- 1Soybean Research Institute/MARA National Center for Soybean Improvement/MARA Key Laboratory of Biology and Genetic Improvement of Soybean/National Key Laboratory for Crop Genetics and Germplasm Enhancement/Jiangsu Collaborative Innovation Center for Modern Crop Production, Nanjing Agricultural University, Nanjing, China
- 2Department of Crop Science, Faculty of Agriculture, Food and Consumer Sciences, University for Development Studies, Tamale, Ghana
Soybean pubescence plays an important role in insect resistance, drought tolerance, and other stresses. Hence, a deep understanding of the molecular mechanism underlying pubescence is a prerequisite to a deeper understanding of insect resistance and drought tolerance. In the present study, quantitative trait loci (QTL) mapping of pubescence traits was performed using a high-density inter-specific linkage map of one recombinant inbred line (RIL) population, designated NJRINP. It was observed that pubescence length (PL) was negatively correlated with pubescence density (PD). A total of 10 and 9 QTLs distributed on six and five chromosomes were identified with phenotypic variance (PV) of 3.0–9.9% and 0.8–15.8% for PL and PD, respectively, out of which, eight and five were novel. Most decreased PL (8 of 10) and increased PD (8 of 9) alleles were from the wild soybean PI 342618B. Based on gene annotation, Protein ANalysis THrough Evolutionary Relationships and literature search, 21 and 12 candidate genes were identified related to PL and PD, respectively. In addition, Glyma.12G187200 from major QTLs qPL-12-1 and qPD-12-2, was identified as Ps (sparse pubescence) before, having an expression level of fivefold greater in NN 86-4 than in PI 342618B, hence it might be the candidate gene that is conferring both PL and PD. Based on gene expression and cluster analysis, three and four genes were considered as the important candidate genes of PL and PD, respectively. Besides, leaves with short and dense (SD) pubescence, which are similar to the wild soybean pubescence morphology, had the highest resistance to common cutworm (CCW) in soybean. In conclusion, the findings in the present study provide a better understanding of genetic basis and candidate genes information of PL and PD and the relationship with resistance to CCW in soybean.
Introduction
Plants are sessile in nature, therefore, they are exposed to various abiotic and biotic stresses, such as drought, chilling injury, insects, and diseases attack (Zhu, 2016). Pubescence in plants offers the opportunity for them to withstand a number of stresses. Trichomes, the epidermal outgrowths with single-cell or multicellular structures covering most aerial plant tissues, are present in the enormous number of plant species (Huchelmann et al., 2017), playing extremely important roles in plant growth and development, such as protecting plants from herbivore attacks and pathogens (Hanley et al., 2007; Bickford, 2016), protecting against damaging ultraviolet (UV) radiation, avoiding excessive transpiration (Manetas, 2003; Pattanaik et al., 2014; Matias-Hernandez et al., 2016), and so on. In addition, the pubescence of single cell could be used as a model to research cell differentiation and fate (Hulskamp, 2004; Serna, 2004; Yang and Ye, 2013). Hence, it is of great significance to have a deeper understanding of the genetic basis and regulatory network of pubescence development.
In soybean, pubescence is single stalked and covers almost all aerial organs except cotyledons and hypocotyls (Liu et al., 2020). Previous research proved that pubescence density (PD) of soybean is related to some agronomic traits, such as yield, insect resistance, plant height, and evapotranspiration rates (Ghorashy et al., 1971; Singh et al., 1971; Specht et al., 1985; Clawson et al., 1986; Lam and Pedigo, 2001). Insect damage is one of the most serious stresses in crops, such as soybean, in view of this, many farmers use biotechnology-engineered crops, insecticidal seed treatments, soil-applied insecticides, and foliar sprays to manage insects (Chang and Hartman, 2017; Hurley and Mitchell, 2020). Pubescence on the surface of soybean plays an indispensable role in insect resistance (Oki et al., 2012). In the past, many researchers have studied the relationship between different pubescence morphology and insect resistance. For instance, dense pubescence and extra-dense pubescence can provide resistance to soybean mosaic virus by limiting the spread of aphid vectors (Gunasinghe et al., 1988). It has also been found that regardless of PD, soybean with long and erect pubescence has higher resistance to potato leafhopper (PLH) than their counterparts with short and close pubescence (Turnipseed, 1977). In addition, soybean variety “Camp” with low trichome density on the abaxial surface of soybean trifoliate was less attractive to Megacopta cribraria, and further research revealed that “Camp” also exhibited antibiosis by suppressing nymph development (Lahiri et al., 2020a,b). To date, a number of quantitative trait loci (QTL) for insect resistance have been reported in soybean and some colocalized with pubescence-related traits, for example, the PLH locus is close but distinct to a PD QTL on the chromosome (Chr) 12 (Chang and Hartman, 2017). The pubescence QTLs on Chr07 and Chr12 were located near the antixenosis resistance QTLs: qRslx1 and qRslx2, respectively (Oki et al., 2012).
Many statistical methods have been developed for QTL detection with composite interval mapping (CIM) as one of the most widely used methods. CIM was proposed to combine interval mapping and multiple regression analysis (Zeng, 1993). Molecular markers were used to limit genetic background effects, thereby, reducing false positives and improving mapping accuracy. However, there are some limitations, among them including its inability to analyze epistatic QTLs, additive by additive, and additive by environment interactions. To resolve the above shortcomings, mixed model-based composite interval mapping (MCIM) was proposed by Zhu and Weir (1998). This method takes population phenotypic mean and various main genetic effects (additive effect, dominant effect, and epistatic effect) of QTL as fixed effects, and the markers, environment, markers environment interaction effect as random effects. QTL mapping analysis and effect value estimation were combined for joint QTL analysis in multiple environments, to improve the accuracy and efficiency of QTL mapping.
Research to uncover inheritance of soybean pubescence started about a century ago, three dominant mutants named P1 (glabrous), Ps (sparse pubescence), and Pd (dense pubescence) were found related to PD (Owen, 1927; Bernard and Singh, 1969). Then these three genes were mapped on Chr01 (Pd1) (Cregan et al., 1999), Chr12 (Ps) (Specht et al., 2001; Bandillo et al., 2017), and Chr09 (P1) (Bandillo et al., 2017). With the rapid development of sequencing technology and data statistics, several QTLs related to pubescence length (PL) and PD were identified in the past decades. Two QTLs of PL (on Chr07 and Chr12) and two QTLs of PD (on Chr01 and Chr12) were identified using a recombinant inbred population (Oki et al., 2012). A major QTL on Chr12 and some other minor QTLs on Chr01, Chr02, Chr07, Chr08, Chr09, and Chr15 of PD were identified using a recombinant inbred line (RIL) population that derived from a cross between soybean cultivars Kefeng 1 and Nannong 1138-2 (Du et al., 2009). Two and four QTLs related to PL and PD were mapped on Chr01, Chr12, and Chr01, Chr08, Chr12, and Chr20, respectively (Xing et al., 2013).
Genetic and molecular studies of the past years have shown that pubescence formation is regulated in a complex and precise way (Pattanaik et al., 2014). A large number of transcription factors (TFs) that regulate trichome development had been identified (Ishida et al., 2008). In recent years, availability of user-friendly genomic resources and easy-to-use bioinformatics tools, a number of studies have been conducted and some candidate genes identified with validation (Nakaya et al., 2020). The function of GmCPR5 (ortholog of Arabidopsis CPR5) involved in pubescence development was tested by CRISPR/Cas9 (Campbell et al., 2019). Recently, genes responsible for the classic loci Pd1, Ps, and P1 were cloned, and further analysis validated that these three genes can form a complex feedback network to precisely regulate pubescence formation in soybean (Liu et al., 2020).
With the well-established molecular technology and genetic transformation in soybean, genes responsible for pubescence and their function have been gradually clarified; however, there still exists limited knowledge on the molecular basis for pubescence development and regulatory pathways. Pubescence is controlled by major genes and polygenes; therefore, it is very difficult to identify these genes through conventional methods. Most populations used in previous studies were derived from cultivated parents with relatively narrow phenotypic differences, hence making it difficult to uncover the genetic information from wild soybean (Du et al., 2009).
In the present study, an inter-specific RIL population, which is derived from a cultivated soybean (Glycine max Merr.) (Nannong 86-4, NN 86-4) and a wild soybean line (Glycine soja) (PI 342618B), was used. The female parent NN 86-4 has long and sparse pubescence, while the male parent PI 342618B has SD pubescence. The present study aimed to uncover the genetic architecture of PL and PD, to predict potential candidate genes, and to analyze the relationship between pubescence morphology and common cutworm (CCW; Spodoptera litura Fabricius) resistance.
Materials and Methods
Plant Material and Growth Conditions
The NJRINP contains 284 lines derived via single seed descent. All the 284 RILs along with their parents were planted in two environments viz. Jiangpu Experimental Station, Nanjing, Jiangsu Province (Latitude 33°03′ N; Longitude 118°63′ E) in 2011 (JP2011) and Baima Experimental Station, Nanjing, Jiangsu Province (Latitude 31°62′ N; Longitude 119°18′ E) in 2020 (BM2020). Each line was planted in one-row plot (length × width, 1.5 × 1 m). Field management followed standard conditions in each location.
Phenotypic Analysis of Pubescence Length and Density
The third leaf from the top of each stem was taken from three plants in the field at V6 stage, then put in icebox and transported to the laboratory. Samples were dissected between the main vein and lateral vein near the base of the middle-leaflet of trifoliolate with 8 mm diameter puncher (avoiding primary veins) (Xing et al., 2013). Then the leaf discs were used to take photographs with an area of 12 mm−2 under a Leica stereo microscope. The software ImageJ1 was used to generate PL and PD. PD was converted from 12 mm−2 to an area of 10 mm−2 as the final density. As for PL, the pubescence on leaf surface was divided into two types: long and short, the length of three representative hairs of each type was measured, and average length was calculated by the weighted average method as
Where is a weighted average of PL, is the average length of three representative long pubescence, fl is the number of long pubescence, is the average length of three representative short pubescence, and fs is the number of short pubescence.
Statistical Analysis of Phenotypic Data
R software was used to draw the frequency distribution of phenotypic data. The descriptive statistics, such as mean, maximum and minimum, coefficient of variation (CV), correlation analysis, and ANOVA of traits were calculated using SAS software (SAS Institute, 2010. SAS/STAT software version 9.2. SAS Institute Inc., Cary, NC, United States). The broad-sense heritability (h2) for individual environments (Eq. 1) and combined environments (CE; Eq. 2) were computed following the formula proposed by Nyquist and Baker (1991).
Where is the genotypic variance, is the phenotypic variance (PV), is the genotype by environment interaction variance, is the error variance, n is the number of environments, and r is the number of replications.
Genetic Linkage Map Construction and Quantitative Trait Loci Mapping Analysis
In the present study, a high-density genetic linkage map was constructed using restriction site-associated DNA sequencing (RAD-seq) (Wang et al., 2016). Briefly, restriction enzymes were used to digest the purified genomic DNA firstly, then ligated digested products with P1 adapter by T4 DNA ligase. Every 24 RILs were collected together and randomly sheared ultrasonically and used a purification kit to purify DNA fragments. Next, the fragment end was repaired with a Quick Blunting kit (NEB). Finally, the collected fragments were enriched by PCR amplification and purified by a QIAquick PCR purification kit. In addition, standardized samples were sequenced on HiSeq 2000 instruments. The soybean genome sequence (G. max, Wm82.a1. v1) was used as a reference to predict digestion sites. A total of 5,728 bin markers were obtained from 89,680 single nucleotide polymorphisms, spanning a total genetic distance of 2,204.6 cM with an average distance of 0.4 cM between neighboring bins. The linkage map of bin markers was constructed for the RIL population using R with the package LinkageMapView.
