- 1Department of Plant Biology, University of Illinois at Urbana-Champaign, Urbana, IL, United States
- 2Department of Crop Sciences, University of Illinois at Urbana-Champaign, Urbana, IL, United States
- 3Carl R. Woese Institute for Genomic Biology, University of Illinois at Urbana-Champaign, Urbana, IL, United States
- 4Lancaster Environment Centre, Lancaster University, Lancaster, United Kingdom
- 5Department of Plant Sciences, University of Cambridge, Cambridge, United Kingdom
Improving leaf intrinsic water-use efficiency (iWUE), the ratio of photosynthetic CO2 assimilation to stomatal conductance, could decrease crop freshwater consumption. iWUE has primarily been studied under steady-state light, but light in crop stands rapidly fluctuates. Leaf responses to these fluctuations substantially affect overall plant performance. Notably, photosynthesis responds faster than stomata to decreases in light intensity: this desynchronization results in substantial loss of iWUE. Traits that could improve iWUE under fluctuating light, such as faster stomatal movement to better synchronize stomata with photosynthesis, show significant natural diversity in C3 species. However, C4 crops have been less closely investigated. Additionally, while modification of photosynthetic or stomatal traits independent of one another will theoretically have a proportionate effect on iWUE, in reality these traits are inter-dependent. It is unclear how interactions between photosynthesis and stomata affect natural diversity in iWUE, and whether some traits are more tractable drivers to improve iWUE. Here, measurements of photosynthesis, stomatal conductance and iWUE under steady-state and fluctuating light, along with stomatal patterning, were obtained in 18 field-grown accessions of the C4 crop sorghum. These traits showed significant natural diversity but were highly correlated, with important implications for improvement of iWUE. Some features, such as gradual responses of photosynthesis to decreases in light, appeared promising for improvement of iWUE. Other traits showed tradeoffs that negated benefits to iWUE, e.g., accessions with faster stomatal responses to decreases in light, expected to benefit iWUE, also displayed more abrupt losses in photosynthesis, resulting in overall lower iWUE. Genetic engineering might be needed to break these natural tradeoffs and achieve optimal trait combinations, e.g., leaves with fewer, smaller stomata, more sensitive to changes in photosynthesis. Traits describing iWUE at steady-state, and the change in iWUE following decreases in light, were important contributors to overall iWUE under fluctuating light.
Introduction
Water is the primary abiotic factor limiting crop productivity (Boyer, 1982), with agriculture consuming up to 85% of freshwater withdrawals (WWAP, 2015; D’Odorico et al., 2020). Breeding has almost tripled productivity of major crops over the last 60 years, without parallel improvement in the amount of water required to produce a ton of crop biomass (Sinclair et al., 1984; Ort and Long, 2014). With changing patterns of precipitation and increased drought frequency (Dai, 2013; Spinoni et al., 2018; Sun et al., 2018), rising atmospheric vapor pressure deficit due to global warming (Ort and Long, 2014), and decreasing groundwater supply around the world (Dalin et al., 2017), supplying sufficient water to crops is increasingly difficult and unsustainable (Lobell et al., 2014; WWAP, 2015). Therefore, improving crop water-use efficiency is important to achieve the crop productivity required to meet global demand (Bonsch et al., 2016; Flexas, 2016; FAO et al., 2018; Leakey et al., 2019).
The dependence of crop productivity on water supply derives from the interaction between leaf photosynthetic CO2 assimilation (A) and transpiration. Stomatal pores on the leaf surface allow CO2 diffusion into the leaf for A, but also allow water vapor to escape from the leaf. The inverse of the resistance to water vapor loss collectively imposed by the stomatal pores is measured as the stomatal conductance to water vapor (gs). The ratio of A to gs gives leaf intrinsic water-use efficiency (iWUE) (Leakey et al., 2019). Most inter- and intra-specific surveys of A, gs and iWUE, and analyses of their limitations have concerned steady-state conditions (Galmes et al., 2007; Giuliani et al., 2013; Driever et al., 2014; Jahan et al., 2014; Sollenberger et al., 2014; Viswanathan et al., 2014; Tomeo and Rosenthal, 2017; Yabiku and Ueno, 2017; Leakey et al., 2019).
Although steady-state measurements are important to understand plant physiological performance (von Caemmerer and Farquhar, 1981; von Caemmerer, 2000), leaves in field-grown crop canopies are rarely in steady-state light conditions, and experience frequent fluctuations in incident photosynthetic photon flux density (PPFD) that cause substantial deviations from steady-state carbon and water fluxes (Pearcy, 1990; Zhu et al., 2004; Kaiser et al., 2015, 2018; McAusland et al., 2016; Vialet-Chabrand et al., 2017; Slattery et al., 2018; Acevedo-Siaca et al., 2020; De Souza et al., 2020; Wang et al., 2020). Evidence of increased water deficit stress (Sun et al., 2017), temperature stress (Leakey et al., 2003), and sensitivity of A to elevated CO2 (Leakey et al., 2002; Tomimatsu and Tang, 2016) under fluctuating PPFD, and recent breakthroughs in improving A under fluctuating PPFD by manipulating photoprotection (Kromdijk et al., 2016; Hubbart et al., 2018), chlorophyll content (Gu et al., 2017), and stomatal light-sensing (Papanatsiou et al., 2019), have demonstrated the value of considering leaf behavior under non-steady-state lighting conditions.
A and gs typically require several minutes to rise to a new steady-state following an increase in PPFD, as in a sun fleck within a crop canopy. The rate of increase in gs may be mechanically limited by stomatal opening kinetics and stomatal patterning. The rate of increase in A may be limited by the kinetics of biochemical processes, in particular Rubisco activation (Soleh et al., 2016, 2017; Acevedo-Siaca et al., 2020), or the kinetics of stomatal opening and the associated mitigation of intercellular CO2 (ci)-limitation to A (Lawson and Blatt, 2014; McAusland et al., 2016; De Souza et al., 2020). Because the response times of A and gs are similar following an increase in PPFD, the deviation of iWUE from steady-state is relatively modest (McAusland et al., 2016). In contrast, the response times of A and gs are more distinct following a decrease in PPFD, as A typically declines to a new steady-state within seconds while gs decreases over the course of several minutes as stomata close (Lawson et al., 2011; Way and Pearcy, 2012; Lawson and Blatt, 2014; McAusland et al., 2016; Lawson and Vialet-Chabrand, 2019). This lag in gs relative to A leads to continued water loss, resulting in reduced iWUE. While the decrease in A is often simplified as a step-change from one steady-state to the next (McAusland et al., 2016; Bellasio et al., 2017), physiological processes such as post-illumination decarboxylation of photorespiratory metabolites and slow relaxation of photoprotective mechanisms may temporarily suppress A following a decrease in PPFD (Doncaster et al., 1989; Kaiser et al., 2015; Kromdijk et al., 2016; Wang et al., 2020).
Attempts to improve leaf physiology under fluctuating light have generally followed three axes: (1) accelerate induction of A following increases in PPFD (Soleh et al., 2016, 2017; Taylor and Long, 2017; Acevedo-Siaca et al., 2020; Wang et al., 2020), (2) limit the inhibition of A following decreases in PPFD (Kromdijk et al., 2016; Wang et al., 2020), (3) accelerate stomatal movement to reduce gs response time, leading to improved A following increases in PPFD and improved iWUE following decreases in PPFD (Wang et al., 2014; Papanatsiou et al., 2019; Lawson and Matthews, 2020). An essential component of this research has been the discovery of broad diversity in A, gs, and iWUE in fluctuating light across species (McAusland et al., 2016; Deans et al., 2019) and within C3 species such as rice (Acevedo-Siaca et al., 2020), cassava (De Souza et al., 2020), and soybean (Soleh et al., 2016, 2017; Wang et al., 2020). In contrast, within-species diversity in C4 species is not well understood, despite use of the C4 pathway by some of the world’s most productive crops, including maize (Zea mays L.), sorghum (Sorghum bicolor (Lu.) Moench), and sugarcane (Saccharum officinarum L.). Further, while modification of A or gs traits independent of one another will theoretically have a proportionate effect on iWUE, in reality these traits are inter-dependent. It is unclear how interactions between these traits affect natural diversity in iWUE, and whether some traits might be more tractable drivers for improvement of iWUE. In C4 crops, there is the added complexity of the carbon-concentrating mechanism, which may alter the relationship between A and gs relative to C3 species (McAusland et al., 2016). Similarly, it is unclear whether steady-state and non-steady-state traits interact with one another and are predictive of overall leaf gas-exchange under fluctuating light.
Here, steady-state and non-steady-state iWUE were examined in the model C4 crop sorghum. Sorghum is a key food crop for water-limited regions of the globe and fiber sorghum is among the most productive potential cellulosic bioenergy crops of the warm temperate zone, characterized by high drought-tolerance water-use efficiency and productivity (Regassa and Wortmann, 2014; Hadebe et al., 2017). This study asked three questions:
(1) Is there significant diversity in non-steady-state responses of A, gs and iWUE to light fluctuations among diverse sorghum accessions?
(2) What are the main drivers for natural variation in iWUE in terms of steady-state A and gs, non-steady-state A and gs, and stomatal patterning?
(3) Are steady-state and non-steady-state gas-exchange traits predictive of leaf performance under fluctuating PPFD?
We analyzed gas exchange and stomatal patterning in 18 diverse sorghum accessions under steady-state and fluctuating PPFD conditions. Our results identify drivers for improvement of A and iWUE, including stomatal size and non-steady-state A and iWUE traits, and demonstrate that the sorghum pan-genome harbors significant potential for improving the already impressive iWUE of sorghum via breeding.
Materials and Methods
Plant Material and Growing Conditions
Seeds of 18 sorghum accessions were planted on May 28, 2017 at the University of Illinois Energy Farm near Urbana, IL, United States (40°07′N, 5 88°21′W, 228 m above sea level) in 3 m rows at 25 seeds m–1, in plots of four rows spaced 76 cm apart. The accessions were a subset of a biomass sorghum diversity panel that was previously screened to identify transient stomatal responses to a decrease in PPFD (Pignon, 2017). Soils are deep silt loam Flanagan and silty clay loam Drummer. Plants were fertilized (101 kg/ha N) and rainfed. In August, the youngest fully expanded leaf was sampled from 3 to 5 plants per accession. Leaves were cut pre-dawn, recut with the cut-end underwater, and transferred to the laboratory for analysis.
Gas Exchange Measurements
Leaf cuvettes of two portable photosynthetic gas-exchange systems (LI-6400XT; LI-COR, Inc., Lincoln, NE, United States with LI-6400-40 Leaf-Chamber-Fluorometer) were clamped onto each side of the midrib at the middle of each leaf. PPFD was set to 2000 μmol m–2 s–1, block temperature to 25°C, flow rate to 700 μmol s–1, [CO2] in the sample cell set to 400 ppm and leaf-to-air VPD maintained <2 kPa. LEDs provided 10% blue and 90% red light. Leaves were acclimated to these conditions for an hour, then measurements began.
Because increases in PPFD are typically less impactful to iWUE than decreases in PPFD, this study focused on extracting non-steady-state traits following decreases in PPFD. For each leaf, one cuvette measured steady-state PPFD response curves, which were used to obtain A, gs, and iWUE at steady-state, and to evaluate the transition from one steady-state to the next after decreases in PPFD. Curves were obtained by decreasing PPFD (2000, 1500, 1000, 500, 200, 100, and 0 μmol m–2 s–1) in 15 min steps, with data recorded every 10 s. Though useful for the purposes of quantifying steady-state and non-steady-state gas-exchange, this PPFD timecourse was highly artificial and provided limited information about repeated PPFD fluctuations, since it was only composed of lengthy step declines in PPFD. Therefore, in the second cuvette, additional measurements were used to determine whether the traits derived from steady-state PPFD response curves were predictive of performance in a repeatedly fluctuating PPFD environment. Fluctuating PPFD response curves were obtained by varying PPFD in 5.5 min steps in the following sequence: 2000, 1500, 2000, 1000, 2000, 500, 2000, 200, 2000, and 100 μmol m–2 s–1, with data recorded every 10 s.
Variation in environmental variables throughout gas-exchange measurements is given in Supplementary Table 1. On completion of gas-exchange the measured leaf tissue was sampled and frozen for stomatal phenotyping.
