- Key Laboratory of Germplasm Enhancement, Physiology and Ecology of Food Crops in Cold Region, Ministry of Education, Northeast Agricultural University, Harbin, China
Low-temperature tolerance during the bud-bursting stage is an important characteristic of direct-seeded rice. The identification of cold-tolerance quantitative trait loci (QTL) in species that can stably tolerate cold environments is crucial for the molecular breeding of rice with such traits. In our study, high-throughput QTL-sequencing analyses were performed in a 460-individual F2:3 mapping population to identify the major QTL genomic regions governing cold tolerance at the bud-bursting (CTBB) stage in rice. A novel major QTL, qCTBB9, which controls seed survival rate (SR) under low-temperature conditions of 5°C/9 days, was mapped on the 5.40-Mb interval on chromosome 9. Twenty-six non-synonymous single-nucleotide polymorphism (nSNP) markers were designed for the qCTBB9 region based on re-sequencing data and local QTL mapping conducted using traditional linkage analysis. We mapped qCTBB9 to a 483.87-kb region containing 58 annotated genes, among which six predicted genes contained nine nSNP loci. Quantitative reverse transcription-polymerase chain reaction (qRT-PCR) analysis revealed that only Os09g0444200 was strongly induced by cold stress. Haplotype analysis further confirmed that the SNP 1,654,225 bp in the Os09g0444200 coding region plays a key role in regulating the cold tolerance of rice. These results suggest that Os09g0444200 is a potential candidate for qCTBB9. Our results are of great significance to explore the genetic mechanism of rice CTBB and to improve the cold tolerance of rice varieties by marker-assisted selection.
Introduction
Rice (Oryza sativa L.) is an important food crop that has adapted to the tropical climate of the world. However, chilling injury of rice that occurs at high latitudes and high altitudes has severely restricted its production (Jena et al., 2012; Liu C. T. et al., 2019). Fast and uniform seed germination and vigorous seedling growth are essential for stable crop growth. The germination rate and early growth of seedlings after germination are the two main characteristics that are directly related to seedling vigor. Cold stress usually damages and delays germination and seedling growth of rice, leading to inferior stand establishment and uneven maturity, especially in areas where direct-seeded rice and cultivation are performed under low local temperatures (Iwata and Fujino, 2010; Manangkil et al., 2013). Therefore, low-temperature sensitivity at the bud-bursting stage remains a major challenge for direct-seeded rice. Improved cold tolerance at the bud-bursting (CTBB) stage is an important agronomic characteristic of direct-seeded rice breeding programs.
Because of the complexity and polygenicity of cold tolerance, numerous efforts have been directed to the detection and mapping of quantitative trait loci (QTL). In the past 20 years, more than 550 QTLs have been reported for different growth stages (germination, seedling, and reproductive/booting stage) for low-temperature stress using molecular markers of different genetic backgrounds derived from bi-parental mapping populations and diverse genetic resources of rice accessions (Najeeb et al., 2020). For example, numerous double haploid lines, backcross-inbred lines, recombinant inbred lines (RIL), and near-isogenic lines have been developed, with cold-tolerant varieties such as Kunming Xiaobaigu (Dai et al., 2004), Lijiang Xintuanheigu (Shirasawa et al., 2012), Koshihikari (Endo et al., 2016), M202 (Andaya and Tai, 2006), Norin-PL8 (Saito et al., 2010), and a Chinese type of wild rice (Dongxiang wild rice) (Mao et al., 2015) as donors. These representative cold-tolerant germplasms have contributed valuable QTLs or genes for research on the cold tolerance of rice. However, fewer QTLs have been identified at the bud-bursting stage than at the other developmental stage. As of 2015, 29 QTLs related to CTBB were integrated into chromosomes through meta-analysis (Zhu et al., 2015). Among them, qCTBP2, qCTBP4, and qCTBP7 were mapped using F3 populations based on simple sequence repeat (SSR) markers (Qiao et al., 2005). Four QTLs on chromosomes 3, 7, and 12 were detected using 98 backcross-inbred lines (Yang et al., 2009). In total, four QTLs for CTBB were preliminarily mapped on chromosomes 5 and 7 using a set of 95 chromosome segment substitution lines (CSSLs) derived from indica rice 9311 and Japonica rice Nipponbare (Lin et al., 2010). In the past 5 years, five new QTLs have explained 4.17–6.42% of the total phenotypic variation explained during the bud-bursting stage based on an RIL derived from H335 and CHA-1 (Yang et al., 2020). The other two cold-tolerant QTLs (qCTBB-5 and qCTBB-6) at the bud-bursting stage were mapped using single-segment substitution lines (Yang et al., 2016). These results indicate that the research on CTBB of rice is still in its infancy. Most QTLs lie within a range of 10–30 cM through primary populations, and it is difficult to perform molecular marker-assisted breeding. To date, only two QTLs have been cloned; qLTG3-1 encodes a protein with unknown function, which might regulate the vacuolization of aleurone and epidermal cells, thereby causing relaxation of these tissues and enhancing the germination potential of seeds at low temperatures (Fujino et al., 2008; Fujino and Matsuda, 2010; Fujino and Sekiguchi, 2011), whereas OsSAP16 encodes a zinc-finger domain protein and serves as the major causal gene for low-temperature germinability (Wang et al., 2018). These examples demonstrate that the molecular regulation of CTBB is extremely complex, and the aforementioned QTLs were identified by bi-parental cross-linkage mapping, which is a labor- and time-intensive method to map genotypes of a large number of individuals in a segregated population. Therefore, to obtain reliable QTLs, it is necessary to implement a fast and efficient method to identify QTLs.
Recently, with the rapid development of next-generation sequencing (NGS) technology, the combination of bulked segregant analysis with NGS has become an important path to mine QTLs and genes (Abe et al., 2012; Vikram et al., 2012; Kadambari et al., 2018; Wambugu et al., 2018). Compared with traditional linkage mapping, QTL-sequencing (Seq) can improve work efficiency and provide high-density variation. The efficiency of QTL-Seq has been successfully demonstrated in many plants, such as cucumber (Lu et al., 2014), soybean (Song et al., 2017), rice (Wambugu et al., 2018), and tomato (Wen et al., 2019). Although the technique allows for the quick identification of major QTLs, confidence interval (CI) resolution is still rough. Therefore, researchers have to combine QTL-Seq with fine-mapping (Zhang X. et al., 2018) and RNA-Seq (Park et al., 2019) to identify candidate QTL genes. For example, CaqSW1.1 (Das et al., 2015) was mapped from 1.37 Mb to 35 kb by QTL-Seq and classical QTL mapping, and the final candidate genes were identified. In another case, four candidate genes (SlCathB2, SlGST, SlUBC5, and SlARG1) associated with heat tolerance were detected by QTL-Seq and RNA-Seq in tomato (Wen et al., 2019). Thus, the method of obtaining a major QTL interval by QTL-Seq combined with fine-mapping to mine target genes provides a new perspective.
In our study, two strategies, QTL-Seq followed by linkage mapping, were employed to identify the major QTL interval for CTBB in rice. An F2:3 population derived from a cross between Dongnong430 (DN430, cold-sensitive variety) and Dongfu104 (DF104, strongly cold-tolerant variety) was used for QTL-Seq analysis. Furthermore, a linkage mapping strategy was considered to anchor major QTLs using a KASP marker. Furthermore, quantitative reverse transcription-polymerase chain reaction (qRT-PCR) and haplotype analysis were employed to verify the association between candidate genes and cold tolerance. The results obtained in this study are expected to help reveal the mechanism by which cold sensitivity changes into cold tolerance in rice at the molecular level and will improve the understanding of mechanisms underlying CTBB in rice.
Materials and Methods
Plant Materials
In this study, two Japonica varieties, the cold-sensitive female parent Dongnong 430 (DN430) and the cold-tolerant male parent Dongfu104 (DF104), were used as the parental lines to develop the 460 F2:3 lines. The materials mentioned previously herein were all obtained from Northeast Agriculture University in the northeast region of China.
Cold-Tolerance Evaluation at Bud-Bursting Stage
One hundred seeds of each F2:3 family and two parental were incubated at 40°C for ∼36 h to break dormancy and were soaked in deionized water at 30°C for approximately 72 h for germination. Fifty 5-mm-long buds of the two parental seeds were selected, washed with sterile water, and transferred to a petri dish with two layers of filter paper. In addition, 50 germinated seeds from 460 progeny lines were transferred to 96-well PCR plates. These 50 germinated seeds were then further subjected to a cold treatment at 5°C for 9 days in a growth chamber in darkness and were allowed to resume growth at 28°C for 3 days to investigate the severity of damage (SD). The SD of all 460 F2:3 lines and their parents was evaluated using the following scale: a cold score of 0 indicated that the seedling had normal leaf color and grew well with no damage, to a cold score of 7, which indicated that the germinated seed was dead with no green leaves, as described in previous studies (Zhang M. et al., 2018). The SD value of a plant represented the average score of 50 seeds. The seed survival rate (SR) was evaluated after 7 days of recovery of growth and was calculated as follows: SR (%) = (surviving seedlings/50) × 100 (Zhou et al., 2012). Three biological replicates were performed.
