- 1Organismal and Evolutionary Biology Research Programme, Faculty of Biological and Environmental Sciences, Viikki Plant Science Centre, University of Helsinki, Helsinki, Finland
- 2Department of Biochemistry, Molecular Plant Biology, University of Turku, Turku, Finland
- 3Institute of Biotechnology, HILIFE – Helsinki Institute of Life Science, University of Helsinki, Helsinki, Finland
Plants live in a world of changing environments, where they are continuously challenged by alternating biotic and abiotic stresses. To transfer information from the environment to appropriate protective responses, plants use many different signaling molecules and pathways. Reactive oxygen species (ROS) are critical signaling molecules in the regulation of plant stress responses, both inside and between cells. In natural environments, plants can experience multiple stresses simultaneously. Laboratory studies on stress interaction and crosstalk at regulation of gene expression, imply that plant responses to multiple stresses are distinctly different from single treatments. We analyzed the expression of selected marker genes and reassessed publicly available datasets to find signaling pathways regulated by ozone, which produces apoplastic ROS, and high light treatment, which produces chloroplastic ROS. Genes related to cell death regulation were differentially regulated by ozone versus high light. In a combined ozone + high light treatment, the light treatment enhanced ozone-induced cell death in leaves. The distinct responses from ozone versus high light treatments show that plants can activate stress signaling pathways in a highly precise manner.
Introduction
To be a plant is to prioritize between conflicting obligations – developmental programs should be balanced with inputs from the environment including varying light conditions and unfavorable growth conditions such as extreme temperatures, lack of water or pathogen attack. Maintaining permanently active defenses diverts energy from growth and typically plants with constitutively activate defenses are dwarfed. Hence, activation of defenses should only take place when necessary. Multiple signaling molecules take part in plant responses to the environment, including reactive oxygen species (ROS), Ca2+, and plant hormones, such as abscisic acid (ABA), salicylic acid (SA), jasmonic acid (JA), and ethylene.
Reactive oxygen species including hydrogen peroxide, superoxide, and singlet oxygen (H2O2, O2.–, and 1O2, respectively), are continuously formed as metabolic by-products in mitochondria, chloroplasts, and peroxisomes. However, the emerging consensus is that ROS are also actively produced as signaling molecules in the apoplast and chloroplasts to drive developmental processes and plant responses to abiotic and biotic stress (Phua et al., 2021). Each type of ROS can be characterized by its own reactivity and lifetime (Waszczak et al., 2018). Additionally the site of ROS production, e.g., apoplast, chloroplast, mitochondria, or peroxisome, leads to activation of different signaling pathways (Gadjev et al., 2006; Vaahtera et al., 2014). The apoplastic ROS burst is an early response to recognition of many pathogens via pathogen-associated molecular patterns (PAMPs), and is produced by cell wall peroxidases and respiratory burst oxidase homologue D (RBOHD). The air pollutant ozone (O3) breaks down in the apoplast to O2.– and H2O2, followed by activation of the cells own ROS production machinery (Wohlgemuth et al., 2002; Vainonen and Kangasjarvi, 2015). While ozone levels at heavily ozone polluted areas vary between 60 and 100 ppb (Tiwari et al., 2008), higher concentrations up to 350 ppb O3 have been used as a research tool to activate and study apoplastic ROS signaling (Vainonen and Kangasjarvi, 2015; Xu et al., 2015a). In chloroplasts, formation of ROS is commonly associated with high light (HL) stress. In addition, the chloroplast regulates many aspects of plant defense and pathogen responses. This includes the biosynthesis steps for several stress hormones, including ABA, JA, and SA (Littlejohn et al., 2021). In pathogen infections, not only RBOHD and cell wall peroxidases generates apoplastic ROS, but also the chloroplast provides ROS for defense signaling (Zabala et al., 2015; Littlejohn et al., 2021). The photosynthetic machinery is dynamically regulated in order to keep energy transduction reactions in balance and to minimize ROS-producing side reactions (Tikkanen and Aro, 2014). Nevertheless, the regulatory machinery not only functions against ROS production, but can also be an important factor controlling the initiation and strength of ROS signals from chloroplast (Frenkel et al., 2009; Tikkanen et al., 2014; Gollan et al., 2015). There is no scientific consensus for what constitutes a HL treatment to initiate chloroplast retrograde or ROS signaling, but what is often used to study HL stress in Arabidopsis thaliana is a shift from fairly low light conditions (50–100 μmol m–2 s–1) to HL (>1,000 μmol m–2 s–1) (Table 1). Under these conditions, the HL treatment leads to production of 1O2, O2.–, and H2O2 in the chloroplast and results in large scale changes in gene expression (Table 1; Waszczak et al., 2018). In addition, the HL treatment also activates systemic signaling that regulates several biological processes including expression of defense related genes (Karpinski et al., 1999; Zandalinas et al., 2019b).