Stomatal Phenotyping
Leaf tissue was mounted on glass slides and the abaxial surface was imaged using an optical topometer (μsurf Explorer, NanoFocus, Karlsruhe, Germany) with 20× (0.8 mm2 leaf surface, 20× M Plan APO, Olympus Corporation, Tokyo, Japan) and 50× air objectives (0.32 mm2 leaf surface, 50× UM Plan FL N, Olympus Corporation). Reconstructions of the leaf epidermis were obtained from serial optical sections measured from surface to inside of leaf, with measurement depth of 40 μm and 20 μm for the 20× and 50× objectives, respectively (μsurf Metrology, NanoFocus). Four images at 20×, and one image at 50×, were taken per leaf. Stomata on 20× images were counted to estimate stomatal density. The size, i.e., planar surface area, of the stomatal complex was measured by outlining relevant pixels of four stomata per 50× image using ImageJ (ImageJ1.51j8, NIH, United States).
Gas-Exchange Data Analysis
Analysis of Steady-State PPFD Response Curves
Steady-state net rate of leaf photosynthetic CO2 uptake (A2000), stomatal conductance to water vapor (gs 2000) and intrinsic water-use efficiency (iWUE2000) at PPFD = 2000 μmol m–2 s–1 were obtained as the average for the two cuvettes (i.e., two technical reps) for each leaf over the last 40 s of the initial 1 h acclimation period. Steady-state A, gs, and iWUE were also obtained for each PPFD in the steady-state PPFD response curve, as the average over the last 40 s of each PPFD.
After each decrease in PPFD, A declined from one steady-state to the next, often displaying a temporary inhibition as it decreased below steady-state (i.e., undershoot), then increased again to steady-state. This process was described by two traits: t95 A and Aundershoot. t95 A was the time required for A to come within 5% of steady-state after a decrease in PPFD, where smaller t95 A indicates that A reached steady-state more rapidly. Aundershoot was the difference between steady-state A and the minimum A reached at a given PPFD, where more negative Aundershoot indicates more pronounced undershoot of steady-state. Corresponding traits were used to describe gs (t95 gs, gs undershoot).
The response of iWUE to each decrease in PPFD was different from that of A and gs, as iWUE first abruptly declined, reflecting an instantaneous loss of A while gs remained relatively high. In the following minutes, as gs declined, iWUE increased toward steady-state. t95 iWUE was calculated analogous to t95 A and t95 gs, and iWUEundershoot was calculated analogous to Aundershoot and gs undershoot.
Analysis of Fluctuating PPFD Response Curves
Average A, gs and iWUE were computed throughout the fluctuating PPFD response curves. If responses of A, gs, and iWUE to changes in PPFD were instantaneous, then gas-exchange under fluctuating PPFD would be equivalent to steady-state gas-exchange at each PPFD. Instead, A, gs, and iWUE showed substantial deviations from steady-state following changes in PPFD. Therefore, overall gas-exchange under fluctuating PPFD could be described as the sum of steady-state gas-exchange and the deviation of gas-exchange from steady-state following each PPFD fluctuation. To assess the importance of non-steady-state traits separate from steady-state, the deviation of gas-exchange from steady-state under fluctuating PPFD was calculated. Because steady-state and fluctuating PPFD response curves were measured on the same leaves, data from both types of curves were combined. Deviation of A from steady-state was calculated as follows: (1) A from the fluctuating PPFD response curves was normalized to A2000, (2) steady-state A from the steady-state PPFD response curve was obtained for each PPFD, and also normalized to A2000, (3) The difference between (1) and (2) was calculated at each PPFD, yielding the deviation of A from steady-state, normalized to A2000. This deviation is positive when A is greater than steady-state, negative when A is less than steady-state, and 0 when A is equal to steady-state. The average deviation of A from steady-state was calculated following increases and decreases in PPFD. The same method was applied to iWUE.
To test whether leaves cut from plants and measured in the laboratory might behave differently from leaves still attached to plants, an experiment was performed comparing the two types of leaves. There was no substantial effect of leaf excision on steady-state or non-steady-state A, gs, and iWUE (Supplementary Figure 9).
Statistical Analysis
A summary of all traits analyzed in this study is given in Table 1. ANOVA was used to test the fixed effect of sorghum accession on gas-exchange and physiology traits with the aov() function in the package stats (R 3.6.1, R Core Team, 2017). There were 3–5 plants sampled per accession, with one plot per accession, such that each plant was a pseudo replicate. Time of day of measurements and leaf-to-air VPD were included as cofactors for gas-exchange measurements. If neither cofactor was significant for a given trait, then a simpler model testing only the effect of accession was used. A2000, gs 2000, and iWUE2000 were the average of two technical replicates per plant, and stomatal density and size were the average of four technical replicates per plant; all other measurements had one technical replicate per plant.
Homogeneity of variances was tested by the Levene test using function LeveneTest() in package DescTools (Signorell, 2020) and normality of studentized residuals tested by Shapiro–Wilk using function ols_test_normality in package olsrr (Hebbali, 2020) at p = 0.01 threshold. The assumption of normality was violated for t95 A, so the Kruskal–Wallis test was used to analyze this trait using kruskal.test() function in package stats (R Core Team, 2017).
Pairwise correlations were tested at p < 0.05 (significant) and p < 0.1 (marginally significant) thresholds between means per accession for the traits described above using cor.mtest() function in package corrplot (Wei and Simko, 2017).
Results
Variation Among Accessions in Steady-State Gas Exchange and in the Transition From One Steady-State to the Next Following Decreases in PPFD
Two representative accessions, PI153852 and PI152636, exemplify the genetic variation that was observed in steady-state and non-steady-state A, gs, and iWUE (Figure 1). In PI153852, steady-state A and gs at PPFD = 2000 μmol m–2 s–1 were less than in PI152636 (i.e., A2000 and gs 2000, pink datapoints in Figures 1A–D, respectively). After each decrease in PPFD, A and gs declined from one steady-state to the next. In PI153852, decline of A occurred over the course of several minutes, during which A decreased below steady-state (i.e., undershoot), then increased again to steady-state (Figure 1A). In contrast, in PI152636, A reached a new steady-state in under a minute, with a less pronounced undershoot (Figure 1B). In PI153852, gs gradually decreased below steady-state (i.e., undershoot), then increased again to steady-state (Figure 1C). In contrast, in PI152636 gs declined more rapidly to steady-state, with a slightly less pronounced undershoot (Figure 1D).
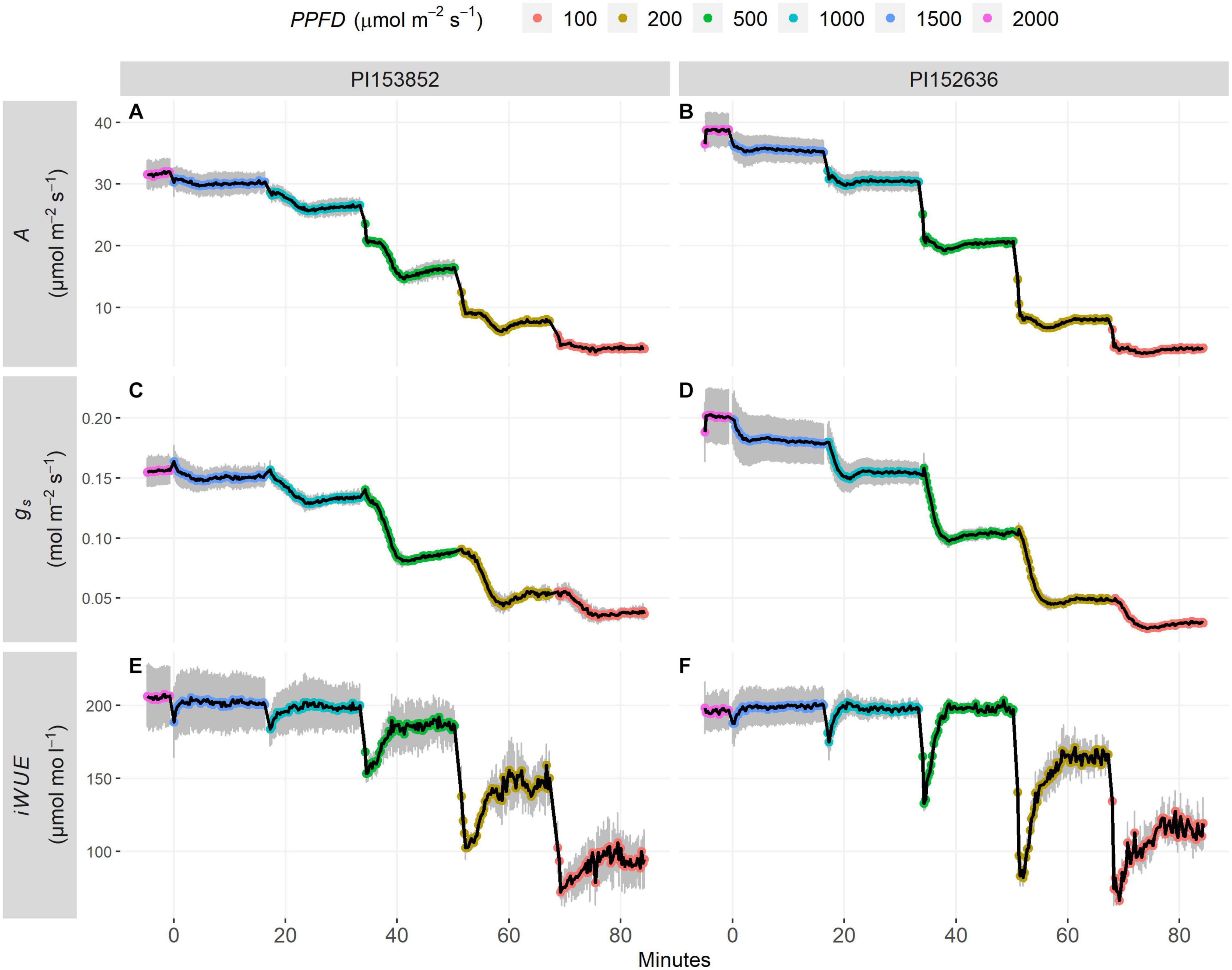
Figure 1. Timecourses of steady-state PPFD response curves in two accessions: (A,C,E) PI153852 and (B,D,F) PI152636. For each curve, leaves were acclimatized to PPFD of 2000 μmol m–2 s–1 for 1 h, then PPFD declined in steps every 15 min. (A,B) A, (C,D) gs, and (E,F) iWUE were logged throughout. Each point is a mean ± s.e. of 4 plants. The final measurements at each PPFD were used to estimate steady-state A, gs, and iWUE at each PPFD. Timecourses were also used to describe the transition from one steady-state to the next after each decrease in PPFD, including the time for A, gs, and iWUE to come within 5% of steady-state, and the undershoot of steady-state by A, gs, and iWUE.
After each decrease in PPFD, iWUE abruptly declined below steady-state (i.e., undershoot), reflecting an instantaneous loss of A while gs remained relatively high. This decline was less pronounced in PI153852 (Figure 1E) than PI152636 (Figure 1F). In the following minutes, iWUE increased to reach steady-state, reflecting a decline of gs to steady-state. This occurred slightly more rapidly in PI153852 (Figure 1E) than in PI152636 (Figure 1F). This suggests that the differences between these two accessions in A and gs at steady-state and non-steady-state translated to differences in iWUE. Steady-state iWUE was relatively stable from PPFD = 500–2000 μmol m–2 s–1, but began to decline at lower PPFD (Figures 1E,F and Supplementary Figure 1).
Among all 18 accessions, there was significant variation in A2000 (p < 0.001, 1.63-fold variation among accessions, Figure 2A), gs 2000 (p < 0.001, 1.78-fold variation among accessions, Figure 2B), and iWUE2000 (p = 0.001, 1.29-fold variation among accessions, Figure 2C), as well as the time required for A (t95 A, p = 0.010, 11-fold variation among accessions, Figure 2D) and gs (t95 gs, p = 0.041, 1.98-fold variation among accessions, Figure 2E) to come within 5% of steady-state after a decrease in PPFD. However, there was no significant variation in the time required for iWUE to come within 5% of steady-state after a decrease in PPFD (t95 iWUE, p = 0.318, 1.65-fold variation among accessions, Figure 2F). There was significant variation in the undershoot of steady-state by A (Aundershoot, p = 0.010, 2.50-fold variation among accessions, Figure 2G), gs (gs undershoot, p = 0.019, 2.51-fold variation among accessions, Figure 2H), and iWUE (iWUEundershoot, p < 0.001, 1.98-fold variation among accessions, Figure 2I) after each decrease in PPFD. A approached steady-state within seconds in most accessions (t95 A < 1 min, Figure 2D), with the slowest decline of A in PI153852 (t95 A = 2.86 min, Figures 1A, 2D). gs and iWUE approached steady-state more slowly than A (t95 gs and t95 iWUE ranging from 2.22 to 4.40 min, Figures 2E,F).