Construction of Segregating Pools
Young leaves of the 460 F2 individuals were collected separately for total genomic DNA extraction using a minor modified cetyltrimethylammonium bromide (CTAB) method (Murray and Thompson, 1980). The genomic DNAs of 30 extremely cold-tolerant and 30 extremely cold-sensitive individuals were selected as two bulked pools according to the SR of the F2:3 population, ranging from 0 to 100%. To simplify the following description, we abbreviate DF104 as T, DN430 as S, the cold-tolerant pool as the T-pool, and the cold-sensitive pool as the S-pool. Isolated DNA was quantified using a Nanodrop 2000 spectrophotometer (Thermo Scientific, Fremont, CA, United States). All DNAs from the T-pool and S-pool plants were quantified on a Qubit® 2.0 Fluorometer (Life Technologies, Carlsbad, CA, United States), and equal amounts of DNA from the T-pool and S-pool plants were mixed.
NGS Sequencing and QTL-Seq Analysis
Total genomic DNA was extracted from bulked pools, and at least 3 μg genomic DNA was used to construct paired-end libraries with an insert size of 500 bp using the Paired-End DNA Sample Prep kit (Illumina Inc., San Diego, CA, United States). These libraries were sequenced using the HiSeq X10 (Illumina Inc., San Diego, CA, United States) NGS platform at Genedenovo (Guangzhou, China). Quality trimming is an essential step in generating high confidence in variant calling; raw reads were processed to obtain high-quality clean reads according to three stringent filtering standards as follows: (1) reads with ≥ 10% unidentified nucleotides (N) were removed; (2) reads with > 50% bases with a phred quality score of ≤ 20 were removed; and (3) reads were aligned to the barcode adapter.
To identify single-nucleotide polymorphisms (SNPs) and InDels, filtered reads were aligned to the Nipponbare reference genome sequence (International, 2005) using Burrows-Wheeler Aligner (BWA, v 0.7.16a-r1181) with parameter “mem -M”; -M is an option used to mark shorter split alignment hits as secondary alignments (Kumar et al., 2019). Variant calling was performed using the GATK UnifiedGenotyper (v3.5). SNPs and InDels were filtered using the GATK VariantFiltration function with proper standards (-Window 4, -filter “QD < 4.0 | | FS > 60.0 | | MQ < 40.0,” -G_filter “GQ < 20”). All mutations were annotated for genes and functions, as well as genomic regions, using ANNOVAR (Wang et al., 2010). Association analysis was performed using the SNP-index (Abe et al., 2012), Δ(SNP-Index) (Takagi et al., 2013), calculation of G statistic (Magwene et al., 2011; Mansfeld and Grumet, 2018), Euclidean distance (ED) (Hill et al., 2013), and two-tailed Fisher exact test (Fisher, 1922) based on the SNPs. Finally, the overlapping interval of the four methods was considered the final QTL interval.
Development of KASP Markers and Linkage Mapping
To develop markers for validation of QTL-Seq results and narrow the candidate region, 26 non-synonymous SNPs (nSNPs) with significant peaks were found based on the CDS sequence of the two parents in the qCSBB9 region under the four association analysis algorithms [Δ (SNP-Index), G-value, ED-value, and Fisher exact test] and were considered as further markers for linkage mapping. KASP primers were designed using Primer express 3.0.11; the primers are listed in Supplementary Table 1. All markers were selected for polymorphism between parents, and genotyping of the 460 individuals was performed using the polymorphic markers, which were used to construct the linkage map and narrow the candidate region using the inclusive composite interval mapping (ICIM) module of QTL IciMapping 4.22 The threshold of the logarithm of odds (LOD) for declaring the presence of a significant QTL was determined by the permutation test with 1,000 repetitions at P < 0.01.
Prediction of Candidate Genes for the CTBB
To predict possible candidate genes for the CTBB, the following three strategies were comprehensively considered in this study. First, candidate gene prediction was performed to compare the DNA sequences of those genes within the QTL regions using the whole-genome DNA resequencing database of the parents. In the QTL regions, we focused on open reading frames with non-synonymous mutant SNPs between the two parents. For the second, the most possible candidate genes were reselected according to their functional annotations, screening the rice genome database3. For the third, gene functions were annotated by gene ontology (GO) (Ashburner et al., 2000) and Kyoto Encyclopedia of Genes and Genomes (KEGG) (Kanehisa et al., 2004) databases with BLAST software (Altschul et al., 1997). Finally, the candidate genes were obtained.
Verification of the Expression Level of Candidate Genes
Five-millimeter-long buds of DN430 and DF104 were collected at 2, 6, 12, 24, and 48 h after cold treatment for three repetitions, frozen in liquid nitrogen, and stored at −80°C for total RNA extraction. Control plants were also collected and stored similarly. qRT-PCR was used to quantify the expression levels of candidate genes under cold treatment. Total RNA was extracted from rice tissues using TRIzol reagent (Thermo Fisher Scientific, Waltham, MA, United States) and treated with DNase I to eliminate any DNA contamination. RNA quality was assessed by electrophoresis, and the RNA was stored at −80°C until use. First-strand cDNA (10 μL) was synthesized according to the instructions for the PrimeScriptTM RT Master Mix [Takara Biomedical Technology (Beijing) Co., Ltd., Beijing, China]. Primers were designed with Primer Premier v. 5.0 (PREMIER Biosoft International, United States) and are listed in Supplementary Table 2. The housekeeping gene Actin1 (Os05g36290) was used as an internal control (Siahpoosh et al., 2012), and qRT-PCR was performed using a Roche LightCycler 2.10 with a 2× SYBR Green I PCR Master Mix. qRT-PCR analysis was performed as previously described (Zhang Z. et al., 2018).
Haplotype Analysis of Candidate Genes
Here, we present one method of analyzing haplotypes of candidate genes, which are variants of candidate genes in the 30 T-pool, 30 S-pool, and 295 northern China Japonica rice strains (Li et al., 2019). Primer sequences were designed for candidate genes at SNP sites between parents, and the DNA fragments of the sequences in 60 lines were amplified by PCR to perform haplotype statistics on the distribution of target genes. All specific primer sequences are listed in Supplementary Table 2. The PCR reaction mixture had a total volume of 20 μL, containing 1.5 μL of forward primer (10 μm), 1.5 μL of reverse primer (10 μm), 2 μL of genomic DNA (50 ng/μL), 5 μL of ddH2O, and 10 μL of Pfu master mix (Chinese Beijing cwbio), which included Taq DNA polymerase, PCR buffer, Mg2+, and dNTPs. The PCR reaction was performed in an Eppendorf 5333 Mastercycler using the same protocol as that used for qRT-PCR (Zhang Z. et al., 2018). The products were examined by 1% agarose gel electrophoresis. Direct sequencing of PCR products was performed by BGI Life Technology Co., Ltd.
Results
Phenotypic Analysis of the Parental Lines and F2:3 Population
Two parental varieties, DN430 and DF104 (Figure 1A), along with their 460 F2:3 lines (Figure 1B), were evaluated for two cold tolerance indices (SD and SR) at the bud-bursting stage at 5°C/9 days. The SR of the cold-tolerant variety DF104 was significantly higher than that of cold-sensitive DN430, indicating that DF104 had a stronger CT than DN430. In the F2:3 population, SR exhibited continuous variation from 0 to 100% (Figure 1C and Supplementary Table 3), and SD showed variation from 0.00 to 7.00 (Figure 1D and Supplementary Table 3). Interestingly, the scatter plot revealed that all individuals with SD values of 0 or 1 had SR values between 90 and 100%, whereas lines with SD values of 5 or 7 had SR values distributed between 0 and 20% (Figure 1E). Correlation analysis showed that the correlation coefficient between SR and SD was −0.938 (P = 2.985E-213). Figure 1C shows that among the 460 lines, 30 cold-tolerant and 30 cold-sensitive lines were identified. These were then selected to prepare the T-pool and S-pool, respectively, which were then used for DNA re-sequencing.