Two QTL mapping models were adopted to map additive effect QTLs in the present study to discover the genetic basis of pubescence development. Firstly, CIM was implemented in WinQTLCart 2.5 software with a 10 cM window at a walking speed of 1 cM to map additive effect QTLs. The log of odd (LOD) threshold was determined by 1,000 permutation tests for each trait with an experimental-wise error rate of P = 0.05 to determine whether the QTL was significant. The QTLs detected with overlapping or closely linked confidence intervals (CIs) in different environments were recognized as the same QTL.
Secondly, QTL Network v2.0 software with MCIM model was used to map additive effect QTLs with the critical F-value calculated with 1,000 permutation tests. In addition, the QTL effects were estimated using the Markov Chain Monte Carlo (MCMC) method with 20,000 Gibbs sampler iterations. The significance level configuration of candidate interval selection, putative QTL detection, and QTL effects were calculated with an experiment-wise type I error under α = 0.05. The above analyses were done for individual environments (JP2011 and BM2020), averages from JP2011 and BM2020 were designated as the CE.
Candidate Gene Prediction and Quantitative Real-Time PCR Analysis
The physical position of two flanking markers of major QTLs can be obtained by mapping sequencing data to Wm82.a1. v1. Both the model genes and annotation information within the physical genomic interval of major QTLs were obtained from SoyBase.2 The expression data of model genes were downloaded from SoyBase3 and Phytozome.4 The genes that expressed in young leaf were further classified according to Protein Analysis THrough Evolutionary Relationships (PANTHER).5 Based on functional annotations, PANTHER analysis, and available literatures, some genes were selected as candidate genes and their relative expression levels available on SoyBase and Phytozome were heatmapped using TBtools (Chen et al., 2020).
To perform qRT-PCR, total RNA of leaf samples of two parents (NN 86-4 and PI 342618B) were isolated using RNA-prep Pure Plant Kit (TIANGEN DP-432, China) and full-length cDNA was reverse transcribed using a cDNA synthesis kit (Vazyme, R223) according to the protocol of the manufacturer. qRT-PCR was performed using ChamQ SYBR qPCR Master mix (Vazyme Q311) on Roche LightCycler 480 II. The housekeeping gene GmActin11 was used as the reference. Three biological replicates were conducted for each analysis. The relative expressions of selected genes were computed using a 2–△CT method (Livak and Schmittgen, 2001). The primer sequences for qRT-PCR are listed in Supplementary Table 1. The cluster analysis was performed using the Neighbor-Joining method in MEGA6 (Tamura et al., 2013). The percentage of replicate trees in which the associated taxa clustered together in the bootstrap test (1,000 replicates) are shown next to the branches.
Resistance Evaluation to Common Cutworm
Common cutworm pupa stock was obtained from Soybean Research Institute of Nanjing Agriculture University. Third-instar larvae with uniform size were used for the experiment as described by Xing et al. (2017). The third leaf from the top of the stem of four kinds of pubescence morphology [long and sparse (LS); short and sparse (SS); long and dense (LD); and short and dense (SD)] was used to feed CCW larvae (five larvae per replication), and 10 different representative lines were selected for each pubescence morphology (PL/PD class). This experiment was conducted with three biological replicates per line. The initial larval weight was recorded and measured 3 days after forcibly feeding. The increased larval weight was subjected to ANOVA as a resistance indicator and multiple comparisons of different pubescence morphology (LS, SS, LD, and SD) were conducted via the least significant difference at 5% probability in SAS software.
Results
Phenotypic Analysis of Pubescence Length and Density
PL and PD of 284 RILs and their parents in JP2011, BM2020, and CE are presented in Figure 1. PL and PD of the male parent PI 342618B were ranged 0.18–0.33 mm and 54.53–90.83 hairs 10 mm−2, respectively, which were shorter and denser than that of the female parent NN 86-4 (0.45–0.58 mm and 17.22–21.67 hairs 10 mm−2) (Table 1 and Figure 1). The variation between two parents offered a broader genetic resource among the RILs for quantitative trait analysis. The mean value of some RILs exceeded two parents in both directions, indicating that RILs showed transgressive segregation in PL and PD (Figure 1). The phenotypic variation of PL and PD among RILs showed continuous distribution, suggesting both two traits are controlled by multiple genes, and thus suitable for QTL mapping.
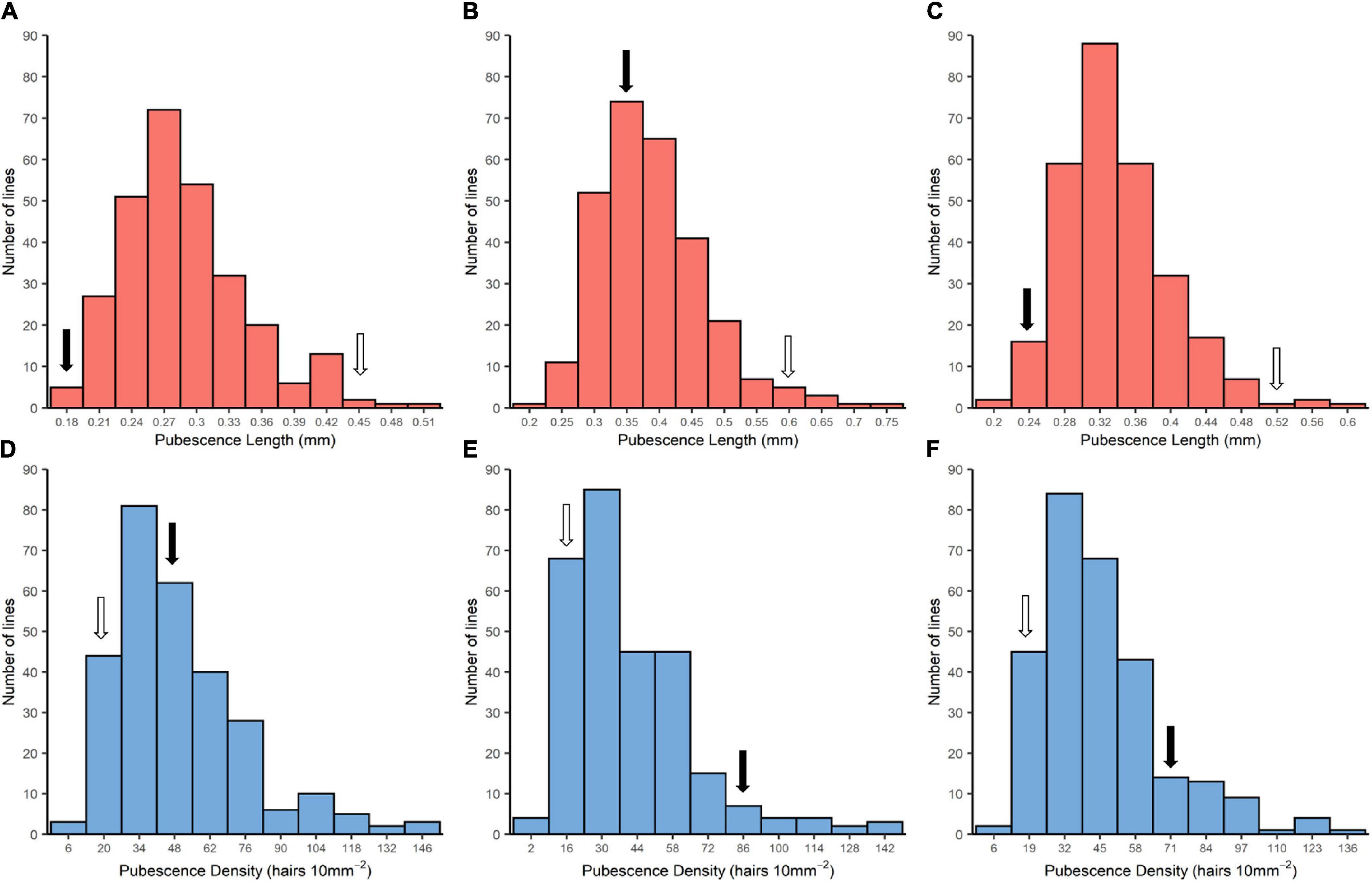
Figure 1. Frequency distribution of pubescence length (PL) and pubescence density (PD) of the RIL population NJRINP and parents. RIL, recombinant inbred line. (A–C) Frequency distribution of PL of JP2011, DT2020, and combined environment, respectively. (D–F) Frequency distribution of PD of JP2011, DT2020, and combined environment, respectively. The black arrow represents the wild soybean PI 342618B and the white arrow represents the cultivar NN 86-4.
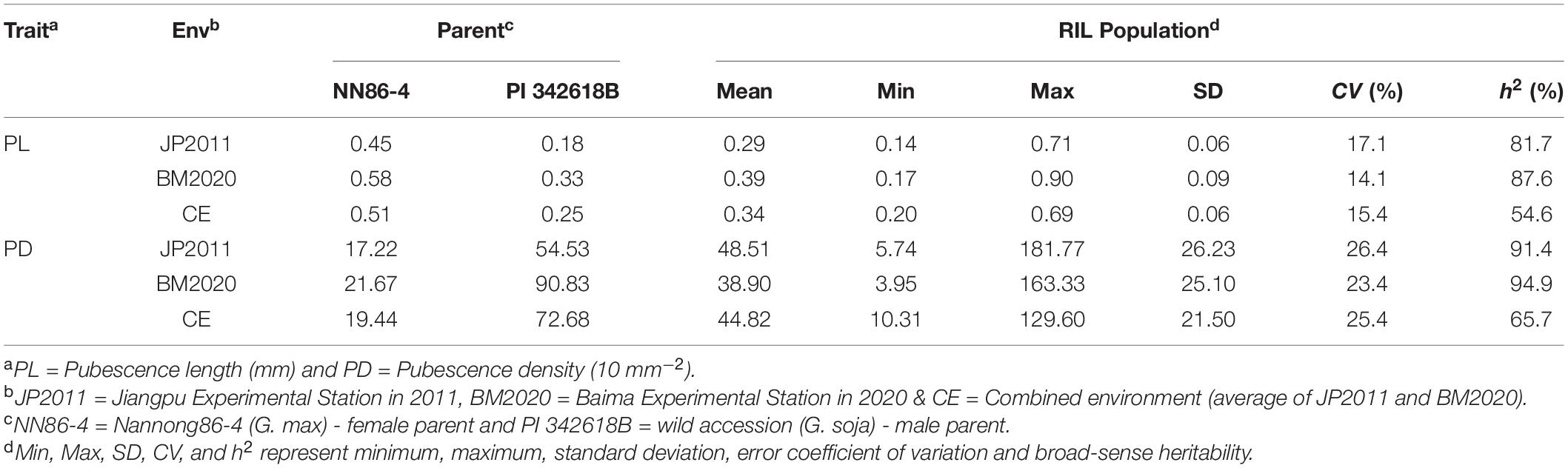
Table 1. Descriptive statistics, broad-sense heritability for pubescence length and density in the RIL population NJRINP and parents.
In addition to the above, ANOVA and correlation analysis suggested that both traits (PL and PD) were influenced by environment and genotype by environment interaction (G × E) (Table 1, Supplementary Table 2, and Figure 1). The high h2 of the two traits (PL and PD) indicated that either of the traits is largely regulated by genetic factors. The correlation coefficients (r) of the same trait in different environments were ranged from 0.40 to 0.49, but a negative correlation was observed between PL and PD (r = −0.17 to −0.36; Supplementary Figure 1).