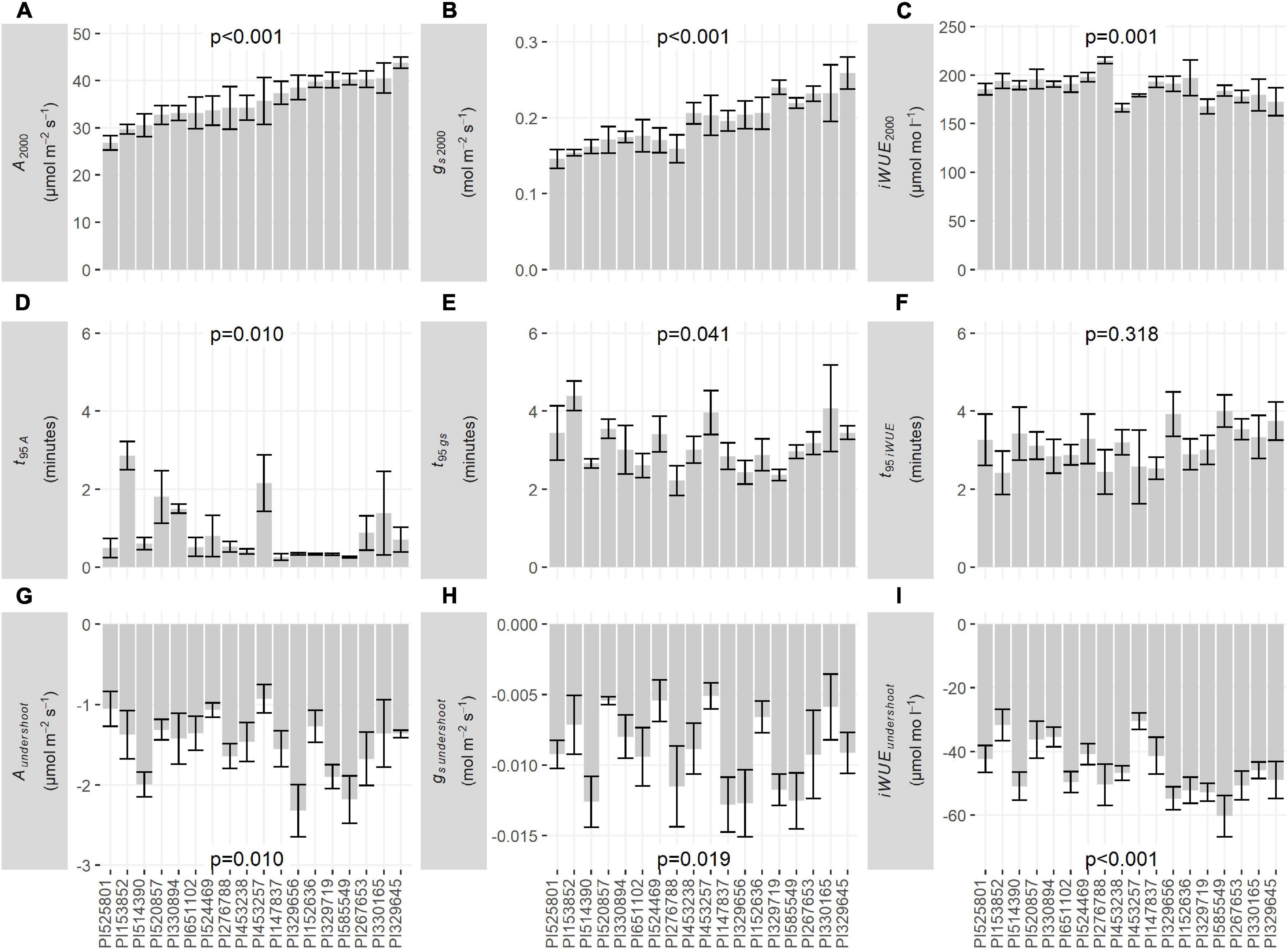
Figure 2. Bar graphs of traits derived from steady-state PPFD response curves: (A) steady-state A at PPFD = 2000 μmol m–2 s–1 (A2000), (B) steady-state gs at PPFD = 2000 μmol m–2 s–1 (gs 2000), (C) steady-state iWUE at PPFD = 2000 μmol m–2 s–1 (iWUE2000), (D) time required for A to come within 5% of steady-state after a decrease in PPFD (t95 A), (E) time required for gs to come within 5% of steady-state after a decrease in PPFD (t95 gs), (F) time required for iWUE to come within 5% of steady-state after a decrease in PPFD (t95 iWUE), (G) undershoot of steady-state by A after a decrease in PPFD, i.e., difference between steady-state A and the minimum A reached at each PPFD (Aundershoot), (H) undershoot of steady-state by gs after a decrease in PPFD, i.e., difference between steady-state gs and the minimum gs reached at each PPFD (gs undershoot), (I) undershoot of steady-state by iWUE after a decrease in PPFD, i.e., difference between steady-state iWUE and the minimum iWUE reached at each PPFD (iWUEundershoot). Bars are mean ± s.e. p-values are from ANOVA testing the fixed effect of accession on each trait.
Variation Among Accessions in Gas Exchange Under Fluctuating PPFD
Fluctuating PPFD response curves were used to determine whether the traits derived from steady-state PPFD response curves were predictive of performance in a fluctuating PPFD environment. Following decreases in PPFD, A rapidly declined to steady-state, while increases in PPFD triggered more gradual increases of A toward steady-state (Figure 3A,B). As a result, A was slightly above steady-state following decreases in PPFD, but substantially below steady-state following increases in PPFD (Figures 3C,D). As in the steady-state PPFD response curves, in accessions such as PI152636 there was little deviation of A from steady-state following decreases in PPFD, whereas in PI153852, A remained above steady-state for several minutes (Figures 3C,D).
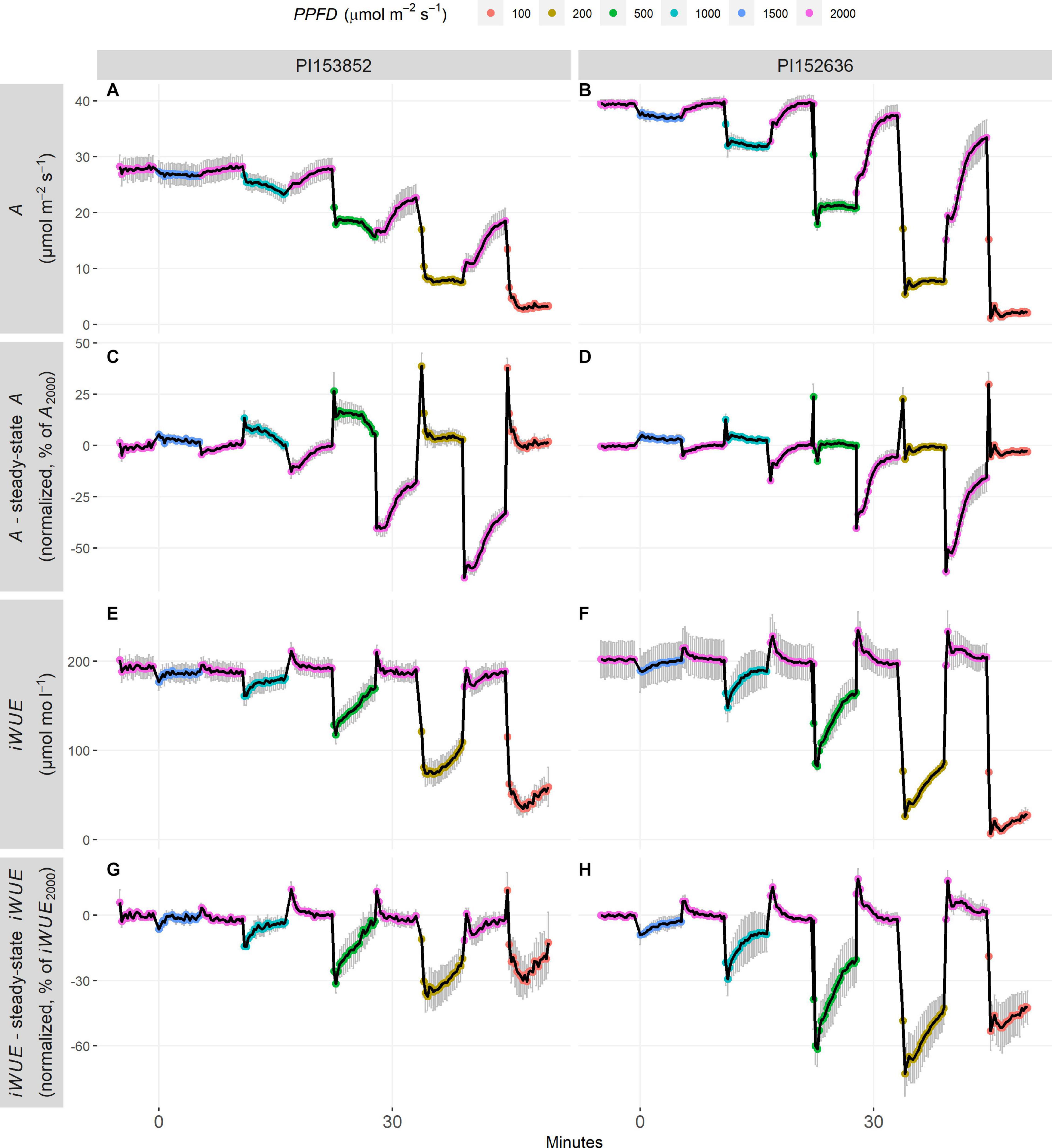
Figure 3. Timecourses of fluctuating PPFD response curves in two accessions: (A,C,E,G) PI153852 and (B,D,F,H) PI152636. For each curve, leaves were acclimatized to PPFD of 2000 μmol m–2 s–1 for 1 h, then PPFD cycled between non-saturating and saturating PPFD every 5.5 min. (A,B) A, (C,D) Deviation of A from steady-state, normalized to A2000, (E,F) iWUE, (G,H) Deviation of iWUE from steady-state, normalized to iWUE2000. Each point is a mean ± s.e. of 3–5 plants. Deviation of A from steady-state was calculated as follows: (1) A from a fluctuating PPFD response curve was normalized to A2000, (2) the steady-state A from a steady-state PPFD curve measured on the same leaf was obtained for each PPFD, and also normalized to A2000, (3) The difference between (1) and (2) was calculated, yielding the deviation of A from steady-state, normalized to A2000, as seen in panels (C,D). This deviation is positive when A is greater than steady-state, negative when A is less than steady-state, and 0 when A is equal to steady-state. The same method was applied to iWUE, as seen in panels (G,H).
Following decreases in PPFD, iWUE abruptly declined, then gradually increased toward steady-state (Figures 3E,F). In contrast, increases in PPFD caused iWUE to rise slightly above steady-state, then return to steady-state (Figures 3E,F). In other words, iWUE was slightly above steady-state following increases in PPFD, but substantially less than steady-state following decreases in PPFD (Figures 3G,H). This resulted from the fact that A declined faster than gs following decreases in PPFD, whereas A and gs increased at a similar rate following increases in PPFD (Supplementary Figures 5–7). In accessions such as PI152636, there was substantial loss of iWUE relative to steady-state following decreases in PPFD, and a slight gain of iWUE relative to steady-state following increases in PPFD, compared to the less pronounced deviation of iWUE from steady-state in accessions such as PI153852 (Figures 3G,H).
Among all accessions, there was significant or marginally significant variation in average A under fluctuating PPFD (p = 0.002, 1.50-fold variation among accessions, Figure 4A), average gs under fluctuating PPFD (p = 0.037, 1.78-fold variation among accessions, Figure 4B), and average iWUE under fluctuating PPFD (p = 0.069, 1.22-fold variation among accessions, Figure 4C), as well as the deviation of A from steady-state following increases in PPFD (p = 0.010, 2.50-fold variation among accessions, Figure 4D), the deviation of iWUE from steady-state following increases in PPFD (p = 0.088, 4.02-fold variation among accessions, Figure 4E), and the deviation of A from steady-state following decreases in PPFD (p = 0.078, 3.07-fold variation among accessions, Figure 4F). However, the experiment could not resolve significant differences among accessions for the deviation of iWUE from steady-state following decreases in PPFD (p = 0.121, 3.15-fold variation among accessions, Figure 4G). Finally, there was significant variation among accessions in stomatal density (p < 0.001, 1.72-fold variation among accessions, Figure 4H) and stomatal size (p < 0.001, 1.59-fold variation among accessions, Figure 4I) determined from optical topometry of the epidermis (Figure 5).