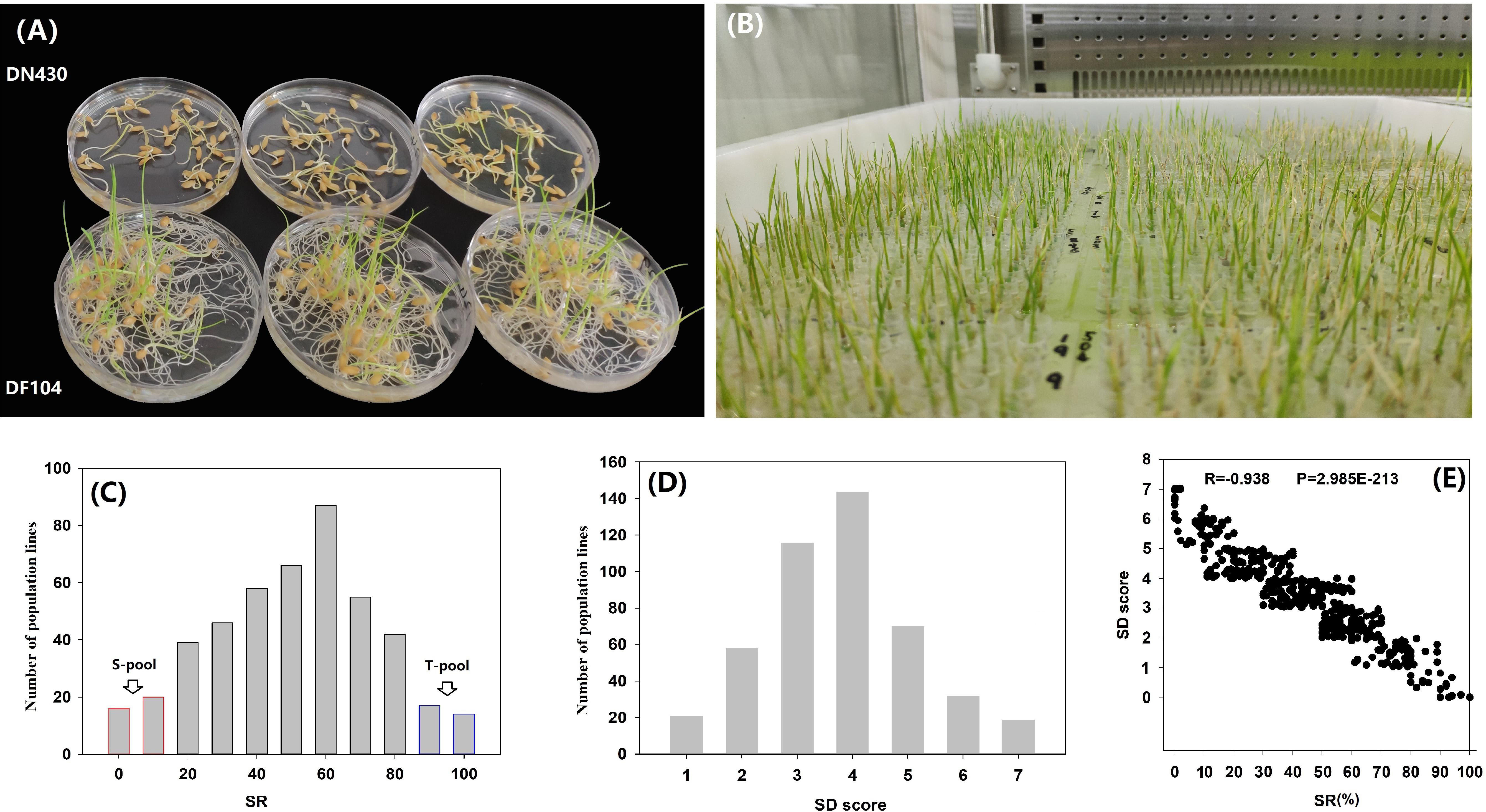
Figure 1. Phenotypic analysis of cold tolerance in rice bud-bursting stage. (A) The performance of DN420 and DF104 under cold treatment (5°C/9 days). (B) Identification of cold tolerance of F2 population in artificial climate chamber. (C,D) Frequency distribution of seed survival rate (SR) and severity of damage (SD) variation measured among 460 mapping individuals of F2:3 populations. (E) Paired scatter plot analysis of SR and SD. T-Pool, DNA pool of cold-tolerant lines, S-pool, and DNA pool of cold-sensitive lines.
Whole-Genome Re-sequencing and QTL-Seq Analysis
Illumina high-throughput sequencing generated 566.87 million raw reads; 562.53 million clean reads (99.20%) were obtained after filtering, and the four samples generated 84,033,193,174-bp bases. When compared with the “Nipponbare” reference genome, these four samples generated 23,53,180 SNPs and 4,16,850 Indels (Supplementary Table 4). These high-quality data provided a solid foundation for subsequent analysis.
For all obtained SNPs, using the association threshold of 95 and 99%, the values of Δ (SNP-Index) (Figure 2A), ED (Figure 2B), G′ value (Figure 2C), and Fisher’s exact test (Figure 2D) were used to intercept the association interval for CTBB. There were four QTL regions to be identified, which mapped to chromosome 2, 3, 5, and 9 (Table 1 and Figure 2). For chromosome 2, one genomic region (17.06–19.24 Mb) was obtained by the ED algorithm at the 95% significance level, which we designated qCTBB2. The other three genomic regions, namely, qCTBB3 (16.32–22.58 Mb), qCTBB5 (0–3.54 Mb), and qCTBB9 (9.54–21.38 Mb) on chromosomes 3, 5, and 9 for CTBB at the 95% significance level were revealed. However, the highest peak-value could be seen on chromosome 9 in the four algorithms. Of note, only a single QTL, qCTBB9, was obtained when the cut-off CI value of 99% was applied. For Δ (SNP-Index), qCTBB9 spanned 9.34 Mb intervals, and the ED algorithm completely included the result given by the Δ (SNP-Index) algorithm. The G′ value and Fisher’s exact test similarly captured the results of the first two algorithms. Here, the ED, G-value, and Fisher’s exact test identified a small QTL interval. Obviously, a larger peak-value was obtained at the 99% significance level than at the 95% significance level. Therefore, qCTBB9 is considered the most significant target for CTBB; considering the overlap interval of the four calculation models, qCTBB9 was narrowed to a region of 5.4 Mb, which contains 647 predicted genes. According to QTL-Seq analysis, for the qCTBB9 coding region, 281 nSNPs and seven Indels were detected (Supplementary Table 5).
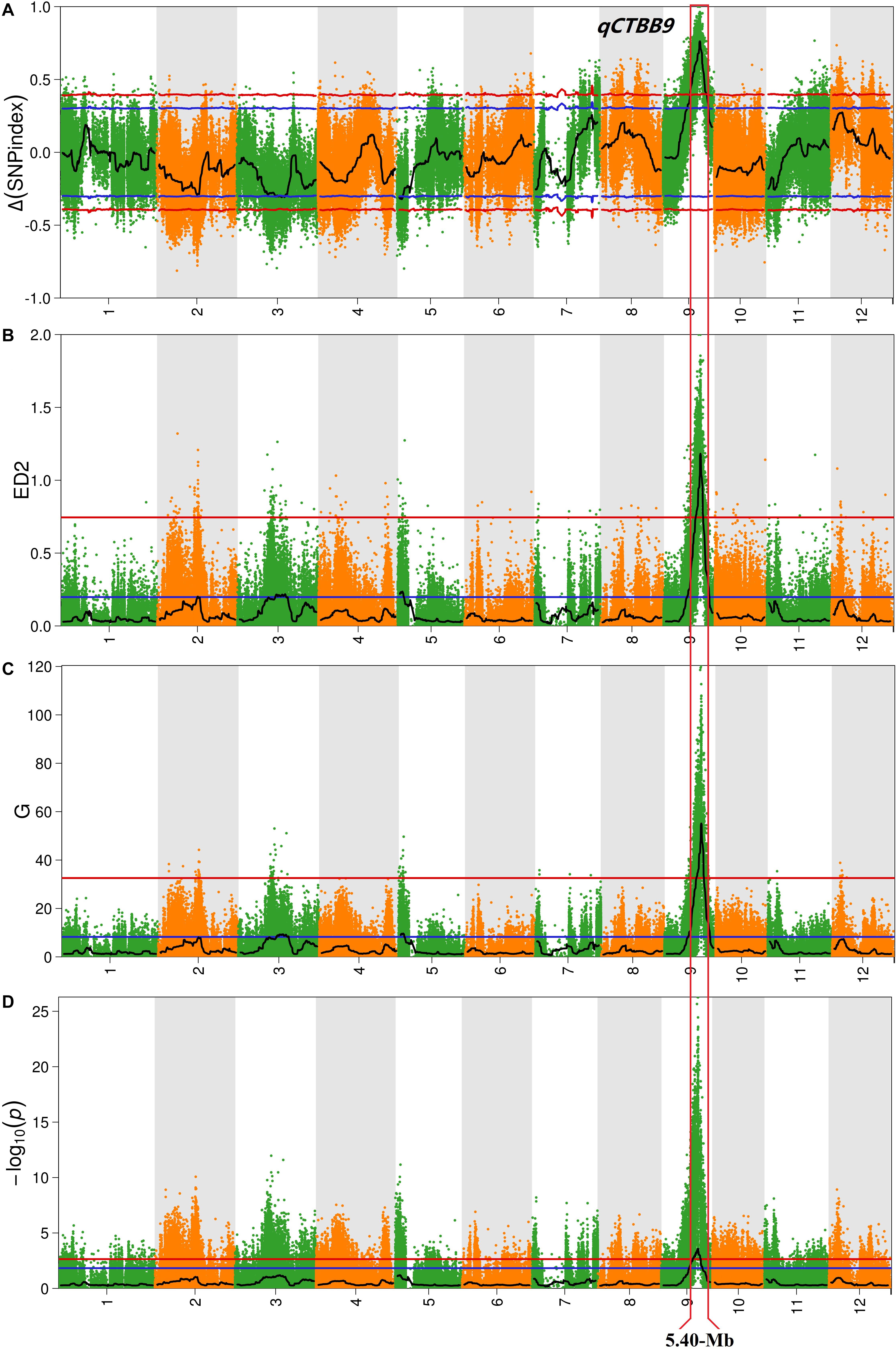
Figure 2. Analysis of quantitative trait loci of cold tolerance at the bud-bursting stage based on QTL-Seq. (A) Manhattan plot shows the distribution of SNP-index and Δ (SNP-index) on chromosomes. (B) The Manhattan plot shows the distribution of the square of the Euclidean distance on the chromosomes. (C) The Manhattan plot shows the distribution of G′-Value on the chromosomes. (D) The Manhattan plot shows the distribution of –log10(P) value on the chromosomes based on Fisher exact test. The blue and red lines represent 95 and 99% confidence intervals, respectively.
Fine Mapping of qCTBB9
Because the 5.4 Mb region still contained numerous genes, to fine-map qCTBB9 based on the nucleotide polymorphism information of the 5.4 Mb it covers, the highly credible SNPs obtained by the four association algorithms were fully considered, and the SNPs with low credibility under some algorithms were filtered out. Finally, SNPs with a relative distance of less than 200 bp were eliminated, and 26 excellent nSNPs (Supplementary Table 1) were used to scan the genotypes of the 460 lines and were used to obtain a qCTBB9 linkage map. A QTL was considered significant when the LOD value was equal to or larger than 3.0 (Zhao et al., 2018). The SR of the 460 lines under cold stress was analyzed by linkage mapping. qCTBB9 was simultaneously linked with SR and anchored to the 483.87 kb interval between 1,60,59,891 and 1,65,43,763 bp (Figures 3A,B); this explained 24.91% of the phenotypic variation in seed SR, and the peak-value for the LOD score was 14.01 (Table 2). The positive allele qCTBB9 was contributed by DF104, and qCTBB9 was optimized to a physical interval of 483.87 kb.