Quantitative Trait Loci Mapping of Pubescence Length and Density by Composite Interval Mapping Method
The bin-marker distribution on each chromosome is shown in Supplementary Table 3 and Supplementary Figure 2. A total of 16 QTLs comprising nine and seven for PL and PD, respectively, were identified by the CIM model with LOD (3.4–14.0) and phenotypic variation explained (R2) (4.2%–15.8%) (Table 2). The highest number of seven QTLs (qPL-12-1, qPL-12-2, qPL-12-3, qPL-12-4, qPD-12-1, qPD-12-2, and qPD-12-3) was mapped on Chr12 followed by three QTLs (qPL-1-1, qPL-1-2, and qPD-1-1) on Chr01, two QTLs (qPD-11-1, qPD-11-2) on Chr11 (Table 2 and Figure 2). These results suggested that PL and PD are largely controlled by Chr12, Chr01, and Chr11.
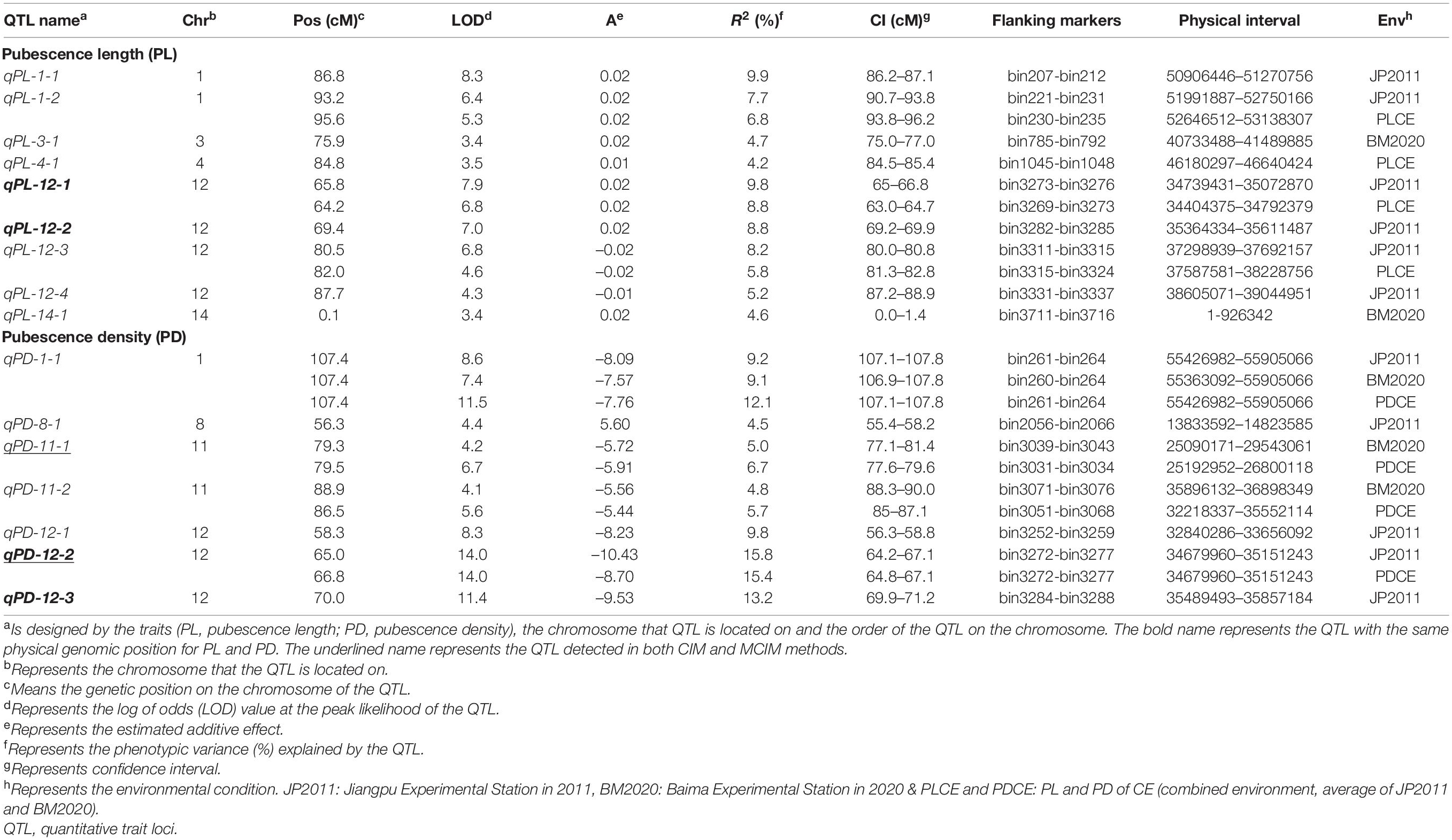
Table 2. The QTLs identified for pubescence length and density in the inter-specific RIL population (NJRINP) with composite interval mapping (CIM) model.
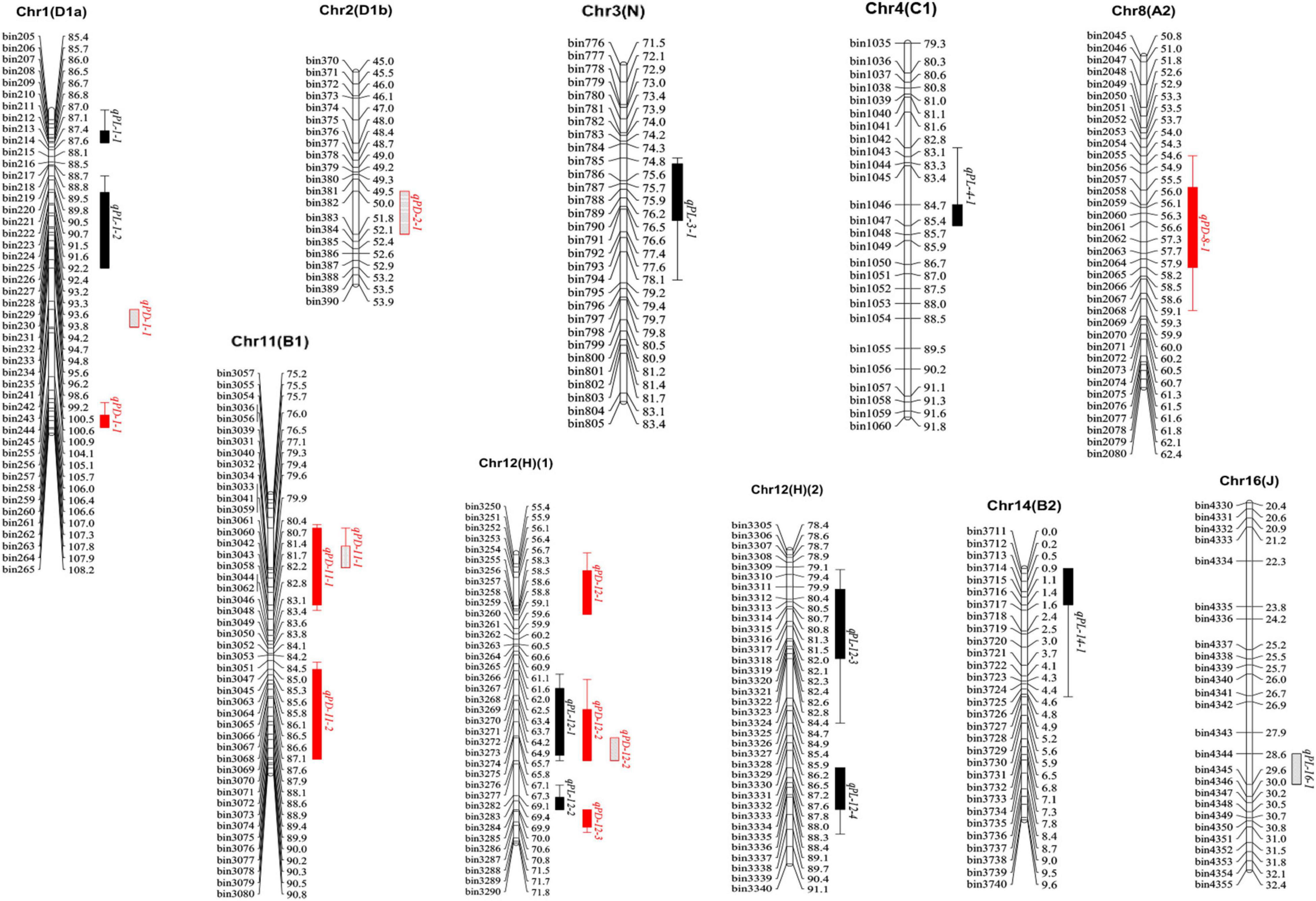
Figure 2. Locations of QTLs on genetic linkage map for pubescence length (PL) and pubescence density (PD). Black graphics represent the QTLs were responsible for PL. Red graphics represent the QTL were responsible for PD, and the filled graphics represent QTLs detected by the CIM method, while the hollow graphics represent QTLs detected by the MCIM method (Due to the high-density markers, this figure only shows the region where the QTL is located, the complete map is shown in Supplementary Figure 2). QTL, quantitative trait loci; CIM, composite interval mapping; MCIM, mixed model-based composite interval mapping.
For PL, nine QTLs were identified on five chromosomes (Chr01, Chr03, Chr04, Chr12, and Chr14) with phenotypic variation ranging from 4.2 to 9.9% and LOD ≥ 3.4 (Table 2 and Figure 2). Among these, QTLs qPL-1-2, qPL-12-1, and qPL-12-3 were detected in JP2011 and CE with LOD values of 5.3–6.4, 6.8–7.9, and 4.6–6.8, R2 values of 6.8–7.7%, 8.8–9.8%, and 5.8–8.2%, respectively (Table 2). qPL-1-1 was detected in JP2011 and accounted for an R2 value of 9.9%. The remaining five QTLs have relatively smaller R2 (4.2–8.8%). Aside from the additive effect of qPL-12-3 and qPL-12-4 that are negative, alleles for increased PL emanated from wild soybean PI 342618B, while others obtained increased PL additive effect from NN 86-4 (Table 2).
The QTLs related to PD were identified on four chromosomes (Chr01, Chr08, Chr11, and Chr12) with LOD scores ranging from 4.1 to 14.0, which could explain phenotypic variation from 4.5 to 15.8% (Table 2 and Figure 2). Among them, qPD-1-1 could be detected in both environments and CE with LOD_vs_R2 values of 7.4–11.5_vs_9.1%–12.1%, qPD-11-1, qPD-11-2, and qPD-12-2 could be detected in one environment and CE with LOD_vs_R2 values of 4.2–6.7_vs_5.0–6.7%, 4.1–5.6_vs_4.8–5.7%, and 14.0_vs_15.4–15.8%, respectively. Besides, qPD-12-3 was detected in only one environment (JP2011) but caused the highest R2 of 13.2%. The remaining two QTLs (qPD-8-1 and qPD-12-1) were detected in single environment and could explain relatively lower phenotypic variation (Table 2). Interestingly, except qPD-8-1 with a positive additive allele that increased PD allele from NN 86-4, all additive effects of other QTLs were negative with alleles for increasing PD from PI 342618B, suggesting PD is a domestication related trait which may have been lost or reduced during domestication. Hence the wild soybean PI 342618B contains beneficial alleles which could be exploited to increase PD in its domesticated progenies (Table 2).