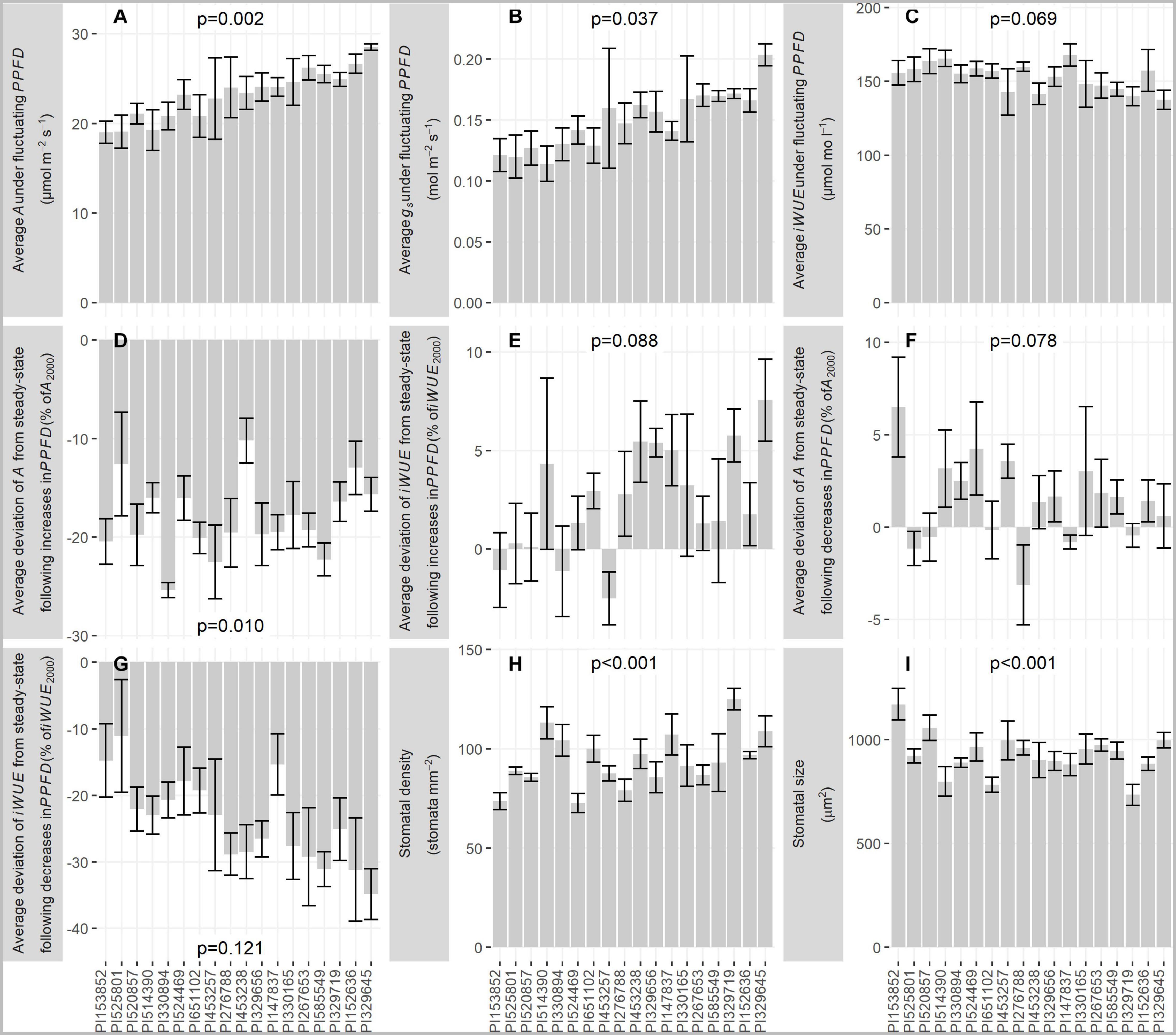
Figure 4. Bar graphs of traits derived from fluctuating PPFD response curves and leaf stomatal profiles: (A) Average A under fluctuating PPFD, (B) Average gs under fluctuating PPFD, (C) Average iWUE under fluctuating PPFD, (D) Deviation of A from steady-state following increases in PPFD, expressed as a% of A2000, (E) Deviation of iWUE from steady-state following increases in PPFD, expressed as a% of iWUE2000, (F) Deviation of A from steady-state following decreases in PPFD, expressed as a% of A2000, (G) Deviation of iWUE from steady-state following decreases in PPFD, expressed as a% of iWUE2000, (H) stomatal density, (I) stomatal size, i.e., planar surface area of the stomatal complex. Bars are mean ± s.e. p-values are from ANOVA testing the fixed effect of accession on each trait.
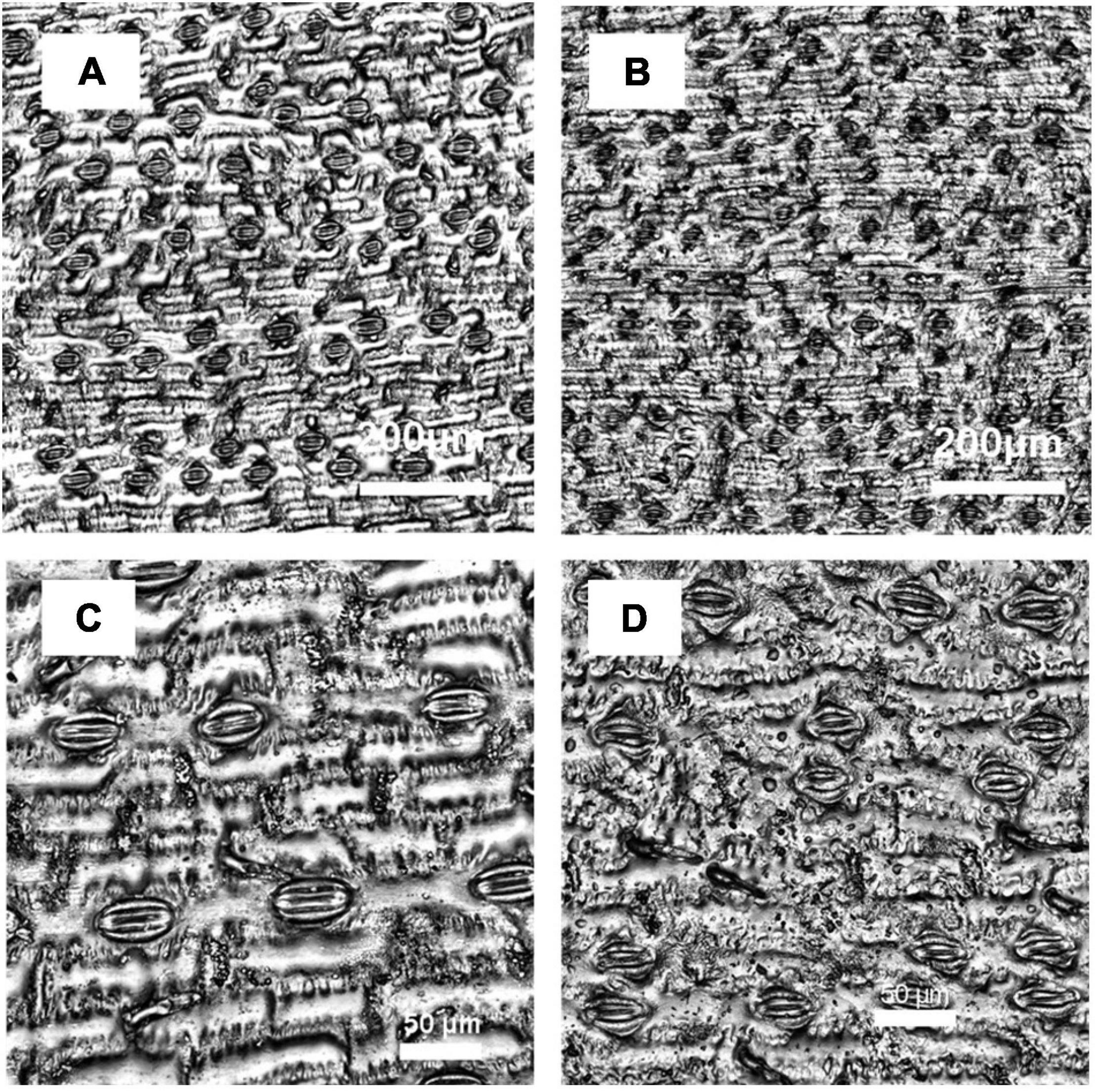
Figure 5. Optical topometry images of leaf abaxial surfaces used for stomatal profiles, measured with: (A,B), 20× magnification, (C,D), 50× magnification. Panels (A,C) show representative examples for an accession (PI524469) with low stomatal density (73 stomata mm–2) and large stomata (964 μm2), and (B,D) for an accession (PI329719) with high stomatal density (125 stomata mm–2) and small stomata (734 μm2).
Correlations Between A, gs, iWUE, and Stomatal Patterning Traits
Traits Correlated With Steady-State A, gs, and iWUE
Steady-state gs 2000 was positively correlated with A2000 (p < 0.001, R2 = 0.87, Figure 6) and negatively correlated with iWUE2000 (p = 0.0019, R2 = 0.46, Figure 6). After a decrease in PPFD, iWUE increased toward steady-state more slowly in accessions with greater A2000 and gs 2000 (positive correlation of A2000 with t95 iWUE, p = 0.094, R2 = 0.17; positive correlation of gs 2000 with t95 iWUE, p = 0.059, R2 = 0.2, Figure 6). After a decrease in PPFD, undershoot of steady-state iWUE was more pronounced in accessions with greater A2000 and gs 2000 (negative correlation of A2000 with iWUEundershoot, p = 0.03, R2 = 0.26; negative correlation of gs 2000 with iWUEundershoot, p = 0.074, R2 = 0.19, Figure 6). Accordingly, deviation of iWUE from steady-state following decreases in PPFD was more negative in accessions with greater A2000 (p < 0.001, R2 = 0.61, Figure 6) and greater gs 2000 (p < 0.001, R2 = 0.52, Figure 6).
