- Plant Biology Section, School of Integrative Plant Science, Cornell University, Ithaca, NY, United States
Plant intracellular immune receptor NLR (nucleotide-binding leucine-rich repeat) proteins sense the presence of pathogens and trigger strong and robust immune responses. NLR genes are known to be tightly controlled at the protein level, but little is known about their dynamics at the transcript level. In this study, we presented a meta-analysis of transcript dynamics of all 207 NLR genes in the Col-0 accession of Arabidopsis thaliana under various biotic and abiotic stresses based on 88 publicly available RNA sequencing datasets from 27 independent studies. We find that about two thirds of the NLR genes are generally induced by pathogens, immune elicitors, or salicylic acid (SA), suggesting that transcriptional induction of NLR genes might be an important mechanism in plant immunity regulation. By contrast, NLR genes induced by biotic stresses are often repressed by abscisic acid, high temperature and drought, suggesting that transcriptional regulation of NLR genes might be important for interaction between abiotic and biotic stress responses. In addition, pathogen-induced expression of some NLR genes are dependent on SA induction. Interestingly, a small group of NLR genes are repressed under certain biotic stress treatments, suggesting an unconventional function of this group of NLRs. This meta-analysis thus reveals the transcript dynamics of NLR genes under biotic and abiotic stress conditions and suggests a contribution of NLR transcript regulation to plant immunity as well as interactions between abiotic and biotic stress responses.
Introduction
Plants in nature are constantly challenged by a variety of environmental stresses including pathogen attacks. In order to fend off pathogens, plants utilize cell-surface receptors and intracellular immune receptors to sense the presence of microbes (Wang et al., 2020). The recognition of pathogens by immune receptors triggers a series of immune responses such as reactive oxygen species burst, Ca2+ influx, accumulation of salicylic acid (SA), and transcriptional reprograming (Tsuda and Katagiri, 2010; Buscaill and Rivas, 2014). Transcriptional upregulation of defense genes and reduction of growth-related genes are critical for a successful inhibition or blocking of invasion and propagation of pathogens (Lewis et al., 2015). The plant hormone SA is often induced during defense responses, and key enzymes for SA biosynthesis are regulated by a few transcription factors. SAR DEFICIENT 1 (SARD1) and its close homolog CALMODULIN BINDING PROTEIN 60-LIKE G (CBP60g) are recruited directly to the promoter of ISOCHORISMATE SYNTHASE 1 (ICS1), a key SA biosynthesis gene, to promote its transcription after pathogen infection (Zhang et al., 2010; Wang et al., 2011). ICS1 can also be bound by members of the TEOSINTE BRANCHED 1/CYCLOIDEA/PCF (TCP) transcription factor family with TCP8 and TCP9 exhibiting the strongest ICS1-promoter binding activity (Wang et al., 2015). In addition to positive regulators, several negative regulators have been identified in regulating ICS1 transcription, and they include ETHYLENE INSENSITIVE 3 (EIN3), EIN3-Like 1 (EIL1) (Chen et al., 2009), and NAC (NAM/ATAF1, ATAF2/CUC2) 19 (Zheng et al., 2012). Interestingly, TCPs interact with SARD1 and NAC019, and some TCPs are induced while others are repressed after pathogen infection (Wang et al., 2015). This suggests that a high-order transcription factor complex is orchestrating and fine-tuning ICS1 transcription in response to pathogen infection.
Much less is known about the transcript control of plant intracellular immune receptor genes or NLR (nucleotide-binding leucine-rich repeat) genes during defense responses. Plant NLR proteins consist of highly conserved central nucleotide-binding site (NBS), C-terminal leucine-rich repeats (LRRs), and variable N-termini. The N-terminal domain divides NLRs into three groups: toll-interleukin 1 receptor-NLR (TNL), coiled-coil-NLR (CNL), and RPW8-type CC-NLR (RNL) (Monteiro and Nishimura, 2018). They constitute one of the biggest gene families in plants and exhibit great genetic diversity within and among plant species (Van de Weyer et al., 2019; Kourelis and Kamoun, 2020). In the Col-0 accession of Arabidopsis thaliana, 207 NLR and NLR-like genes are identified by sequence homology (Meyers et al., 2003) and 51 of them are experimentally validated by a measurable function in disease resistance, defense responses, or autoimmunity (Kourelis and Kamoun, 2020). Some NLRs work as functional singletons that contain both pathogen detection (sensor) and immune signaling (helper) functions, whereas others work either as a sensor or helper in pairs to initiate responses through a complex signaling network (Adachi et al., 2019). The helper NLRs are important signaling hubs for a variety of sensor NLRs (Jubic et al., 2019) and they are encoded by two gene families, ACTIVATED DISEASE RESISTANCE 1 (ADR1) and N REQUIRED GENE 1 (NRG1) in A. thaliana (Bonardi et al., 2011; Castel et al., 2019; Lapin et al., 2019; Wu et al., 2019; Saile et al., 2020). It has long been established that NLR protein activity is tightly controlled. Recognition of effectors directly or indirectly causes conformation changes in NLR protein from ADP binding to ATP binding and triggers downstream defense responses (Qi and Innes, 2013; Burdett et al., 2019). Recent reports of the structures of a CNL protein ZAR1 (Wan et al., 2019) and two TNL proteins Roq1 (Martin et al., 2020) and RPP1 (Ma et al., 2020) reveal that recognition of effectors by NLRs triggers oligomerization-dependent NLR activation and downstream immune responses. Whether or not NLR genes are induced during plant pathogen interaction has not been extensively investigated.
Most NLR genes are thought to be expressed at low levels under non-pathogenic conditions (Tan et al., 2007) because constitutive activation of NLR genes often leads to plant dwarfism and sometimes lethality (Gou and Hua, 2012; van Wersch et al., 2016; Wan et al., 2020). However, NLR genes need to be expressed at proper levels to initiate plant immune responses upon pathogen attack (Mohr et al., 2010). Fine control of NLR transcript level under changing environment is therefore potentially critical for balancing defense and growth.
Environmental factors other than pathogens also have a large influence on plant immunity (Hua, 2013; Cheng et al., 2019; Saijo and Loo, 2020). High temperature (Yang and Hua, 2004; Wang et al., 2009; Kim et al., 2010) and high humidity (Jambunathan et al., 2001; Panchal et al., 2016) are often associated with decreased disease resistance while low temperature (Huang et al., 2010; Yang et al., 2010; Kim et al., 2017) and high light (Mühlenbock et al., 2008) are often associated with high disease resistance. The intersection of abiotic factors with immunity could happen at multiple points, and NLR proteins likely occupy key intersection points. High temperature inhibits nuclear accumulation of NLR proteins SNC1 and RPP4, leading to the repression of their induced immune responses at elevated temperature (Zhu et al., 2010; Mang et al., 2012). However, whether or not abiotic stresses impact plant immunity through the regulation of NLR transcription is not thoroughly investigated.
Upregulation of NLR gene expression, in addition to their protein activity activation, has been linked to autoimmunity where immune responses are activated under normal non-pathogenic conditions often leading to spontaneous cell death and dwarfism (van Wersch et al., 2016; Wu et al., 2020). For instance, the immune response in the hos15-4 mutant defective in histone deacetylation is hyper-activated, which is partially dependent on a NLR gene SUPPRESSOR OF npr1, CONSTITUTIVE 1 (SNC1) (Yang et al., 2020). Additionally, about one third of total NLR genes are upregulated in the hos15-4 mutant (Yang et al., 2020), suggesting a contribution of NLR activation to autoimmunity. Autoimmune mutants often have increased SA accumulation and spontaneous cell death, which are also highly related to NLR function. For instance, the acd6-1 mutant is a gain-of-function mutant with increased disease resistance to Pseudomonas syringae, and the amount of SA in this mutant is positively correlated with the degree of disease resistance and defense gene expression (Lu et al., 2009). Similarly, the autoimmune mutant ssi2-1 defective in a stearoyl-ACP desaturase accumulates a high level of SA under normal growth conditions (Shah et al., 2001). The bak1-4 serk4-1 double mutant defective in two receptor kinases coding BAK1 and SERK4 exhibited spontaneous cell death and other autoimmune phenotypes (de Oliveira et al., 2016). However, comprehensive analysis of NLR gene expression in these autoimmune mutants is still lacking.
In this meta-analysis, we systematically analyzed the transcript dynamics of all 207 NLR genes in the Col-0 accession of A. thaliana under various biotic and abiotic stresses as well as in autoimmune mutants based on 88 RNA sequencing (RNAseq) datasets from 27 independent studies. We found that about 146 NLR genes were generally induced by pathogens and defense elicitors but repressed by abscisic acid, heat, and drought. The expression pattern of NLR genes in autoimmune mutants was very similar to that in response to pathogen infection. Among the 131 NLR genes induced by pathogens, 87 NLR genes are induced and 44 NLR genes are not induced by SA or its analog BTH. Additionally, 26 NLR genes were repressed under biotic stress conditions. Therefore, this meta-analysis illustrates dynamics of transcript abundance of NLR genes under different conditions, which provides a foundation for further understanding of NLR gene regulation in plant immunity as well as interactions between abiotic and biotic stress responses.
Methods
Selection of RNAseq Data for the NLR Expression Study
In this meta-analysis, we utilized publicly available RNAseq data to investigate the transcript dynamics of all 207 NLR genes in the Col-0 accession of A. thaliana in response to biotic and abiotic stresses as well as in autoimmune mutants. These NLR or NLR-like genes were listed in previous studies (Meyers et al., 2003; Yang et al., 2020). We also included in the analysis several key SA-related genes because SA signaling is closely linked with NLR activation (Shirano et al., 2002; Xiao et al., 2003; Yang and Hua, 2004). These SA-related genes include SA biosynthesis genes (ICS1, ICS2, PBS3, EPS1, PAL1, PAL2, PAL3, and PAL4), two master ICS1 transcription factors CBP60g and SARD1, and three EDS1 family genes (EDS1, PAD4, and SAG101) that mediate SA and NLR signaling, as well as EDS5 encoding a transporter for SA precursor. Also included are biosynthesis genes of another defense mediator N-Hydroxypipecolic Acid (NHP), ALD1, SARD4, and FMO1, which are coordinately regulated by the SA regulators SARD1 and CBP60g (Huang et al., 2020).
All the datasets in this meta-analysis were collected from peer-reviewed publications published before August 2020 (Supplementary Data 1). We searched literature via Google Scholar for RNAseq data of plants grown under various conditions using the keywords “transcriptome,” “RNA sequencing,” “biotic stress (pathogen infection; SA; flg22; chitin),” “abiotic stress (low temperature; cold; heat; high temperature; salt stress; ABA; drought),” and “autoimmunity.” The datasets used in the meta-analysis were selected based on the following criteria: datasets were performed on leaves or seedlings of Col-0 accessions; differentially expressed genes (DEGs) were listed in the publication or processed reads count for each gene (“reads count-only” datasets) were available in NCBI GEO database. We directly extracted the differentially expressed NLR genes, SA- and NHP-related genes from references if DEGs were listed in the references, and most of these studies used the same criteria (p or FDR < 0.05, FC ≥ 2). Otherwise, “reads count-only” datasets were first downloaded from NCBI GEO under the accession numbers provided in references and then DEGs were extracted by in-house pipeline in R with edgeR package. Genes with p value < 0.05 were defined as DEGs by the treatment. Because increase of the transcripts of NLR genes is often not very large (sometimes less than 0.5-fold change) after pathogen infection (Zou et al., 2014; Yang et al., 2020) and with or without fold change (FC) does not change the relative numbers of upregulated and downregulated DEGs, we used FDR criteria without an additional FC criterion to allow more sensitive capture of early induction of NLR genes.