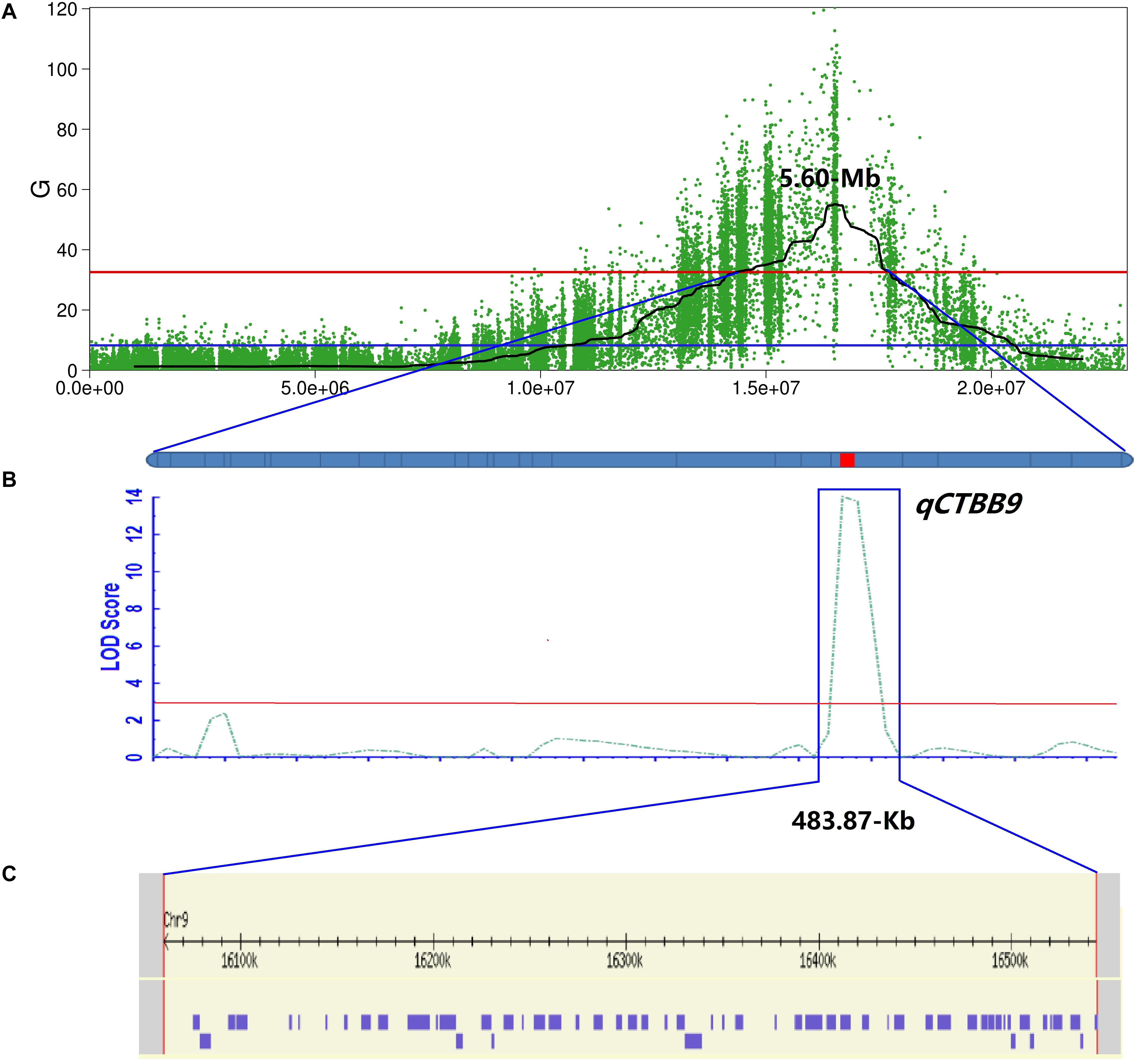
Figure 3. Further mapping of the qCTBB9. (A) The Manhattan diagram shows the location of the qCTBB9 on chromosome 9. (B) qCTBB9 detected by using the ICIM module of QTL IciMapping 4.2. Linkage map based on KASP markers. The red lines represent the logarithm of odds (LOD) threshold of 2.0. (C) 58 genes in qCTBB9 region were obtained through the annotation information on the Nipponbare genome.
Putative Candidate Genes and Candidate SNPs in the Genomic Regions for CTBB
The 483.87 kb interval was intercepted through the annotation information of the Nipponbare genome3, and 58 annotation genes were captured (Supplementary Table 6 and Figure 3C). Some important genes or gene families related to cold tolerance in rice or other crops have been reported, such as cytochrome P450 (Su et al., 2010), a hypothetical protein (Zhao et al., 2017), a chromatin remodeling factor (Yang et al., 2019), an F-box domain (Saito et al., 2010), lipid metabolism genes (Zhang et al., 2019), a protein kinase (Ding et al., 2019), and a TATA-binding protein (Xu et al., 2020). Although similar genes or proteins were included in the list of 58 candidate genes, the 43 genes obtained from GO analysis were not annotated to the term response to cold. The most enriched terms of biological process, molecular function, and cellular component ontology were metabolic processes (i.e., GO:0006629, GO:0008152, and GO:0044237), activity (i.e., GO:0016491, GO:0003824, and GO:0016787), and cell (i.e., GO:0005575, GO:0005623, and GO:0044464), respectively (Supplementary Table 7 and Supplementary Figure 1). Further, 483.87 kb on chromosome 9 had 240 effective SNPs between parental lines and a Δ (SNP−index), G-value, ED, and P-value of the two-tailed Fisher exact test higher than the statistical confidence at P < 0.01 (Supplementary Table 6). Of the 240 SNPs, 206 SNPs were intergenic and 34 SNPs were genic, including 16 intronic, nine non−synonymous, four synonymous, and five in UTRs. The nine nSNPs could be identified using both parents as a reference and affected six candidate genes encoding three hypothetical conserved genes (Os09g0436500, Os09g0443400, and Os09g0443700), one TATA-binding related factor domain containing protein (Os09g0443500), one similar to minus dominance protein (Os09g0444100), and one cholesterol acyltransferase family protein (Os09g0444200) (Table 3). As mentioned, these six types of genes might play an important role in responding to cold stress in plants. Of the six candidate genes, only Os09g0444200 was screened in the KEGG pathway (pathway ID K00679) (Supplementary Table 7 and Supplementary Figure 2), which was involved in the glycerolipid metabolism, and glycerolipid is considered significantly related to plant cold sensitivity (Eriksson et al., 2011). Based on this, we identified the six final possible candidate genes through three approaches as follows: the possible relationship between gene response and cold stress, non-synonymous mutations in the gene coding region, and the participation of genes in specific metabolic pathways that affect cold tolerance.
Candidate Genes Verified by qRT-PCR and Haplotype Analysis
To identify strong candidate genes for qCTBB9, the expression levels of six genes under cold stress were further analyzed. The results showed that only Os09g0444200 showed strong induction of the cold stress response in parents with extremely strong CTBB (Figure 4), suggesting that Os09g0444200 is the most likely candidate gene for qCTBB9. In contrast, under normal conditions, all six candidate genes had no significant expression levels (Supplementary Figure 3). The strong correlation between RNA expression levels and SR in these 60 lines indicates the regulatory function of Os09g0444200 for CTBB (Figure 5). Based on these findings, we considered Os09g0444200 as a possible candidate CTBB gene based on qCTBB9 for further analysis.
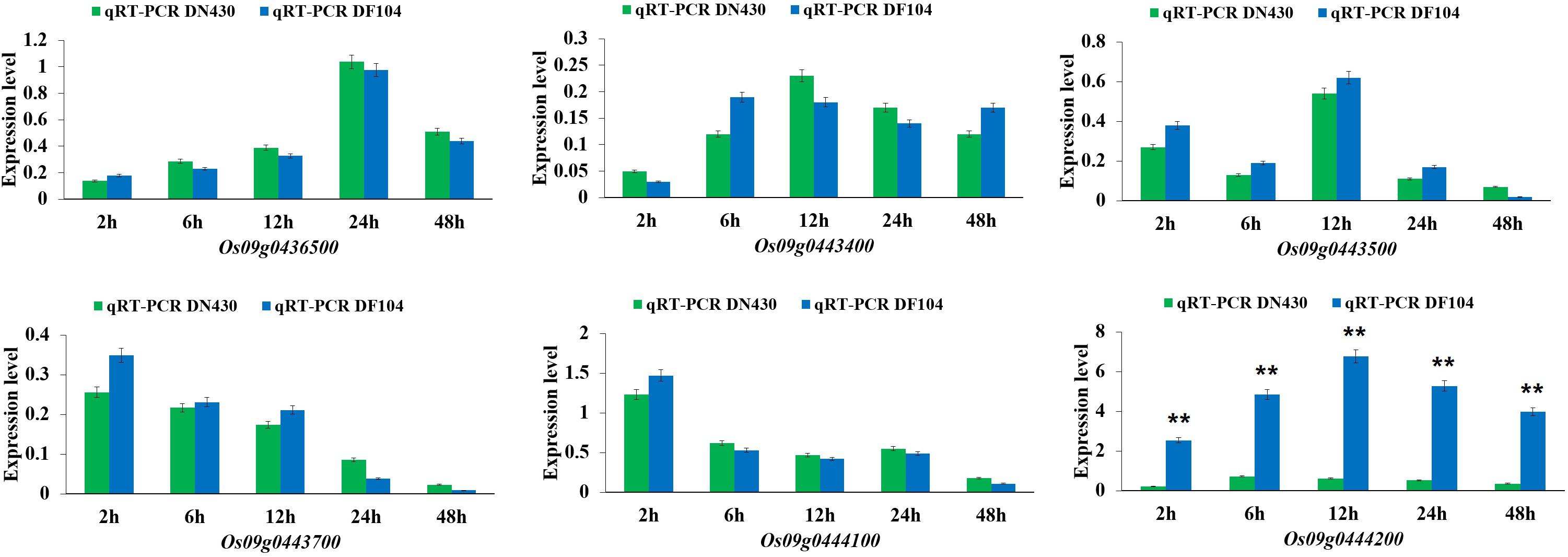
Figure 4. Expression levels of the six candidate genes in DN430 and DF104 after cold stress measured by qRT-PCR. The results were statistically analyzed using Student’s t-test (**P < 0.01).