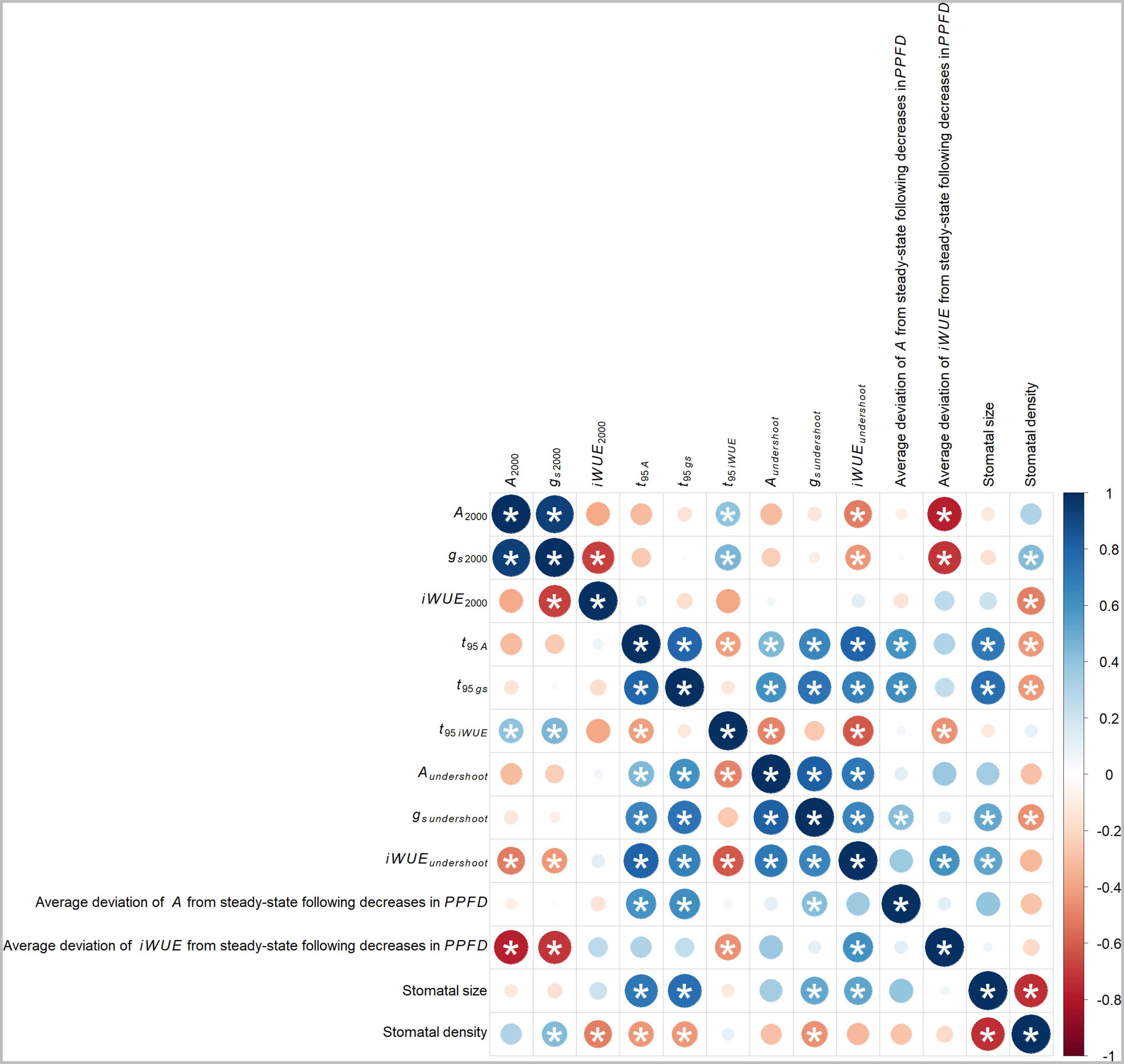
Figure 6. Pearson’s correlation coefficients (r) for traits potentially underlying variation in non-steady-state iWUE following decreases in PPFD. The size of circles gives the strength of correlation and the color gives the direction of correlation: red (negative) and blue (positive). Significance (p < 0.1 threshold) is marked by *. The corresponding pairwise correlation scatterplots are given in Supplementary Figure 8.
Traits Correlated With Non-Steady-State A
For non-steady-state A to be maximized following a decrease in PPFD, A should slowly approach steady-state (i.e., high t95 A) with minimal undershoot (i.e., greater, i.e., less negative, Aundershoot). This non-steady-state A may also be related to non-steady-state gs. Accordingly, accessions with a more positive deviation of A from steady-state following decreases in PPFD, also had greater t95 A (p = 0.0088, R2 = 0.36, Figure 6), greater t95 gs (p = 0.0062, R2 = 0.38, Figure 6), and less negative gs undershoot (p = 0.078, R2 = 0.18, Figure 6). In accessions in which A and gs approached steady-state more slowly (i.e., greater t95 A and t95 gs), the undershoot of steady-state A and gs was less pronounced (i.e., less negative Aundershoot and gs undershoot). This was evidenced by the positive correlation of t95 A with Aundershoot (p = 0.063, R2 = 0.2, Figure 6) and gs undershoot (p = 0.0033, R2 = 0.43, Figure 6) and the positive correlation of t95 gs with Aundershoot (p = 0.0081, R2 = 0.36, Figure 6) and gs undershoot (p < 0.001, R2 = 0.54, Figure 6).
Non-steady-state A and gs were coordinated following a decrease in PPFD. In accessions in which A approached steady-state more slowly (i.e., greater t95 A) with a less pronounced undershoot (i.e., less negative Aundershoot), the same was also seen for gs (i.e., greater t95 gs and less negative gs undershoot). This was evidenced by the positive correlation of t95 A with t95 gs (p < 0.001, R2 = 0.63, Figure 6) and the positive correlation of Aundershoot with gs undershoot (p < 0.001, R2 = 0.66, Figure 6).
Traits Correlated With Non-Steady-State iWUE
For non-steady-state iWUE to be maximized following a decrease in PPFD, iWUE should rapidly approach steady-state (i.e., small t95iWUE) with minimal undershoot (i.e., greater, i.e., less negative iWUEundershoot). Accordingly, accessions with a less negative deviation of iWUE from steady-state following decreases in PPFD also had smaller t95iWUE (p = 0.051, R2 = 0.21, Figure 6), and greater iWUEundershoot (p = 0.0074, R2 = 0.37, Figure 6).
Non-steady-state iWUE traits were associated with A rather than gs. Specifically, in accessions in which iWUE approached steady-state more slowly (i.e., greater t95iWUE), A approached steady-state more rapidly with a more pronounced undershoot (i.e., smaller t95 A and more negative Aundershoot). This was evidenced by the negative correlation of t95iWUE with t95 A (p = 0.085, R2 = 0.17, Figure 6) and Aundershoot (p = 0.035, R2 = 0.25, Figure 6). Additionally, accessions which displayed a less pronounced undershoot of steady-state by iWUE following decreases in PPFD (i.e., greater, i.e., less negative iWUEundershoot) also had a slower decrease of A and gs to reach steady-state (positive correlation of iWUEundershoot with t95 A, p < 0.001, R2 = 0.65; positive correlation of iWUEundershoot with t95 gs, p = 0.0021, R2 = 0.46, Figure 6). Further, in accessions which displayed a less pronounced undershoot of steady-state by iWUE, the same was seen for A and gs (positive correlation of iWUEundershoot with Aundershoot, p < 0.001, R2 = 0.51; positive correlation of iWUEundershoot with gs undershoot, p = 0.0034, R2 = 0.42, Figure 6). Finally, accessions in which iWUE approached steady-state more slowly (i.e., greater t95 iWUE) had a more pronounced undershoot of steady-state iWUE (negative correlation of t95 iWUE with iWUEundershoot, p = 0.0068, R2 = 0.38, Figure 6).
These surprising findings are exemplified in accessions PI153852 and PI152636 (Figure 1). PI152636 displayed faster declines in gs after each decrease in PPFD (i.e., smaller t95 gs, Figure 1D), which theoretically would lead to increased iWUE, when compared to the slower gs response of PI153852 (Figure 1C). However, in PI153852 the slower response of gs was also associated with slower, more gradual declines in A after each decrease in PPFD (i.e., greater t95 A, Figure 1A), which would also theoretically lead to increased iWUE, when compared to the faster A response of PI152636 (Figure 1B). The net result in terms of iWUE yielded a benefit to PI153852 (Figure 1E), with a less pronounced undershoot of steady-state iWUE (i.e., less negative iWUEundershoot) and a faster return to steady-state iWUE (i.e., smaller t95 iWUE) when compared to PI152636 (Figure 1F). In other words, when comparing PI153852, with slow responses of A and gs to decreases in PPFD, to PI152636, with fast responses of A and gs to decreases in PPFD, PI153852 showed the greatest iWUE at non-steady-state.
Correlations With Stomatal Density and Size
Stomatal density and size were negatively correlated (p < 0.001, R2 = 0.54, Figure 6). Accessions with more numerous, smaller stomata had greater steady-state gs and more rapid responses of A and gs to decreases in PPFD. Specifically, accessions with greater stomatal density had greater gs 2000 (p = 0.074, R2 = 0.19, Figure 6), lower iWUE2000 (p = 0.032, R2 = 0.26, Figure 6), smaller t95 A (p = 0.073, R2 = 0.19, Figure 6), smaller t95 gs (p = 0.069, R2 = 0.19, Figure 6), and more negative gs undershoot (p = 0.059, R2 = 0.21, Figure 6). Accessions with smaller stomata had smaller t95 A (p < 0.001, R2 = 0.51, Figure 6), smaller t95 gs (p < 0.001, R2 = 0.56, Figure 6), more negative gs undershoot (p = 0.029, R2 = 0.26, Figure 6), and more negative iWUEundershoot (p = 0.024, R2 = 0.28, Figure 6).
Overall A and iWUE Under Fluctuating PPFD: Contributions of Steady-State and Non-Steady-State Gas-Exchange
Overall gas-exchange under fluctuating PPFD could be described as the sum of steady-state gas-exchange and the deviation of gas-exchange from steady-state following each PPFD fluctuation. An additional correlation analysis was used to explore whether steady-state and non-steady-state gas-exchange were significantly associated with overall gas-exchange under fluctuating PPFD. Average A and iWUE under fluctuating PPFD were tested for correlation with steady-state A2000 and iWUE2000, and with the deviation of A and iWUE from steady-state following decreases and increases in PPFD. The majority of variation in average A under fluctuating PPFD was associated with variation in steady-state A2000 (p < 0.001, R2 = 0.88, Figure 7A) but not with deviation of A from steady-state following decreases (p = 0.41, Figure 7B) or increases (p = 0.58, Figure 7C) in PPFD. The majority of variation in average iWUE under fluctuating PPFD was associated with variation in steady-state iWUE2000 (p < 0.001, R2 = 0.61, Figure 7D) and there was also substantial variation associated with deviation of iWUE from steady-state following decreases (p = 0.012, R2 = 0.33, Figure 7E), but not increases (p = 0.4, Figure 7F), in PPFD. This suggests a potentially important role for non-steady-state iWUE following decreases in PPFD, causing a substantial loss of iWUE under fluctuating PPFD.
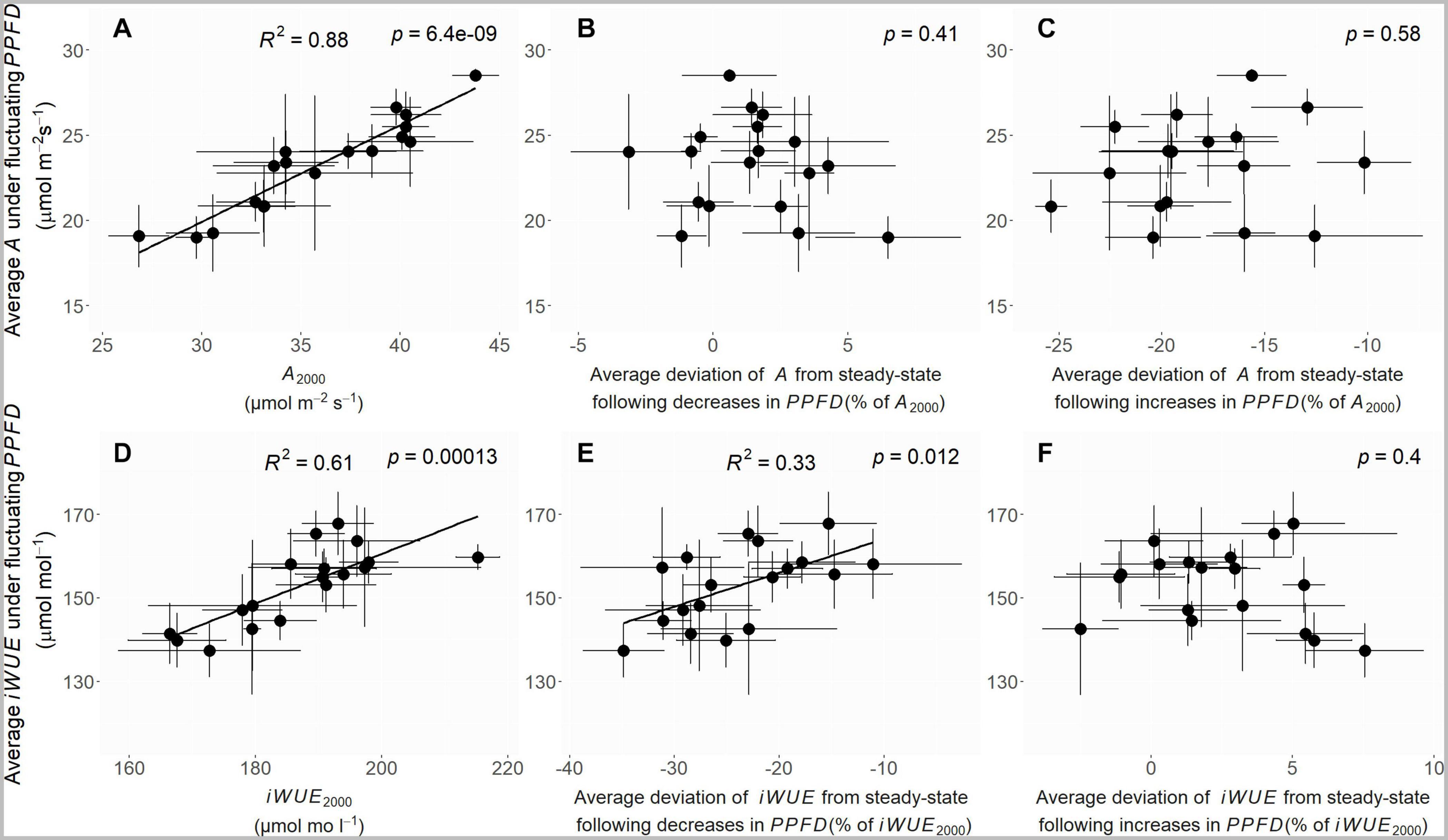
Figure 7. Correlation plots of average A under fluctuating PPFD against: (A) A2000, (B) the average deviation of A from steady-state following decreases in PPFD, expressed as a% of A2000, (C) the average deviation of A from steady-state following increases in PPFD, expressed as a% of A2000; and correlation plots of average iWUE under fluctuating PPFD against: (D) iWUE2000, (E) the average deviation of iWUE from steady-state following decreases in PPFD, expressed as a% of iWUE2000, (F) the average deviation of iWUE from steady-state following increases in PPFD, expressed as a% of iWUE2000.