RNAseq data with biotic and abiotic treatments as well as RNAseq data for autoimmune mutants were selected. For biotic stresses, we selected infection by representative strains of bacterial pathogen P. syringae pv. tomato (Pst) (Pst DC3000, Pst DC3000 AvrRps4, Pst DC3000 AvrRpm1, Pst DC3000 AvrRpt2, and Pst DC3000 cor-), fungal pathogen Botrytis cinerea, immunity elicitors flg22 and chitin, as well as SA and its functional analog BTH. For abiotic stresses, treatments by ABA, temperature, drought, and salt were included. When data were available, we included at least two independent datasets of the same treatment to increase data confidence. Also included are autoimmune mutants including hos15-4, acd6-1, ssi2-1, and bak1-4 serk4-1. In total, 88 RNAseq datasets from 27 independent studies were analyzed, including 57 datasets in response to biotic factors, 26 datasets to abiotic factors, and 5 datasets in autoimmune mutants (Table 1). The growth conditions and treatments used in the 27 independent studies are listed in Supplementary Data 1.
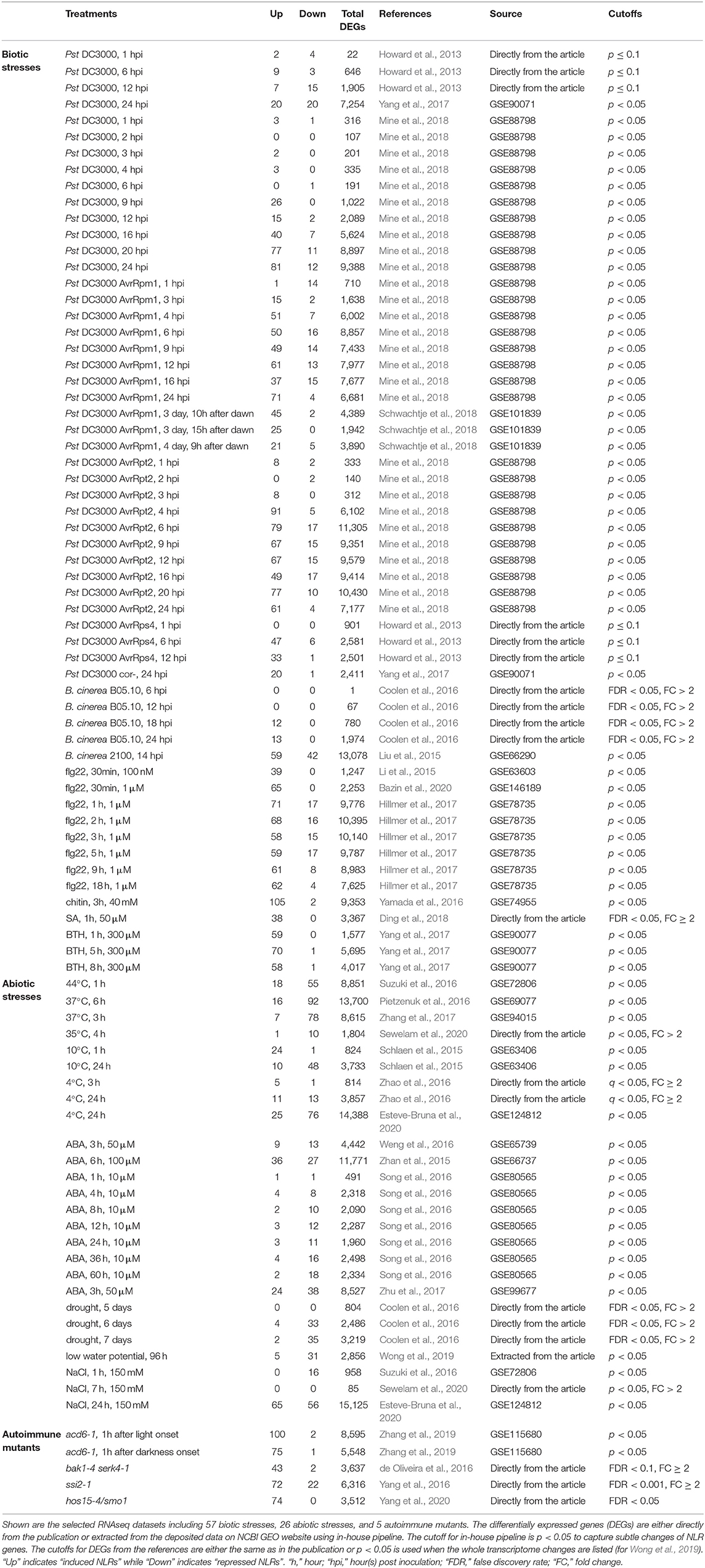
Table 1. The number of induced and repressed NLR genes extracted from the RNAseq datasets used in this study.
Procedures for Extracting DEGs From “Reads Count-Only” Datasets
Reads count of each treatment or mutant was downloaded from NCBI under GSE accessions provided in the references (Table 1). R/EDGER was used to call DEGs. The cpm (counts per million) values of each gene were calculated using “y <- DGEList(counts =x,group=group)”; “y <- calcNormFactors(y),” and genes with cpm value > 1 in at least two samples were used for DEG analysis using “keep <-rowSums(cpm(y)≥1) ≥2”; “y <-y[keep,].” Then, DEGs were called using “design <-model.matrix(~0+group)”; “y <- estimateGLMCommonDisp(y,design)”; “y <- estimateGLMTrendedDisp(y,design)”; “y <- estimateGLMTagwiseDisp(y,design)”; “fit <-glmFit(y,design)”; “lrt.2vs1 <- glmLRT[fit, contrast=c(-1,1)]”; “top2v1 <- topTags(lrt.2vs1, n=30000)”. For more details, please see https://biohpc.cornell.edu/workshops.aspx.
Hierarchical Cluster Analysis of NLR Genes and SA- and NHP-Related Genes
The hierarchical cluster analysis was performed using “hclust” function in R program (https://www.r-project.org/). Because some NLR genes have been shown to have feedback regulation with SA, which is tightly connected with NHP, the SA- and NHP-related genes were also included in this analysis. To increase robustness of the analysis, we excluded 15 RNAseq datasets with fewer than 10 differentially expressed NLR genes. To reduce redundancy, one time point with the most drastic changes of NLR genes was selected for each of the time-course treatment. In total, 37 different samples were used for cluster analysis, with 16 biotic stress treatment, 16 abiotic stress treatment, and 5 autoimmune mutants. Briefly, the values of each gene with upregulation, downregulation, and no change were considered as 1, −1, and 0, respectively. The dissimilarity values were calculated with the “dist” function and then used as input for “hclust.” The Ward method was used for hierarchical clustering, because it identified the strongest clustering structure among the four methods (Average, 0.5804108; Single, 0.4357676; Complete, 0.7109366; Ward, 0.897766) analyzed. The clusters were identified with the “cutree” function, and the number of optimal clusters was determined with the Elbow method. Heatmap was generated in Excel. For visualization, NLR genes induced by the treatments or upregulated in the mutants were colored red while genes repressed were colored blue. Genes not expressed or the transcript level not altered were left blank. Among the 207 total NLR genes, 29 NLR genes had no altered expression or were not expressed in response to any of the 37 conditions selected in this analysis (Table 2). None of these genes were experimentally validated and 13 of them did not contain NBS and LRR domains (Table 2). Additionally, 11 of them are no longer considered as valid genes on TAIR (Table 2). Therefore, we excluded these genes from the cluster analysis for clarity.
Results
NLR Genes Are in General Induced Under Biotic Stresses
We tallied NLR genes that have increased or reduced expression respectively for each treatment (Table 1). In 47 out of 57 biotic stress treatments, more NLR genes had increased expression than reduced expression after treatment (Table 1). For instance, 81 NLR genes were induced while 12 were repressed at 24 h post infiltrating with Pst DC3000 (Table 1). Also, chitin treatment at 40 mM for 3 h induced 105 NLRs while repressed only 2 NLRs (Table 1). The 10 treatments that did not show more NLR genes induced than repressed were either not reproduced in independent studies or were for the early time point after infection and became to have more induced NLR genes in later time points. Therefore, all biotic treatments analyzed, Pst DC3000 strains (virulent, avirulent, or non-virulent), B. cinerea, flg22, chitin, and SA, led to more NLR genes having increased gene expression than reduced gene expression.
We further viewed the transcript dynamics in response to different treatments by plotting the number of increased and reduced NLR genes over time in the same treatment with a study. In general, the number of NLR genes with altered expression increased as the treatment progressed, and this was especially pronounced for the number of NLR genes with increased expression (Figure 1). The study of Mine et al. (2018) contained a set of treatment by various Pst DC3000 strains, which allowed us to compare over time and across different strains for DEGs selected by p < 0.05. After Pst DC3000 infection, fewer than 5 NLR genes had altered expression before 6 hpi (hours post-inoculation) while 40, 77, and 81 NLR genes were induced at 16, 20, and 24 hpi, respectively (Table 1; Figure 1A). By contrast, no more than 13 NLR genes were repressed during all the time points (Table 1; Figure 1A). A similar increase of number of induced NLRs over time was also observed under the infection by avirulent bacterial pathogens Pst DC3000 AvrRpm1 and Pst DC3000 AvrRpt2. Very few NLRs had altered gene expression before 3 hpi, but more NLR genes had increased expression after 4 hpi (Table 1; Figures 1B,C). A maximum of 71 and 91 NLR genes were induced by Pst DC3000 AvrRpm1 and Pst DC3000 AvrRpt2, respectively (Table 1; Figures 1B,C). The number of induced NLR genes went up much faster in response to avirulent Pst DC3000 strains compared to the virulent Pst DC3000. No more than 26 NLR genes were induced in response to Pst DC3000 before 16 hpi whereas 51 and 91 NLR genes were induced at 4 hpi by Pst DC3000 AvrRpm1 and Pst DC3000 AvrRpt2, respectively (Table 1; Figures 1A–C). A similar pattern was also observed in the study of Howard et al. (2013). With DEG selected by p ≤ 0.1, no more than 10 NLR genes were induced in response to Pst DC3000 at 1, 6, and 12 hpi while Pst DC3000 AvrRps4 induced 47 and 33 NLR genes at 6 and 12 hpi, respectively (Table 1; Figures 1D,E). This suggested a faster defense response in incompatible interaction than compatible interaction, as observed earlier in overall transcriptome responses (Tao et al., 2003).