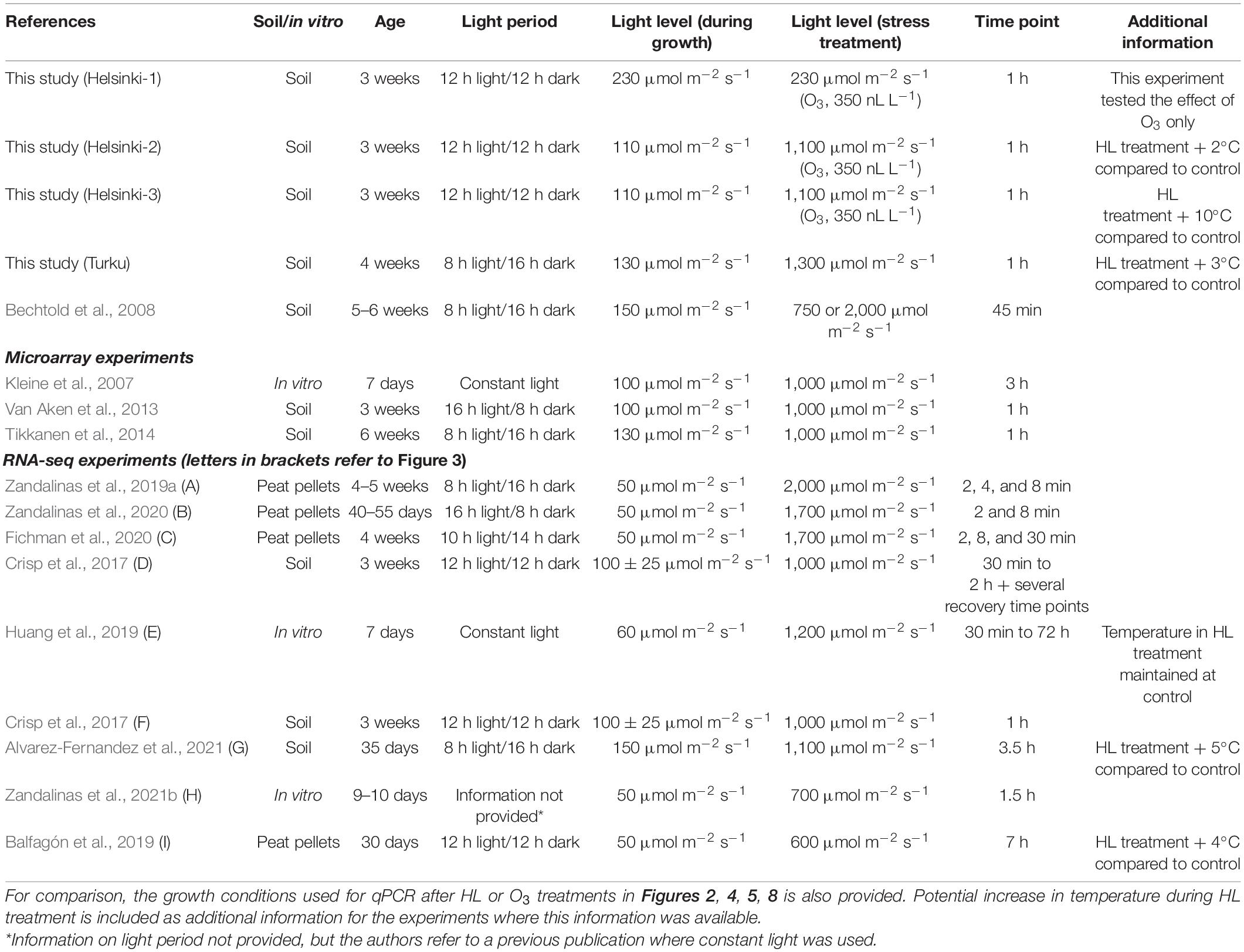
Table 1. Growth conditions used for Arabidopsis HL experiments and transcriptome analysis with qPCR, microarrays or RNA-seq.
There is an ongoing discussion in the literature to which extent there is interaction between HL and heat stress signaling. From an environment point of view, the interaction is clear, as days with very HL levels would typically also be warmer (Zandalinas et al., 2021a). In laboratory experiments, there is a similar question about the interaction as depending on the light source, in HL experiments there can also be an associated increase in temperature unless precaution is taken to control the temperature or filter the light through water (see for example, Jung et al., 2013). At the molecular level, using marker genes to report transcriptional changes, there is interaction between HL and heat stress, as the commonly used marker gene APX2 (ASCORBATE PEROXIDASE 2) shows synergistic interaction with higher transcript levels when HL is combined with increased temperature (Jung et al., 2013; Huang et al., 2019). From array and RNA-seq experiments in different combined stresses including HL + heat stress, there is an emerging consensus that stress combination leads to a transcriptional response that is distinct from single stress treatments and cannot be predicted from experiments using only single treatments (reviewed in Balfagon et al., 2020; Zandalinas et al., 2021b). Regulators of the interaction between several stresses is suggested to include ABA, JA, and ROS (Balfagon et al., 2020). In this context, combined treatments with O3 and HL makes it possible to test the interaction of ROS from two distinct subcellular locations, as O3 will lead to ROS signals from the apoplast and HL from the chloroplast. The O3 and HL interaction could also be of ecological relevance, as days with more light are associated with increased O3 levels (Tiwari et al., 2008).
The availability of Arabidopsis mutants has significantly facilitated the discovery of proteins that act in specific ROS signaling pathways. The EXECUTER proteins act in the chloroplast to mediate 1O2 signaling (Zhang et al., 2014). After HL treatment, in the nucleus Topoisomerase VI acts as a positive regulator of 1O2 responsive genes but as negative regulator of H2O2 regulated genes (Simkova et al., 2012). Similarly, the small zinc finger proteins METHYLENE BLUE SENSITIVITY 1 and 2, regulate 1O2 but not H2O2 responsive genes after HL treatment (Shao et al., 2013). Studies have also revealed key signaling roles for chloroplast-derived metabolites, including triosephosphates, 3′-phosphoadenosine 5′-phosphate (PAP), β-cyclocitral, and methylerythritol cyclodiphosphate (MEcPP) (Leister, 2019). RCD1 (RADICAL-INDUCED CELL DEATH1), a protein that interacts with multiple transcription factors, also forms a regulatory node in signaling and differentially regulates plant responses to ROS from different subcellular sources (Shapiguzov et al., 2019). Several transcription factors regulate various aspects of the HL transcriptional response: heat shock transcription factors regulate the early response (Jung et al., 2013), BBX32 regulate down-regulation of genes associated with defense to pathogens (Alvarez-Fernandez et al., 2021), and HY5 associated with blue light and UV-B receptors (Kleine et al., 2007). A large collection of mutants defective in hormone biosynthesis, perception or down-stream signaling are also available, and for example, ABA biosynthesis or signaling mutants are impaired in expression of HL responsive genes and show increased damage by HL treatment (Galvez-Valdivieso et al., 2009; Huang et al., 2019).
Even though recent discoveries have identified a number of regulators in ROS signaling, there are still large gaps in our understanding how plants perceive and signal the presence of ROS as triggered by different types of external factors, and how plants prioritize between potentially conflicting defense signals. Here we applied O3 and HL treatments together with analysis of marker gene expression and transcript profiles to study the signaling effects elicited by apoplastic versus chloroplastic ROS. We also studied the effect of combined O3 + HL treatments to dissect possible interactions between these treatments. We further performed the combined treatments at different temperatures, as the HL response is strongly influenced by temperature. We show that genes related to cell death regulation were differentially regulated by O3 versus HL, that HL enhanced cell death caused by O3, and HL repressed the effect of O3 on transcriptional regulation of pathogen and cell death related genes.