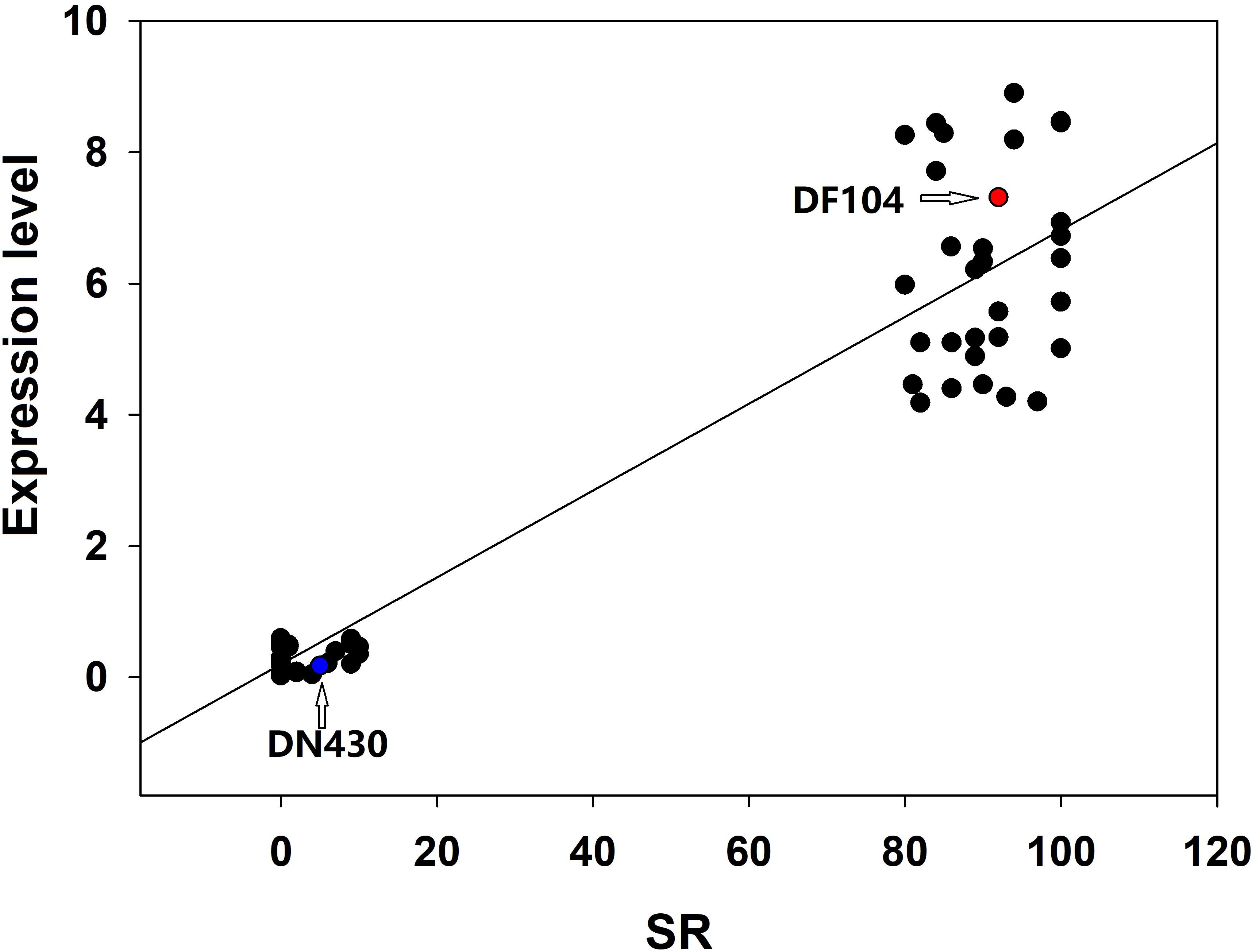
Figure 5. Correlation between the expression level of Os09g0444200 and seed survival rate in 30 cold-tolerant lines and 30 cold-sensitive lines after cold treatment.
Os09g0444200 encodes lecithin–cholesterol acyltransferase. To reveal the significance of variations in the Os09g0444200 coding region, we selected 60 lines each from the T-pool and the S-pool and sequenced their Os09g0444200 genes by Sanger sequencing. Four SNPs were observed in the CDS sequence of Os09g0444200–SNP-16543710, SNP-16543763, SNP-16544225, and SNP-16546293. The four SNPs divided 460 F2:3 genotypes into four haplotypes (HapI, HapII, HapIII, and HapIV) (Supplementary Table 8). DN430 was classified as HapIII, and DF104 was classified as HapI. The 30 cold-tolerant and 30 cold-sensitive lines showed consistent isolation of the nSNP (1,654,225 bp), suggesting that this might lead to phenotypic differences in CTBB. We then referred to the data of the 3010 Rice Genomes Project and found that HapI (TAGT) and HapIII (CGAC) mainly exist in the genotype of Japonica rice (Supplementary Table 9). We subsequently analyzed the polymorphism types of Os09g0444200 in 295 China northern Japonica rice and found that HapI did not occur in the samples (Supplementary Table 10). It could thus be inferred that HapI is a rare natural variation. Therefore, it can be used as a special functional variation in DF104 to improve the CTBB of rice varieties. However, it cannot be ruled out that other Japonica Os09g0444200 markers might have similar SNPs.
Discussion
Chilling injury events have been frequently observed in Northeast China in the past few years (Ma et al., 2017; Shen et al., 2019). The pre-germinated rice seeds directly planted in the soil are affected by the low temperature of air and/or irrigation water, which considerably reduces the germination rate and can lead to seed death (Fujino and Matsuda, 2010). Consequently, cold-tolerant rice varieties will contribute to food security and sustainable development. To locate the major QTLs of CTBB, in this study, we used several approaches to obtain the most reliable QTL interval. First, we used a large population to identify their phenotypes in low-temperature conditions, thereby obtaining accurate cold-tolerant phenotypes for each line. Second, two DNA pools with strict cold tolerance phenotypic differences were used to perform QTL-Seq analysis. Moreover, we used four bioinformatic analysis approaches to map QTL regions at the 99% significance level. Finally, only one highly significant peak (qCTBB9) was detected on chromosome 9 (Table 1 and Figure 2), with the QTL mapped between 13.34 and 18.74 Mb. Some QTLs and genes for cold tolerance on chromosomes 9 have been reported in recent years. The QTL qPGCG9-1 (Schlappi et al., 2017) was studied for the CTBB of rice, and according to the “Nipponbare” reference genome, was mapped to chromosome 9, at a position 6.83 Mb away from qCTBB9. qLTS9 and qCTS9-5 were associated with cold tolerance at the seedling stage (Lv et al., 2016), and they were located in the 22.701–22.887 and 4.304–4.488 Mb intervals on chromosome 9, respectively; their physical distances from the qCTBB9 were 4.00 and 5.852 Mb, respectively. The location of qCTBB9 in our study completely overlaps the previously reported region of qCTS-9 (Zhao et al., 2017), which has been linked to cold tolerance at the seeding stage. Moreover, in the qCTBB9 interval, OsWRKY76 (Yokotani et al., 2013), OsDREB1A, and OsDREB1B (Ito et al., 2006) were involved in the regulatory mechanism of the rice cold-tolerance response. In addition, we did not find any cold-tolerance QTLs or genes related to the bud-bursting stage on chromosome 9. Notably, qCTBB9 was associated with the only strong peak under the four calculation models (Figure 2). This shows that there is a significant difference in the allele ratio between the two mixed pools. Therefore, qCTBB9 can be considered the most significant target for cold tolerance to explore candidate genes. Moreover, the other two QTL intervals, qCTBB3 and qCTBB5, were detected by three approaches [Δ(SNP-index), G′ value, and ED] at the 95% significance level (Table 1); for qCTBB3, no existing cold-tolerance gene or QTL was found in its genome region, and in the qCTBB5 interval, only OsLti6b, encoding a hydrophobic protein homologous, increased cold tolerance by overexpression (Kim et al., 2007). However, the peak of these two QTLs was less than one-third that of qCTBB9 (Table 1 and Figure 2). As suggested by previous studies, the causative genes underlying QTL probably reside in genomic regions with the highest peak-values (Win et al., 2017). Therefore, to identify genomic regions controlling CTBB with higher probability, we applied the P < 0.01 cutoff rather than P < 0.05.