Discussion
Improving iWUE under fluctuating PPFD is important to sustain or further increase crop yields (Leakey et al., 2019). This study shows significant variation among sorghum accessions in steady-state and non-steady-state A and gs and stomatal density and size, reveals how variation in these traits drives variation in iWUE, and discusses how tradeoffs between these should shape strategies for decreasing crop water use.
Decreases in PPFD Substantially Impair iWUE Under Fluctuating PPFD, With Loss of Non-Steady-State iWUE Associated With A Rather Than gs
Almost all variation in average A under fluctuating PPFD was associated with steady-state A2000 (Figures 7A–C). In contrast, much of the variation in average iWUE under fluctuating PPFD was associated with the non-steady-state loss of iWUE following decreases in PPFD in addition to steady-state iWUE2000 (Figures 7D–F). This points to non-steady-state iWUE as an important contributor to overall iWUE under fluctuating PPFD, with decreases in PPFD being more impactful than increases in PPFD. This could in large part be attributed to the undershoot of steady-state by iWUE following decreases in PPFD (i.e., iWUEundershoot), which showed significant variation among accessions and so could be a promising target for improvement.
The response of iWUE to a decrease in PPFD was biphasic, with an abrupt loss of iWUE driven by A, followed by a gradual return to steady-state driven by gs (Figure 1). Loss of iWUE was mitigated in accessions in which the undershoot of steady-state iWUE was less pronounced (i.e., greater, i.e., less negative, iWUEundershoot) and iWUE returned to steady-state more rapidly (i.e., smaller t95 iWUE, Figure 6). The finding that t95 iWUE and iWUEundershoot were associated with corresponding traits of A rather than gs is novel, and suggests that breeding for improved non-steady-state A traits, i.e., high t95 A and less negative Aundershoot, could mitigate loss of iWUE under fluctuating PPFD (Figure 6).
Non-steady-state A following decreases in PPFD is influenced by different physiological processes. Undershoot of steady-state by A following decreases in PPFD may be attributed to kinetics of protective energy-dissipating mechanisms, collectively termed non-photochemical quenching, and photorespiration (Kaiser et al., 2015, 2018), and has been observed in C3 dicots such as Arabidopsis, French bean (McAusland et al., 2016) and tobacco (Kromdijk et al., 2016). This could explain the rapid and pronounced undershoot of steady-state A following decreases in PPFD in accessions such as PI329656 (Supplementary Figure 2G). Large pools of metabolites involved in C4 photosynthesis could buffer energy supply and sustain a higher A for some time following a decrease in PPFD (Stitt and Zhu, 2014). For instance, a large pool of active malate carries enough reductive power to reduce CO2 for several seconds after a light to dark transition (Slattery et al., 2018). C4 activity could be insufficient to achieve CO2 saturation of Rubisco and eliminate photorespiration at low PPFD, leading to reduced steady-state A (Kromdijk et al., 2010). If so, a leaf with CO2-saturated Rubisco at high PPFD could maintain CO2-saturation for some time after a decrease in PPFD, effectively maintaining photorespiration below its steady-state and therefore boosting A. This could explain the relatively gradual decline of A following decreases in PPFD in accessions such as PI153852 (Figure 1A), enabling it to maintain A above steady-state for several minutes following decreases in PPFD (Figure 3C). Here, we show that potential for improvement of non-steady-state A in sorghum through breeding is supported by significant variation in t95 A and Aundershoot, which may result from variation in the processes above (Figure 2). This highlights the value of the methodology used in the present study to assess steady-state and non-steady-state gas-exchange traits, and demonstrates that sorghum is a relevant crop species to study diversity in these traits.
Interactions Between Non-Steady-State A and gs Impair iWUE Following Decreases in PPFD: This Could Be Resolved in Leaves With Smaller, More Sensitive Stomata
Many efforts to improve iWUE under fluctuating light have focused on faster stomatal closure (Lawson and Blatt, 2014; Bellasio et al., 2017; Lawson and Vialet-Chabrand, 2019). In a simplified model of leaf gas-exchange following a decrease in PPFD, where A has an instant step-change from one steady-state to the next, a faster decrease in gs would directly lead to a faster increase in iWUE, leading to overall improvement in iWUE under fluctuating PPFD. In the sorghum accessions studied here, this process was complicated by interactions between gs and A, which may reflect precise stomatal sensing of A (reviewed: Lawson and Matthews, 2020). In other words, accessions with rapid decreases in gs also had rapid decreases in A, negating much of the benefit to iWUE (Figures 1, 6). In fact, the undershoot of steady-state iWUE following decreases in PPFD (i.e., iWUEundershoot) was most negative in accessions with faster stomatal responses (i.e., smaller t95 gs, Figure 6). If the same coordination between non-steady state A and gs applies across species, this could explain the observation that faster stomatal closing speed did not translate to improved water saving across diverse plant species (Deans et al., 2019).
Because of this tradeoff, fast gs response to decreasing PPFD may be a difficult target for improvement of iWUE through breeding in sorghum, though it may be possible to bypass this tradeoff through transgenic means. The optimal leaf response following decreases in PPFD would be a slow decline in A with minimal undershoot of steady-state, paired with a rapid decline in gs. This might be achieved in leaves with enhanced stomatal sensitivity to A, in which even a slow decline in A following a decrease in PPFD could trigger a rapid stomatal response. In particular, stomatal aperture responds to light via two separate pathways: the photosynthesis-independent and guard-cell specific blue light pathway, and the photosynthesis-dependent red light pathway. The latter is thought to be the main mechanism coordinating stomatal behavior with photosynthesis (Matthews et al., 2020). Therefore, manipulation of components involved in red light sensing, such as the redox state of the chloroplastic plastoquinone pool (Głowacka et al., 2018), could be a good target for manipulation to increase stomatal sensitivity to changes in A and improve coordination of A and gs.
Another factor influencing the speed of change in gs is stomatal size, with smaller stomata generally showing faster movement, possibly due to greater guard cell membrane surface area to volume ratio (Drake et al., 2013; Raven, 2014; Lawson and Vialet-Chabrand, 2019; Lawson and Matthews, 2020). Therefore, leaves with smaller stomata might allow greater sensitivity of gs to A by enabling mechanically faster stomatal closure. However, stomatal size is usually negatively correlated with stomatal density (Hetherington and Woodward, 2003). Here, stomatal density and size were negatively correlated (Figure 6), and leaves with more numerous and smaller stomata had faster t95 gs but also had reduced iWUE2000, more negative iWUEundershoot, and increased gs 2000 (Figures 5, 6). This points to a tradeoff between leaves with more numerous, smaller stomata which show high steady-state gs but rapid gs responses to PPFD, and leaves with fewer, larger stomata which show low steady-state gs but slow gs responses to PPFD. The finding that steady-state iWUE2000 was mainly associated with gs 2000 rather than A2000 is consistent with prior observations in sorghum (Geetika et al., 2019) and other C4 grasses (Leakey et al., 2019), but the tradeoff with non-steady-state gs identified here (Figure 6) is not widely recognized.
Sorghum leaves with fewer and smaller stomata might achieve the best of both worlds with low steady-state gs but rapid stomatal responses to PPFD. In C4 crops such as sorghum, where photosynthesis is typically CO2-saturated even under sub-ambient conditions, modestly reducing gs at steady-state may not impair A, compounding benefits to iWUE (Leakey et al., 2019; Pignon and Long, 2020). Further, at a given SD, C4 grass stomata are smaller than those of related C3 grasses (Taylor et al., 2012). Transgenic approaches may hold potential to break the relationship between stomatal density and size (reviewed: Leakey et al., 2019).
Increases in PPFD were much less disruptive to iWUE than decreases in PPFD (Figure 3). The fact that iWUE was slightly above steady-state following increases in PPFD suggests that increase in A was faster than gs: this is in agreement with findings from a C4 stomata model (Bellasio et al., 2017) applied to photosynthesis induction data in maize (Chen et al., 2013). By comparison, in many other C3 and C4 dicots and monocots, the return of iWUE to steady-state following an increase in PPFD was much slower (e.g., >30 min), reflecting a pronounced desynchronization between A and gs (McAusland et al., 2016). In our study, the response of gs to an increase in PPFD occurred within seconds, whereas in C3 species there may be a lag of up to several minutes before stomata begin to open (Lawson and Blatt, 2014). Together these findings suggest exceptional coordination of A and gs following increases in PPFD in sorghum.
Relative to Other Species, iWUE in Sorghum Is High Both at Steady-State and Non-Steady-State
The range of natural variation among sorghum accessions tested here in iWUE2000 of 166–215 μmol mol–1 (Figure 2) is similar to published variation in sorghum RILs (100–140 μmol mol–1; Kapanigowda et al., 2014) and accessions (143–176 μmol mol–1; Xin et al., 2009), but less variable compared to measurements in closely related NADP-ME C4 grasses such as maize (80–140 μmol mol–1; Yabiku and Ueno, 2017), sugarcane (100–180 μmol mol–1; Viswanathan et al., 2014) and elephant grass (100–160 μmol mol–1; Sollenberger et al., 2014), whereas the widest range of variation tends to be found in C3 species such as soybean (40–115 μmol mol–1; Tomeo and Rosenthal, 2017), wheat (25–65 μmol mol–1; Jahan et al., 2014), and rice (50–80 μmol mol–1; Giuliani et al., 2013).
For both steady-state and non-steady-state traits, variation in iWUE was narrower than for A and gs (Figures 2, 4). This resulted from coordination in A and gs, e.g., accessions with high A2000 also had high gs 2000 and accessions with high t95 A also had high t95 gs (Figure 6). The accessions studied here showed faster gs responses to changes in PPFD and higher iWUE compared to diverse gymnosperms and C3 dicots (Deans et al., 2019), C3 monocot crops such as wheat and rice, and even closely related C4 monocots such as maize and Miscanthus (Chen et al., 2013; McAusland et al., 2016). Understanding how sorghum maintains coordination between A and gs to sustain high iWUE may be valuable to design strategies for improvement in species where coordination is less tight.
In our study, gs declined over the course of the fluctuating PPFD timecourse, suggesting that stomatal opening during increases in PPFD was slower than stomatal closing during decreases in PPFD (Figure 3). Faster stomatal closure than opening may be a consequence of sorghum’s adaptation to dry, high-light environments, where water is more limiting than light and rapid stomatal closing can maximize iWUE (Vico et al., 2011; McAusland et al., 2016). On the contrary, species adapted to shaded environments such as a forest understory, where light is more limiting than water, typically show faster stomatal opening than closing, which can maximize A with little penalty to iWUE: these patterns suggest that dynamic stomatal traits are driven by ecological adaptation rather than evolutionary lineage (Deans et al., 2019). Increased steady-state iWUE of C4 photosynthesis may have been a driver for evolution of this pathway (Osborne and Sack, 2012). The fact that C4 grasses such as sorghum display fast stomatal responses to fluctuating light (Grantz and Assmann, 1991; Knapp, 1993; McAusland et al., 2016) may be an additional evolved mechanism to improve non-steady-state iWUE.