Materials and Methods
Plant Growth
Seeds of A. thaliana Col-0 and mutants were obtained from Nottingham Arabidopsis Stock Centre or were a gift from Prof. Hannes Kollist pyr pyl 112458 (Gonzalez-Guzman et al., 2012). The coi1-16 and slac1-3 mutants were previously described (Vahisalu et al., 2008; Xu et al., 2015a). In Helsinki, seeds were sown on 1:1 peat: vermiculite, stratified for 3 days, and then grown at 22/19°C (day/night), relative humidity of 70/90% (day/night), under a 12-h light/12-h dark cycle for a week. Subsequently the geminated seedlings were transplanted into new 1:1 peat: vermiculite mixture. All plants were grown in controlled chamber (Weiss Bio1300; Weiss Gallenkamp), at 22/19°C, and relative humidity of 70/90%, under a 12-h light/12-h dark cycle. Light levels in different conditions are outlined in Table 1; Helsinki-1, used 230 μmol of photons m–2 s–1, Helsinki 2 and 3 used 110 μmol of photons m––2 s––1. For O3 and combined O3 + HL experiments, treatments with 1 h 350 nL L––1 O3 were performed with 3-week-old plants. LED white lights1 were placed inside Weiss chambers to provide the HL treatment (1,100 μmol of photons m–2 s–1). The LED lights also increased the temperature at plant level with +10°C (growth condition Helsinki-3), to minimize the influence of temperature experiments were also performed with cooling of the LED lights (growth condition Helsinki-2). For quantification of cell death plants were treated 2 h 300 nL L–1 O3, 1,100 μmol of photons m–2 s–1 or both simultaneously. Subsequently plants were put to 50 ml tubes with 15 ml MilliQ-water and ion leakage was measured 6 h after the start of the treatments with a conductivity meter (Mettler Toledo LE703). In Turku (growth condition Turku, Table 1), plants were grown under 130 μmol of photons m–2 s–1 at 20°C, 8/16 h light/dark cycle, and 50% humidity. Four-week-old plants were shifted to a light intensity of 1,300 μmol of photons m–2 s–1 at 20°C for 1 h, this was associated with an increase in temperature of 3°C.
RNA Isolation and qPCR
Gene expression analysis of selected marker genes was performed with qRT-PCR (Supplementary Table 1 includes primer sequences and primer amplification efficiencies). RNA was isolated with GeneJET Plant RNA Purification Mini Kit (ThermoFisher Scientific). RNA (2 μg) was DNAseI treated and reverse transcribed with Maxima Reverse Transcriptase and Ribolock Rnase inhibitor (ThermoFisher Scientific) and the reaction diluted to the final volume of 100 μl. qPCR was performed in triplicate using 5x HOT FIREPol EvaGreen qPCR Mix Plus (Solis Biodyne). The cycle conditions with Bio-Rad CFX384 were: 1 cycle initiating with 95°C 10 min, 45 cycles with 95°C 15 s, 60°C 30 s, 72°C 30 s and ending with melting curve analysis. Normalization of the data was performed in qBase 2.3 (Biogazelle2), with the reference genes TIP41, YLS8, and SAND for the O3 experiment and PP2AA3, TIP41, and YLS8 in the HL and combined O3 + HL experiments. Primer amplification efficiencies were determined in qBase from a cDNA dilution series. qBase provides the qPCR results as calibrated normalized relative quantity for each gene (Hellemans et al., 2007). To facilitate comparison between treatments, fold induction by treatment was calculated as treatment/control. Statistical analysis of the qPCR was performed on log2 transformed data with t-test, one-way or two-way ANOVA in GraphPad Prism 6.07.
Transcriptome and Cluster Analysis
Raw data from the Affymetrix ATH1-121501 and Agilent Arabidopsis 4 × 44K chips platform was obtained from several data sources. NASCARRAYS-392 (BTH treatment).3 From Gene Expression Omnibus: GSE39385 (SA 3 h); GSE19520 (ABA 3 h); GSE28800 (ABA 6 h); GSE45543 (ABA 6h); GSE5684 (Botrytis cinerea infection); GSE7743 (cry1 and hy5 treated with HL for 6h); GSE14247 (Ethylene 4 h); GSE5615 (Flg22); GSE19109 (lht1); GSE10646 (mkk1 mkk2); GSE32566 (Na2S); GSE18978 (Pseudomonas syringae ES4326); GSE14961 (SA 24 h); GSE6583 (siz1); GSE46107 (wrky40 and wrky63).4 From ArrayExpress: E-ATMX-13 (MeJA).5 Raw data for acd11 (Palma et al., 2010) was obtained from John Mundy. Raw data for stn7, npq4, and tap38 treated with HL for 1 h (Tikkanen et al., 2014). RNA-seq raw data from O3 treatment for 2 h was obtained from Gene Expression Omnibus: GSE61542 (Col-0, C24, and Te) and GSE65740 (Col-0, coi1-16 ein2 sid2, and tga2 tga5 tga6) (Xu et al., 2015a,b). The pre-processing of Affymetrix data was performed with “robust multiarray average” normalization using affy package in R (Gautier et al., 2004). The Agilent microarray data was processed using the “half” background correction method and followed by “quantile normalization” using Limma package in R. RNA-seq data analysis of O3 data was performed with several packages in the JAVA-based client-server system Chipster (Kallio et al., 2011) and as previously described (Xu et al., 2015a). Differential expression for each experiment was computed by log2-base fold changes in a linear model between treatment and control, or between wild type and mutants. The false discovery rate of differentially expressed genes for treatment/control and between-treatment comparisons was based on the Benjamini and Hochberg (BH) method. The genes in Col-0 with a B-score >0 in response to O3 (GSE61542) and HL treatments [GSE46107 and (Tikkanen et al., 2014)] were selected as significantly expressed genes. The shared set of genes with oppositely regulated expression between O3 and HL treatments were extracted as candidate genes for comparing stress responses induced by O3 and HL. The processed data was discretized and clustered using Bayesian agglomerative hierarchical clustering algorithm (Wrzaczek et al., 2010). Gene Ontology (GO) term enrichment was performed using the AgriGO website with advanced settings of “Plant GO slim” in GO type (Du et al., 2010).