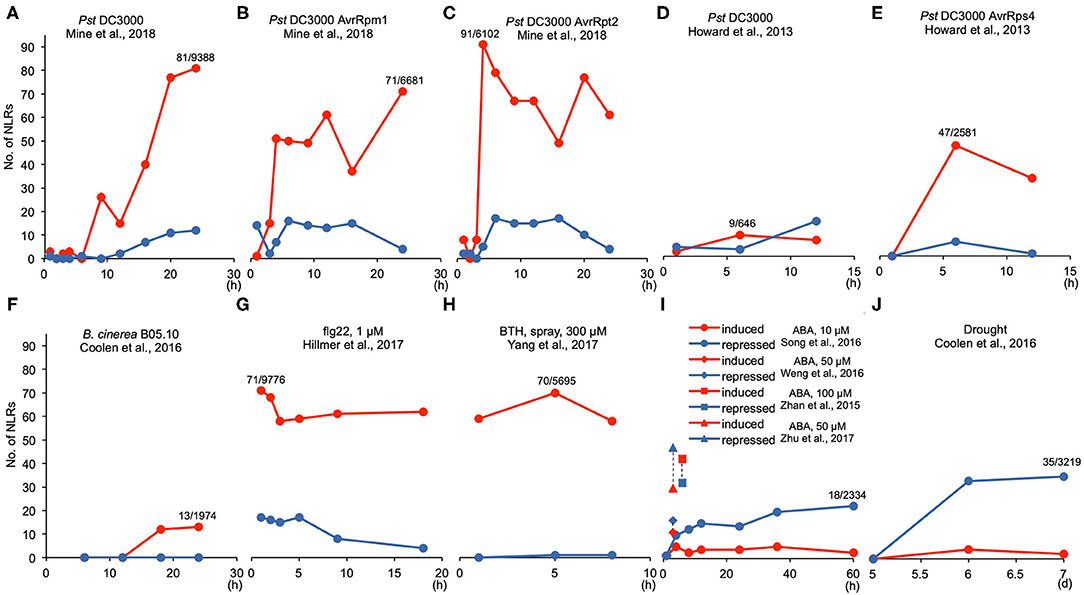
Figure 1. Transcript dynamics of NLR genes during biotic and abiotic stresses. (A–J) Line graphs showing the number of induced (red color) and repressed (blue color) NLR genes at each time point for different treatments. The numbers are made based on Table 1. For biotic stress treatments, the maximum number of induced NLR genes and the total DEG number are listed as induced NLR number/total DEG number above the corresponding time point. Similarly for abiotic stress treatments, the maximum number of repressed NLR genes and the corresponding total DEGs are listed. “h” indicates “hours” and “d” means “days”.
The infection by fungal pathogens also induced the transcript level of some NLR genes. In response to fungal pathogen B. cinerea B05.10 strain, 12 and 13 NLR genes were upregulated at 18 and 24 hpi, respectively, and no NLR genes were repressed in the study of Coolen et al. (2016) (Table 1; Figure 1F). In another dataset of infection by the B. cinerea 2100 strain, with p < 0.05, 59 NLR genes were upregulated and 42 were downregulated at 14 hpi (Table 1). The latter dataset had an unusual high number of DEGs (comprising half of the genome), which might contribute to the high number of NLR genes differentially expressed in this dataset (Table 1). More datasets will be needed for determining the general NLR expression patterns during fungal pathogen infection.
The treatment of flg22, chitin, SA, and BTH all led to more NLR genes with increased expression than with repressed gene expression (Table 1). Using the same DEG selection criteria, the induction apparently occurred earlier than pathogen infection even by avirulent Pst DC3000 strains (Table 1; Figures 1G,H). In Hillmer et al. (2017), 1 μM flg22 induced a maximum of 71 NLR genes at 1 h post treatment (Figure 1G) and 65 NLR genes were induced at 30 min post treatment of 1 μM flg22 in another study (Bazin et al., 2020) with the same DEG selection criteria p < 0.05 (Table 1). Likewise, 38 NLR genes were induced at 1 h post treatment of 50 μM SA (Table 1). Additionally, no NLR genes were repressed by SA treatment, and only one or two NLR genes were repressed by BTH or chitin (Table 1). The PAMP (Pathogen-Associated Molecular Pattern) signal flg22 at 100 nM and 1 μM treatment did not repress NLR gene expression at early time point (30 min), but repressed at maximum 17 NLR genes at 1 μM, while it induced expression of 39–71 NLRs under all conditions (Table 1). After the early induction with these molecules, the number of NLR gene with increased expression sustained throughout the duration of treatment (Figures 1G,H).
NLR Genes Are in General Repressed in Response to Heat, ABA, and Drought
Abiotic stresses including high temperature and ABA are found to impact plant immunity through affecting NLR protein localization (Zhu et al., 2010; Mang et al., 2012). In addition, a previous study showed that the variation in NLR gene expression may be under natural section to better adapt to the environment (MacQueen and Bergelson, 2016). Here, we analyzed NLR transcript change in response to abiotic stresses including heat, ABA, and drought and found that these abiotic stresses in general repressed the transcript level of NLR genes (Table 1).
Under three heat shock treatments, 44°C for 1 h, 37°C for 3 or 6 h, and 35°C for 4 h, much more NLR genes were repressed than induced (Table 1). Three-hour 37°C treatment repressed 78 NLR genes and induced 7 NLR genes among a total of 8,615 DEGs (Table 1). Similarly, 1-h 44°C treatment repressed 55 NLR genes and induced 18 NLR genes among 8,851 DEGs (Table 1). Therefore, more NLR genes had decreased transcripts than increased expression, which might contribute to high-temperature inhibition of disease resistance. By contrast, low temperature had a more complex effect on NLR transcript level depending on the duration of cold treatment. At the early period of low-temperature treatment (4°C for 3 h or 10°C for 1 h), no more than 25 NLR genes had altered expression with more genes induced than repressed by low temperature as observed in two independent studies by Zhao et al. (2016) and Schlaen et al. (2015) (Table 1). However, under 24 h of 4 and 10°C treatment, 13 and 48 NLR genes were repressed, along with 11 and 10 NLR genes induced, respectively (Table 1). This was supported by another study (Esteve-Bruna et al., 2020) in which 76 NLR genes were repressed while only 25 NLR genes were induced after 24 h treatment of 4°C (Table 1).
Similar to heat, ABA and drought generally repressed the expression of NLR genes (Table 1; Figures 1I,J). Under 10 μM ABA treatment in Song et al. (2016), more NLR genes were repressed than induced and the number of repressed NLR genes increased as treatment time increased (Table 1; Figure 1I). Similarly, higher concentrations of ABA generally have more NLR genes repressed than induced (Table 1; Figure 1I). Drought treatment, including low water potential treatment, also drastically repressed the transcript levels of NLR genes (Table 1). A total of 33 and 35 NLR genes were repressed under drought treatment of 6 and 7 days, respectively, while fewer than 5 NLRs were induced (Table 1; Figure 1J). Likewise, 31 NLR genes were repressed while only 5 NLR genes were induced among 2,856 DEGs under low water potential treatment for 96 h (Table 1).
NaCl treatment did not appear to cause specific transcript changes of NLR genes (Table 1). NaCl treatment at 150 mM for 1 h (Suzuki et al., 2016) led to 16 NLR genes repressed and no NLR induced with p < 0.05. However, NaCl treatment at 150 mM for 7 h from another study (Sewelam et al., 2020) did not alter any NLR gene expression with p < 0.05 and FC > 2. A longer treatment time of 24 h (Esteve-Bruna et al., 2020) altered expression of a large number of NLR genes, with 65 upregulated and 56 downregulated. It is worth noting that three quarters of genes in the genome had altered expression in this study (Table 1), and therefore whether or not NaCl has a specific effect on NLR gene expression at this stage of salt stress still needs investigation.
NLR Genes Are Induced in Autoimmune Mutants
Because activation of NLR genes has been linked to autoimmunity, we examined all NLR genes to determine which and how many were differentially expressed in autoimmune mutants. The results showed that the transcripts of 43–100 NLR genes were increased in all the mutants with fewer than three genes repressed for all but the ssi2-1 mutant (Table 1). The acd6-1 mutant had 100 and 75 induced NLR genes at 1 h after light or darkness onset, respectively, and only 1–2 NLR genes were repressed under these conditions with p < 0.05 (Table 1). Likewise, the bak1-4 serk4-1 double mutant had 43-induced NLR genes while only 2 repressed NLR genes with FDR < 0.1 and FC ≥ 2 (Table 1). The hos15-4 mutant had 74-induced NLR genes, and no NLR genes were repressed in this mutant with FDR < 0.05 (Table 1). Although 22 NLR genes were repressed, the ssi2-1 mutant had 72-induced NLR genes among 6,316 DEGs under relatively stringent DEG selection criteria of FDR < 0.001 and FC ≥ 2 (Table 1). These analyses suggest that activation of NLR genes is a shared phenomenon for autoimmune mutants.
Expression of Majority of NLR Genes Are Induced by Biotic Stresses and Repressed by Abiotic Stresses
To reveal potential gene network among all NLR genes, as well as SA- and NHP-related genes, we did a hierarchical cluster analysis of these genes based on their induction or repression under selected stress conditions and in autoimmune mutants (see Methods for details). Expression pattern was displayed for clustered NLR genes with grouped abiotic treatment, biotic treatment, and autoimmune mutants. Overall pattern from the cluster analysis revealed that abiotic and biotic stresses in general had opposite effects on expression of half of the NLR gene expression (Figure 2). Based on the expression pattern, NLR genes could be grouped into four modules A–D (Figure 2). Modules C and D comprised about half of the NLR genes and they were more similar to each other than to modules A and B. NLR genes in modules C and D were generally induced by biotic stresses and repressed by abiotic stresses, but they slightly differed in the extent of expressional changes.
