: Food and Agriculture Organization of the United Nations.
Frey, K., Cox, T., Rodgers, D., and Bramel-Cox, P. (1984). “Increasing cereal yields with genes from wild and weedy species,” in Genetics, New Frontiers, ed. V. E. A. Chopra (Oxford: Oxford & IBH Publishing), 51–68.
Fukuda, A., Shimizu, H., Shiratsuchi, H., Yamaguchi, H., Ohdaira, Y., and Mochida, H. (2012). Complementary genes that cause black ripening hulls in F1 plants of crosses between Indica and Japonica rice cultivars. Plant Prod. Sci. 15, 270–273. doi: 10.1626/pps.15.270
Fulton, T. M., Chunwongse, J., and Tanksley, S. D. (1995). Microprep protocol for extraction of DNA from tomato and other herbaceous plants. Plant Mol. Biol. Rep. 13, 207–209. doi: 10.1007/BF02670897
Furukawa, J., Yamaji, N., Wang, H., Mitani, N., Murata, Y., Sato, K., et al. (2007). An aluminum-activated citrate transporter in barley. Plant Cell Physiol. 48, 1081–1091. doi: 10.1093/pcp/pcm091
Gao, Z., Zeng, D., Cui, X., Zhou, Y., Yan, M., Huang, D., et al. (2003). Map-based cloning of the ALK gene, which controls the gelatinization temperature of rice. Sci. China C Life Sci. 46, 661–668. doi: 10.1360/03yc0099
Gibbons, J. W., Anders, M., Bryant, R., Cartwright, R., Norman, R. J., Meullenet, J. F., et al. (2006). Registration of ‘Cybonnet’ rice. Crop Sci. 46, 2317–2318. doi: 10.2135/cropsci2006.03.0173
Godfray, H. C., Beddington, J. R., Crute, I. R., Haddad, L., Lawrence, D., Muir, J. F., et al. (2010). Food security: the challenge of feeding 9 billion people. Science 327, 812–818. doi: 10.1126/science.1185383
Gu, X. Y., Kianian, S. F., Hareland, G. A., Hoffer, B. L., and Foley, M. E. (2005). Genetic analysis of adaptive syndromes interrelated with seed dormancy in weedy rice (Oryza sativa). Theor. Appl. Genet. 110, 1108–1118. doi: 10.1007/s00122-005-1939-2
Guo, Y. L., and Ge, S. (2005). Molecular phylogeny of Oryzeae (Poaceae) based on DNA sequences from chloroplast, mitochondrial, and nuclear genomes. Am. J. Bot. 92, 1548–1558. doi: 10.3732/ajb.92.9.1548
Gur, A., and Zamir, D. (2004). Unused natural variation can lift yield barriers in plant breeding. PLoS Biol. 2:e245. doi: 10.1371/journal.pbio.0020245
Hajjar, R., and Hodgkin, T. (2007). The use of wild relatives in crop improvement: a survey of developments over the last 20 years. Euphytica 156, 1–13. doi: 10.1007/s10681-007-9363-0
Haussmann, B., Parzies, H., Presterl, T., Susic, Z., and Miedaner, T. (2004). Plant genetic resources in crop improvement. Plant Genet. Resour. 2, 3–21.