In our study, several studies confirmed that combining QTL-Seq and traditional linkage mapping to uncover a very narrow candidate region is well suited for faster gene targeting (Feng et al., 2019; Liu G. et al., 2019). To screen potential candidate genes of the qCTBB9 interval, based on the QTL-seq results, 26 nSNP markers were further used to perform genotype scanning on all 460 lines; using this approach, we reduced the number of candidate genes within the qCTBB9 interval defined by QTL-Seq from 647 to 58 genes. Among these 58 genes, only six had nine functional base variations. Furthermore, the expression level of Os09g0444200 in the two parents showed that it was strongly induced after cold treatment. The expression analysis of Os09g0444200 in 60 lines with differences in extreme cold responses revealed a correlation between cold-tolerance and gene expression levels (Figure 5). Notably, there were four SNP differences between the two parents in the coding region of the Os09g0444200 gene, which directly led to the difference in the expression level of the Os09g0444200 gene under cold stress (Figure 4). Further haplotype analysis results show that the nSNP (1,654,225 bp) is responsible for the phenotypic difference (Supplementary Table 8). In addition, the 3010 Rice Genome Project database showed that the Os09g0444200DF104 and Os09g0444200DN430 haplotypes mainly exist in the genotype of Japonica rice (Supplementary Table 9). However, haplotype events did not occur in the 295 northern cultivars (Supplementary Table 10). This means that the four SNPs in the DF104 coding region are rare variants of Japonica rice in northern China, which could serve as a genomic marker for improved CTBB. Moreover, the molecular mechanism of the interaction between Os09g0444200 and its cofactors needs to be investigated in future studies. Although we have found correlations between the nSNP in Os09g0444200 and the studied phenotypic traits, this is not enough to assign a certain tolerant phenotype to a single SNP. In contrast, we believe that a favorable allelic variant in one key gene is not sufficient to provide cold tolerance, and the final cold-tolerance phenotype of the plant should be considered a result of a combination of favorable allelic variations from different key genes.
Cold tolerance is a complex trait controlled by multiple genes (Zhang et al., 2014), and the genes that have been isolated thus far can be divided into two different types, those that regulate gene expression during stress responses, including signaling components and transcription factors (TFs) (Huang et al., 2012), and those that have specific functions and participate in systemic metabolism to defend against cold stress (Huang et al., 2012). For example, OsLTPL159 enhances the cold tolerance of rice at the early seedling stage by decreasing the toxic effect of reactive oxygen species, enhancing cellulose deposition in the cell wall, and promoting osmolyte accumulation (Zhao et al., 2020). Moreover, CTB4a, as a leucine-rich repeat receptor-like kinase, positively regulates the activity and content of ATP under cold stress by interacting with the β subunit of ATP synthase, thereby increasing pollen fertility (Zhang et al., 2017). In our study, we observed that Os09g0444200 encodes a lecithin–cholesterol acyltransferase through the annotation information of the Nipponbare genome, and KEGG database annotation results revealed that Os09g0444200 is involved in the lipid metabolism pathway (ko01100//Metabolic pathways; ko00561//Glycerolipid metabolism) (Supplementary Table 7). For plants, chilling tolerance is closely related to the composition, structure, and metabolic processes of membrane lipids (Zhang et al., 2019), and plants can adjust membrane stability and fluidity by changing the unsaturation of fatty acids in membrane lipids, which is important for resisting cold stress (Karabudak et al., 2014). With the development of biotechnology, the genetic engineering of rice cold tolerance has considerably progressed. Several genes related to cold tolerance in fatty acid metabolism pathways have been cloned and transferred to plants for functional research (Nair et al., 2009; Cao et al., 2010); for rice, qPSST6, a major gene involved in the synthesis of long-chain fatty acids, was identified as a cold-tolerance gene at the booting stage of rice (Sun et al., 2018). However, whether Os09g0444200 regulates cold tolerance by participating in lipid metabolism requires further construction of transgenic rice for verification. Moreover, as a newly identified CTBB regulator, the signaling transduction system and the downstream pathways of Os09g0444200 are still unclear. The biological functions of Os09g0444200 and its associated genomic variations need to be further confirmed using gene editing and high-efficiency overexpression transformation systems.
Data Availability Statement
The datasets presented in this study can be found in online repositories. The names of the repository/repositories and accession number(s) can be found below: NCBI (accession: PRJNA688381).
Author Contributions
LY and DZ conceived and designed the research. LY, LL, and PL participated in data analysis. JW, HZ, CW, FY, JC, CW, and LL performed material development, sample preparation, and data analysis. LY wrote the manuscript. DZ corrected the manuscript. All authors read and approved the final manuscript.
Funding
This research was financially supported by the “Breeding of high-quality and resistant rice varieties” (Grant No. 2020ZX16B01), a major scientific and technological project of “Hundreds and Thousands” in Heilongjiang Province, China, and the China Postdoctoral Science Foundation (Grant No. 2019M651249).
Conflict of Interest
The authors declare that the research was conducted in the absence of any commercial or financial relationships that could be construed as a potential conflict of interest.
Acknowledgments
We thank Editage (www.editage.cn) for English language editing.
Supplementary Material
The Supplementary Material for this article can be found online at: https://www.frontiersin.org/articles/10.3389/fpls.2021.647239/full#supplementary-material
Supplementary Figure 1 | Clustering map of GO annotation of genes in 483.87 kb regions. The abscissa is the content of GO categories, and the left of the ordinate is the number of genes. This figure shows the gene classification of GO secondary functions in the context of all genes in the associated region.
Supplementary Figure 2 | Clustering map of KEGG annotation genes in 483.87 kb regions. The ordinate is the name of the KEGG pathway, and the abscissa is the number of genes annotated under the pathway.
Supplementary Figure 3 | Expression levels of the six candidate genes in DN430 and DF104 under normal condition measured by qRT-PCR. The results were statistically analyzed using Student’s t-test (∗∗P < 0.01).
Footnotes
References
Abe, A., Kosugi, S., Yoshida, K., Natsume, S., Takagi, H., Kanzaki, H., et al. (2012). Genome sequencing reveals agronomically important loci in rice using MutMap. Nat. Biotechnol. 30, 174–178. doi: 10.1038/nbt.2095
Altschul, S. F., Madden, T. L., Schäffer, A. A., Zhang, J., Zhang, Z., Miller, W., et al. (1997). Gapped BLAST and PSI-BLAST: a new generation of protein database search programs. Nucleic Acids Res. 25, 3389–3402. doi: 10.1093/nar/25.17.3389
Andaya, V., and Tai, T. (2006). Fine mapping of the qCTS12 locus, a major QTL for seedling cold tolerance in rice. Theor. Appl. Genet. 113, 467–475.
Ashburner, M., Ball, C. A., Blake, J. A., Botstein, D., Butler, H., Cherry, J. M., et al. (2000). Gene ontology: tool for the unification of biology. Nat. Genet. 25, 25–29. doi: 10.1038/75556
Cao, Y. P., Shi, J. L., Li, Z., and Ming, F. (2010). Isolation of OsFAD2, OsFAD6 and FAD family members response to abiotic stresses in Oryza sativa L. Hereditas 32, 839–847. doi: 10.3724/SP.J.1005.2010.00839
Dai, L. Y., Lin, X. H., Ye, C. R., Ise, K., Saito, K., Kato, A., et al. (2004). Identification of quantitative trait loci controlling cold tolerance at the reproductive stage in Yunnan landrace of rice, Kunmingxiaobaigu. Breed. Sci. 54, 253–258. doi: 10.1270/jsbbs.54.253
Das, S., Upadhyaya, H. D., Bajaj, D., Kujur, A., Badoni, S., and Laxmi, et al. (2015). Deploying QTL-seq for rapid delineation of a potential candidate gene underlying major trait-associated QTL in chickpea. DNA Res. 22, 193–203. doi: 10.1093/dnares/dsv004
Ding, Y., Shi, Y., and Yang, S. (2019). Advances and challenges in uncovering cold tolerance regulatory mechanisms in plants. New Phytol. 222, 1690–1704. doi: 10.1111/nph.15696
Endo, T., Chiba, B., Wagatsuma, K., Saeki, K., Ando, T., Shomura, A., et al. (2016). Detection of QTLs for cold tolerance of rice cultivar ‘Kuchum’and effect of QTL pyramiding. Theor. Appl. Genet. 129, 631–640. doi: 10.1007/s00122-015-2654-2
Eriksson, S. K., Kutzer, M., Procek, J., Gröbner, G., and Harryson, P. (2011). Tunable membrane binding of the intrinsically disordered dehydrin Lti30, a cold-induced plant stress protein. Plant Cell 23, 2391–2404. doi: 10.1105/tpc.111.085183
Feng, X. X., Cheng, H. L., Zuo, D. Y., Zhang, Y. P., Wang, Q. L., Liu, K., et al. (2019). Fine mapping and identification of the fuzzless gene GaFzl in DPL972 (Gossypium arboreum). Theor. Appl. Genet. 132, 2169–2179. doi: 10.1007/s00122-019-03330-3
Fisher, R. A. (1922). On the interpretation of χ 2 from contingency tables, and the calculation of P. J. R. Stat. Soc. 85, 87–94.