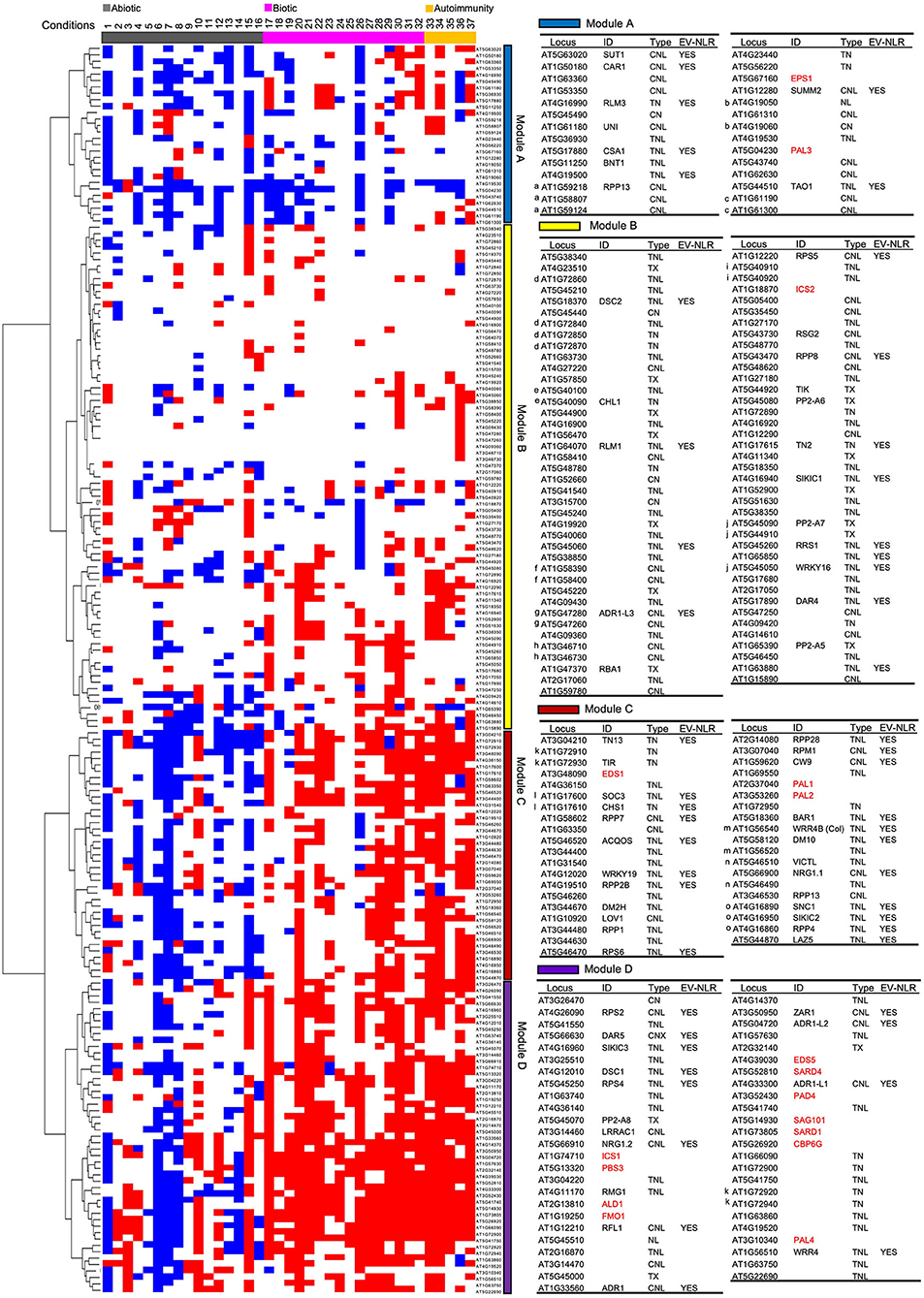
Figure 2. Expression profile of 178 NLR genes as well as 17 SA- and NHP-related genes under stress conditions and in autoimmune mutants. Cluster display of 178 NLR or NLR-like genes along with 17 SA- and NHP-related genes by their transcript levels in 37 conditions. Gene induction is marked as a red box while gene repression is marked as a blue box. Expression not altered are left as blank. Modules A, B, C, and D are discussed in the text. The detailed information of the genes from each module is shown in the right tables, including gene ID number on TAIR website, common name, NLR type, and whether genes are experimentally validated or not. In the tables, 17 SA- and NHP-related genes are colored red. “EV-NLR” means “experimentally validated NLRs” defined by Kourelis and Kamoun (2020). The letter on the left of a gene indicates that this gene is co-regulated with at least another gene in the respective gene cluster and the same letter before the gene ID indicates genes are in the same gene cluster on the chromosome. The 37 conditions are numbered and they are (1) 4°C, 24 h; (2) 4°C, 24 h; (3) 10°C, 1 h; (4) 10°C, 24 h; (5) 35°C, 4 h; (6) 37°C, 3 h; (7) 37°C, 6 h; (8) 44°C, 1 h; (9) ABA, 3 h, 50 μM; (10) ABA, 6 h, 100 μM; (11) ABA, 60 h, 10 μM; (12) ABA, 3 h, 50 μM; (13) drought, 7 days; (14) NaCl, 1 h, 150 mM; (15) NaCl, 24 h, 150 mM; (16) low water potential, −0.7 Mpa, 96 h; (17) Pst DC3000, 24 hpi, OD600 of 0.001; (18) Pst DC3000, 12 hpi, 107 cfu/ml; (19) Pst DC3000, 24 hpi, OD600 of 0.2; (20) Pst DC3000 AvrRpt2, 4 hpi, infiltration, OD600 of 0.001; (21) Pst DC3000 AvrRps4, 6 hpi, 107 cfu/ml; (22) Pst DC3000 AvrRpm1, 24 hpi, OD600 of 0.001; (23) Pst DC3000 AvrRpm1, 3 day, 10 h after dawn, OD600 of 0.02; (24) Pst DC3000 cor-, 24 hpi, OD600 of 0.2; (25) B. cinerea B05.10, 24 hpi, 5 μl 1 ×105 spores/ml; (26) B. cinerea 2100, 14 hpi, 2 μl 2.5 ×105 spores/ml; (27) flg22, 30 min, 100 nM; (28) flg22, 30 min, 1 μM; (29) flg22, 1 h, 1 μM; (30) chitin, 3 h, 40 mM; (31) SA, 1 h, 50 μM; (32) BTH, 5 h, spray, 300 μM; (33) acd6-1, ZT13, 1 h after darkness onset; (34) acd6-1, ZT1, 1 h after light onset; (35) bak1-4 serk4-1; (36) ssi2-1; (37) hos15-4/smo1. References and detailed information for these conditions (1–37) are in Supplementary Data 1. Abiotic stresses are colored gray, biotic stresses are colored magenta, and autoimmune mutants are colored orange. “h” indicates “hour” and “hpi” means “hour(s) post inoculation”.
Modules C and D contained 74 NLR genes, which were induced by most of the biotic stresses and repressed by most of the abiotic stresses (Figure 2). NLR genes in module D had more induction under biotic stresses while less repression under abiotic stresses as compared to NLR genes in module C. Fifty-five of them contained a TIR domain, suggesting that TNLs were more likely to respond to biotic and abiotic stresses than CNLs. Additionally, 31 out of the 51 experimentally validated NLR genes were in these two modules. For instance, RPM1 in module C was induced by both Pst DC3000 and Pst DC3000 AvrRpm1 at 24 hpi while it was repressed at 3 h or 6 h at 37°C. Notably, five major helper NLR genes ADR1, ADR1-L1, ADR1-L2, NRG1.1, and NRG1.2 were in these two modules (Figure 2). The drastic distinct expression changes of NLR genes under biotic and abiotic stresses suggested that transcriptional regulation of NLR genes might be an important mechanism for plants to cope with different environmental stresses.
Module B had 78 NLR genes including 13 experimentally validated NLRs, and 24 of them did not contain the LRR domain (Figure 2). NLR genes in this module were generally induced under a small number of biotic stress treatments and repressed under a small number of abiotic conditions, although some of them were induced under some abiotic conditions and repressed under certain biotic stresses (Figure 2). These NLR genes might have specificity in response to biotic and abiotic stresses. Alternatively, the expression level was too small to be detected in the RNAseq. Consequently, module B had a more heterogeneous pattern than modules C and D, which exhibited drastic expression changes of NLR genes under stress conditions.
A Small Number of NLR Genes Are Repressed by Biotic Stresses
Module A contained 26 NLR genes that were generally repressed under both biotic and abiotic stresses and in autoimmune mutants, with abiotic stresses more often repressing their expression than biotic stresses (Figure 2). Sixteen of them contained the CC domain, and 7 were experimentally validated NLR genes including SUMM2 (SUPPRESSOR OF MKK1 MKK2 2) and TAO1 (TARGET OF AVRB OPERATION 1). For instance, the transcript level of a CNL SUMM2 was decreased by 150 mM NaCl treatment at 24 h and 50 μM ABA treatment at 3 h. At the same time, it was repressed by biotic stresses including B. cinerea, Pst DC3000, and Pst DC3000 AvrRpt2. This might suggest a unique mode of action of CNLs under certain pathogenic conditions and further studies are needed to explore the biological relevance of this group of NLR genes in response to biotic and abiotic stresses.
A Large Group of NLR Genes Have Similar Expression Pattern With SA- and NHP-Related Genes
All the 17 SA- and NHP-related genes except for ICS2, EPS1, and PAL3 were in module C or D, and 11 of them were in module D, which had stronger induction of NLR genes under biotic stresses (Figure 2). Specifically, PAD4, SAG101, CBP60g, and SARD1 were in the same small subclade with ADR1-L1, AT5G41740, AT5G41750, AT1G66090, and AT1G72900. EDS5 and SARD4 were in the same subclade with ADR1, ADR1-L2, ZAR1, AT4G14370, AT1G57630, and AT2G32140. The PAD4 subclade and the EDS5 subclade were close to each other in the cluster. ICS1 and PBS3 were in another subclade, and so were ALD1 and FMO1. The SA biosynthesis genes PAL1 and PAL2 were in a subclade of module C. EDS1, a gene functionally related to PAD4, was not in the PAD4 subclade but was in the same subclade with two experimentally validated NLR genes AT1G17600 (SOC3) and AT1G17610 (CHS1). By contrast, EPS1 and PAL3 were in module A and ICS2 was in module B (Figure 2). In addition, the expression patterns of ADR1s (ADR1, ADR-L1, and ADR-L2) were more similar to SA-related genes as compared to that of NRG1s (NRG1.1, NRG1.2) (Figure 2). This was consistent with previous studies in which the ADR1 gene family regulates immunity through regulation of SA accumulation and subsequent activation of SA-dependent responses while the NRG1 gene family is not involved in SA regulation (Bonardi et al., 2011; Castel et al., 2019). Notably, 31 out of 38 SA-induced and 57 out of 78 BTH-induced NLR genes were also in modules C and D. These results suggest that SA and biotic stresses mostly activated a similar set of NLR genes.
Assessment of the Contribution of SA to NLR Induction Under Biotic Stresses
We further investigated the contribution of SA to NLR induction under biotic stresses. Datasets with time-course biotic stress treatments along with SA and BTH treatments were used for plotting the transcript dynamics of NLR genes. NLR genes were categorized into four major groups. Group I contained 90 NLR genes that are induced by SA or BTH, and 87 NLR genes among them were also induced by at least one of the biotic stresses (Figure 3). Most NLR genes are induced by pathogens at 4 hpi when SA-related genes, including PAD4, EDS1, SARD1, CBP60g, SAG101, EDS5, PBS3, and ICS1, were induced (Figure 3). SA may potentially be involved in the induction of some NLR genes in this group. Group II consisted of 44 NLR genes that were induced by pathogens but not SA or BTH (Figure 3). All 131 NLR genes that were induced by pathogens or pathogen patterns were within Groups I and II, and 44 of them are not induced by SA or BTH, indicating a SA-independent induction by pathogens. Group III had 52 NLR genes including previously identified 29 non-expressed or “non-altered” NLR genes (Figure 3; Table 2). The transcripts of most NLR genes in this group were not altered in response to pathogen infection or SA and BTH treatment, except for two NLRs AT1G72840 and AT1G57850 showing inconsistent transcript changes in response to avirulent pathogens (Figure 3). Group IV contained 21 NLR genes repressed by pathogens, and 2 of them were repressed by BTH (Figure 3). These analyses indicate that biotic stresses had larger effects on NLR gene transcription and response to SA treatment is similar to responses to pathogens.