Hori, K., Nonoue, Y., Ono, N., Shibaya, T., Ebana, K., Matsubara, K., et al. (2015). Genetic architecture of variation in heading date among Asian rice accessions. BMC Plant Biol. 15:115. doi: 10.1186/s12870-015-0501-x
Huang, C. L., Hwang, S. Y., Chiang, Y. C., and Lin, T. P. (2008). Molecular evolution of the Pi-ta gene resistant to rice blast in wild rice (Oryza rufipogon). Genetics 179, 1527–1538. doi: 10.1534/genetics.108.089805
Huggins, T. D., Chen, M. H., Fjellstrom, R. G., Jackson, A. K., McClung, A. M., and Edwards, J. D. (2019). Association analysis of three diverse rice (Oryza sativa L.) germplasm collections for loci regulating grain quality traits. Plant Genome 12, 1–21. doi: 10.3835/plantgenome2017.09.0085
Inoue, C., Htun, T. M., Inoue, K., Ikeda, K., Ishii, T., and Ishikawa, R. (2015). Inhibition of abscission layer formation by an interaction of two seed-shattering loci, sh4 and qSH3, in rice. Genes Genet. Syst. 90, 1–9. doi: 10.1266/ggs.90.1
Ishikawa, R., Nishimura, A., Htun, T. M., Nishioka, R., Oka, Y., Tsujimura, Y., et al. (2017). Estimation of loci involved in non-shattering of seeds in early rice domestication. Genetica 145, 201–207. doi: 10.1007/s10709-017-9958-x
Itoh, H., and Izawa, T. (2013). The coincidence of critical day length recognition for florigen gene expression and floral transition under long-day conditions in rice. Mol. Plant 6, 635–649. doi: 10.1093/mp/sst022
Khush, G. S. (2005). What it will take to feed 5.0 billion rice consumers in 2030. Plant Mol. Biol. 59, 1–6. doi: 10.1007/s11103-005-2159-5
Kim, H., Jung, J., Singh, N., Greenberg, A., Doyle, J. J., Tyagi, W., et al. (2016). Population dynamics among six major groups of the Oryza rufipogon species complex, wild relative of cultivated Asian rice. Rice 9:56.
Kojima, S., Takahashi, Y., Kobayashi, Y., Monna, L., Sasaki, T., Araki, T., et al. (2002). Hd3a, a rice ortholog of the Arabidopsis FT gene, promotes transition to flowering downstream of Hd1 under short-day conditions. Plant Cell Physiol. 43, 1096–1105. doi: 10.1093/pcp/pcf156
Konishi, S., Izawa, T., Lin, S. Y., Ebana, K., Fukuta, Y., Sasaki, T., et al. (2006). An SNP caused loss of seed shattering during rice domestication. Science 312, 1392–1396. doi: 10.1126/science.1126410
Kurata, N., and Yamazaki, Y. (2006). Oryzabase. An integrated biological and genome information database for rice. Plant Physiol. 140, 12–17. doi: 10.1104/pp.105.063008
Lee, S., Costanzo, S., Jia, Y., Olsen, K. M., and Caicedo, A. L. (2009). Evolutionary dynamics of the genomic region around the blast resistance gene Pi-ta in AA genome Oryza species. Genetics 183, 1315–1325. doi: 10.1534/genetics.109.108266
Li, A., Liu, D., Yang, W., Kishii, M., and Mao, L. (2018). Synthetic hexaploid wheat: yesterday, today, and tomorrow. Engineering 4, 552–558. doi: 10.1016/j.eng.2018.07.001
Li, C., Zhou, A., and Sang, T. (2006). Rice domestication by reducing shattering. Science 311, 1936–1939. doi: 10.1126/science.1123604
Lin, Z., Griffith, M. E., Li, X., Zhu, Z., Tan, L., Fu, Y., et al. (2007). Origin of seed shattering in rice (Oryza sativa L.). Planta 226, 11–20.
Lin, Z., Li, X., Shannon, L. M., Yeh, C. T., Wang, M. L., Bai, G., et al. (2012). Parallel domestication of the Shattering1 genes in cereals. Nat. Genet. 44, 720–724. doi: 10.1038/ng.2281
Mackill, D. J., and Khush, G. S. (2018). IR64: a high-quality and high-yielding mega variety. Rice 11:18.
Maekawa, M. (1984). Geographical distribution of the genes for black hull coloration. Rice Genet. Newsl. 1, 104–105.