While a drought treatment was not included, we show genetic variation that may be exploited to reduce crop water demand and avoid drought (Leakey et al., 2019). An important next step will be to determine whether the trait correlations identified here are also observed in water-limited plants. Plants that develop under water-limited conditions can produce fewer leaves with fewer and/or smaller stomata to mitigate water loss. In these smaller plants, reduced canopy density may limit the prevalence of light fluctuations and alter the microenvironment including VPD and temperature. However, leaves that develop with sufficient water supply but are water-limited afterward have fewer options to acclimate. With stomatal density and size already fixed during development, stomatal closure is the main mechanism available to reduce steady-state gs. Leaves that permanently operate in a reduced range of stomatal apertures could have an altered relationship between steady-state traits (e.g., gs 2000) and non-steady-state traits (e.g., t95 gs, gs undershoot). This could also affect the relative association of A and gs traits with iWUE: at low apertures, stomatal control may be less precise (Kaiser and Kappen, 2001) and so more wasteful in terms of water loss. An important next step will be to determine the degree of plasticity in steady-state and non-steady-state A, gs and iWUE traits under different environments.
Conclusion
In this study, we show that a common measurement, the steady-state PPFD response curve, can be used to derive valuable insight into both steady-state and non-steady-state A, gs and iWUE (Figures 1, 2). The relevance of these non-steady-state traits could be seen when leaves were exposed to a fluctuating PPFD regime, as natural diversity in traits such as t95 A, Aundershoot, and iWUEundershoot correlated with the deviation of A and iWUE from steady-state under fluctuating PPFD (Figures 3, 4, 6). Remarkably, the deviation of A and iWUE from steady-state under fluctuating PPFD was substantial even under the relatively lengthy PPFD fluctuations, spaced 5.5 min apart, of the fluctuating PPFD response curves used here (Figure 7). In a crop canopy, where most light fluctuations are more rapid (<5 s) (Kaiser et al., 2018), the non-steady-state processes quantified here, especially the photosynthetic traits t95 A and Aundershoot, would likely be even more important in driving overall iWUE. Variation among accessions in steady-state and non-steady-state traits may be exploited to reduce crop water demand and avoid drought, but our results emphasize that translating this into breeding strategies will require careful consideration of emerging tradeoffs due to co-variation between traits.
Data Availability Statement
The original contributions presented in the study are included in the article/Supplementary Material, further inquiries can be directed to the corresponding author/s.
Author Contributions
CP designed and performed the research, data collection, analysis and interpretation, and wrote the manuscript. AL, SL, and JK performed the data analysis and interpretation and wrote the manuscript. All authors contributed to the article and approved the submitted version.
Funding
The information, data, or work presented herein was funded in part by the Advanced Research Projects Agency-Energy (ARPA-E), United States Department of Energy, under Award Number DE-DE-AR0000661. The views and opinions of authors expressed herein do not necessarily state or reflect those of the United States Government or any agency thereof.
Conflict of Interest
The authors declare that the research was conducted in the absence of any commercial or financial relationships that could be construed as a potential conflict of interest.
Acknowledgments
The authors thank Dr. Patrick J. Brown for developing the diversity panel.
Supplementary Material
The Supplementary Material for this article can be found online at: https://www.frontiersin.org/articles/10.3389/fpls.2021.627432/full#supplementary-material
Supplementary Figure 1 | Steady-state PPFD response curves for A, gs, and iWUE in all accessions.
Supplementary Figure 2 | Timecourses of steady-state A-PPFD response curves in all accessions.
Supplementary Figure 3 | Timecourses of steady-state gs-PPFD response curves in all accessions.
Supplementary Figure 4 | Timecourses of steady-state iWUE-PPFD response curves in all accessions.
Supplementary Figure 5 | Timecourses of fluctuating PPFD response curves for A in all accessions.
Supplementary Figure 6 | Timecourses of fluctuating PPFD response curves for gs in all accessions.
Supplementary Figure 7 | Timecourses of fluctuating PPFD response curves for iWUE in all accessions.
Supplementary Figure 8 | Pearson’s correlation coefficients, pairwise correlation scatterplots, and density plots for traits potentially underlying variation in non-steady-state iWUE following decreases in PPFD.
Supplementary Figure 9 | Test of the effect of leaf excision on steady-state and non-steady-state leaf gas-exchange.
Supplementary Table 1 | Variation in environmental controls during gas-exchange measurements.
References
Acevedo-Siaca, L. G., Coe, R., Wang, Y., Kromdijk, J., Quick, W. P., and Long, S. P. (2020). Variation in photosynthetic induction between rice accessions and its potential for improving productivity. New Phytol. 227, 1097–1108. doi: 10.1111/nph.16454
Bellasio, C., Quirk, J., Buckley, T. N., and Beerling, D. J. (2017). A dynamic hydro-mechanical and biochemical model of stomatal conductance for C4 photosynthesis. Plant Physiol. 175, 104–119. doi: 10.1104/pp.17.00666
Bonsch, M., Humpenoder, F., Popp, A., Bodirsky, B., Dietrich, J. P., Rolinski, S., et al. (2016). Trade-offs between land and water requirements for large-scale bioenergy production. Glob. Change Biol. Bioener. 8, 11–24. doi: 10.1111/gcbb.12226
Boyer, J. S. (1982). Plant productivity and environment. Science 218, 443–448. doi: 10.1126/science.218.4571.443
Chen, J. W., Yang, Z. Q., Zhou, P., Hai, M. R., Tang, T. X., Liang, Y. L., et al. (2013). Biomass accumulation and partitioning, photosynthesis, and photosynthetic induction in field-grown maize (Zea mays L.) under low- and high-nitrogen conditions. Acta Physiol. Plant. 35, 95–105. doi: 10.1007/s11738-012-1051-6
Dai, A. G. (2013). Increasing drought under global warming in observations and models. Nat. Clim. Change 3, 52–58. doi: 10.1038/nclimate1633
Dalin, C., Wada, Y., Kastner, T., and Puma, M. J. (2017). Groundwater depletion embedded in international food trade. Nature 543, 700–704. doi: 10.1038/nature21403
De Souza, A. P., Wang, Y., Orr, D. J., Carmo-Silva, E., and Long, S. P. (2020). Photosynthesis across African cassava germplasm is limited by Rubisco and mesophyll conductance at steady state, but by stomatal conductance in fluctuating light. New Phytol. 225, 2498–2512. doi: 10.1111/nph.16142
Deans, R. M., Brodribb, T. J., Busch, F. A., and Farquhar, G. D. (2019). Plant water-use strategy mediates stomatal effects on the light induction of photosynthesis. New Phytol. 222, 382–395. doi: 10.1111/nph.15572
D’Odorico, P., Chiarelli, D. D., Rosa, L., Bini, A., Zilberman, D., and Rulli, M. C. (2020). The global value of water in agriculture. Proc. Natl. Acad. Sci. U.S.A. 117, 21985–21993.
Doncaster, H. D., Adcock, M. D., and Leegood, R. C. (1989). Regulation of photosynthesis in leaves of C4 plants following a transition from high to low light. Biochim. Biophys. Acta 973, 176–184. doi: 10.1016/s0005-2728(89)80419-0
Drake, P. L., Froend, R. H., and Franks, P. J. (2013). Smaller, faster stomata: scaling of stomatal size, rate of response, and stomatal conductance. J. Exp. Bot. 64, 495–505. doi: 10.1093/jxb/ers347
Driever, S. M., Lawson, T., Andralojc, P. J., Raines, C. A., and Parry, M. A. J. (2014). Natural variation in photosynthetic capacity, growth, and yield in 64 field-grown wheat genotypes. J. Exp. Bot. 65, 4959–4973. doi: 10.1093/jxb/eru253
FAO, IFAD, UNICEF, WFP, and WHO. (2018). The State of Food Security and Nutrition in the World 2018. Building Climate Resilience for Food Security and Nutrition. Rome: FAO, 202.
Flexas, J. (2016). Genetic improvement of leaf photosynthesis and intrinsic water use efficiency in C3 plants: why so much little success? Plant Sci. 251, 155–161. doi: 10.1016/j.plantsci.2016.05.002
Galmes, J., Medrano, H., and Flexas, J. (2007). Photosynthetic limitations in response to water stress and recovery in Mediterranean plants with different growth forms. New Phytol. 175, 81–93. doi: 10.1111/j.1469-8137.2007.02087.x
Geetika, G., van Oosterom, E. J., George-Jaeggli, B., Mortlock, M. Y., Deifel, K. S., McLean, G., et al. (2019). Genotypic variation in whole-plant transpiration efficiency in sorghum only partly aligns with variation in stomatal conductance. Funct. Plant Biol. 46, 1072–1089. doi: 10.1071/fp18177
Giuliani, R., Koteyeva, N., Voznesenskaya, E., Evans, M. A., Cousins, A. B., and Edwards, G. E. (2013). Coordination of leaf photosynthesis, transpiration, and structural traits in rice and wild relatives (Genus Oryza). Plant Physiol. 162, 1632–1651. doi: 10.1104/pp.113.217497
Głowacka, K., Kromdijk, J., Kucera, K., Xie, J., Cavanagh, A. P., Leonelli, L., et al. (2018). Photosystem II Subunit S overexpression increases the efficiency of water use in a field-grown crop. Nat. Commun. 9:868.
Grantz, D. A., and Assmann, S. M. (1991). Stomatal response to blue light: water-use efficiency in sugarcane and soybean. Plant Cell Environ. 14, 683–690. doi: 10.1111/j.1365-3040.1991.tb01541.x
Gu, J. F., Zhou, Z. X., Li, Z. K., Chen, Y., Wang, Z. Q., and Zhang, H. (2017). Rice (Oryza sativa L.) with reduced chlorophyll content exhibit higher photosynthetic rate and efficiency, improved canopy light distribution, and greater yields than normally pigmented plants. Field Crops Res. 200, 58–70. doi: 10.1016/j.fcr.2016.10.008
Hadebe, S. T., Modi, A. T., and Mabhaudhi, T. (2017). Drought tolerance and water use of cereal crops: a focus on sorghum as a food security crop in sub-Saharan Africa. J. Agron. Crop Sci. 203, 177–191. doi: 10.1111/jac.12191
Hetherington, A. M., and Woodward, F. I. (2003). The role of stomata in sensing and driving environmental change. Nature 424, 901–908. doi: 10.1038/nature01843
Hubbart, S., Smillie, I. R. A., Heatley, M., Swarup, R., Foo, C. C., Zhao, L., et al. (2018). Enhanced thylakoid photoprotection can increase yield and canopy radiation use efficiency in rice. Commun. Biol. 1:22.