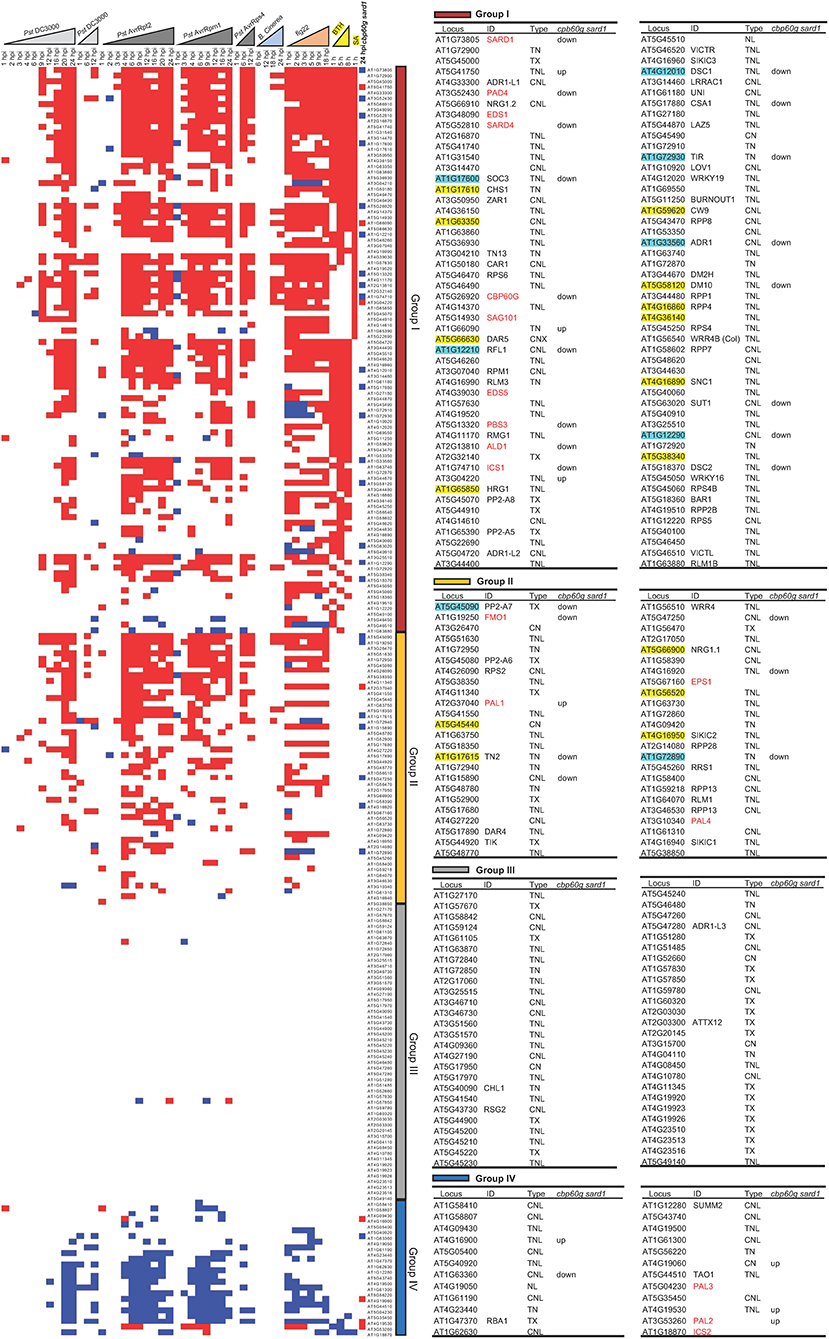
Figure 3. Expression profile of 207 NLR genes as well as 17 SA- and NHP-related genes under time-course biotic stress treatment. Expression levels of 207 NLR or NLR-like genes and 17 SA- and NHP-related genes during the time course of biotic stress treatments that are marked as triangles (light gray for Pst DC3000; dark gray for avirulent pathogens including Pst DC3000 AvrRpt2, Pst DC3000 AvrRpm1, and Pst DC3000 AvrRps4; light blue for B. cinerea; orange for flg22; and yellow for BTH). The genes are ordered by their induction by SA and BTH (first ordered by the induction by SA, then ordered by the induction by BTH), from induced (indicated by red square), to non-alteration (blank) and repressed (blue square) from top to bottom. These genes are grouped into I (dark red), II (orange), III (gray), and IV (dark blue) four groups based on the transcript changes under biotic stresses as discussed in Results. NLR genes that exhibited a reduced expression or an increased expression in the cbp60g sard1 mutant compared to the wild type at 24 hpi after P. syringae pv maculicola ES4326 infection are displayed as blue square or red square, respectively, in the last column of the expression heatmap. The 17 SA- and NHP-related genes are colored red. The 15 NLR genes dependent on SA and the 8 NLR genes of which full induction is dependent on SA are highlighted with yellow and light blue, respectively. The detailed information of the genes from each group is shown in the right tables, including gene ID number on TAIR website, common name, NLR type, and whether genes are upregulated (up) or downregulated (down) in the cbp60g sard1 mutant compared to wild-type plant at 24 hpi after P. syringae pv maculicola ES4326 infection.
In order to determine whether or not SA accumulation is responsible for induction of some NLRs during pathogen infection, we analyzed RNAseq data of the cbp60g sard1 double mutant where SA induction by pathogen was greatly compromised (Lu et al., 2018). As expected, the six genes related to biosynthesis of SA and NHP, PAD4, PBS3, ICS1, SARD4, ALD1, and FMO1 have reduced expression in the double mutant compared to the wild type after infection by P. syringae pv maculicola ES4326 (Pma) (Figure 3). With p < 0.05, a total of 61 NLRs were induced by Pma in the wild-type plants but only 38 NLRs were induced in the cbp60g sard1 double mutant (Figure 4A). Among the 61 NLR genes induced by Pma in wild type, 31 NLR genes were also induced in the cbp60g sard1 double mutant while 30 NLR genes were induced only in wild type, making them candidate NLRs whose induction by pathogen is dependent on SA induction (Figure 4B). Surprisingly, 15 out of these 30 NLR genes had an increased expression in the cbp60g sard1 double mutant compared to the wild type under mock condition (Figure 4B). While it is yet to be determined why these NLRs, most of which can be induced by SA, had increased expression in the mutant deficient in SA induction, the higher expression under normal condition might contribute to their non-induction by Pma infection. The other 15 NLR genes were induced by Pma infection only in the wild type and did not have a higher expression in the mutant compared to the wild type under mock condition (Figures 3, 4B). The induction of these 15 NLR genes by pathogen in the wild type is dependent on CBP60g/SARD1 and their mediated SA induction. In addition, 17 NLRs had reduced expression in the mutant compared to the wild type after Pma infection, and 10 of them were induced by Pma in the wild type (Figures 3, 4A). Therefore, the induction of these 10 NLRs in the wild type was compromised in the cbp60g sard1 mutant. Two of these 10 NLRs were among the 15 NLR genes induced by Pma only in the wild type, indicating that 8 others had reduced induction by pathogen in the mutant compared to the wild type (Figure 3). These data indicate that the induction or full induction of a total of 23 (15 + 8) NLRs are dependent on CBP60g and SARD1, suggesting that their pathogen-induced expression is likely triggered by an increased SA production after pathogen infection.
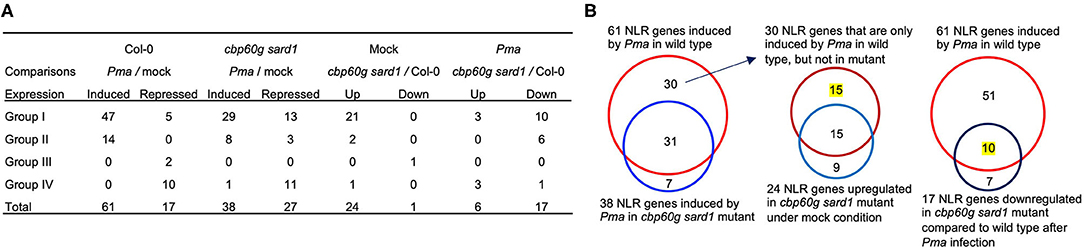
Figure 4. Number of NLR genes with altered expression in the cbp60g sard1 double mutant. (A) Number of NLR DEGs in each group in the cbp60g sard1 mutant and the wild type in response to P. syringae pv maculicola ES4326. DEGs were extracted from GSE10087 (Lu et al., 2018) by in-house pipeline with p < 0.05. “Up” and “Down” indicate increased or reduced expression in the mutant compared to the wild type, respectively. (B) Venn diagrams showing SA-dependent NLR gene induction by pathogen. The left panel is NLR genes induced by Pma in wild type and NLR genes in the cbp60g sard1 double mutant. The middle panel is the NLR genes induced by Pma only in wild type and NLR genes upregulated in cbp60g sard1 double mutant compared to the wild type under mock induction. The right panel shows the NLR genes induced by Pma in wild type and NLR genes downregulated in cbp60g sard1 double mutant compared to wild type after Pma infection. The number of NLR genes whose pathogen induction or whose full induction are dependent on CBP60G/SARD1 is highlighted with yellow. Pma, P. syringae pv maculicola ES4326.
Genes in the Same Gene Cluster More Likely Have Similar Expression Patterns
We analyzed the expression patterns of NLR genes residing in the same gene cluster to determine if they were co-regulated. The Col-0 accession of A. thaliana has a total of 42 NLR gene clusters with 2–11 of NLR genes in one cluster (Meyers et al., 2003). Co-expression was defined as genes residing in the same or adjacent subclade in the Cluster analysis. Fifteen NLR gene clusters had at least two genes in the cluster showing co-expression (Figure 2). For example, SOC3 (AT1G17600) and CHS1 (AT1G17610) are two well-known NLR genes with TIR domain in the same gene cluster, and they were next to each other in the expression cluster subclade, indicating a similar expression pattern (Figure 2). Likewise, SNC1, RECOGNITION OF PERONOSPORA PARASITICA 4 (RPP4), and SIDEKICK SNC1 2 (SIKIC2) resided in the RPP5 gene cluster, which contains eight NLR genes in the Col-0 background (Meyers et al., 2003), and they were clustered together in the expression cluster subclade (Figure 2). However, not all the genes in the same gene cluster shared a similar expression pattern. For instance, in the RPP5 gene cluster, SNC1, RPP4, and SIKIC2 were clustered together, while five other NLR genes in the RPP5 gene cluster were in other modules (Figure 2). Co-regulation of NLR genes in the RPP5 gene cluster has been implicated in previous studies (Yi and Richards, 2007; Zou et al., 2014, 2017). These results indicate that NLR genes in the same cluster are more likely to have a similar expression pattern, which might enable plants to initiate a timely and effective immune response.
Limitations of This Meta-Analysis
Because this meta-analysis was from different datasets of separate studies, this raises an issue when comparing gene list across different studies. Biological differences and methodological differences could potentially make some cross comparisons impossible. These differences may come from (a) plant growth conditions (light quality and quantity; light cycle; humidity; growth medium); (b) developmental stage of plants; (c) tissues (leaf or whole plants) sampled for RNAseq analysis; (d) RNAseq method (library preparation; sequencing method and depth); and (e) RNAseq data analysis (methods and cutoffs for extracting DEGs). These factors can affect the DEG list extracted for analysis. The following measures have been used to minimize these differences: (1) Datasets from similar biological conditions were selected for this study. For instance, only leaves or young seedlings were selected (Supplementary Data 1); (2) When possible, datasets with processed reads count were selected so that the same in-house pipeline can be used to extract DEGs with the same selection criteria. In fact, DEGs from 66 out of the 88 RNAseq datasets were selected using the same criterion of p < 0.05. In addition, the selection criteria for the rest of the datasets (directly from the article) were mostly using the same selection criteria (p value, q value, or FDR < 0.05 with an additional FC ≥ 2). Characteristics that are not dependent on selection criteria or sample quality (such as more genes up than down) are used for cross-comparison between different studies. Characteristics that are dependent on selection methods are only compared within the same experimental set. Although we made efforts to minimize both biological and methodological differences, these factors need to be considered when it comes to cross-comparison of the number of NLR genes induced or repressed under stress conditions among different studies.
Discussion
Plant NLR proteins are central intracellular immune receptors critical for pathogen recognition and immune response activation. They are known to be tightly regulated at the protein level for immunity/growth balance. However, their dynamics at the RNA transcript level was not extensively investigated before. This meta-analysis mined 88 RNAseq data and systematically revealed transcript dynamics of NLR genes during plant pathogen interaction and under abiotic stresses, which provides an extensive description of NLR expression under the changing environment.
Transcriptional Induction of NLR Genes Is Prevalent in Plant Immune Response
The first striking feature of the meta-analysis is that more than half of NLR genes are induced by pathogens or defense elicitors and much fewer NLR genes were repressed than induced by biotic stresses (Table 1; Figures 1, 2). Additionally, more NLR genes were induced at later stage after pathogen infection, and avirulent pathogens and defense elicitors triggered NLR gene activation much faster compared to virulent pathogens (Table 1; Figure 1). Also, the number of NLR genes induced or repressed fluctuated throughout the duration of pathogen or defense elicitor treatments (Figure 1), indicating that the transcription of NLR genes is very dynamic in immune responses. Indeed, NLR genes need to be induced upon pathogen infection to confer disease resistance while the transcription of NLR genes also needs to be tightly monitored to prevent overactivation. For instance, most NLR genes upregulated in the hos15-4 mutant are induced by pathogens while these NLRs are simultaneously repressed by HOS15 under pathogenic condition (Yang et al., 2020). These results suggest that regulation of NLR gene expression, in addition to the activation of NLR proteins, might be an important mechanism for plant to better fend off pathogen invasion.