Mammadov, J., Buyyarapu, R., Guttikonda, S. K., Parliament, K., Abdurakhmonov, I. Y., and Kumpatla, S. P. (2018). Wild relatives of maize, rice, cotton, and soybean: treasure troves for tolerance to biotic and abiotic stresses. Front. Plant Sci. 9:886. doi: 10.3389/fpls.2018.00886
McCouch, S. R., Sweeney, M., Li, J. M., Jiang, H., Thomson, M., Septiningsih, E., et al. (2007). Through the genetic bottleneck: O-rufipogon as a source of trait-enhancing alleles for O-sativa. Euphytica 154, 317–339. doi: 10.1007/s10681-006-9210-8
McCouch, S. R., Teytelman, L., Xu, Y., Lobos, K. B., Clare, K., Walton, M., et al. (2002). Development and mapping of 2240 new SSR markers for rice (Oryza sativa L.) (supplement). DNA Res. 9, 257–279. doi: 10.1093/dnares/9.6.257
Migicovsky, Z., and Myles, S. (2017). Exploiting wild relatives for genomics-assisted breeding of perennial crops. Front. Plant Sci. 8:460. doi: 10.3389/fpls.2017.00460
Morales, K. Y., Singh, N., Perez, F. A., Ignacio, J. C., Thapa, R., Arbelaez, J. D., et al. (2020). An improved 7K SNP array, the C7AIR, provides a wealth of validated SNP markers for rice breeding and genetics studies. PLoS One 15:e0232479. doi: 10.1371/journal.pone.0232479
Morishima, H. O., and Oka, H. (1981). Phylogenetic differentiation of cultivated rice, xxii. Numerical evaluation of the Indica-Japonica differentiation. Jpn. J. Breed. 31, 402–413. doi: 10.1270/jsbbs1951.31.402
Ogiso-Tanaka, E., Matsubara, K., Yamamoto, S., Nonoue, Y., Wu, J., Fujisawa, H., et al. (2013). Natural variation of the RICE FLOWERING LOCUS T 1 contributes to flowering time divergence in rice. PLoS One 8:e75959. doi: 10.1371/journal.pone.0075959
Okagaki, R. J., and Wessler, S. R. (1988). Comparison of non-mutant and mutant waxy genes in rice and maize. Genetics 120, 1137–1143.
Olufowote, J. O., Xu, Y., Chen, X., Park, W. D., Beachell, H. M., Dilday, R. H., et al. (1997). Comparative evaluation of within-cultivar variation of rice (Oryza sativa L.) using microsatellite and RFLP markers. Genome 40, 370–378. doi: 10.1139/g97-050
Onishi, K., Horiuchi, Y., Ishigoh-Oka, N., Takagi, K., Ichikawa, N., Maruoka, M., et al. (2007). A QTL cluster for plant architecture and its ecological significance in Asian wild rice. Breed. Sci. 57, 7–16. doi: 10.1270/jsbbs.57.7
Orjuela, J., Garavito, A., Bouniol, M., Arbelaez, J. D., Moreno, L., Kimball, J., et al. (2010). A universal core genetic map for rice. Theor. Appl. Genet. 120, 563–572. doi: 10.1007/s00122-009-1176-1
Pilling, D., Belanger, J., and Hoffmann, I. (2020). Declining biodiversity for food and agriculture needs urgent global action. Nat. Food 1, 144–147. doi: 10.1038/s43016-020-0040-y
Prohens, J., Gramazio, P., Plazas, M., Dempewolf, H., Kilian, B., Diez, M., et al. (2017). Introgressiomics: a new approach for using crop wild relatives in breeding for adaptation to climate change. Euphytica 213:158.
Redden, R. J., Yadav, S. S., Maxted, N., Dulloo, M. E., Guarino, L., and Smith, P. F. (eds). (2015). Crop Wild Relatives and Climate Change. Hoboken, NJ: Wiley-Blackwell.
Rosenzweig, C., Elliott, J., Deryng, D., Ruane, A. C., Muller, C., Arneth, A., et al. (2014). Assessing agricultural risks of climate change in the 21st century in a global gridded crop model intercomparison. Proc. Natl. Acad. Sci. U.S.A. 111, 3268–3273. doi: 10.1073/pnas.1222463110
Rosyara, U., Kishii, M., Payne, T., Sansaloni, C. P., Singh, R. P., Braun, H. J., et al. (2019). Genetic contribution of synthetic hexaploid wheat to CIMMYT’s spring bread wheat breeding germplasm. Sci. Rep. 9:12355.
Stein, J. C., Yu, Y., Copetti, D., Zwickl, D. J., Zhang, L., Zhang, C., et al. (2018). Genomes of 13 domesticated and wild rice relatives highlight genetic conservation, turnover and innovation across the genus Oryza. Nat. Genet. 50, 285–296.