By comparing QTLs for PL and PD, qPL-12-1 and qPD-12-2, qPL-12-2 and qPD-12-3 overlapped, respectively. These four QTLs were flanked by markers bin3269-bin3276, bin3272-bin3277, bin3282-bin3285, and bin3284-bin3288, respectively (Table 2 and Figure 2). The increased pubescence traits alleles of PL and PD were from different parents, hence they could be the same QTL with pleiotropic effect, and explain the negative correlation between PL and PD.
Additive Quantitative Trait Loci Conferring Pubescence Length and Density Detected by Mixed Model-Based Composite Interval Mapping Method
In all, one additive QTL (qPL-16-1) of PL and four additive QTLs (qPD-1-2, qPD-2-1, qPD-11-1, and qPD-12-2) of PD were detected by MCIM implemented in QTL Network v2.0 software.
For PL, qPL-16-1 could cause phenotypic variation of 3.0%. It is a novel locus detected for the first time with an increased PL additive effect from NN 86-4 (Table 3). A total of four QTLs were detected related to PD accounted for 0.8%–12.8% phenotypic variation, among which qPD-12-2 could explain the phenotypic variation of 12.8%. All the QTLs inherited their increased PD alleles from PI 342618B (Table 3), supporting our earlier assertion that pubescence may be one of the domestication syndrome traits in soybean. qPL-12-1 and qPD-12-2 by CIM were overlapped with qPD-12-2 by MCIM (Tables 2, 3), hence this locus was considered as the major QTL for pubescence development in the present panel. In addition, qPD-11-1 was detected by CIM and MCIM, respectively (Tables 2, 3). Therefore this region was considered as major QTL for regulating PD in this population.
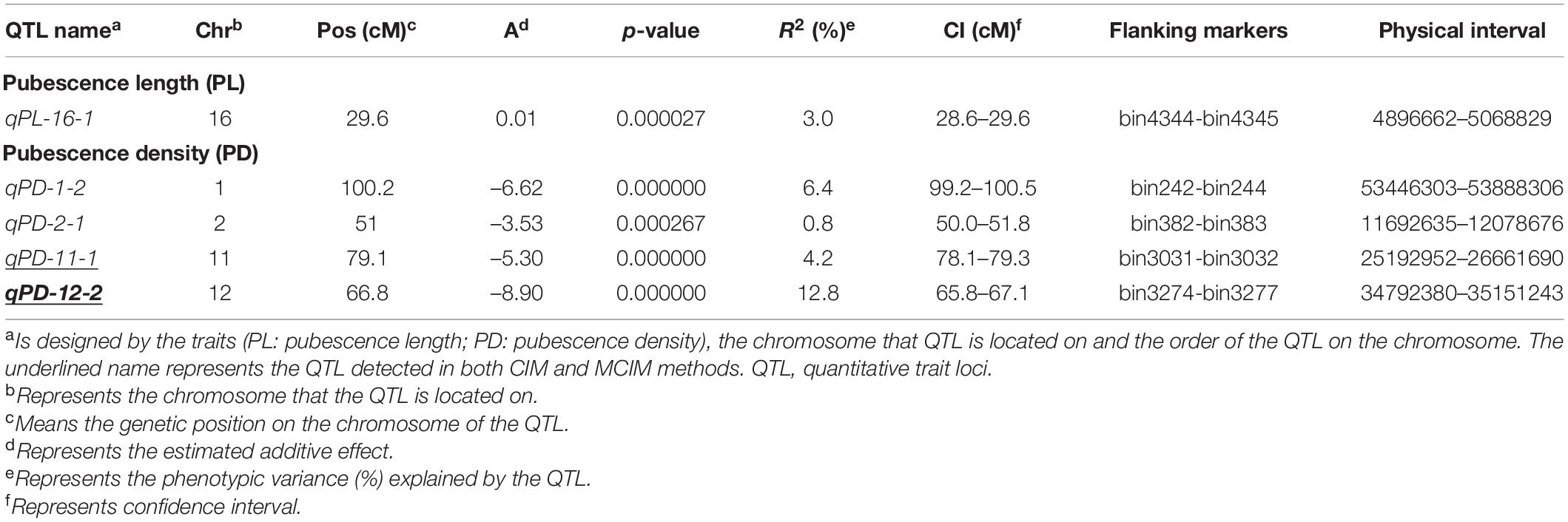
Table 3. The additive QTLs identified for pubescence length and density in the inter-specific RIL population (NJRINP) with the mixed model-based composite interval mapping (MCIM) method.
Candidate Gene Screening of Pubescence Length and Density Within Major Quantitative Trait Loci
For PL: qPL-1-2, qPL-12-1, and qPL-12-3 were detected in JP2011 and CE, which were considered as major QTLs. These three QTLs contain 131, 60, and 80 genes (total 271), respectively (Wm82.a1. v1). For PD, qPD-1-1 was mapped in both JP2011 and BM2020 and CE, while qPD-11-1 and qPD-12-2 were detected by both CIM and MCIM. Besides, qPD-12-3 with the LOD value of greater than 10 caused 13.2% phenotypic variation in PD and overlapped with Pubescence density 2-7 (Du et al., 2009), hence this could be considered as a major QTL. qPD-11-2 was not considered as a major QTL due to lower phenotypic variation. Within the genomic regions of qPD-1-1, qPD-11-1, qPD-12-2, and qPD-12-3, 70, 75, 41, and 39 model genes (total 225) were downloaded, respectively.
A total 247 of 271 genes for PL and 200 of 225 genes for PD were informatively annotated, respectively (Supplementary Tables 4, 5). Expression data of distinct tissues in soybean have been completed in previous research (Severin et al., 2010). A total 203 and 151 genes of PL and PD with expression in young leaf were selected for PANTHER analysis (Wm82.a2. v1) (Supplementary Tables 6, 7). For PL, 93 out of the 203 genes were included in PANTHER protein classes and involved in 14 pathways. Then 17 out of the 93 genes were considered as the candidate genes according to literatures and annotation information (Table 4). Furthermore, there were other four candidate genes that did not include in protein classes (Table 4). For PD, 70 out of the 151 genes were included in PANTHER protein classes and involved in nine pathways. A total of nine genes were considered as the candidate genes according to literatures and annotation information. Except for the above genes, three candidate genes were not included in protein classes (Table 4). Among above 21 and 12 candidate genes of PL and PD, respectively, Glyma.12g185500, Glyma.12g188600, and Glyma.12g188800 were responsible for both PL and PD, suggesting a possible pleiotropic effect of some candidate genes.
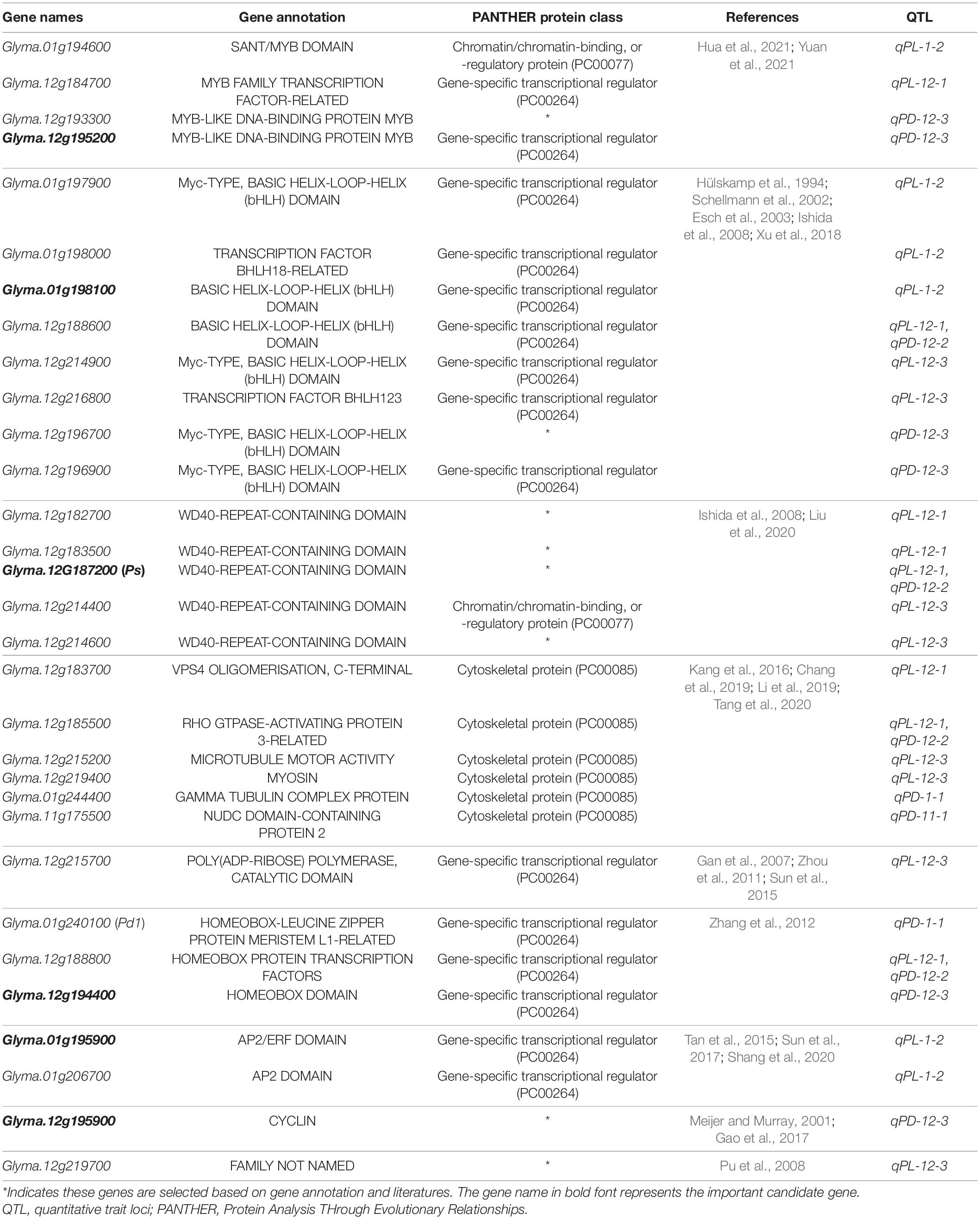
Table 4. Candidate genes within major QTL regions identified based on gene annotation, PANTHER analysis, and available literatures.
Most of these 30 (three genes are the same for both PL and PD) candidate genes with relative higher expression in young leaf or shoot apical meristem (SAM) (Supplementary Table 8 and Figure 3). Their expression was measured subsequently by qRT-PCR in the leaves of two parents: PI 342618B and NN 86-4 (Figures 4A,B). Eight and five genes of PL and PD, respectively, were expressed differentially by more than 2-fold between two parents. For PL, Glyma.01g198100 expressed more than 50 folds higher in NN 86-4 than PI 342618B (Figure 4A). For PD, Glyma.12g195900 has an expression level of more than 9-fold in PI 342618B compared with NN 86-4 (Figure 4B). Also, Glyma.12g195900 is the homologous gene of CYCU1 which could promote meristem cell division in Arabidopsis (Peng et al., 2014). Therefore, Glyma.01g198100 and Glyma.12g195900 were considered as the important candidate genes for PL and PD, respectively.