Transcriptional Repression of NLR Genes Might Be a Mechanism for Plant Adaption to Abiotic Stresses
The second feature is that the same set of NLR genes that are induced under biotic stress conditions are repressed by heat, ABA, and drought (Figure 2). These abiotic factors have been shown to inhibit disease resistance in general. For instance, the ABA biosynthetic loss-of-function mutant aba3-1 is more resistant to Pst DC3000 and the ABA biosynthetic gain-of-function mutant cds2-1D exhibits susceptibility to various P. syringae strains as compared to wild type (Fan et al., 2009). The repression of plant defense response by ABA at least partially comes from its cross talk with SA (Mohr and Cahill, 2007; Yasuda et al., 2008; Fan et al., 2009). ABA treatment reduces SA concentration in plants and represses many genes involved in phenylpropanoid biosynthesis pathway, which is closely associated with SA biosynthesis (Mohr and Cahill, 2007). On the other hand, SA antagonizes ABA signaling through multiple mechanisms including inhibiting ABA-induced gene expression and acting against the role of ABA on protein degradation or stabilization (Manohar et al., 2017). Therefore, the opposite effects on NLR gene transcription by ABA and biotic stresses, which often induce SA accumulation, might be due to the antagonism between ABA and SA. In addition, plant drought response is largely through the regulation of ABA signaling pathways, which likely results in similar effects on NLR transcription by ABA and drought. Likewise, high temperature stimulates ABA biosynthesis (Toh et al., 2008), and it is possible that an ABA increase by heat stress contributes to the decrease of NLR transcript level at high temperature. Therefore, the induction of NLRs by biotic stresses and repression by abiotic stresses could result from antagonistic effects between SA and ABA. This study reveals that regulation of NLR gene expression might be an important node for balancing biotic and abiotic stress responses. It is not uncommon for a NLR gene to be functional in one natural accession but non-functional in another accession. Although this may result mainly from co-evolution between plants and pathogens, the balance between biotic and abiotic responses might also play a role. Indeed, functional and non-functional NLR gene ACQUIRED OSMOTOLERANCE (ACQOS) was maintained in Arabidopsis natural accessions due to trade-off between biotic and abiotic stress adaption (Ariga et al., 2017). The ACQOS gene was in module C, and it was generally induced under biotic stresses while repressed under abiotic stresses (Figure 2). Therefore, repression of NLR transcription might have evolved as a general mechanism for plant to survive under abiotic stresses.
A Small Set of NLR Genes Are Repressed During Plant–Microbe Interaction
Interestingly, a small set of NLR genes are repressed by biotic stresses in contrast to the majority of NLR genes (Figure 2). Most of these genes are not functionally characterized (Figure 2). Expression suppression of some NLR genes may occur simultaneously with expression increase of other NLR genes in order to prevent overactivation of immune responses and its consequent fitness costs. For example, an immunity regulator ENHANCED DOWNY MILDEW 2 (EDM2) positively regulates expression of RECOGNITION OF PERONOSPORA PARASITICA 7 (RPP7) and a small number of other NLR genes, whereas it represses the expression of a number of other NLR genes (Lai et al., 2020). Studying the molecular mechanism and biological relevance of NLR genes that are often repressed by pathogens in the context of plant–microbe interaction is expected to bring a new insight into NLR function.
SA Contributes to NLR Induction Under Pathogenic Conditions
Co-expression of SA-related genes with many NLRs under biotic stresses suggests a role of SA in defense signaling transduction and amplification. SA induction was reported for several NLR genes and was postulated to amplify their function (Shirano et al., 2002; Xiao et al., 2003; Yang and Hua, 2004). This study revealed that the majority of NLR genes (in modules C and D) that were induced by pathogens were also induced by SA and co-expressed with SA-related genes (Figure 2). Conversely, SA and BTH induced expression of about 90 NLR genes, and almost all of them were also induced by pathogens (Figure 3). Induction of some of these NLRs by pathogen invasion happened before measurable SA induction, suggesting that SA might amplify their induction during infection. Analysis of SA-deficient mutant cbp60g sard1 revealed that induction of 15 NLRs and full induction of the other 8 NLRs are dependent on CBP60g and SARD1 and therefore likely SA accumulation. Therefore, SA is an inducer and an amplifier of some NLR genes. Additionally, NLR or NLR-like genes with TIR domain were more likely to be induced by SA (Figure 2). This is consistent with the previous finding that SA induced the expression of several TNL genes including SSI4, RPP1, and RPS4, but had little effect on the expression of two CNL genes RPM1 and RPS2 (Shirano et al., 2002). This might be due to a more prominent role of EDS1 and SA in TNL-mediated compared to CNL-mediated immune responses.
This analysis also identified 44 NLR genes whose expression are not significantly induced by SA or BTH. Their induction by pathogens is therefore likely SA independent. The transcriptional regulation of these NLR genes during pathogen infection awaits to be explored in the future. The systematic analyses of all NLR genes under various stress conditions in this study will be a useful resource for better understanding the correlation between SA and NLR induction during pathogen infection process.
Co-regulation of NLR Genes in the Same Gene Cluster Is a Common Phenomenon
This study also revealed that co-expression of NLR genes in the same gene cluster is quite common during plant pathogen interaction. More than one-third of NLR gene clusters have co-expressed genes under stress conditions (Figure 2). This co-expression might be efficient in co-ordinate genes with similar functions as genes in the same cluster tend to have high sequence similarity or are functionally related (such as gene pair). Mechanisms for co-expression have been studied for the RPP5 gene cluster. Chromatin-based gene regulation and RNA silencing have been postulated for achieving co-expression of multiple genes (Yi and Richards, 2007; Zou et al., 2014, 2017). An open or close chromatin structure initiated by transcriptional proteins influencing one NLR gene could cause chromatin structure changes in the gene cluster and thus affect the expression of neighboring genes. Alternatively, a common regulatory element (such as an enhancer) could be shared by NLR genes in the same gene cluster and thus enable co-expression. Co-regulation of NLR genes is expected to facilitate a timely and effective immune response upon pathogen infection.
Conclusion
In sum, NLR genes have dynamic transcript expression patterns in response to both biotic and abiotic stresses. The majority of NLR genes are induced by biotic stresses and are repressed by heat and drought. The opposite effects from biotic and abiotic stresses suggest an important role of NLR gene expression in plant adaptation to environmental stresses. Plant hormones SA and ABA, respectively induce and repress NLR expression in general, suggesting a contribution of these two hormones to NLR gene regulation by biotic and abiotic stresses. Strikingly, a small set of NLR genes are repressed by both biotic and abiotic stresses, and their function will warrant further investigation. This study revealed a broad picture of dynamics of NLR transcript level under environmental stresses, which will facilitate a molecular understanding of immunity regulation under diverse stress conditions.
Data Availability Statement
The original contributions presented in the study are included in the article/Supplementary Materials, further inquiries can be directed to the corresponding author/s.
Author Contributions
JH and LY designed the study. LY and ZW performed the data analyses and made the figures and tables. LY and JH wrote the manuscript with input from ZW. All authors contributed to the article and approved the submitted version.
Funding
This work was supported by National Science Foundation USA IOS-1353738 and IOS-1946174 to JH.
Conflict of Interest
The authors declare that the research was conducted in the absence of any commercial or financial relationships that could be construed as a potential conflict of interest.
Supplementary Material
The Supplementary Material for this article can be found online at: https://www.frontiersin.org/articles/10.3389/fpls.2021.625729/full#supplementary-material
References
Adachi, H., Derevnina, L., and Kamoun, S. (2019). NLR singletons, pairs, and networks: evolution, assembly, and regulation of the intracellular immunoreceptor circuitry of plants. Curr. Opin. Plant Biol. 50, 121–131. doi: 10.1016/j.pbi.2019.04.007
Ariga, H., Katori, T., Tsuchimatsu, T., Hirase, T., Tajima, Y., Parker, J. E., et al. (2017). NLR locus-mediated trade-off between abiotic and biotic stress adaptation in Arabidopsis. Nat. Plants 3:1–8. doi: 10.1038/nplants.2017.72
Bazin, J., Mariappan, K., Jiang, Y., Blein, T., Voelz, R., Crespi, M., et al. (2020). Role of MPK4 in pathogen-associated molecular pattern-triggered alternative splicing in Arabidopsis. PLoS Pathog. 16:e1008401. doi: 10.1371/journal.ppat.1008401
Bonardi, V., Tang, S., Stallmann, A., Roberts, M., Cherkis, K., and Dangl, J. L. (2011). Expanded functions for a family of plant intracellular immune receptors beyond specific recognition of pathogen effectors. Proc. Natl. Acad. Sci. U.S.A. 108:16463–16468. doi: 10.1073/pnas.1113726108
Burdett, H., Bentham, A. R., Williams, S. J., Dodds, P. N., Anderson, P. A., Banfield, M. J., et al. (2019). The plant “Resistosome”: structural insights into immune signaling. Cell Host. Microbe 26, 193–201. doi: 10.1016/j.chom.2019.07.020
Buscaill, P., and Rivas, S. (2014). Transcriptional control of plant defence responses. Curr. Opin. Plant. Biol. 20, 35–46. doi: 10.1016/j.pbi.2014.04.004
Castel, B., Ngou, P. M., Cevik, V., Redkar, A., Kim, D. S., Yang, Y., et al. (2019). Diverse NLR immune receptors activate defence via the RPW 8-NLR NRG 1. New Phytol. 222, 966–980. doi: 10.1111/nph.15659
Chen, H., Xue, L., Chintamanani, S., Germain, H., Lin, H., Cui, H., et al. (2009). ETHYLENE INSENSITIVE3 and ETHYLENE INSENSITIVE3-LIKE1 repress SALICYLIC ACID INDUCTION DEFICIENT2 expression to negatively regulate plant innate immunity in Arabidopsis. Plant Cell. 21, 2527–2540. doi: 10.1105/tpc.108.065193
Cheng, Y. T., Zhang, L., and He, S. Y. (2019). Plant-microbe interactions facing environmental challenge. Cell. Host. Microbe 26, 183–192. doi: 10.1016/j.chom.2019.07.009
Coolen, S., Proietti, S., Hickman, R., Davila Olivas, N. H., Huang, P. P., Van Verk, M. C., et al. (2016). Transcriptome dynamics of Arabidopsis during sequential biotic and abiotic stresses. Plant J. 86, 249–267. doi: 10.1111/tpj.13167
de Oliveira, M. V., Xu, G., Li, B., de Souza Vespoli, L., Meng, X., Chen, X., et al. (2016). Specific control of Arabidopsis BAK1/SERK4-regulated cell death by protein glycosylation. Nat. Plants 2, 1–10. doi: 10.1038/nplants.2015.218
Ding, Y., Sun, T., Ao, K., Peng, Y., Zhang, Y., Li, X., et al. (2018). Opposite roles of salicylic acid receptors NPR1 and NPR3/NPR4 in transcriptional regulation of plant immunity. Cell 173, 1454–1467 doi: 10.1016/j.cell.2018.03.044
Esteve-Bruna, D., Carrasco-López, C., Blanco-Touriñán, N., Iserte, J., Calleja-Cabrera, J., Perea-Resa, C., et al. (2020). Prefoldins contribute to maintaining the levels of the spliceosome LSM2–8 complex through Hsp90 in Arabidopsis. Nucleic Acids Res. 48, 6280–6293. doi: 10.1093/nar/gkaa354
Fan, J., Hill, L., Crooks, C., Doerner, P., and Lamb, C. (2009). Abscisic acid has a key role in modulating diverse plant-pathogen interactions. Plant Physiol. 150, 1750–1761. doi: 10.1104/pp.109.137943
Gou, M., and Hua, J. (2012). Complex regulation of an R gene SNC1 revealed by autoimmune mutants. Plant Signal. Behav. 7, 213–216. doi: 10.4161/psb.18884
Hillmer, R. A., Tsuda, K., Rallapalli, G., Asai, S., Truman, W., Papke, M. D., et al. (2017). The highly buffered Arabidopsis immune signaling network conceals the functions of its components. PLoS Genet. 13:e1006639. doi: 10.1371/journal.pgen.1006639
Howard, B. E., Hu, Q., Babaoglu, A. C., Chandra, M., Borghi, M., Tan, X., et al. (2013). High-throughput RNA sequencing of pseudomonas-infected Arabidopsis reveals hidden transcriptome complexity and novel splice variants. PLoS ONE 8:e74183. doi: 10.1371/journal.pone.0074183
Hua, J. (2013). Modulation of plant immunity by light, circadian rhythm, and temperature. Curr Opin Plant Biol. 16, 406–413. doi: 10.1016/j.pbi.2013.06.017
Huang, W., Wang, Y., Li, X., and Zhang, Y. (2020). Biosynthesis and regulation of salicylic acid and N-hydroxypipecolic acid in plant immunity. Mol. Plant. 13, 31–41. doi: 10.1016/j.molp.2019.12.008
Huang, X., Li, J., Bao, F., Zhang, X., and Yang, S. (2010). A gain-of-function mutation in the Arabidopsis disease resistance gene RPP4 confers sensitivity to low temperature. Plant Physiol. 154, 796–809. doi: 10.1104/pp.110.157610
Jambunathan, N., Siani, J. M., and McNellis, T. W. (2001). A humidity-sensitive Arabidopsis copine mutant exhibits precocious cell death and increased disease resistance. Plant Cell. 13, 2225–2240. doi: 10.1105/tpc.010226
Jubic, L. M., Saile, S., Furzer, O. J., El Kasmi, F., and Dangl, J. L. (2019). Help wanted: helper NLRs and plant immune responses. Curr. Opin. Plant Biol. 50, 82–94. doi: 10.1016/j.pbi.2019.03.013
Kim, S. H., Gao, F., Bhattacharjee, S., Adiasor, J. A., Nam, J. C., and Gassmann, W. (2010). The Arabidopsis resistance-like gene SNC1 is activated by mutations in SRFR1 and contributes to resistance to the bacterial effector AvrRps4. PLoS Pathog. 6:e1001172. doi: 10.1371/journal.ppat.1001172
Kim, Y. S., An, C., Park, S., Gilmour, S. J., Wang, L., Renna, L., et al. (2017). CAMTA-mediated regulation of salicylic acid immunity pathway genes in Arabidopsis exposed to low temperature and pathogen infection. Plant Cell. 29:2465–2477. doi: 10.1105/tpc.16.00865
Kourelis, J., and Kamoun, S. (2020). RefPlantNLR: a comprehensive collection of experimentally validated plant NLRs. bioRxiv [Preprint]. Available online at: https://www.biorxiv.org/content/10.1101/2020.07.08.193961v1 (accessed July 9, 2020).