High light or excessive light RNA-seq data were downloaded as raw fastq files from GEO using accession IDs (Supplementary Table 2). The quality of fastq files was assessed using FastQC tool version v0.11.8. Raw reads were aligned to Arabidopsis reference genome (TAIR10.51) using STAR aligner version 2.7.8a and the quality of BAM files was checked using RseQC tool. Gene-level expression abundances were estimated using HTSeq tool, union mode (Putri et al., 2022). For identification of differentially expressed genes, raw read counts were imported to R/Bioconductor package Limma version 3.48.3 (Ritchie et al., 2015). Genes with insufficient number of read counts were filtered out before running the statistical test. This was done using filterByExpr() function from edgeR package with default parameters following the Limma package manual (Robinson et al., 2010; Ritchie et al., 2015). Differentially expressed genes with Benjamini-Hochberg FDR < 0.05 and log2 fold change > ±1 were extracted and used to find overlap between different datasets using InteractiVenn (Heberle et al., 2015).
Results
Expression of Light, Reactive Oxygen Species, Ozone, Heat Shock, and Pathogen Responsive Marker Genes in Abiotic, Biotic, and Hormone Treatments
A current limitation in our understanding of plant stress responses is the heterogeneity of experimental conditions used to grow plants for experiments. We collected information on experimental protocols for Arabidopsis HL experiments in Table 1, which included a variety of different growth conditions and day lengths. As intracellular ROS levels are higher in short day grown plants and cell death is regulated differently in short versus long day grown plants (Michelet and Krieger-Liszkay, 2012; Krasensky-Wrzaczek and Kangasjarvi, 2018), it is likely that growth conditions used to generate plants for experiments will influence the responses to subsequent HL treatment. To probe the molecular responses to light/photooxidative stress (initiated from chloroplasts) versus O3 treatments (that initiate ROS signaling from the apoplast), we selected several marker genes used in previous publications related to light and O3 treatments (Xu et al., 2015a), and visualized their expression levels in response to abiotic, biotic, and hormone treatments using data from the Genevestigator database (Figure 1; Hruz et al., 2008). As expected, marker genes used for HL stress (APX2, ELIP2, and ZAT12) were regulated in a majority of HL experiments, although with some exceptions. For example, APX2 was not regulated in Genevestigator accession AT-00812 which contain the data from Huang et al. (2019). Transcript levels for APX2 were also regulated by heat, consistent with regulation of APX2 by heat shock transcription factors (Jung et al., 2013). Transcript levels for ELIP2 was also regulated by cold, and this marker gene is proposed to have increased expression by several environmental stresses related to photoinhibition (Hayami et al., 2015). ZAT12 transcript levels were regulated by all abiotic and biotic treatments, accordingly ZAT12 is often referred to as a general ROS marker gene (Lim et al., 2019). To investigate the regulatory context of APX2, ELIP2, and ZAT12 we used the updated version of the Arabidopsis Coexpression Tool (Zogopoulos et al., 2021), which identifies genes that are co-expressed with the target gene (Supplementary Figure 1). Consistent with the regulatory context from Genevestigator (Figure 1), APX2 was co-regulated with numerous heat shock proteins (Supplementary Figure 1); ELIP2 was co-regulated with many flavonoid biosynthesis genes that lead to production of pigments that protect against light and oxidative stress (Ferreyra et al., 2021); and ZAT12 with genes related to biotic stress and hormone responses. Collectively, this suggests that using these three marker genes will report on different parts of the Arabidopsis transcriptional response to HL stress.
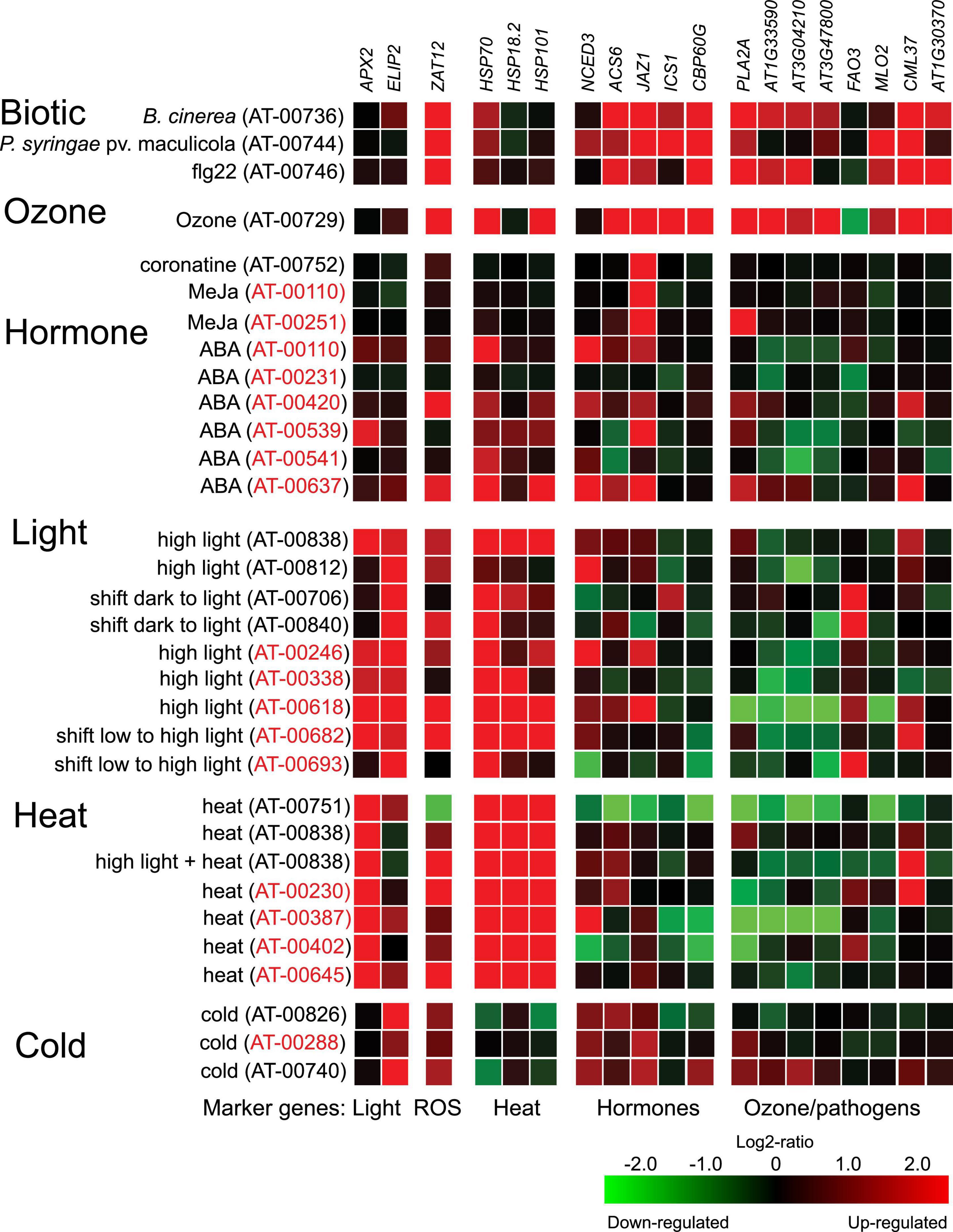
Figure 1. Expression of marker genes in publicly available transcriptome data. Experiments from the Genevestigator perturbation tool were selected to include O3, HL, heat, cold, hormones, and biotic stress treatments. The identification number for each experiment refers to the identifier in the Genevestigator database. Samples with identifiers in red comes from Affymetrix ATH1 array and in black from RNA-seq experiments.