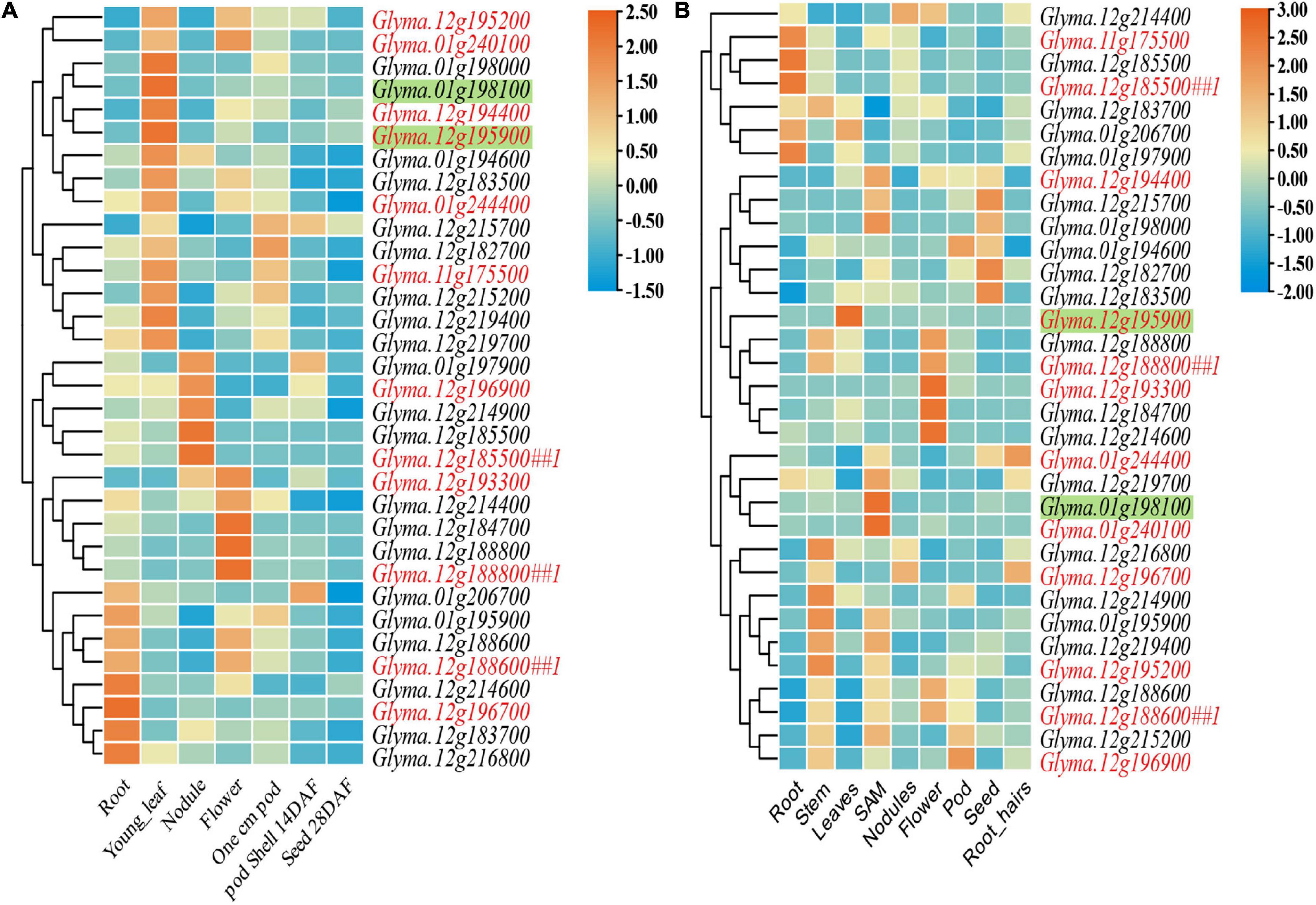
Figure 3. Heatmap of expression data from SoyBase (A) and Phytozome (B) for 21 and 12 candidate genes of pubescence length (PL) and pubescence density (PD). The candidate gene names of PL and PD are in black and red, respectively. The gene name with ##1 represents the candidate gene for both PL and PD. The gene name with shadow represents the important candidate genes identified by differential expression in two parents.
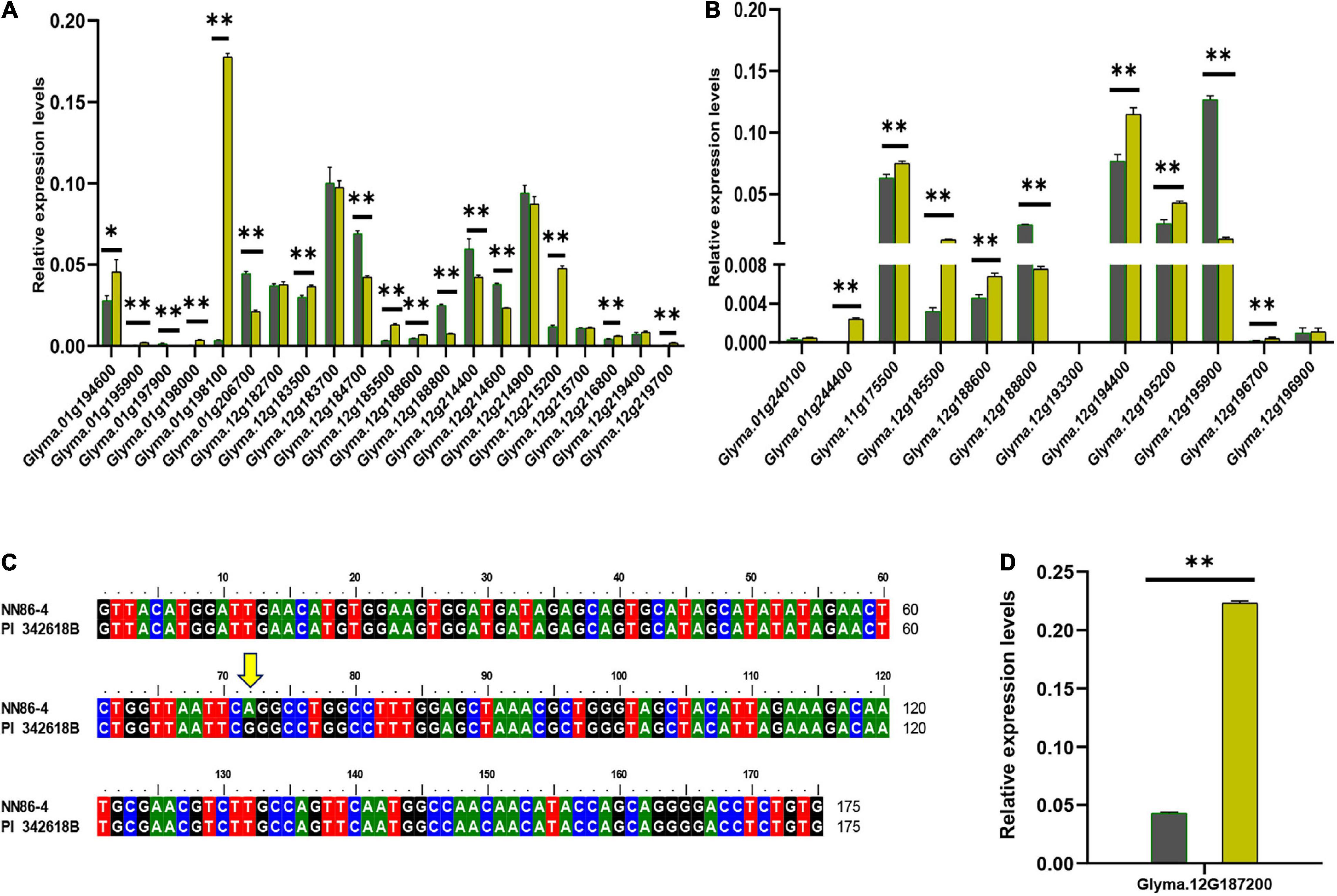
Figure 4. Difference analysis of candidate genes of pubescence length (PL) and pubescence density (PD). (A,B) The expression levels of 21 and 12 candidate genes of PL (A) and PD (B), respectively, in parents PI 342618B (black column) and NN 86-4 (yellow column). (C) DNA sequence alignment of the seventh exon of Glyma.01g240100 (Pd1) in two parents. The yellow arrow represents the SNP between two parents. (D) Expression analysis of Glyma.12G187200 (Ps) in parents PI 342618B (black column) and NN 86-4 (yellow column) (*P ≤ 0.05; **P ≤ 0.01).
Glyma.01g240100, one of the 80 model genes of qPD-1-1, has been identified as Pd1 due to a T to C single nucleotide polymorphism (SNP) in the last exon (Liu et al., 2020). Only one G to A synonymous mutation was detected in the seventh exon between PI 342618B and NN 86-4 in the present study (Figure 4C and Supplementary Figure 3). Glyma.12G187200, a candidate gene of major QTL qPL-12-1 and qPD-12-2 with incompletely annotated information, was identified as Ps due to different copy numbers and expression level (Liu et al., 2020). The expression level of Glyma.12G187200 in the leaves of NN 86-4 was fivefold greater than in PI 342618B (Figure 4D). These results suggested that Glyma.01g240100 may not be the candidate gene of qPD-1-1, and Glyma.12G187200 was the candidate gene that not only controls PD of soybean leaves, but it also contributes to PL in the present study. Then a cluster analysis of the above candidate genes was conducted with their homologs, which have been known to be associated with pubescence development in several species (Supplementary Figure 4). Most candidate genes clustered together with their homologs, with seven were reliable (bootstrap values > 65%), such as Ps and Pd1 (Supplementary Figure 4). Among them, Glyma.01g195900 and Glyma.12G187200 (Ps) of PL and Glyma.12g194400, Glyma.12G187200 (Ps), Glyma.12g195200, and Glyma.12g195900 of PD were suggested as important candidate genes due to significant differences in the expression between parents. Based on qRT-PCR and cluster analysis, three (Glyma.01g195900, Glyma.01g198100, and Glyma.12G187200) and four (Glyma.12G187200, Glyma.12g194400, Glyma.12g195200, and Glyma.12g195900) important candidate genes were identified for PL and PD, respectively.
Resistance of Different Pubescence Morphology Lines to Common Cutworm
To determine the relationship between pubescence morphology and resistance to CCW, an antibiotic test was carried out using lines with four types of pubescence morphology and the typical photographs of pubescence are shown in Figures 5A–D. There was a significant difference between increased larval weight of feeding with leaves with SD pubescence (similar to the pubescence morphology of wild soybean PI 342618B) and leaves with the other three pubescence morphology types. The former (SD, viz. wild soybean pubescence morphology) had the strongest resistance to CCW, followed by LD, SS, and LS (Figures 5E–I). The increased larval weight had positive and negative correlations with PL and PD, respectively (Table 5).