Lai, Y., Lu, X. M., Daron, J., Pan, S., Wang, J., Wang, W., et al. (2020). The Arabidopsis PHD-finger protein EDM2 has multiple roles in balancing NLR immune receptor gene expression. PLoS Genet. 16:e1008993. doi: 10.1371/journal.pgen.1008993
Lapin, D., Kovacova, V., Sun, X., Dongus, J. A., Bhandari, D., von Born, P., et al. (2019). A coevolved EDS1-SAG101-NRG1 module mediates cell death signaling by TIR-domain immune receptors. Plant Cell. 31, 2430–2455. doi: 10.1105/tpc.19.00118
Lewis, L. A., Polanski, K., de Torres-Zabala, M., Jayaraman, S., Bowden, L., Moore, J., et al. (2015). Transcriptional dynamics driving MAMP-triggered immunity and pathogen effector-mediated immunosuppression in Arabidopsis leaves following infection with Pseudomonas syringae pv tomato DC3000. Plant Cell 27, 3038–3064. doi: 10.1105/tpc.15.00471
Li, B., Jiang, S., Yu, X., Cheng, C., Chen, S., Cheng, Y., et al. (2015). Phosphorylation of trihelix transcriptional repressor ASR3 by MAP KINASE4 negatively regulates Arabidopsis immunity. Plant Cell 27, 839–856. doi: 10.1105/tpc.114.134809
Liu, S., Kracher, B., Ziegler, J., Birkenbihl, R. P., and Somssich, I. E. (2015). Negative regulation of ABA signaling by WRKY33 is critical for Arabidopsis immunity towards Botrytis cinerea 2100. Elife 4:e07295. doi: 10.7554/eLife.07295.033
Lu, H., Salimian, S., Gamelin, E., Wang, G., Fedorowski, J., LaCourse, W., et al. (2009). Genetic analysis of acd6-1 reveals complex defense networks and leads to identification of novel defense genes in Arabidopsis. Plant J. 58, 401–412. doi: 10.1111/j.1365-313X.2009.03791.x
Lu, Y., Truman, W., Liu, X., Bethke, G., Zhou, M., Myers, C. L., et al. (2018). Different modes of negative regulation of plant immunity by calmodulin-related genes. Plant Physiol. 176, 3046–3061. doi: 10.1104/pp.17.01209
Ma, S., Lapin, D., Liu, L., Sun, Y., Song, W., Zhang, X., et al. (2020). Direct pathogen-induced assembly of an NLR immune receptor complex to form a holoenzyme. Science 370:3069. doi: 10.1126/science.abe3069
MacQueen, A., and Bergelson, J. (2016). Modulation of R-gene expression across environments. J. Exp. Bot. 67, 2093–2105. doi: 10.1093/jxb/erv530
Mang, H. G., Qian, W., Zhu, Y., Qian, J., Kang, H. G., Klessig, D. F., et al. (2012). Abscisic acid deficiency antagonizes high-temperature inhibition of disease resistance through enhancing nuclear accumulation of resistance proteins SNC1 and RPS4 in Arabidopsis. Plant Cell 24, 1271–1284. doi: 10.1105/tpc.112.096198
Manohar, M., Wang, D., Manosalva, P. M., Choi, H. W., Kombrink, E., and Klessig, D. F. (2017). Members of the abscisic acid co-receptor PP2C protein family mediate salicylic acid–abscisic acid crosstalk. Plant Direct. 1:e00020. doi: 10.1002/pld3.20
Martin, R., Qi, T., Zhang, H., Liu, F., King, M., Toth, C., et al. (2020). Structure of the activated Roq1 resistosome directly recognizing the pathogen effector XopQ. Science 370:abd9993. doi: 10.1126/science.abd9993
Meyers, B. C., Kozik, A., Griego, A., Kuang, H., and Michelmore, R. W. (2003). Genome-wide analysis of NBS-LRR–encoding genes in Arabidopsis. Plant Cell 15, 809–834. doi: 10.1105/tpc.009308
Mine, A., Seyfferth, C., Kracher, B., Berens, M. L., Becker, D., and Tsuda, K. (2018). The defense phytohormone signaling network enables rapid, high-amplitude transcriptional reprogramming during effector-triggered immunity. Plant Cell 30, 1199–1219. doi: 10.1105/tpc.17.00970
Mohr, P. G., and Cahill, D. M. (2007). Suppression by ABA of salicylic acid and lignin accumulation and the expression of multiple genes, in Arabidopsis infected with Pseudomonas syringae pv. tomato. Funct. Integr. Genomic. 7, 181–191. doi: 10.1007/s10142-006-0041-4
Mohr, T. J., Mammarella, N. D., Hoff, T., Woffenden, B. J., Jelesko, J. G., and McDowell, J. M. (2010). The Arabidopsis downy mildew resistance gene RPP8 is induced by pathogens and salicylic acid and is regulated by W box cis elements. Mol. Plant Microbe Interact. 23, 1303–1315. doi: 10.1094/MPMI-01-10-0022
Monteiro, F., and Nishimura, M. T. (2018). Structural, functional, and genomic diversity of plant NLR proteins: an evolved resource for rational engineering of plant immunity. Annu. Rev. Phytopathol. 56, 243–267. doi: 10.1146/annurev-phyto-080417-045817
Mühlenbock, P., Szechyńska-Hebda, M., Płaszczyca, M., Baudo, M., Mateo, A., Mullineaux, P. M., et al. (2008). Chloroplast signaling and LESION SIMULATING DISEASE1 regulate crosstalk between light acclimation and immunity in Arabidopsis. Plant Cell 20, 2339–2356. doi: 10.1105/tpc.108.059618
Panchal, S., Chitrakar, R., Thompson, B. K., Obulareddy, N., Roy, D., Hambright, W. S., et al. (2016). Regulation of stomatal defense by air relative humidity. Plant Physiol. 172, 2021–2032. doi: 10.1104/pp.16.00696
Pietzenuk, B., Markus, C., Gaubert, H., Bagwan, N., Merotto, A., Bucher, E., et al. (2016). Recurrent evolution of heat-responsiveness in Brassicaceae COPIA elements. Genome Biol. 17, 1–15. doi: 10.1186/s13059-016-1072-3
Qi, D., and Innes, R. W. (2013). Recent advances in plant NLR structure, function, localization, and signaling. Front. Immunol. 4:348. doi: 10.3389/fimmu.2013.00348
Saijo, Y., and Loo, E. P. (2020). Plant immunity in signal integration between biotic and abiotic stress responses. New Phytol. 225, 87–104. doi: 10.1111/nph.15989
Saile, S. C., Jacob, P., Castel, B., Jubic, L. M., Salas-Gonzalez, I., Bäcker, M., et al. (2020). Two unequally redundant “helper” immune receptor families mediate Arabidopsis thaliana intracellular “sensor” immune receptor functions. PLoS Biol. 18:e3000783. doi: 10.1371/journal.pbio.3000783
Schlaen, R. G., Mancini, E., Sanchez, S. E., Perez-Santángelo, S., Rugnone, M. L., Simpson, C. G., et al. (2015). The spliceosome assembly factor GEMIN2 attenuates the effects of temperature on alternative splicing and circadian rhythms. Proc. Natl. Acad. Sci. U.S.A. 112, 9382–9387. doi: 10.1073/pnas.1504541112
Schwachtje, J., Fischer, A., Erban, A., and Kopka, J. (2018). Primed primary metabolism in systemic leaves: a functional systems analysis. Sci. Rep-UK 8, 1–11. doi: 10.1038/s41598-017-18397-5
Sewelam, N., Brilhaus, D., Bräutigam, A., Alseekh, S., Fernie, A. R., and Maurino, V. G. (2020). Molecular plant responses to combined abiotic stresses put a spotlight on unknown and abundant genes. J. Exp. Bot. 71, 5098–5112. doi: 10.1093/jxb/eraa250
Shah, J., Kachroo, P., Nandi, A., and Klessig, D. F. (2001). A recessive mutation in the Arabidopsis SSI2 gene confers SA-and NPR1-independent expression of PR genes and resistance against bacterial and oomycete pathogens. Plant J. 25, 563–574. doi: 10.1046/j.1365-313x.2001.00992.x
Shirano, Y., Kachroo, P., Shah, J., and Klessig, D. F. (2002). A gain-of-function mutation in an Arabidopsis toll interleukin 1 receptor–nucleotide binding site–Leucine-rich repeat type R gene triggers defense responses and results in enhanced disease resistance. Plant Cell 14, 3149–3162. doi: 10.1105/tpc.005348
Song, L., Huang, S. S., Wise, A., Castanon, R., Nery, J. R., Chen, H., et al. (2016). A transcription factor hierarchy defines an environmental stress response network. Science 354:598. doi: 10.1126/science.aag1550
Suzuki, N., Bassil, E., Hamilton, J. S., Inupakutika, M. A., Zandalinas, S. I., Tripathy, D., et al. (2016). ABA is required for plant acclimation to a combination of salt and heat stress. PLoS ONE 11:e0147625. doi: 10.1371/journal.pone.0147625
Tan, X., Meyers, B. C., Kozik, A., West, M. A., Morgante, M., St Clair, D. A., et al. (2007). Global expression analysis of nucleotide binding site-leucine rich repeat-encoding and related genes in Arabidopsis. BMC Plant Biol. 7:56. doi: 10.1186/1471-2229-7-56
Tao, Y., Xie, Z., Chen, W., Glazebrook, J., Chang, H. S., Han, B., et al. (2003). Quantitative nature of Arabidopsis responses during compatible and incompatible interactions with the bacterial pathogen Pseudomonas syringae. Plant Cell 15, 317–330. doi: 10.1105/tpc.007591
Toh, S., Imamura, A., Watanabe, A., Nakabayashi, K., Okamoto, M., Jikumaru, Y., et al. (2008). High temperature-induced abscisic acid biosynthesis and its role in the inhibition of gibberellin action in Arabidopsis seeds. Plant Physiol. 146, 1368–1385. doi: 10.1104/pp.107.113738
Tsuda, K., and Katagiri, F. (2010). Comparing signaling mechanisms engaged in pattern-triggered and effector-triggered immunity. Curr. Opin. Plant Biol. 13, 459–465. doi: 10.1016/j.pbi.2010.04.006