Fujino, K., and Matsuda, Y. (2010). Genome-wide analysis of genes targeted by qLTG3-1 controlling low-temperature germinability in rice. Plant Mol. Biol. 72, 137–152. doi: 10.1007/s11103-009-9559-x
Fujino, K., and Sekiguchi, H. (2011). Origins of functional nucleotide polymorphisms in a major quantitative trait locus, qLTG3-1, controlling low-temperature germinability in rice. Plant Mol. Biol. 75, 1–10. doi: 10.1007/s11103-010-9697-1
Fujino, K., Sekiguchi, H., Matsuda, Y., Sugimoto, K., Ono, K., and Yano, M. (2008). Molecular identification of a major quantitative trait locus, qLTG3-1, controlling low-temperature germinability in rice. Proc. Natl. Acad. Sci. U.S.A. 105, 12623–12628. doi: 10.1073/pnas.0805303105
Hill, J. T., Demarest, B. L., Bisgrove, B. W., Gorsi, B., Su, Y. C., and Yost, H. J. (2013). MMAPPR: mutation mapping analysis pipeline for pooled RNA-seq. Genome Res. 23, 687–697. doi: 10.1101/gr.146936.112
Huang, G. T., Ma, S. L., Bai, L. P., Zhang, L., Ma, H., Jia, P., et al. (2012). Signal transduction during cold, salt, and drought stresses in plants. Mol. Biol. Rep. 39, 969–987. doi: 10.1007/s11033-011-0823-1
International, R. G. S. P. (2005). The map-based sequence of the rice genome. Nature 436, 793–800. doi: 10.1038/nature03895
Ito, Y., Katsura, K., Maruyama, K., Taji, T., Kobayashi, M., Seki, M., et al. (2006). Functional analysis of rice DREB1/CBF-type transcription factors involved in cold-responsive gene expression in transgenic rice. Plant Cell Physiol. 47, 141–153. doi: 10.1093/pcp/pci230
Iwata, N., and Fujino, K. (2010). Genetic effects of major QTLs controlling low-temperature germinability in different genetic backgrounds in rice (Oryza sativa L.). Genome 53, 763–768. doi: 10.1139/g10-060
Jena, K., Kim, S., Suh, J., Yang, C., and Kim, Y. (2012). Identification of cold-tolerant breeding lines by quantitative trait loci associated with cold tolerance in rice. Crop Sci. 52, 517–523. doi: 10.2135/cropsci2010.12.0733
Kadambari, G., Vemireddy, L. R., Srividhya, A., Nagireddy, R., Jena, S. S., Gandikota, M., et al. (2018). QTL-Seq-based genetic analysis identifies a major genomic region governing dwarfness in rice (Oryza sativa L.). Plant Cell Rep. 37, 677–687. doi: 10.1007/s00299-018-2260-2
Kanehisa, M., Goto, S., Kawashima, S., Okuno, Y., and Hattori, M. (2004). The KEGG resource for deciphering the genome. Nucleic Acids Res. 32, 277–280. doi: 10.1093/nar/gkh063
Karabudak, T., Bor, M., Ozdemir, F., and Turkan, I. (2014). Glycine betaine protects tomato (Solanum lycopersicum) plants at low temperature by inducing fatty acid desaturase7 and lipoxygenase gene expression. Mol. Biol. Rep. 41, 1401–1410. doi: 10.1007/s11033-013-2984-6
Kim, S. H., Kim, J. Y., Kim, S. J., An, K. S., An, G., and Kim, S. R. (2007). Isolation of cold stress-responsive genes in the reproductive organs, and characterization of the OsLti6b gene from rice (Oryza sativa L.). Plant Cell Rep. 26, 1097–1110. doi: 10.1007/s00299-006-0297-0
Kumar, S., Agarwal, S., and Ranvijay. (2019). Fast and memory efficient approach for mapping NGS reads to a reference genome. J. Bioinformatics and Comput. Biol. 17:1950008. doi: 10.1142/s0219720019500082
Li, N., Zheng, H., Cui, J., Wang, J., Liu, H., Sun, J., et al. (2019). Genome-wide association study and candidate gene analysis of alkalinity tolerance in japonica rice germplasm at the seedling stage. Rice 12:24. doi: 10.1186/s12284-019-0285-y
Lin, J., Zhu, W. Y., Zhang, Y. D., Zhu, Z., and Wang, C. L. (2010). Detection of quantitative trait loci for cold tolerance at the bud bursting stage by using chromosome segment substitution lines in rice (Oryza sativa). Chin. J. Rice 24, 233–236. doi: 10.3724/SP.J.1011.2010.01385
Liu, C. T., Schlappi, M. R., Mao, B. G., Wang, W., Wang, A. J., Chu, C., et al. (2019). The bZIP73 transcription factor controls rice cold tolerance at the reproductive stage. Plant Biotechnol. J. 17, 1834–1849. doi: 10.1111/pbi.13104
Liu, G., Zhao, T., You, X., Jiang, J., Li, J., and Xu, X. (2019). Molecular mapping of the Cf-10 gene by combining SNP/InDel-index and linkage analysis in tomato (Solanum lycopersicum). BMC Plant Biol. 19:15. doi: 10.1186/s12870-018-1616-7
Lu, H. F., Lin, T., Klein, J., Wang, S. H., Qi, J. J., Zhou, Q., et al. (2014). QTL-seq identifies an early flowering QTL located near Flowering Locus T in cucumber. Theor. Appl. Genet. 127, 1491–1499. doi: 10.1007/s00122-014-2313-z
Lv, Y., Guo, Z., Li, X., Ye, H., Li, X., Xiong, L., et al. (2016). New insights into the genetic basis of natural chilling and cold shock tolerance in rice by genome-wide association analysis. Plant Cell Environ. 39, 556–570. doi: 10.1111/pce.12635
Ma, Q. Y., Zhang, J. Q., Lai, Q., Zhang, F., Dong, Z. H., A, L. S., et al. (2017). Temporal and spatial variations of extreme climatic events in Songnen Grassland, Northeast China during 1960-2014. J. Appl. Ecol. 28, 1769–1778. doi: 10.13287/j.1001-9332.201706.002
Magwene, P. M., Willis, J. H., and Kelly, J. K. (2011). The statistics of bulk segregant analysis using next generation sequencing. PLoS Comput. Biol. 7:e1002255. doi: 10.1371/journal.pcbi.1002255
Manangkil, O. E., Vu, H. T. T., Mori, N., Yoshida, S., and Nakamura, C. (2013). Mapping of quantitative trait loci controlling seedling vigor in rice (Oryza sativa L.) under submergence. Euphytica 192, 63–75. doi: 10.1007/s10681-012-0857-z
Mansfeld, B. N., and Grumet, R. (2018). QTLseqr: an R package for bulk segregant analysis with next-generation sequencing. Plant Genome 11:180006. doi: 10.3835/plantgenome2018.01.0006
Mao, D. H., Yu, L., Chen, D. Z., Li, L. Y., Zhu, Y. X., Xiao, Y., et al. (2015). Multiple cold resistance loci confer the high cold tolerance adaptation of Dongxiang wild rice (Oryza rufipogon) to its high-latitude habitat. Theor. Appl. Genet. 128, 1359–1371. doi: 10.1007/s00122-015-2511-3
Murray, M., and Thompson, W. F. (1980). Rapid isolation of high molecular weight plant DNA. Nucleic Acids Res. 8, 4321–4326. doi: 10.1093/nar/8.19.4321
Nair, P. M. G., Kang, I.-S., Moon, B.-Y., and Lee, C.-H. (2009). Effects of low temperature stress on rice (Oryza sativa L.) plastid ω-3 desaturase gene, OsFAD8 and its functional analysis using T-DNA mutants. Plant Cell Tissue Organ Cult. 98, 87–96. doi: 10.1007/s11240-009-9541-y
Najeeb, S., Ali, J., Mahender, A., Pang, Y., Zilhas, J., Murugaiyan, V., et al. (2020). Identification of main-effect quantitative trait loci (QTLs) for low-temperature stress tolerance germination-and early seedling vigor-related traits in rice (Oryza sativa L.). Mol. Breed. 40:10. doi: 10.1007/s11032-019-1090-4
Park, M., Lee, J.-H., Han, K., Jang, S., Han, J., Lim, J. H., et al. (2019). A major QTL and candidate genes for capsaicinoid biosynthesis in the pericarp of Capsicum chinense revealed using QTL-seq and RNA-seq. Theor. Appl. Genet. 132, 515–529. doi: 10.1007/s00122-018-3238-8
Qiao, Y., Han, L., An, Y., Cao, G., Gao, Y., and Koh, H. J. (2005). Molecular mapping of QTLs for cold tolerance at the bud bursting period in rice. Sci. Agric. Sin. 38, 217–221.