Street, K., Bari, A., Mackay, M., and Amri, A. (2016). “How the focused identification of germplasm strategy (FIGS) is used to mine plant genetic resources collections for adaptive traits,” in Enhancing Crop Genepool Use: Capturing Wild Relative and Landrace Diversity for Crop Improvement, eds N. Maxted, M. Dulloo, and B. Ford-Lloyd (Wallingford: CABI), 54–65. doi: 10.1079/9781780646138.0054
Sun, X., Zhang, Z., Chen, C., Wu, W., Ren, N., Jiang, C., et al. (2018). The C-S-A gene system regulates hull pigmentation and reveals evolution of anthocyanin biosynthesis pathway in rice. J. Exp. Bot. 69, 1485–1498. doi: 10.1093/jxb/ery001
Sweeney, M., Thomson, M., Pfeil, B., and McCouch, S. (2006). Caught red-handed: Rc encodes a basic helix-loop-helix protein conditioning red pericarp in rice. Plant Cell 18, 283–294. doi: 10.1105/tpc.105.038430
Takano-Kai, N., Jiang, H., Kubo, T., Sweeney, M., Matsumoto, T., Kanamori, H., et al. (2009). Evolutionary history of GS3, a gene conferring grain length in rice. Genetics 182, 1323–1334. doi: 10.1534/genetics.109.103002
Tamaki, S., Matsuo, S., Wong, H. L., Yokoi, S., and Shimamoto, K. (2007). Hd3a protein is a mobile flowering signal in rice. Science 316, 1033–1036. doi: 10.1126/science.1141753
Tamura, K., and Nei, M. (1993). Estimation of the number of nucleotide substitutions in the control region of mitochondrial DNA in humans and chimpanzees. Mol. Biol. Evol. 10, 512–526. doi: 10.1093/oxfordjournals.molbev.a040023
Tanksley, S. D., and McCouch, S. R. (1997). Seed banks and molecular maps: unlocking genetic potential from the wild. Science 277, 1063–1066. doi: 10.1126/science.277.5329.1063
Thomson, M. J., Singh, N., Dwiyanti, M. S., Wang, D. R., Wright, M. H., Perez, F. A., et al. (2017). Large-scale deployment of a rice 6 K SNP array for genetics and breeding applications. Rice 10:40.
Thomson, M. J., Zhao, K. Y., Wright, M., McNally, K. L., Rey, J., Tung, C. W., et al. (2012). High-throughput single nucleotide polymorphism genotyping for breeding applications in rice using the BeadXpress platform. Mol. Breed. 29, 875–886. doi: 10.1007/s11032-011-9663-x
Tian, Z., Qian, Q., Liu, Q., Yan, M., Liu, X., Yan, C., et al. (2009). Allelic diversities in rice starch biosynthesis lead to a diverse array of rice eating and cooking qualities. Proc. Natl. Acad. Sci. U.S.A. 106, 21760–21765. doi: 10.1073/pnas.0912396106
Tilman, D., Cassman, K. G., Matson, P. A., Naylor, R., and Polasky, S. (2002). Agricultural sustainability and intensive production practices. Nature 418, 671–677. doi: 10.1038/nature01014
Toriyama, K., and Kazama, T. (2016). Development of cytoplasmic male sterile IR24 and IR64 using CW-CMS/Rf17 system. Rice 9:22.
United Nations Department of Economic and Social Affairs (2017). World Population Prospects. New York, NY: United Nations Department of Economic and Social Affairs.
van Berloo, R. (2008). GGT 2.0: versatile software for visualization and analysis of genetic data. J. Hered. 99, 232–236. doi: 10.1093/jhered/esm109
Vaughan, D. A., Lu, B.-R., and Tomooka, N. (2008). The evolving story of rice evolution. Plant Sci. 174, 394–408. doi: 10.1016/j.plantsci.2008.01.016
Virmani, S. S., and Kumar, I. (2004). Development and use of hybrid rice technology to increase rice productivity in the tropics. Int. Rice Res. Notes 29, 10–19.