Van de Weyer, A. L., Monteiro, F., Furzer, O. J., Nishimura, M. T., Cevik, V., Witek, K., et al. (2019). A species-wide inventory of NLR genes and alleles in Arabidopsis thaliana. Cell 178, 1260–1272. doi: 10.1016/j.cell.2019.07.038
van Wersch, R., Li, X., and Zhang, Y. (2016). Mighty dwarfs: Arabidopsis autoimmune mutants and their usages in genetic dissection of plant immunity. Front. Plant Sci. 7:1717. doi: 10.3389/fpls.2016.01717
Wan, L., Essuman, K., Anderson, R. G., Sasaki, Y., Monteiro, F., Chung, E. H., et al. (2019). TIR domains of plant immune receptors are NAD+-cleaving enzymes that promote cell death. Science 365, 799–803. doi: 10.1126/science.aax1771
Wan, W. L., Kim, S. T., Castel, B., Charoennit, N., and Chae, E. (2020). Genetics of autoimmunity in plants: an evolutionary genetics perspective. New Phytol. 229, 1215–1233. doi: 10.1111/nph.16947
Wang, L., Tsuda, K., Truman, W., Sato, M., Nguyen, L. V., Katagiri, F., et al. (2011). CBP60g and SARD1 play partially redundant critical roles in salicylic acid signaling. Plant J. 67, 1029–1041. doi: 10.1111/j.1365-313X.2011.04655.x
Wang, W., Feng, B., Zhou, J. M., and Tang, D. (2020). Plant immune signaling: advancing on two frontiers. J. Integr. Plant Biol. 62, 2–24. doi: 10.1111/jipb.12898
Wang, X., Gao, J., Zhu, Z., Dong, X., Wang, X., Ren, G., et al. (2015). TCP transcription factors are critical for the coordinated regulation of ISOCHORISMATE SYNTHASE 1 expression in Arabidopsis thaliana. Plant J. 82, 151–162. doi: 10.1111/tpj.12803
Wang, Y., Bao, Z., Zhu, Y., and Hua, J. (2009). Analysis of temperature modulation of plant defense against biotrophic microbes. Mol. Plant Microbe Interact. 22, 498–506. doi: 10.1094/MPMI-22-5-0498
Weng, J. K., Ye, M., Li, B., and Noel, J. P. (2016). Co-evolution of hormone metabolism and signaling networks expands plant adaptive plasticity. Cell 166, 881–893. doi: 10.1016/j.cell.2016.06.027
Wong, M. M., Bhaskara, G. B., Wen, T. N., Lin, W. D., Nguyen, T. T., Chong, G. L., et al. (2019). Phosphoproteomics of Arabidopsis highly ABA-induced1 identifies AT-Hook–Like10 phosphorylation required for stress growth regulation. Proc. Natl. Acad. Sci. U.S.A. 116, 2354–2363. doi: 10.1073/pnas.1819971116
Wu, Y., Gao, Y., Zhan, Y., Kui, H., Liu, H., Yan, L., et al. (2020). Loss of the common immune coreceptor BAK1 leads to NLR-dependent cell death. Proc. Natl. Acad. Sci. U.S.A. 117, 27044–27053. doi: 10.1073/pnas.1915339117
Wu, Z., Li, M., Dong, O. X., Xia, S., Liang, W., Bao, Y., et al. (2019). Differential regulation of TNL-mediated immune signaling by redundant helper CNLs. New Phytol. 222, 938–953. doi: 10.1111/nph.15665
Xiao, S., Brown, S., Patrick, E., Brearley, C., and Turner, J. G. (2003). Enhanced transcription of the Arabidopsis disease resistance genes RPW8. 1 and RPW8. 2 via a salicylic acid–dependent amplification circuit is required for hypersensitive cell death. Plant Cell 15, 33–45. doi: 10.1105/tpc.006940
Yamada, K., Yamaguchi, K., Shirakawa, T., Nakagami, H., Mine, A., Ishikawa, K., et al. (2016). The Arabidopsis CERK 1-associated kinase PBL 27 connects chitin perception to MAPK activation. EMBO J. 35, 2468–2483. doi: 10.15252/embj.201694248
Yang, H., Shi, Y., Liu, J., Guo, L., Zhang, X., and Yang, S. (2010). A mutant CHS3 protein with TIR-NB-LRR-LIM domains modulates growth, cell death and freezing tolerance in a temperature-dependent manner in Arabidopsis. Plant J 63, 283–296. doi: 10.1111/j.1365-313X.2010.04241.x
Yang, L., Chen, X., Wang, Z., Sun, Q., Hong, A., Zhang, A., et al. (2020). HOS15 and HDA9 negatively regulate immunity through histone deacetylation of intracellular immune receptor NLR genes in Arabidopsis. New Phytol. 226, 507–522. doi: 10.1111/nph.16380
Yang, L., Teixeira, P. J., Biswas, S., Finkel, O. M., He, Y., Salas-Gonzalez, I., et al. (2017). Pseudomonas syringae type III effector HopBB1 promotes host transcriptional repressor degradation to regulate phytohormone responses and virulence. Cell Host. Microbe 21, 156–168. doi: 10.1016/j.chom.2017.01.003
Yang, S., and Hua, J. (2004). A haplotype-specific resistance gene regulated by BONZAI1 mediates temperature-dependent growth control in Arabidopsis. Plant Cell 16, 1060–1071. doi: 10.1105/tpc.020479
Yang, W., Dong, R., Liu, L., Hu, Z., Li, J., Wang, Y., et al. (2016). A novel mutant allele of SSI2 confers a better balance between disease resistance and plant growth inhibition on Arabidopsis thaliana. BMC Plant Biol. 16, 1–13 doi: 10.1186/s12870-016-0898-x
Yasuda, M., Ishikawa, A., Jikumaru, Y., Seki, M., Umezawa, T., Asami, T., et al. (2008). Antagonistic interaction between systemic acquired resistance and the abscisic acid–mediated abiotic stress response in Arabidopsis. Plant Cell 20, 1678–1692. doi: 10.1105/tpc.107.054296
Yi, H., and Richards, E. J. (2007). A cluster of disease resistance genes in Arabidopsis is coordinately regulated by transcriptional activation and RNA silencing. Plant Cell 19, 2929–2939. doi: 10.1105/tpc.107.051821
Zhan, X., Qian, B., Cao, F., Wu, W., Yang, L., Guan, Q., et al. (2015). An Arabidopsis PWI and RRM motif-containing protein is critical for pre-mRNA splicing and ABA responses. Nat. Commun. 6, 1–12. doi: 10.1038/ncomms9139
Zhang, C., Gao, M., Seitz, N. C., Angel, W., Hallworth, A., Wiratan, L., et al. (2019). LUX ARRHYTHMO mediates crosstalk between the circadian clock and defense in Arabidopsis. Nat. Commun. 10, 1–14. doi: 10.1038/s41467-019-10485-6
Zhang, S. S., Yang, H., Ding, L., Song, Z. T., Ma, H., Chang, F., et al. (2017). Tissue-specific transcriptomics reveals an important role of the unfolded protein response in maintaining fertility upon heat stress in Arabidopsis. Plant Cell 29, 1007–1023. doi: 10.1105/tpc.16.00916
Zhang, Y., Xu, S., Ding, P., Wang, D., Cheng, Y. T., He, J., et al. (2010). Control of salicylic acid synthesis and systemic acquired resistance by two members of a plant-specific family of transcription factors. Proc. Natl. Acad. Sci. U.S.A. 107, 18220–18225. doi: 10.1073/pnas.1005225107
Zhao, C., Zhang, Z., Xie, S., Si, T., Li, Y., and Zhu, J. K. (2016). Mutational evidence for the critical role of CBF transcription factors in cold acclimation in Arabidopsis. Plant Physiol. 171, 2744–2759 doi: 10.1104/pp.16.00533
Zheng, X. Y., Spivey, N. W., Zeng, W., Liu, P. P., Fu, Z. Q., Klessig, D. F., et al. (2012). Coronatine promotes Pseudomonas syringae virulence in plants by activating a signaling cascade that inhibits salicylic acid accumulation. Cell Host Microbe 11, 587–596. doi: 10.1016/j.chom.2012.04.014
Zhu, Y., Qian, W., and Hua, J. (2010). Temperature modulates plant defense responses through NB-LRR proteins. PLoS Pathog. 6:e1000844. doi: 10.1371/journal.ppat.1000844
Zhu, Y., Wang, B., Tang, K., Hsu, C. C., Xie, S., Du, H., et al. (2017). An Arabidopsis nucleoporin NUP85 modulates plant responses to ABA and salt stress. PLoS Genet. 13:e1007124. doi: 10.1371/journal.pgen.1007124
Zou, B., Sun, Q., Zhang, W., Ding, Y., Yang, D. L., Shi, Z., et al. (2017). The Arabidopsis chromatin-remodeling factor CHR5 regulates plant immune responses and nucleosome occupancy. Plant Cell Physiol. 58, 2202–2216. doi: 10.1093/pcp/pcx155
Keywords: NLR, biotic stress, abiotic stress, intracellular immune receptor, Arabidopsis
Citation: Yang L, Wang Z and Hua J (2021) A Meta-Analysis Reveals Opposite Effects of Biotic and Abiotic Stresses on Transcript Levels of Arabidopsis Intracellular Immune Receptor Genes. Front. Plant Sci. 12:625729. doi: 10.3389/fpls.2021.625729
Received: 03 November 2020; Accepted: 01 February 2021;
Published: 04 March 2021.
Edited by:
Giorgio Gambino, National Research Council (CNR), ItalyReviewed by:
Xin Li, University of British Columbia, CanadaEunyoung Chae, National University of Singapore, Singapore
Copyright © 2021 Yang, Wang and Hua. This is an open-access article distributed under the terms of the Creative Commons Attribution License (CC BY). The use, distribution or reproduction in other forums is permitted, provided the original author(s) and the copyright owner(s) are credited and that the original publication in this journal is cited, in accordance with accepted academic practice. No use, distribution or reproduction is permitted which does not comply with these terms.
*Correspondence: Jian Hua, amgyOTlAY29ybmVsbC5lZHU=