Expression of Light and Heat Marker Genes in Response to High Light or Ozone Treatment
In our experimental design, we aimed to address these questions: (1) How robust is the transcriptional response with different stress marker genes across different growth conditions? and (2) What is the difference and is there an interaction between external (apoplastic) ROS and chloroplast (HL) initiated signaling? We performed qPCR analysis using plant material obtained from different conditions, which differed with respect to the growth light intensity, photoperiod, age of the plants, and to which extent the temperature increased during the HL treatment (Table 1). While ELIP2 transcript levels increased in all different HL treatments, APX2 and ZAT12 transcript levels were more variable between different growth conditions (Figure 2). APX2 transcript levels was shown to depend on the temperature associated with the HL treatment, when the HL treatment was associated with a higher increased temperature this led to substantially increased transcript levels (Jung et al., 2013; Huang et al., 2019). In our experiments, APX2 transcript levels was not higher when the HL treatment was associated with a +10°C increased temperature (growth condition Helsinki-3), suggesting that additional environmental factors also influence APX2 transcript levels. In response to O3 (1 h, 350 nL L–1), transcript levels for the general ROS marker ZAT12 increased, but there was no change in light marker genes APX2 and ELIP2 (Figure 2). This suggests that regulation of APX2 and ELIP2 transcript levels respond to signals that originate from inside the cell (chloroplast) and not from the outside (apoplast). In the combined treatment O3 + HL, there was no obvious interaction for these marker genes.
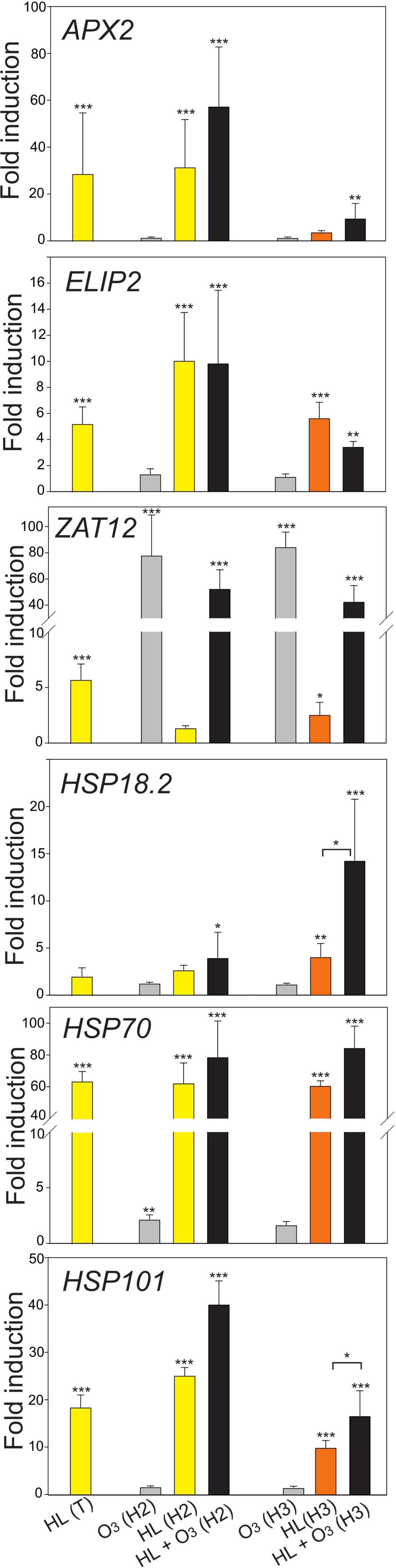
Figure 2. Expression of HL and heat stress marker genes, displayed as fold induction after 1 h HL, O3 or combined treatment. For exact experimental conditions see Table 1, T, Turku; H2, Helsinki 2; H3, Helsinki 3. The average of three biological replicates are shown; error bars depict standard deviation. Two-way ANOVA with Tukey’s test was used for statistical analysis and depicts significant differences compared to Col-0 control (*P < 0.05; **P < 0.01; ***P < 0.001).
As the difference in growth conditions Helsinki 2 and 3 was the extent of increased temperature associated with the HL treatment, we further tested several maker genes for heat shock responses. Transcript levels for HSP70 and HSP101 increased in all HL treatments (Figure 2), with no obvious effect of different temperatures. After O3 treatment there were no or only weak transcriptional changes for the heat shock marker genes. In contrast, for the combined O3 + HL treatment there was increased transcript levels of HSP18.2 and HSP101, especially at increased temperatures (growth condition Helsinki-3). This indicates that unlike the light stress marker gene ELIP2, regulation of heat shock genes respond to signals from both inside and outside the cell. Collectively our results with different light, ROS and heat shock marker genes suggests that several different marker genes should be used when testing for HL stress molecular responses. Further, the combined O3 + HL treatment, showed the presence of genes that are independent for combined treatment (ELIP2) and those that show a synergistic effect (HSP18.2 and HSP101).