Wang, D. R., Han, R., Wolfrum, E. J., and McCouch, S. R. (2017). The buffering capacity of stems: genetic architecture of nonstructural carbohydrates in cultivated Asian rice, Oryza sativa. New Phytol. 215, 658–671. doi: 10.1111/nph.14614
Wang, Z., Zheng, F., Shen, G., Gao, J., Snustad, D., Li, M., et al. (1995). The amylose content in rice endosperm is related to the post transcriptional regulation of the waxy gene. Plant J. 7, 613–622. doi: 10.1046/j.1365-313x.1995.7040613.x
Warschefsky, E., Penmetsa, R. V., Cook, D. R., and von Wettberg, E. J. (2014). Back to the wilds: tapping evolutionary adaptations for resilient crops through systematic hybridization with crop wild relatives. Am. J. Bot. 101, 1791–1800. doi: 10.3732/ajb.1400116
Wilkinson, M. D., Dumontier, M., Aalbersberg, I. J., Appleton, G., Axton, M., Baak, A., et al. (2016). The FAIR guiding principles for scientific data management and stewardship. Sci. Data 3:160018. doi: 10.1038/sdata.2016.18
Xue, W., Xing, Y., Weng, X., Zhao, Y., Tang, W., Wang, L., et al. (2008). Natural variation in Ghd7 is an important regulator of heading date and yield potential in rice. Nat. Genet. 40, 761–767. doi: 10.1038/ng.143
Yamamoto, E., Yonemaru, J., Yamamoto, T., and Yano, M. (2012). OGRO: the overview of functionally characterized genes in rice online database. Rice 5:26. doi: 10.1186/1939-8433-5-26
Yano, M., Katayose, Y., Ashikari, M., Yamanouchi, U., Monna, L., Fuse, T., et al. (2000). Hd1, a major photoperiod sensitivity quantitative trait locus in rice, is closely related to the Arabidopsis flowering time gene CONSTANS. Plant Cell 12, 2473–2483. doi: 10.2307/3871242
Yoshida, K., and Miyashita, N. T. (2009). DNA polymorphism in the blast disease resistance gene Pita of the wild rice Oryza rufipogon and its related species. Genes Genet. Syst. 84, 121–136. doi: 10.1266/ggs.84.121
Yu, Y., Tang, T., Qian, Q., Wang, Y., Yan, M., Zeng, D., et al. (2008). Independent losses of function in a polyphenol oxidase in rice: differentiation in grain discoloration between subspecies and the role of positive selection under domestication. Plant Cell 20, 2946–2959. doi: 10.1105/tpc.108.060426
Zamir, D. (2001). Improving plant breeding with exotic genetic libraries. Nat. Rev. Genet. 2, 983–989. doi: 10.1038/35103590
Keywords: Oryza sativa, crop wild relatives, Oryza rufipogon Species Complex, chromosome segment substitution line, pre-breeding resources
Citation: Singh N, Wang DR, Ali L, Kim H, Akther KM, Harrington SE, Kang J-W, Shakiba E, Shi Y, DeClerck G, Meadows B, Govindaraj V, Ahn S-N, Eizenga GC and McCouch SR (2020) A Coordinated Suite of Wild-Introgression Lines in Indica and Japonica Elite Backgrounds. Front. Plant Sci. 11:564824. doi: 10.3389/fpls.2020.564824
Received: 22 May 2020; Accepted: 12 October 2020;
Published: 12 November 2020.
Edited by:
Yohei Koide, Hokkaido University, JapanReviewed by:
Naoki Hirotsu, Toyo University, JapanWricha Tyagi, Central Agricultural University, India
Copyright © 2020 Singh, Wang, Ali, Kim, Akther, Harrington, Kang, Shakiba, Shi, DeClerck, Meadows, Govindaraj, Ahn, Eizenga and McCouch. This is an open-access article distributed under the terms of the Creative Commons Attribution License (CC BY). The use, distribution or reproduction in other forums is permitted, provided the original author(s) and the copyright owner(s) are credited and that the original publication in this journal is cited, in accordance with accepted academic practice. No use, distribution or reproduction is permitted which does not comply with these terms.
*Correspondence: Susan R. McCouch, c3JtNEBjb3JuZWxsLmVkdQ==; Georgia C. Eizenga, Z2VvcmdpYS5laXplbmdhQHVzZGEuZ292
†ORCID: Liakat Ali, orcid.org/0000-0002-1180-5846; Ehsan Shakiba, orcid.org/0000-0001-7264-6351; Diane R. Wang, orcid.org/0000-0002-2290-3257
‡These authors share first authorship
§Present address: Diane R. Wang, Department of Agronomy, Purdue University, West Lafayette, IN, United States; Liakat Ali, Department of Plant Sciences, University of Missouri-Columbia Delta Center, Portageville, MO, United States; HyunJung Kim, LG Chemical, Ltd., Seoul, South Korea; Ju-Won Kang, Department of Southern Area Crop Science, National Institute of Crop Science, RDA, Miryang, South Korea