Jahan, E., Amthor, J. S., Farquhar, G. D., Trethowan, R., and Barbour, M. M. (2014). Variation in mesophyll conductance among Australian wheat genotypes. Funct. Plant Biol. 41, 568–580. doi: 10.1071/fp13254
Kaiser, E., Morales, A., and Harbinson, J. (2018). Fluctuating light takes crop photosynthesis on a rollercoaster ride. Plant Physiol. 176, 977–989. doi: 10.1104/pp.17.01250
Kaiser, E., Morales, A., Harbinson, J., Kromdijk, J., Heuvelink, E., and Marcelis, L. F. M. (2015). Dynamic photosynthesis in different environmental conditions. J. Exp. Bot. 66, 2415–2426. doi: 10.1093/jxb/eru406
Kaiser, H., and Kappen, L. (2001). Stomatal oscillations at small apertures: indications for a fundamental insufficiency of stomatal feedback-control inherent in the stomatal turgor mechanism. J. Exp. Bot. 52, 1303–1313. doi: 10.1093/jexbot/52.359.1303
Kapanigowda, M. H., Payne, W. A., Rooney, W. L., Mullet, J. E., and Balota, M. (2014). Quantitative trait locus mapping of the transpiration ratio related to preflowering drought tolerance in sorghum (Sorghum bicolor). Funct. Plant Biol. 41, 1049–1065. doi: 10.1071/fp13363
Knapp, A. K. (1993). Gas-exchange dynamics in C3 and C4 grasses - consequences of differences in stomatal conductance. Ecology 74, 113–123. doi: 10.2307/1939506
Kromdijk, J., Glowacka, K., Leonelli, L., Gabilly, S. T., Iwai, M., Niyogi, K. K., et al. (2016). Improving photosynthesis and crop productivity by accelerating recovery from photoprotection. Science 354, 857–861. doi: 10.1126/science.aai8878
Kromdijk, J., Griffiths, H., and Schepers, H. E. (2010). Can the progressive increase of C4 bundle sheath leakiness at low PFD be explained by incomplete suppression of photorespiration? Plant Cell Environ. 33, 1935–1948. doi: 10.1111/j.1365-3040.2010.02196.x
Lawson, T., and Blatt, M. R. (2014). Stomatal size, speed, and responsiveness impact on photosynthesis and water use efficiency. Plant Physiol. 164, 1556–1570. doi: 10.1104/pp.114.237107
Lawson, T., and Matthews, J. (2020). Guard cell metabolism and stomatal function. Ann. Rev. Plant Biol. 71, 273–302. doi: 10.1146/annurev-arplant-050718-100251
Lawson, T., and Vialet-Chabrand, S. (2019). Speedy stomata, photosynthesis and plant water use efficiency. New Phytol. 221, 93–98. doi: 10.1111/nph.15330
Lawson, T., von Caemmerer, S., and Baroli, I. (2011). “Photosynthesis and stomatal behaviour,” in Progress in Botany 72, Vol. 72, eds U. Luttge, W. Beyschlag, B. Budel, and D. Francis (Berlin: Springer-Verlag), 265–304. doi: 10.1007/978-3-642-13145-5_11
Leakey, A. D. B., Ferguson, J. N., Pignon, C. P., Wu, A., Jin, Z., Hammer, G. L., et al. (2019). Water use efficiency as a constraint and target for improving the resilience and productivity of C3 and C4 crops. Ann. Rev. Plant Biol. 70, 781–808. doi: 10.1146/annurev-arplant-042817-040305
Leakey, A. D. B., Press, M. C., and Scholes, J. D. (2003). High-temperature inhibition of photosynthesis is greater under sunflecks than uniform irradiance in a tropical rain forest tree seedling. Plant Cell Environ. 26, 1681–1690. doi: 10.1046/j.1365-3040.2003.01086.x
Leakey, A. D. B., Press, M. C., Scholes, J. D., and Watling, J. R. (2002). Relative enhancement of photosynthesis and growth at elevated CO2 is greater under sunflecks than uniform irradiance in a tropical rain forest tree seedling. Plant Cell Environ. 25, 1701–1714. doi: 10.1046/j.1365-3040.2002.00944.x
Lobell, D. B., Roberts, M. J., Schlenker, W., Braun, N., Little, B. B., Rejesus, R. M., et al. (2014). Greater sensitivity to drought accompanies maize yield increase in the US midwest. Science 344, 516–519. doi: 10.1126/science.1251423
Matthews, J. S. A., Vialet-Chabrand, S., and Lawson, T. (2020). Role of blue and red light in stomatal dynamic behaviour. J. Exp. Bot. 71, 2253–2269. doi: 10.1093/jxb/erz563
McAusland, L., Vialet-Chabrand, S., Davey, P., Baker, N. R., Brendel, O., and Lawson, T. (2016). Effects of kinetics of light-induced stomatal responses on photosynthesis and water-use efficiency. New Phytol. 211, 1209–1220. doi: 10.1111/nph.14000
Osborne, C. P., and Sack, L. (2012). Evolution of C4 plants: a new hypothesis for an interaction of CO2 and water relations mediated by plant hydraulics. Philos. Transact. R. Soc. B Biol. Sci. 367, 583–600. doi: 10.1098/rstb.2011.0261
Papanatsiou, M., Petersen, J., Henderson, L., Wang, Y., Christie, J. M., and Blatt, M. R. (2019). Optogenetic manipulation of stomatal kinetics improves carbon assimilation, water use, and growth. Science 363, 1456–1459. doi: 10.1126/science.aaw0046
Pearcy, R. W. (1990). Sunflecks and photosynthesis in plant canopies. Ann. Rev. Plant Physiol. Plant Mol. Biol. 41, 421–453. doi: 10.1146/annurev.pp.41.060190.002225
Pignon, C. P. (2017). Strategies to Improve C4 Photosynthesis, Water and Resource-Use Efficiency Under Different Atmospheres, Temperatures, and Light Environments. Urbana, IL: University of Illinois at Urbana-Champaign.
Pignon, C. P., and Long, S. P. (2020). Retrospective analysis of biochemical limitations to photosynthesis in 49 species: C4 crops appear still adapted to pre-industrial atmospheric [CO2]. Plant Cell Environ. 43, 2606–2622. doi: 10.1111/pce.13863
R Core Team (2017). R: A Language and Environment for Statistical Computing. Vienna: R Foundation for Statistical Computing.
Regassa, T. H., and Wortmann, C. S. (2014). Sweet sorghum as a bioenergy crop: literature review. Biomass Bioenergy 64, 348–355. doi: 10.1016/j.biombioe.2014.03.052
Sinclair, T. R., Tanner, C. B., and Bennett, J. M. (1984). Water-use efficiency in crop production. Bioscience 34, 36–40.
Slattery, R. A., Walker, B. J., Weber, A. P. M., and Ort, D. R. (2018). The Impacts of fluctuating light on crop performance. Plant Physiol. 176, 990–1003. doi: 10.1104/pp.17.01234
Soleh, M. A., Tanaka, Y., Kim, S. Y., Huber, S. C., Sakoda, K., and Shiraiwa, T. (2017). Identification of large variation in the photosynthetic induction response among 37 soybean Glycine max (L.) Merr. genotypes that is not correlated with steady-state photosynthetic capacity. Photosynth. Res. 131, 305–315. doi: 10.1007/s11120-016-0323-1
Soleh, M. A., Tanaka, Y., Nomoto, Y., Iwahashi, Y., Nakashima, K., Fukuda, Y., et al. (2016). Factors underlying genotypic differences in the induction of photosynthesis in soybean Glycine max (L.) Merr. Plant Cell Environ. 39, 685–693. doi: 10.1111/pce.12674
Sollenberger, L. E., Woodard, K. R., Vendramini, J. M. B., Erickson, J. E., Langeland, K. A., Mullenix, M. K., et al. (2014). Invasive populations of elephantgrass differ in morphological and growth characteristics from clones selected for biomass production. Bioenergy Res. 7, 1382–1391. doi: 10.1007/s12155-014-9478-9
Spinoni, J., Vogt, J. V., Naumann, G., Barbosa, P., and Dosio, A. (2018). Will drought events become more frequent and severe in Europe? Int. J. Climatol. 38, 1718–1736. doi: 10.1002/joc.5291
Stitt, M., and Zhu, X. G. (2014). The large pools of metabolites involved in intercellular metabolite shuttles in C4 photosynthesis provide enormous flexibility and robustness in a fluctuating light environment. Plant Cell Environ. 37, 1985–1988. doi: 10.1111/pce.12290
Sun, H. M., Wang, A. Q., Zhai, J. Q., Huang, J. L., Wang, Y. J., Wen, S. S., et al. (2018). Impacts of global warming of 1.5 degrees C and 2.0 degrees C on precipitation patterns in China by regional climate model (COSMO-CLM). Atmosph. Res. 203, 83–94. doi: 10.1016/j.atmosres.2017.10.024
Sun, J. L., Zhang, Q. Q., Tabassum, M. A., Ye, M., Peng, S. B., and Li, Y. (2017). The inhibition of photosynthesis under water deficit conditions is more severe in flecked than uniform irradiance in rice (Oryza sativa) plants. Funct. Plant Biol. 44, 464–472. doi: 10.1071/fp16383
Taylor, S. H., Franks, P. J., Hulme, S. P., Spriggs, E., Christin, P. A., Edwards, E. J., et al. (2012). Photosynthetic pathway and ecological adaptation explain stomatal trait diversity amongst grasses. New Phytol. 193, 387–396. doi: 10.1111/j.1469-8137.2011.03935.x
Taylor, S. H., and Long, S. P. (2017). Slow induction of photosynthesis on shade to sun transitions in wheat may cost at least 21% of productivity. Philos. Transact. R. Soc. B Biol. Sci. 372:9.
Tomeo, N. J., and Rosenthal, D. M. (2017). Variable mesophyll conductance among soybean cultivars sets a tradeoff between photosynthesis and water-use-efficiency. Plant Physiol. 174, 241–257. doi: 10.1104/pp.16.01940
Tomimatsu, H., and Tang, Y. H. (2016). Effects of high CO2 levels on dynamic photosynthesis: carbon gain, mechanisms, and environmental interactions. J. Plant Res. 129, 365–377. doi: 10.1007/s10265-016-0817-0
Vialet-Chabrand, S. R. M., Matthews, J. S. A., McAusland, L., Blatt, M. R., Griffiths, H., and Lawson, T. (2017). Temporal dynamics of stomatal behavior: modeling and implications for photosynthesis and water use. Plant Physiol. 174, 603–613. doi: 10.1104/pp.17.00125
Vico, G., Manzoni, S., Palmroth, S., and Katul, G. (2011). Effects of stomatal delays on the economics of leaf gas exchange under intermittent light regimes. New Phytol. 192, 640–652. doi: 10.1111/j.1469-8137.2011.03847.x
Viswanathan, R., Chinnaraja, C., Malathi, P., Gomathi, R., Rakkiyappan, P., Neelamathi, D., et al. (2014). Impact of Sugarcane yellow leaf virus (ScYLV) infection on physiological efficiency and growth parameters of sugarcane under tropical climatic conditions in India. Acta Physiol. Plant. 36, 1805–1822. doi: 10.1007/s11738-014-1554-4
von Caemmerer, S. (2000). “Modelling C4 photosynthesis,” in Biochemical Models of Leaf Photosynthesis, Vol. 2, ed. S. Von Caemmerer (Collingwood,VIC: CSIRO Publishing), 91–122.
von Caemmerer, S., and Farquhar, G. D. (1981). Some relationships between the biochemistry of photosynthesis and the gas exchange of leaves. Planta 153, 376–387. doi: 10.1007/bf00384257
Wang, Y., Burgess, S. J., de Becker, E. M., and Long, S. H. P. (2020). Photosynthesis in the fleeting shadows: an overlooked opportunity for increasing crop productivity? Plant J. 101, 874–884. doi: 10.1111/tpj.14663
Wang, Y., Noguchi, K., Ono, N., Inoue, S., Terashima, I., and Kinoshita, T. (2014). Overexpression of plasma membrane H+-ATPase in guard cells promotes light-induced stomatal opening and enhances plant growth. Proc. Natl. Acad. Sci. U.S.A. 111, 533–538. doi: 10.1073/pnas.1305438111
Way, D. A., and Pearcy, R. W. (2012). Sunflecks in trees and forests: from photosynthetic physiology to global change biology. Tree Physiol. 32, 1066–1081. doi: 10.1093/treephys/tps064
WWAP (2015). The United Nations World water Development Report 2015: Water for a Sustainable World. Paris: UNESCO.
Xin, Z. G., Aiken, R., and Burke, J. (2009). Genetic diversity of transpiration efficiency in sorghum. Field Crops Res. 111, 74–80. doi: 10.1016/j.fcr.2008.10.010
Yabiku, T., and Ueno, O. (2017). Variations in physiological, biochemical, and structural traits of photosynthesis and resource use efficiency in maize and teosintes (NADP-ME-type C4). Plant Product. Sci. 20, 448–458. doi: 10.1080/1343943x.2017.1398050
Keywords: water-use efficiency, stomata, photosynthesis, non-steady-state gas-exchange, fluctuating light, dynamic light, sustainability, sorghum
Citation: Pignon CP, Leakey ADB, Long SP and Kromdijk J (2021) Drivers of Natural Variation in Water-Use Efficiency Under Fluctuating Light Are Promising Targets for Improvement in Sorghum. Front. Plant Sci. 12:627432. doi: 10.3389/fpls.2021.627432
Received: 09 November 2020; Accepted: 05 January 2021;
Published: 01 February 2021.
Edited by:
Adriano Nunes-Nesi, Universidade Federal de Viçosa, BrazilReviewed by:
Elizabeth Van Volkenburgh, University of Washington, United StatesChristoph Martin Geilfus, Humboldt University of Berlin, Germany
Copyright © 2021 Pignon, Leakey, Long and Kromdijk. This is an open-access article distributed under the terms of the Creative Commons Attribution License (CC BY). The use, distribution or reproduction in other forums is permitted, provided the original author(s) and the copyright owner(s) are credited and that the original publication in this journal is cited, in accordance with accepted academic practice. No use, distribution or reproduction is permitted which does not comply with these terms.
*Correspondence: Andrew D. B. Leakey, bGVha2V5QGlsbGlub2lzLmVkdQ==; Johannes Kromdijk, ams0MTdAY2FtLmFjLnVr; d2FubmVrcm9tZGlqa0BnbWFpbC5jb20=