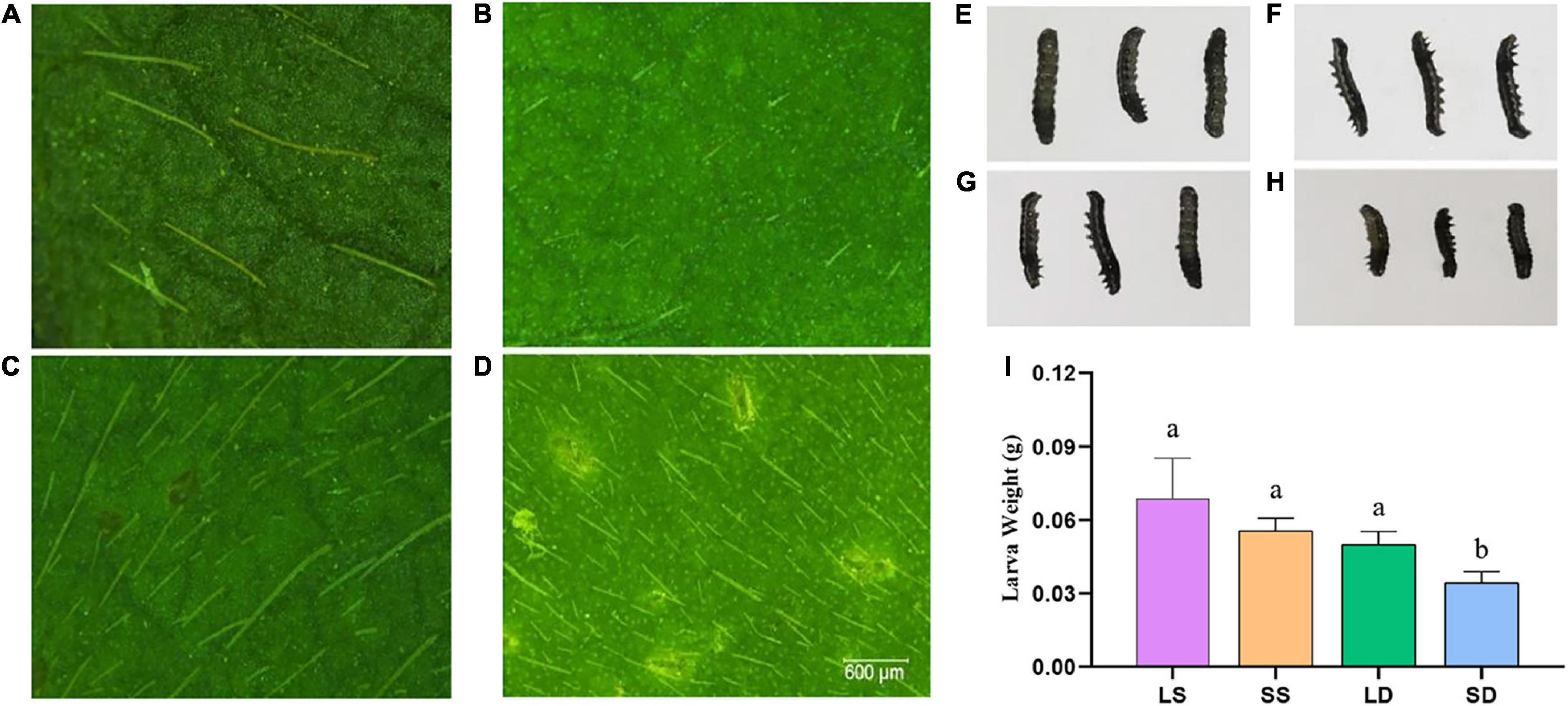
Figure 5. The resistance to CCW of lines with different pubescence length and density. (A–D) Typical photographs of four different pubescence morphology. (A) Long and sparse (LS); (B) short and sparse (SS); (C) long and dense (LD); (D) short and dense (SD). CCW, common cutworm. (E–H) Larvae morphology after feeding with leaves of four different pubescence morphology. (E–H corresponds to A–D, respectively). (I) The added larval weight was inoculated with the leaves of four different pubescence morphology. Significant differences were shown by different letters (P ≤ 0.05).
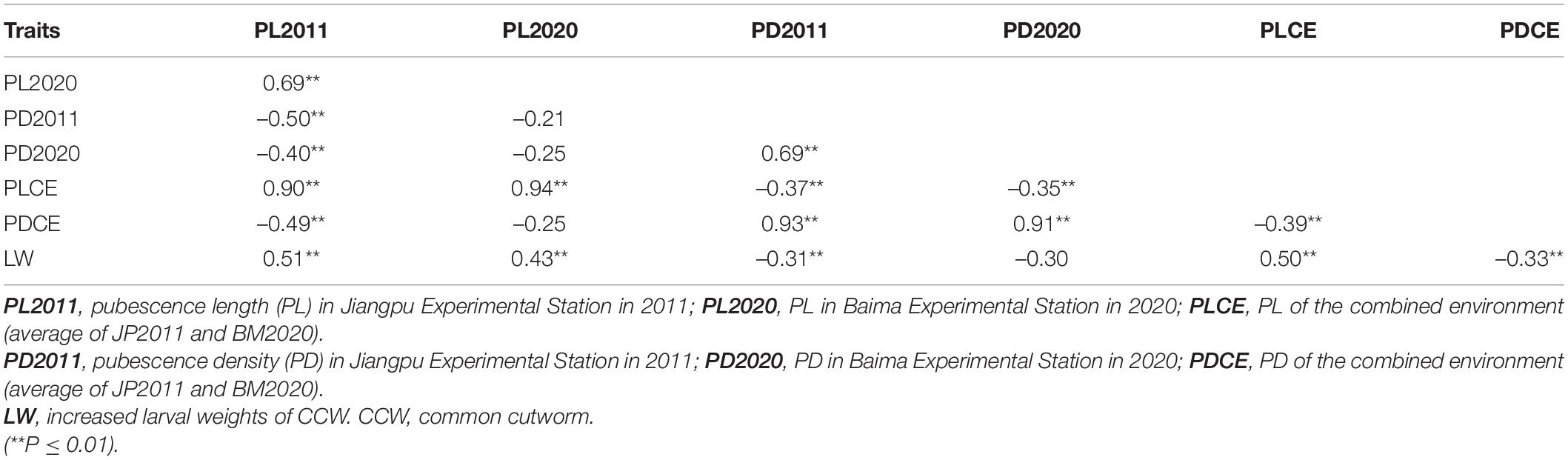
Table 5. The correlation analysis of pubescence traits and resistance to CCW in four kinds of different pubescence morphology lines.
Discussion
Genetic Basis of Pubescence Length and Density
Pubescence can form a physical barrier that protects plants against various biotic and abiotic stresses (Kennedy, 2003; Kang et al., 2016). The effectiveness of this preventive mechanism depends on the length and density of pubescence (Hua et al., 2021). Therefore, it is necessary to understand the genetic mechanisms associated with polygenic quantitative characters PL and PD. Although several QTLs related to PL and PD have been identified and reported over the past decades, most of them have large interval regions due to the small mapping population (<200 lines) and low-density genetic map based on simple sequence repeat (SSR) markers (Du et al., 2009; Oki et al., 2012). These may be difficult to be used in practical plant breeding and predicting probable candidate genes.
In the present study, two QTL mapping methods were used to complement and validate the results of each other and improve the accuracy of QTL mapping. A total of nine and seven QTLs were detected of PL and PD by CIM, respectively, while one and four QTLs were mapped by MCIM. By comparing the results of two methods, two QTLs (qPD-11-1 and qPD-12-2) related to PD were detected in both methods, and qPD-12-2 (Chr12) was the same as previously reported QTLs viz. Pubescence density 2-8, Pubescence density 3-2, and PD12-1 (Du et al., 2009; Oki et al., 2012; Xing et al., 2013), suggesting these loci play a role in pubescence development. Besides, the CIM method detected more QTLs with higher additive effect and R2 value while the MCIM method mapped QTLs with narrow CIs. It was possible to miss some important loci if only the MCIM method was used, thus, it is better to use two methods.
By comparing QTLs detected in two methods with the reported ones in previous studies, qPL-12-2 (Chr12) with an interval of 35,364,334–35,611,487 bp overlapped with Pubescence length 1-2, which had a larger CI (35,108,089–36,780,375 bp) (Oki et al., 2012). qPL-12-3 (Chr12) was overlapped with PL12-1 (Xing et al., 2013). The remaining eight QTLs related to PL were detected for the first time in the present study (Tables 2, 3 and Figure 2). For PD, a total of nine QTLs were detected by CIM and MCIM. In addition to qPD-12-2 mentioned in the previous paragraph, qPD-12-3 (Chr12) was overlapped with Pubescence density 2-7 (Du et al., 2009); qPD-1-1 (Chr01) and qPD-2-1 (Chr02) were same to Pubescence density 3-1, PD1-1 (Oki et al., 2012; Xing et al., 2013) and Pubescence density 2-5 (Du et al., 2009), respectively, but in a narrower genomic region in the present study. The remaining five QTLs were detected for the first time. Most QTLs were novel indicating the distinct and abundant genetic architecture of pubescence in wild soybean. It also suggests the need to utilize more diverse parents to develop a mapping population to reveal the complex genetic basis of pubescence development in soybean and provide more valuable information for the gene identification related to pubescence development. In addition, the majority of QTLs identified in the present study were in small physical genomic regions, suggesting the importance of using a high-resolution genetic map for QTL detection and candidate gene exploration.
Although many studies had demonstrated that dense and long pubescence have higher resistance to abiotic stress (Turnipseed, 1977; Gunasinghe et al., 1988), the purpose of soybean breeding is not always to increase density and length of pubescence. It is important to keep PL and PD within a suitable range for the better growth and development of plants. In addition, it was found that there was a negative correlation between PL and PD in the present study, hence, materials with dense and long pubescence may not be easy to obtain. Our results demonstrated that soybean leaves with SD viz. wild soybean pubescence morphology instead of LS viz. cultivar soybean pubescence morphology had the stronger resistance to CCW thus the former can be used in soybean breeding.
Candidate Gene Analysis of Pubescence Length and Density
It is of great significance for both theoretical research and breeding practice to identify the candidate genes of major QTL regions of pubescence traits in soybean. Many factors were identified to be related to trichome development in other species, providing the useful information to explore candidate genes of soybean pubescence development. The mechanism of Arabidopsis trichome development has been comprehensively explained (Shang et al., 2020). The core regulatory components are the R2R3-MYB/basic helix-loop-helix (bHLH)/WD complex (Ishida et al., 2008). R3-MYB negatively regulates trichome formation by competing with R2R3-MYB for binding to bHLH (Hülskamp et al., 1994; Schellmann et al., 2002; Esch et al., 2003). In tomato, bHLH TF (SlMYC1) and R2R3-MYB TFs (SlTHM1 and SlMYB52) play an important role in the formation of trichomes (Xu et al., 2018; Yuan et al., 2021). The fiber initiation and elongation are somehow similar to trichome development. In cotton, R2R3-MYB TF GhMYB109 is required for cotton fiber development (Pu et al., 2008) and the homologues of GhMYB109 in tomatoes might also participate in the regulation of trichome elongation (Hua et al., 2021). Therefore, MYB TF, bHLH TF, and WD play an extremely important role in both unicellular and multicellular trichome development.
Recently, actin filaments and microtubules were reported to play coordinated but distinct roles in the formation of tomato trichome (Chang et al., 2019). Both in Arabidopsis and tomatoes, mutations in genes of SCAR/WAVE complex could lead to distorted trichomes (Kang et al., 2016; Chang et al., 2019; Li et al., 2019). In soybean, GmNAP1 was involved in actin filament assembling during trichome and pavement cell development (Campbell et al., 2016; Tang et al., 2020). Thus, actin and microtubules were identified as having an undeniable role in trichome development in recent years.
Additionally, a set of C2H2 zinc finger TFs, such as GIS, GIS2, GIS3, ZFP5, and Hair (H) gene, were detected to be involved in trichome development in Arabidopsis and tomatoes, respectively (Gan et al., 2007; Zhou et al., 2011; Sun et al., 2015; Chang et al., 2018). AP2 and AP2/ERF TFs: TAR1 and Hairy Leaf 6 (HL6), play an important role in trichome development in A. annua (Tan et al., 2015) and rice (Sun et al., 2017; Shang et al., 2020). Besides, the WUS-type homeobox gene OsWOX3B was found to be required for macro-hair initiation and trichome development in rice (Zhang et al., 2012). Cyclins were involved in the transition of the cell cycle and function as positive regulators of cell proliferation in eukaryotes (Meijer and Murray, 2001) and a B-type cyclin gene, SlCycB2, plays key roles in trichome initiation in tomatoes (Gao et al., 2017). These results suggested that the above factors may be functional during trichome development.