Saito, K., Hayano Saito, Y., Kuroki, M., and Sato, Y. (2010). Map-based cloning of the rice cold tolerance gene Ctb1. Plant Sci. 179, 97–102. doi: 10.1016/j.plantsci.2010.04.004
Schlappi, M. R., Jackson, A. K., Eizenga, G. C., Wang, A., Chu, C., Shi, Y., et al. (2017). Assessment of five chilling tolerance traits and GWAS mapping in rice using the USDA mini-core collection. Front. Plant Sci. 8:957. doi: 10.3389/fpls.2017.00957
Shen, X., Liu, B., Xue, Z., Jiang, M., Lu, X., Zhang, Q., et al. (2019). Spatiotemporal variation in vegetation spring phenology and its response to climate change in freshwater marshes of Northeast China. Sci. Total Environ. 666, 1169–1177. doi: 10.1016/j.scitotenv.2019.02.265
Shirasawa, S., Endo, T., Nakagomi, K., Yamaguchi, M., and Nishio, T. (2012). Delimitation of a QTL region controlling cold tolerance at booting stage of a cultivar,‘Lijiangxintuanheigu’, in rice, Oryza sativa L. Theor. Appl. Genet. 124, 937–946. doi: 10.1007/s00122-011-1758-6
Siahpoosh, M. R., Sanchez, D. H., Schlereth, A., Scofield, G. N., Furbank, R. T., van Dongen, J. T., et al. (2012). Modification of OsSUT1 gene expression modulates the salt response of rice Oryza sativa cv. Taipei 309. Plant Sci. 182, 101–111. doi: 10.1016/j.plantsci.2011.01.001
Song, J., Li, Z., Liu, Z., Guo, Y., and Qiu, L. J. (2017). Next-generation sequencing from bulked-segregant analysis accelerates the simultaneous identification of two qualitative genes in soybean. Front. Plant Sci. 8:919. doi: 10.3389/fpls.2017.00919
Su, C. F., Wang, Y. C., Hsieh, T. H., Lu, C. A., Tseng, T.-H., and Yu, S. M. (2010). A novel MYBS3-dependent pathway confers cold tolerance in rice. Plant Physiol. 153, 145–158. doi: 10.1104/pp.110.153015
Sun, J., Yang, L., Wang, J., Liu, H., Zheng, H., Xie, D., et al. (2018). Identification of a cold-tolerant locus in rice (Oryza sativa L.) using bulked segregant analysis with a next-generation sequencing strategy. Rice 11:24. doi: 10.1186/s12284-018-0218-1
Takagi, H., Abe, A., Yoshida, K., Kosugi, S., Natsume, S., Mitsuoka, C., et al. (2013). QTL-seq: rapid mapping of quantitative trait loci in rice by whole genome resequencing of DNA from two bulked populations. Plant J. 74, 174–183. doi: 10.1111/tpj.12105
Vikram, P., Swamy, B. P. M., Dixit, S., Ahmed, H. U., Cruz, M. T. S., Singh, A. K., et al. (2012). Bulk segregant analysis: “An effective approach for mapping consistent-effect drought grain yield QTLs in rice”. Field Crops Res. 134, 185–192. doi: 10.1016/j.fcr.2012.05.012
Wambugu, P., Ndjiondjop, M. N., Furtado, A., and Henry, R. (2018). Sequencing of bulks of segregants allows dissection of genetic control of amylose content in rice. Plant Biotechnol. J. 16, 100–110. doi: 10.1111/pbi.12752
Wang, K., Li, M., and Hakonarson, H. (2010). ANNOVAR: functional annotation of genetic variants from high-throughput sequencing data. Nucleic Acids Res. 38:e164. doi: 10.1093/nar/gkq603
Wang, X., Zou, B., Shao, Q., Cui, Y., Lu, S., Zhang, Y., et al. (2018). Natural variation reveals that OsSAP16 controls low-temperature germination in rice. J. Exp. Bot. 69, 413–421. doi: 10.1093/jxb/erx413
Wen, J., Jiang, F., Weng, Y., Sun, M., Shi, X., Zhou, Y., et al. (2019). Identification of heat-tolerance QTLs and high-temperature stress-responsive genes through conventional QTL mapping, QTL-seq and RNA-seq in tomato. BMC Plant Biol. 19:398. doi: 10.1186/s12870-019-2008-3
Win, K. T., Vegas, J., Zhang, C., Song, K., and Lee, S. (2017). QTL mapping for downy mildew resistance in cucumber via bulked segregant analysis using next-generation sequencing and conventional methods. Theor. Appl. Genet. 130, 199–211. doi: 10.1007/s00122-016-2806-z
Xu, Y., Hu, D., Hou, X., Shen, J., Liu, J., Cen, X., et al. (2020). OsTMF attenuates cold tolerance by affecting cell wall properties in rice. New Phytol. 227, 498–512. doi: 10.1111/nph.16549
Yang, J., Li, D., Liu, H., Liu, Y., Huang, M., Wang, H., et al. (2020). Identification of QTLs involved in cold tolerance during the germination and bud stages of rice (Oryza sativa L.) via a high-density genetic map. Breed. Sci. 70, 292–302. doi: 10.1270/jsbbs.19127
Yang, J., Zhong, W., Wang, J., Chen, Z., and Cao, Q. (2009). Identification of QTL for rice plant cold tolerance at early seedling stage. Genomics Appl. Biol. 28, 46–50.
Yang, R., Hong, Y., Ren, Z., Tang, K., Zhang, H., Zhu, J. K., et al. (2019). A role for PICKLE in the regulation of cold and salt stress tolerance in Arabidopsis. Front. Plant Sci. 10:900. doi: 10.3389/fpls.2019.00900
Yang, T., Zhang, S., Zhao, J., Liu, Q., Huang, Z., Mao, X., et al. (2016). Identification and pyramiding of QTLs for cold tolerance at the bud bursting and the seedling stages by use of single segment substitution lines in rice (Oryza sativa L.). Mol. Breed. 36:96. doi: 10.1007/s11032-016-0520-9
Yokotani, N., Sato, Y., Tanabe, S., Chujo, T., Shimizu, T., Okada, K., et al. (2013). WRKY76 is a rice transcriptional repressor playing opposite roles in blast disease resistance and cold stress tolerance. J. Exp. Bot. 64, 5085–5097. doi: 10.1093/jxb/ert298
Zhang, H., Dong, J., Zhao, X., Zhang, Y., Ren, J., Xing, L., et al. (2019). Research progress in membrane lipid metabolism and molecular mechanism in peanut cold tolerance. Front. Plant Sci. 10:838. doi: 10.3389/fpls.2019.00838
Zhang, M., Ye, J., Xu, Q., Feng, Y., Yuan, X., Yu, H., et al. (2018). Genome-wide association study of cold tolerance of Chinese indica rice varieties at the bud burst stage. Plant Cell Rep. 37, 529–539. doi: 10.1007/s00299-017-2247-4
Zhang, Q., Chen, Q. H., Wang, S. L., Hong, Y. H., and Wang, Z. L. (2014). Rice and cold stress: methods for its evaluation and summary of cold tolerance-related quantitative trait loci. Rice 7:24. doi: 10.1186/s12284-014-0024-3
Zhang, X., Wang, W., Guo, N., Zhang, Y., Bu, Y., Zhao, J., et al. (2018). Combining QTL-seq and linkage mapping to fine map a wild soybean allele characteristic of greater plant height. BMC Genomics 19:226. doi: 10.1186/s12864-018-4582-4
Zhang, Z., Li, J., Tang, Z., Sun, X., Zhang, H., Yu, J., et al. (2018). Gnp4/LAX2, a RAWUL protein, interferes with the OsIAA3–OsARF25 interaction to regulate grain length via the auxin signaling pathway in rice. J. Exp. Bot. 69, 4723–4737. doi: 10.1093/jxb/ery256
Zhang, Z. Y., Li, J., Pan, Y., Li, J., Zhou, L., Shi, H., et al. (2017). Natural variation in CTB4a enhances rice adaptation to cold habitats. Nat. Commun. 8:14788. doi: 10.1038/ncomms14788
Zhao, J., Wang, S., Qin, J., Sun, C., and Liu, F. (2020). The lipid transfer protein OsLTPL159 is involved in cold tolerance at the early seedling stage in rice. Plant Biotechnol. J. 18, 756–769. doi: 10.1111/pbi.13243
Zhao, J., Zhang, S., Dong, J., Yang, T., Mao, X., Liu, Q., et al. (2017). A novel functional gene associated with cold tolerance at the seedling stage in rice. Plant Biotechnol. J. 15, 1141–1148. doi: 10.1111/pbi.12704
Zhao, M., Leng, Y., Chao, S., Xu, S. S., and Zhong, S. (2018). Molecular mapping of QTL for Fusarium head blight resistance introgressed into durum wheat. Theor. Appl. Genet. 131, 1939–1951. doi: 10.1007/s00122-018-3124-4
Zhou, L., Zeng, Y., Hu, G., Pan, Y., Yang, S., You, A., et al. (2012). Characterization and identification of cold tolerant near-isogenic lines in rice. Breed. Sci. 62, 196–201. doi: 10.1270/jsbbs.62.196
Keywords: Oryza sativa L., cold tolerance, bulk segregant analysis sequencing, linkage mapping, bud-bursting
Citation: Yang L, Lei L, Li P, Wang J, Wang C, Yang F, Chen J, Liu H, Zheng H, Xin W and Zou D (2021) Identification of Candidate Genes Conferring Cold Tolerance to Rice (Oryza sativa L.) at the Bud-Bursting Stage Using Bulk Segregant Analysis Sequencing and Linkage Mapping. Front. Plant Sci. 12:647239. doi: 10.3389/fpls.2021.647239
Received: 29 December 2020; Accepted: 22 February 2021;
Published: 11 March 2021.
Edited by:
Felipe Klein Ricachenevsky, Federal University of Rio Grande do Sul, BrazilReviewed by:
Jieyun Zhuang, Zhejiang University, ChinaYongzhong Xing, Huazhong Agricultural University, China
Copyright © 2021 Yang, Lei, Li, Wang, Wang, Yang, Chen, Liu, Zheng, Xin and Zou. This is an open-access article distributed under the terms of the Creative Commons Attribution License (CC BY). The use, distribution or reproduction in other forums is permitted, provided the original author(s) and the copyright owner(s) are credited and that the original publication in this journal is cited, in accordance with accepted academic practice. No use, distribution or reproduction is permitted which does not comply with these terms.
*Correspondence: Detang Zou, zoudtneau@126.com
†These authors have contributed equally to this work