The Robust High Light Molecular Response
To probe HL molecular responses, multiple different experimental set-ups have been used (Table 1), and as we show in our qPCR experiments, different growth conditions and the specifics of the HL treatment impact on the transcript levels for HL marker genes (Figures 1, 2). To further explore the role of differences in growth and experimental conditions (Table 1), we used all publicly available RNA-seq experiments from HL treatments (Crisp et al., 2017; Balfagón et al., 2019; Huang et al., 2019; Zandalinas et al., 2019b,2020, 2021b; Fichman et al., 2020; Alvarez-Fernandez et al., 2021). These experiments used different experimental designs with various mutants, time points and local versus systemic signaling; in our re-analysis we used only wild type (Col-0) samples at time points up to 7 h. In experiments with local versus systemic signaling, we used only the local treatment, i.e., the leaves that directly received HL treatment. We processed raw data through the same bioinformatics pipeline (see section “Materials and Methods”), and selected genes with FDR P-value < 0.05 and a twofold up or down regulation. Here it should be noted that in the publications above, some used the twofold cut-off while others did not, and since we applied this cut-off, our number of HL regulated genes were substantially less compared to the original published analysis (Supplementary Table 2). In addition, the software used to identify differentially expressed genes also has an impact on the number of differentially expressed genes found (Seyednasrollah et al., 2015; Corchete et al., 2020). As can be seen from Table 1, the RNA-seq data comes from a wide variety of growth conditions, light periods, light treatments, and plant ages. However, if it is possible to find a set of HL regulated genes that are common in these datasets, it would point toward a robust molecular response that is independent of growth conditions and plant ages. As we expected to see different genes at different time points, we focused on comparisons of similar time points (Figure 3).
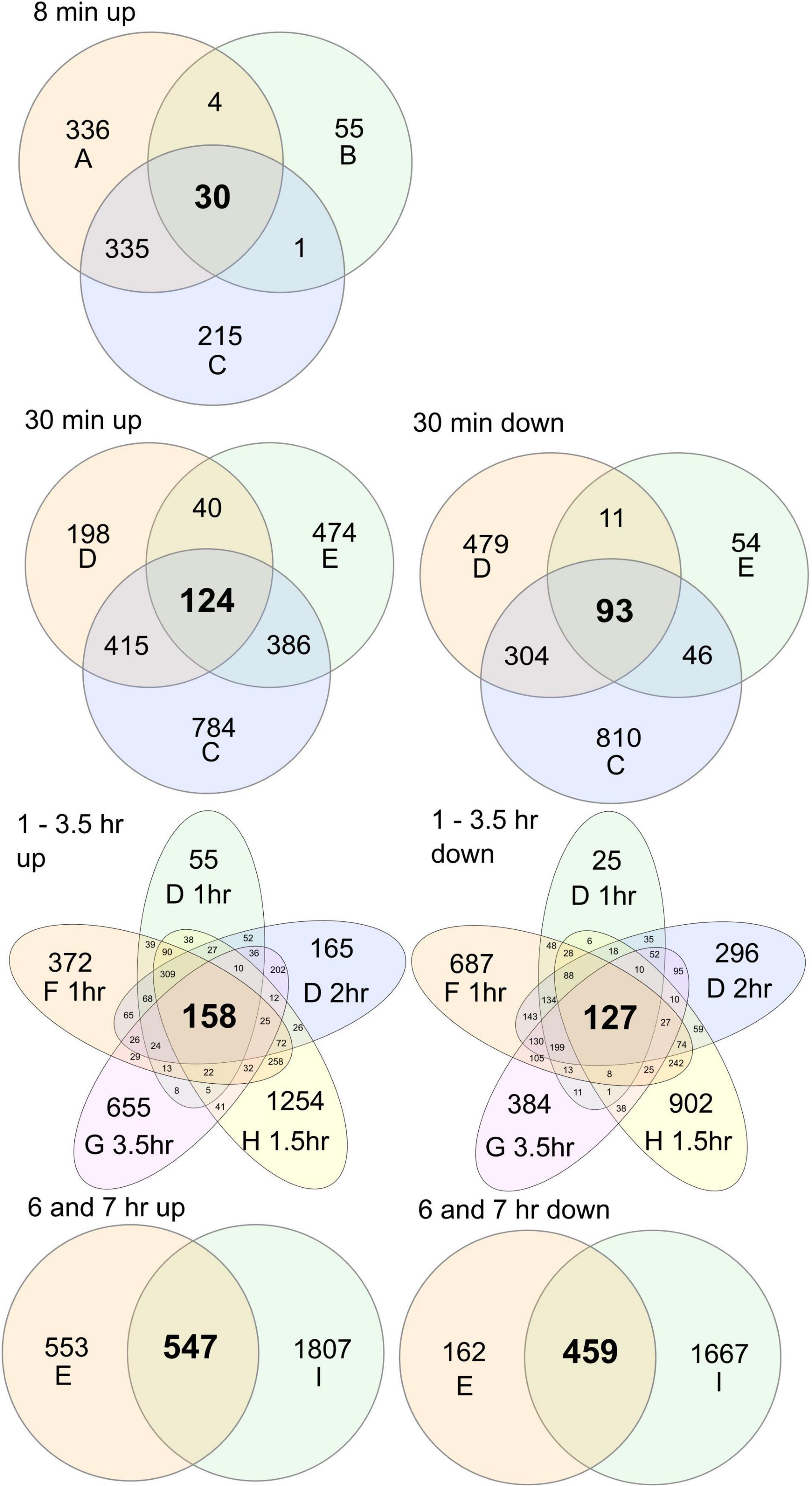
Figure 3. Overlap of differentially expressed genes in different high light RNA-seq datasets. Raw data was processed with the same analysis pipeline and differentially expressed genes selected at FDR corrected P < 0.05 and a cut-off log2 ± 1 (see Supplementary Table 2 for gene lists). For experimental summary of the datasets, see Table 1 and the original publications (referred to as A–I in figure): A, Zandalinas et al., 2019b; B, Zandalinas et al., 2020; C, Fichman et al., 2020; D, Crisp et al., 2017; E, Huang et al., 2019; F, Crisp et al., 2017; G, Alvarez-Fernandez et al., 2021; H, Zandalinas et al., 2021b; I, Balfagón et al., 2019.