A total of 22 and 13 candidate genes (together with Ps) were identified for PL and PD, respectively, based on PANTHER analysis, expression data, and literatures in the present study (Table 4). It should be noted that genes within the physical genomic interval that are not annotated and/or have no expression in young leaves may be ignored. A cluster analysis was conducted of these candidate genes and the homologs mentioned above (Supplementary Figure 4). The genes Ps and Pd1, which have known to be related to soybean PD, were clustered with homologous genes of other species, indicating the reliability of this analysis method. However, more study is needed for their functional validation.
Conclusion
A total of 10 and 9 QTLs of PL and PD were detected, respectively, from which three and four important candidate genes were identified. PL negatively correlated with PD and leaves with short and dense pubescence viz. wild soybean pubescence morphology had the highest resistance to CCW.
Data Availability Statement
The original contributions presented in the study are included in the article/Supplementary Material, further inquiries can be directed to the corresponding author/s.
Author Contributions
TZ and GX conceived and designed the experiments. YL, LC, XL, NZ, YX, YW, ZL, LT, and HY performed the experiments. YL and FC analyzed the data. YL drafted the manuscript. GX, TZ, and BK revised the manuscript. All authors contributed to the article and approved the submitted version.
Funding
This work was supported by the National Key R&D Program (no. 2021YFD1201604), the Natural Science Foundation of China (no. 31571694), Fundamental Research Funds for Central Universities (no. KYZ201504), MOE 111 Project (B08025), MOE Program for Changjiang Scholars and Innovative Research Team in University (PCSIRT13073), MOA CARS-04 Program, Jiangsu Higher Education PAPD Program, and Jiangsu JCIC-MCP Program.
Conflict of Interest
The authors declare that the research was conducted in the absence of any commercial or financial relationships that could be construed as a potential conflict of interest.
Publisher’s Note
All claims expressed in this article are solely those of the authors and do not necessarily represent those of their affiliated organizations, or those of the publisher, the editors and the reviewers. Any product that may be evaluated in this article, or claim that may be made by its manufacturer, is not guaranteed or endorsed by the publisher.
Supplementary Material
The Supplementary Material for this article can be found online at: https://www.frontiersin.org/articles/10.3389/fpls.2021.771850/full#supplementary-material
Supplementary Figure 1 | Pearson’s correlation analysis of pubescence length (PL) and pubescence density (PD) (**P ≤ 0.01). PL2011, the PL in Jiangpu experimental station in 2011; PL2020, the PL in Baima experimental station in 2020; PLCE, the PL of combined environment (the average of JP2011 and BM2020). PD2011, the PD in Jiangpu experimental station in 2011; PD2020, the PD in Baima experimental station in 2020; PDCE, the PD of combined environment (the average of JP2011 and BM2020).
Supplementary Figure 2 | High-density genetic linkage map of 20 chromosomes in the NJRINP constructed based on the RAD-Seq. The different colors represent the distinct marker density.
Supplementary Figure 3 | DNA sequence alignment of the last exon of Glyma.01g240100 (Pd1) in two parents.
Supplementary Figure 4 | Phylogenetic relationship between predicted candidate genes and their homologs based on literature. AT, Arabidopsis thaliana (thale cress); Glyma, Glycine max (Linn.) Merr. (soybean); Os, Oryza sativa (rice); Sl, Solanum lycopersicum (tomato); Am, Antirrhinum majus L. (Snapdragon). Gene names from different species are shown by different colors. Gene names with stars behind them indicate genes that can cluster with homologous genes.
Footnotes
- ^ https://imagej.en.softonic.com/
- ^ https://www.soybase.org/genomeannotation/
- ^ https://www.soybase.org/soyseq/
- ^ https://genome.jgi.doe.gov/portal/pages/dynamicOrganismDownload.jsf?organism=Phytozome
- ^ http://www.pantherdb.org/
References
Bandillo, N. B., Lorenz, A. J., Graef, G. L., Jarquin, D., Hyten, D. L., Nelson, R. L., et al. (2017). Genome-wide association mapping of qualitatively inherited traits in a germplasm collection. Plant Genome 10:28724068. doi: 10.3835/plantgenome2016.06.0054
Bernard, R. L., and Singh, B. B. (1969). Inheritance of pubescence type in soybeans: glabrous, curly, dense, sparse, and puberulent1. Crop. Sci. 9, 192–197. doi: 10.2135/cropsci1969.0011183X000900020025x
Bickford, C. P. (2016). Ecophysiology of leaf trichomes. Funct. Plant Biol. 43, 807–814. doi: 10.1071/fp16095
Campbell, B. W., Hofstad, A. N., Sreekanta, S., Fu, F., Kono, T. J., O’Rourke, J. A., et al. (2016). Fast neutron-induced structural rearrangements at a soybean NAP1 locus result in gnarled trichomes. Theor. Appl. Genet. 129, 1725–1738. doi: 10.1007/s00122-016-2735-x
Campbell, B. W., Hoyle, J. W., Bucciarelli, B., Stec, A. O., Samac, D. A., Parrott, W. A., et al. (2019). Functional analysis and development of a CRISPR/Cas9 allelic series for a CPR5 ortholog necessary for proper growth of soybean trichomes. Sci. Rep. 9:14757. doi: 10.1038/s41598-019-51240-7
Chang, H. X., and Hartman, G. L. (2017). Characterization of insect resistance loci in the USDA soybean germplasm collection using genome-wide association studies. Front. Plant Sci. 8:670. doi: 10.3389/fpls.2017.00670
Chang, J., Xu, Z., Li, M., Yang, M., Qin, H., Yang, J., et al. (2019). Spatiotemporal cytoskeleton organizations determine morphogenesis of multicellular trichomes in tomato. PLoS Genet. 15:e1008438. doi: 10.1371/journal.pgen.1008438
Chang, J., Yu, T., Yang, Q., Li, C., Xiong, C., Gao, S., et al. (2018). Hair, encoding a single C2H2 zinc-finger protein, regulates multicellular trichome formation in tomato. Plant J. 96, 90–102. doi: 10.1111/tpj.14018
Chen, C., Chen, H., Zhang, Y., Thomas, H. R., Frank, M. H., He, Y., et al. (2020). TBtools: an integrative toolkit developed for interactive analyses of big biological data. Mol. Plant 13, 1194–1202. doi: 10.1016/j.molp.2020.06.009
Clawson, K. L., Specht, J. E., Blad, B. L., and Garay, A. F. (1986). Water use efficiency in soybean pubescence density isolines—a calculation procedure for estimating daily values. Agron. J. 78, 483–486. doi: 10.2134/agronj1986.00021962007800030018x
Cregan, P. B., Jarvik, T., Bush, A. L., Shoemaker, R. C., Lark, K. G., Kahler, A. L., et al. (1999). An integrated genetic linkage map of the soybean genome. Crop. Sci. 39, 1464–1490. doi: 10.2135/cropsci1999.3951464x
Du, W., Yu, D., and Fu, S. (2009). Analysis of QTLs for the trichome density on the upper and downer surface of leaf blade in soybean [Glycine max (L.) Merr.]. Agric. Sci. China 8, 529–537. doi: 10.1016/s1671-2927(08)60243-6
Esch, J. J., Chen, M., Sanders, M., Hillestad, M., Ndkium, S., Idelkope, B., et al. (2003). A contradictory GLABRA3 allele helps define gene interactions controlling trichome development in Arabidopsis. Development 130, 5885–5894. doi: 10.1242/dev.00812
Gan, Y., Liu, C., Yu, H., and Broun, P. (2007). Integration of cytokinin and gibberellin signalling by Arabidopsis transcription factors GIS, ZFP8 and GIS2 in the regulation of epidermal cell fate. Development 134, 2073–2081. doi: 10.1242/dev.005017
Gao, S., Gao, Y., Xiong, C., Yu, G., Chang, J., Yang, Q., et al. (2017). The tomato B-type cyclin gene, SlCycB2, plays key roles in reproductive organ development, trichome initiation, terpenoids biosynthesis and Prodenia litura defense. Plant Sci. 262, 103–114. doi: 10.1016/j.plantsci.2017.05.006
Ghorashy, S. R., Pendleton, J. W., Bernard, R. L., and Bauer, M. E. (1971). Effect of leaf pubescence on transpiration, photosynthetic rate and seed yield of three near-isogenic lines of soybeans. Crop Sci. 11, 426–427. doi: 10.2135/cropsci1971.0011183X001100030035x
Gunasinghe, U. B., Irwin, M. E., and Kampmeier, G. E. (1988). Soybean leaf pubescence affects aphid vector transmission and field spread of soybean mosaic virus. Ann. Appl. Biol 112, 259–272. doi: 10.1111/j.1744-7348.1988.tb02062.x
Hanley, M. E., Lamont, B. B., Fairbanks, M. M., and Rafferty, C. M. (2007). Plant structural traits and their role in anti-herbivore defence. Perspect. Plant Ecol. Evol. Syst. 8, 157–178. doi: 10.1016/j.ppees.2007.01.001
Hua, B., Chang, J., Xu, Z., Han, X., Xu, M., Yang, M., et al. (2021). HOMEODOMAIN PROTEIN8 mediates jasmonate-triggered trichome elongation in tomato. New Phytol. 230, 1063–1077. doi: 10.1111/nph.17216
Huchelmann, A., Boutry, M., and Hachez, C. (2017). Plant glandular trichomes: natural cell factories of high biotechnological interest. Plant Physiol. 175, 6–22. doi: 10.1104/pp.17.00727
Hulskamp, M. (2004). Plant trichomes: a model for cell differentiation. Nat. Rev. Mol. Cell Biol. 5, 471–480. doi: 10.1038/nrm1404
Hülskamp, M., Misàa, S., and Jürgens, G. (1994). Genetic dissection of trichome cell development in Arabidopsis. Cell 76, 555–566. doi: 10.1016/0092-8674(94)90118-x
Hurley, T. M., and Mitchell, P. D. (2020). The value of insect management to US maize, soybean and cotton farmers. Pest Manag. Sci. 76, 4159–4172. doi: 10.1002/ps.5974
Ishida, T., Kurata, T., Okada, K., and Wada, T. (2008). A genetic regulatory network in the development of trichomes and root hairs. Annu. Rev. Plant Biol. 59, 365–386. doi: 10.1146/annurev.arplant.59.032607.092949
Kang, J. H., Campos, M. L., Zemelis-Durfee, S., Al-Haddad, J. M., Jones, A. D., Telewski, F. W., et al. (2016). Molecular cloning of the tomato hairless gene implicates actin dynamics in trichome-mediated defense and mechanical properties of stem tissue. J. Exp. Bot. 67, 5313–5324. doi: 10.1093/jxb/erw292