At the very early time point, 8 min, there were 30 common up-regulated genes from three datasets. These included APX2, 10 heat shock proteins and the heat shock transcription factor HsfA2, previously shown to be an important regulator of HL stress responses including regulation of APX2 transcription (Jung et al., 2013). At 8 min, there were also increased transcriptional levels for ethylene biosynthesis ACS6 and four ethylene response factors. In the 30 min time point, there were 124 common up-regulated genes from three datasets. These included the HsfA2 and HsfA3 transcription factors, but surprisingly only one heat shock protein. A large number of heat shock proteins were found in the Fichman et al. (2020) (30 min) and Crisp et al. (2017) (30 min) data, but not in the Huang et al. (2019) (30 min) data, suggesting that the latter has some key difference in its experimental conditions. At 30 min, we also found the general ROS marker ZAT12, and the early light inducible ELIP1.
Three transcription factors were found in common for 30 min, which were also up-regulated at all of the other later time points (1, 1.5, 2, 3.5, 6, and 7 h): BBX32, NAC13, and DREB2A. As this suggest their crucial role, we will discuss them in more detail later. In the next comparison, we compared time points from 1 to 3.5 h, where we found 158 common up-regulated genes from five datasets. These included 18 heat shock proteins along with the HsfA2 and HsfA3 transcription factors. Finally, we compared the 6 and 7 h time points, where we found 547 common up-regulated genes. This included many enzymes from the flavonoid biosynthesis pathways including CHS, CHI, DFR, F3H, and FLS1, as well as the transcription factors that regulate their expression PAP1, MYB11, MYB111, and TT8. This suggest the coordinated regulation for production of protective pigments to screen HL (Ferreyra et al., 2021). Relatively few heat shock proteins were found (four) and the transcription factor HsfA2 was not found at the late time points, indicating that transcriptional regulation of heat shock proteins is an early response to HL stress. Several regulators of JA responses (JAZ5, JAZ9, and JAZ13), biosynthesis of the volatile methyl jasmonate (JASMONIC ACID CARBOXYL METHYLTRANSFERASE) and marker gens for JA signaling (VSP1 and VSP2) were up-regulated at the late time points. While we focused on the common up-regulated genes in the RNA-seq datasets, we also noted hundreds of differentially expressed genes that were unique for each dataset (Figure 3), this suggest that the plant molecular response to HL stress is largely shaped by its growth conditions.
The Role of Abscisic Acid in Regulation of Light and Heat Stress Marker Genes
Several plant hormones, including ABA, JA, and SA are proposed regulators of HL signaling (Galvez-Valdivieso et al., 2009; Balfagón et al., 2019; Beaugelin et al., 2019; Huang et al., 2019). To evaluate the role of ABA signaling we used a strongly ABA insensitive mutant that lack six ABA receptors pyr1 pyl1 pyl2 pyl4 pyl5 pyl8 [from here on abbreviated as pyr/pyl112458 (Gonzalez-Guzman et al., 2012)]. In Turku growth condition (Table 1), light stress regulation of transcript levels for ELIP2 and HSP70 were significantly lower in pyr/pyl112458 (Figure 4). Transcript levels for APX2 followed the same trend, but did not reach statistical significance. In contrast, increased transcript levels for HSP101 was independent of ABA signaling (Figure 4). We conclude that the molecular response to light stress is regulated by both ABA dependent and independent signaling pathways.
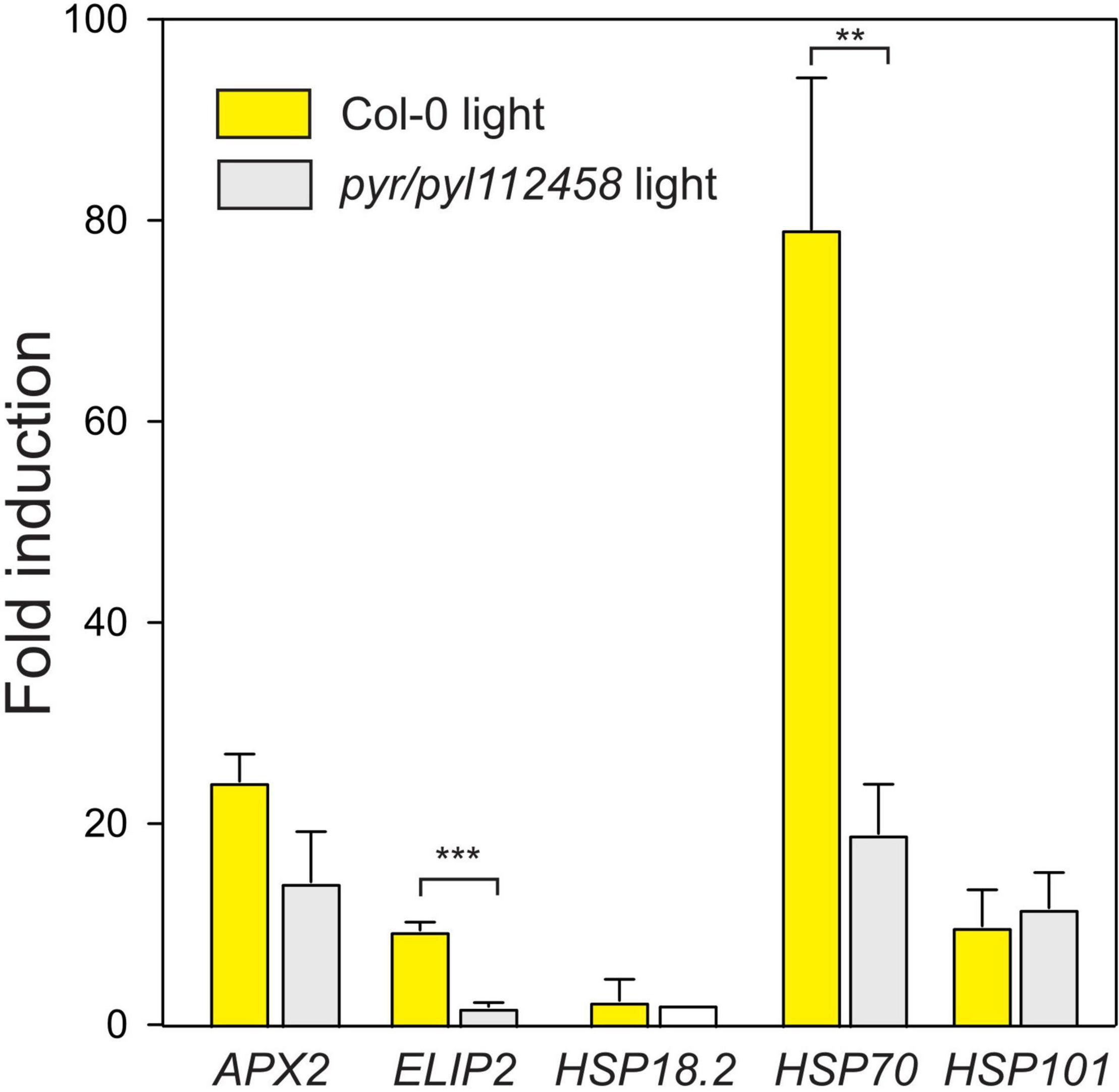
Figure 4. Fold induction of HL and heat stress marker genes after 1 h HL in Col-0 and pyr/pyl112458 (growth condition Turku, Table 1). The average of three biological replicates are shown; error bars depict standard deviation. The t-test was used for statistical analysis and depicts significant differences between Col-0 and pyr/pyl112458 (**P < 0.01; ***P < 0.001).