Kennedy, G. G. (2003). Tomato, pests, parasitoids, and predators: tritrophic interactions involving the genus Lycopersicon. Annu. Rev. Entomol. 48, 51–72. doi: 10.1146/annurev.ento.48.091801.112733
Lahiri, S., Fallen, B. D., Mian, R., Carter, T. E., Greene, J. K., Reay-Jones, F. P. F., et al. (2020a). Soybean host plant resistance to Megacopta cribraria (Hemiptera: Plataspidae) and the potential role of leaf trichome density. Environ. Entomol. 49, 88–97. doi: 10.1093/ee/nvz158
Lahiri, S., Reisig, D. D., Dean, L. L., Reay-Jones, F. P. F., Greene, J. K., Carter, T. E., et al. (2020b). Mechanisms of soybean host-plant resistance against Megacopta cribraria (Hemiptera: Plataspidae). Environ. Entomol. 49, 876–885. doi: 10.1093/ee/nvaa075
Lam, W. K. F., and Pedigo, L. P. (2001). Effect of trichome density on soybean pod feeding by adult bean leaf beetles (Coleoptera: Chrysomelidae). J. Econ. Entomol. 94, 1459–1463. doi: 10.1603/0022-0493-94.6.1459
Li, J., Kim, T., and Szymanski, D. B. (2019). Multi-scale regulation of cell branching: modeling morphogenesis. Dev. Biol. 451, 40–52. doi: 10.1016/j.ydbio.2018.12.004
Liu, S., Fan, L., Liu, Z., Yang, X., Zhang, Z., Duan, Z., et al. (2020). A Pd1-Ps-P1 feedback loop controls pubescence density in soybean. Mol. Plant 13, 1768–1783. doi: 10.1016/j.molp.2020.10.004
Livak, K. J., and Schmittgen, T. D. (2001). Analysis of relative gene expression data using real-time quantitative PCR and the 2−ΔΔCT method. Methods 25, 402–408. doi: 10.1006/meth.2001.1262
Manetas, Y. (2003). The importance of being hairy: the adverse effects of hair removal on stem photosynthesis of Verbascum speciosum are due to solar UV-B radiation. New Phytol. 158, 503–508. doi: 10.1046/j.1469-8137.2003.00768.x
Matias-Hernandez, L., Aguilar-Jaramillo, A. E., Cigliano, R. A., Sanseverino, W., and Pelaz, S. (2016). Flowering and trichome development share hormonal and transcription factor regulation. J. Exp. Bot. 67, 1209–1219. doi: 10.1093/jxb/erv534
Meijer, M., and Murray, J. A. (2001). Cell cycle controls and the development of plant form. Curr. Opin. Plant Biol. 4, 44–49. doi: 10.1016/s1369-5266(00)00134-5
Nakaya, H. I., Thakar, J., and Maracaja-Coutinho, V. (2020). Editorial: user-friendly tools applied to genetics or systems biology. Front. Genet. 11:985. doi: 10.3389/fgene.2020.00985
Nyquist, W. E., and Baker, R. J. (1991). Estimation of heritability and prediction of selection response in plant populations. Crit. Rev. Plant Sci. 10, 235–322. doi: 10.1080/07352689109382313
Oki, N., Komatsu, K., Sayama, T., Ishimoto, M., Takahashi, M., and Takahashi, M. (2012). Genetic analysis of antixenosis resistance to the common cutworm (Spodoptera litura Fabricius) and its relationship with pubescence characteristics in soybean (Glycine max (L.) Merr.). Breed. Sci. 61, 608–617. doi: 10.1270/jsbbs.61.608
Owen, F. V. (1927). Inheritance studies in soybeans. II. glabrousness, color of pubescence, time of maturity, and linkage relations. Genetics 12, 519–529. doi: 10.1093/genetics/12.6.519
Pattanaik, S., Patra, B., Singh, S. K., and Yuan, L. (2014). An overview of the gene regulatory network controlling trichome development in the model plant, Arabidopsis. Front. Plant Sci. 5:259. doi: 10.3389/fpls.2014.00259
Peng, L., Skylar, A., Chang, P. L., Bisova, K., and Wu, X. (2014). CYCP2;1 integrates genetic and nutritional information to promote meristem cell division in Arabidopsis. Dev. Biol. 393, 160–170. doi: 10.1016/j.ydbio.2014.06.008
Pu, L., Li, Q., Fan, X., Yang, W., and Xue, Y. (2008). The R2R3 MYB transcription factor GhMYB109 is required for cotton fiber development. Genetics 180, 811–820. doi: 10.1534/genetics.108.093070
Schellmann, S., Schnittger, A., Kirik, V., Wada, T., Okada, K., Beermann, A., et al. (2002). TRIPTYCHON and CAPRICE mediate lateral inhibition during trichome and root hair patterning in Arabidopsis. EMBO J. 21, 5036–5046. doi: 10.1093/emboj/cdf524
Serna, L. (2004). A network of interacting factors triggering different cell fates. Plant Cell 16, 2258–2263. doi: 10.1105/tpc.104.16093
Severin, A. J., Woody, J. L., Bolon, Y. T., Joseph, B., Diers, B. W., Farmer, A. D., et al. (2010). RNA-Seq Atlas of Glycine max: a guide to the soybean transcriptome. BMC Plant Biol. 10:160. doi: 10.1186/1471-2229-10-160
Shang, F., Mou, W., Wu, H., Xu, F., Xiang, C., and Wang, J. (2020). New allele of HL6 regulates trichome elongation in Rice. Rice Sci. 27, 480–492. doi: 10.1016/j.rsci.2020.09.005
Singh, B. B., Hadley, H. H., and Bernard, R. L. (1971). Morphology of pubescence in soybeans and its relationship to plant vigor. Crop. Sci. 11, 13–16. doi: 10.2135/cropsci1971.0011183X001100010004x
Specht, J. E., Chase, K., Macrander, M., Graef, G. L., Chung, J., Markwell, J. P., et al. (2001). Soybean response to water: a QTL analysis of drought tolerance. Crop. Sci. 41, 493–509. doi: 10.2135/cropsci2001.412493x
Specht, J. E., Williams, J. H., and Pearson, D. R. (1985). Near-isogenic analyses of soybean pubescence genes. Crop. Sci. 25, 92–96. doi: 10.2135/cropsci1985.0011183X002500010024x
Sun, L., Zhang, A., Zhou, Z., Zhao, Y., Yan, A., Bao, S., et al. (2015). GLABROUS INFLORESCENCE STEMS3 (GIS3) regulates trichome initiation and development in Arabidopsis. New Phytol. 206, 220–230. doi: 10.1111/nph.13218
Sun, W., Gao, D., Xiong, Y., Tang, X., Xiao, X., Wang, C., et al. (2017). Hairy leaf 6, an AP2/ERF transcription factor, interacts with OsWOX3B and regulates trichome formation in rice. Mol. Plant 10, 1417–1433. doi: 10.1016/j.molp.2017.09.015
Tamura, K., Stecher, G., Peterson, D., Filipski, A., and Kumar, S. (2013). MEGA6: molecular evolutionary genetics analysis version 6.0. Mol. Biol. Evol. 30, 2725–2729. doi: 10.1093/molbev/mst197
Tan, H., Xiao, L., Gao, S., Li, Q., Chen, J., Xiao, Y., et al. (2015). TRICHOME AND ARTEMISININ REGULATOR 1 Is required for trichome development and artemisinin biosynthesis in Artemisia annua. Mol. Plant 8, 1396–1411. doi: 10.1016/j.molp.2015.04.002
Tang, K., Yang, S., Feng, X., Wu, T., Leng, J., Zhou, H., et al. (2020). GmNAP1 is essential for trichome and leaf epidermal cell development in soybean. Plant Mol. Biol. 103, 609–621. doi: 10.1007/s11103-020-01013-y
Turnipseed, S. G. (1977). Influence of trichome variations on populations of small phytophagous insects in soybean. Environ. Entomol. 6, 815–817. doi: 10.1093/ee/6.6.815
Wang, W., Liu, M., Wang, Y., Li, X., Cheng, S., Shu, L., et al. (2016). Characterizing two inter-specific bin maps for the exploration of the QTLs/genes that confer three soybean evolutionary traits. Front. Plant Sci. 7:1248. doi: 10.3389/fpls.2016.01248
Xing, G., Liu, K., and Gai, J. (2017). A high-throughput phenotyping procedure for evaluation of antixenosis against common cutworm at early seedling stage in soybean. Plant Methods 13:66. doi: 10.1186/s13007-017-0215-1
Xing, G., Liu, Z., Tan, L., Yue, H., Wang, Y., Kim, H., et al. (2013). QTL mapping of pubescence density and length on leaf surface of soybean. Acta Agronom. Sin. 39, 12–20. doi: 10.3724/sp.J.1006.2013.00012
Xu, J., van Herwijnen, Z. O., Dräger, D. B., Sui, C., Haring, M. A., and Schuurink, R. C. (2018). SlMYC1 regulates Type VI glandular trichome formation and terpene biosynthesis in tomato glandular cells. Plant Cell 30, 2988–3005. doi: 10.1105/tpc.18.00571
Yang, C., and Ye, Z. (2013). Trichomes as models for studying plant cell differentiation. Cell Mol. Life Sci. 70, 1937–1948. doi: 10.1007/s00018-012-1147-6
Yuan, Y., Xu, X., Luo, Y., Gong, Z., Hu, X., Wu, M., et al. (2021). R2R3 MYB-dependent auxin signalling regulates trichome formation, and increased trichome density confers spider mite tolerance on tomato. Plant Biotechnol. J. 19, 138–152. doi: 10.1111/pbi.13448
Zeng, Z. (1993). Theoretical basis for separation of multiple linked gene effects in mapping quantitative trait loci. Proc. Natl. Acad. Sci. U.S.A. 90, 10972–10976. doi: 10.1073/pnas.90.23.10972
Zhang, H., Wu, K., Wang, Y., Peng, Y., Hu, F., Wen, L., et al. (2012). A WUSCHEL-like homeobox gene, OsWOX3B responses to NUDA/GL-1 locus in rice. Rice 5:30. doi: 10.1186/1939-8433-5-30
Zhou, Z., An, L., Sun, L., Zhu, S., Xi, W., Broun, P., et al. (2011). Zinc finger protein5 is required for the control of trichome initiation by acting upstream of zinc finger protein8 in Arabidopsis. Plant Physiol. 157, 673–682. doi: 10.1104/pp.111.180281
Zhu, J. (2016). Abiotic stress signaling and responses in plants. Cell 167, 313–324. doi: 10.1016/j.cell.2016.08.029
Keywords: soybean, QTL mapping, pubescence length and density, candidate gene, resistance to common cutworm
Citation: Li Y, Chu L, Liu X, Zhang N, Xu Y, Karikari B, Wang Y, Chang F, Liu Z, Tan L, Yue H, Xing G and Zhao T (2022) Genetic Architecture and Candidate Genes for Pubescence Length and Density and Its Relationship With Resistance to Common Cutworm in Soybean. Front. Plant Sci. 12:771850. doi: 10.3389/fpls.2021.771850
Received: 07 September 2021; Accepted: 05 November 2021;
Published: 07 January 2022.
Edited by:
Rouf Mian, SNFR Unit, Agriculture Research Service, United States Department of Agriculture (USDA), United StatesReviewed by:
Earl William Taliercio, Agricultural Research Service, United States Department of Agriculture (USDA), United StatesTri D. Vuong, University of Missouri, United States
Copyright © 2022 Li, Chu, Liu, Zhang, Xu, Karikari, Wang, Chang, Liu, Tan, Yue, Xing and Zhao. This is an open-access article distributed under the terms of the Creative Commons Attribution License (CC BY). The use, distribution or reproduction in other forums is permitted, provided the original author(s) and the copyright owner(s) are credited and that the original publication in this journal is cited, in accordance with accepted academic practice. No use, distribution or reproduction is permitted which does not comply with these terms.
*Correspondence: Guangnan Xing, xinggn@njau.edu.cn; Tuanjie Zhao, tjzhao@njau.edu.cn