Expression of Hormone Marker Genes in Response to High Light or Ozone Treatment
Plant stress responses are intimately associated with several stress hormones. To follow different signaling pathways, we used marker genes related to ABA, ethylene, JA, SA, and cell death signaling (Figures 1, 5). These were tested in plants treated with 1 h HL (growth condition Turku) or 1 h O3 (growth condition Helsinki-1), in Col-0 and pyr/pyl112458. Transcript levels of the JA marker JAZ1 increased in HL. The ABA marker gene NCED3 was slightly elevated. However, based on NCED3 transcript levels in several different light treatments (Figure 1), it appears that this marker gene is dependent on additional environmental factors. In contrast, the ethylene marker ACS6 and the SA marker ICS1 were not altered by HL and transcript levels of the cell death marker PLA2A significantly decreased (Figure 5).
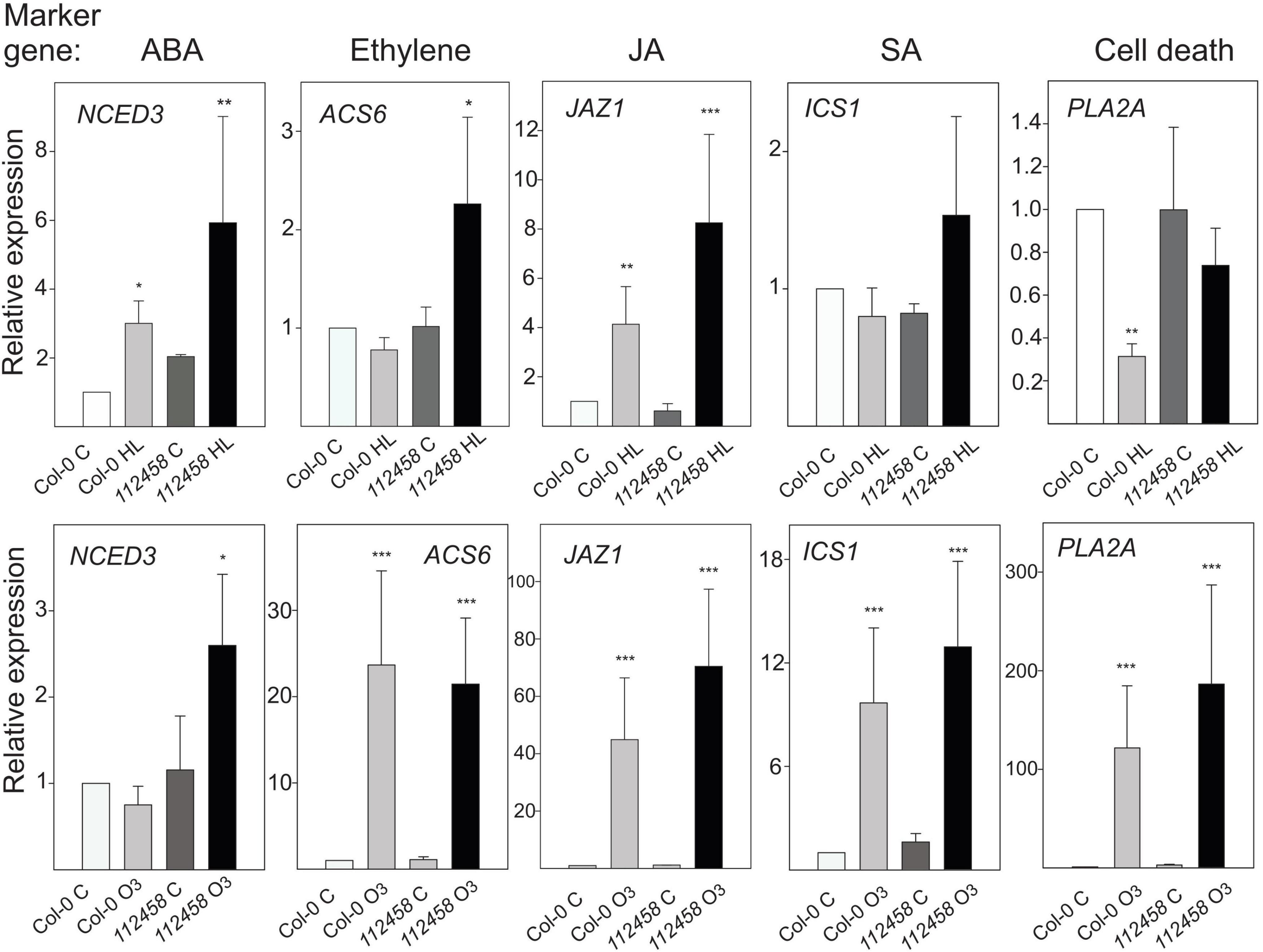
Figure 5. Relative expression scaled to the Col-0 control (set to 1), after 1 h HL (growth condition Turku, Table 1) or 1 h O3 (350 nL L–1, growth condition Helsinki-1) in Col-0 and pyr/pyl112458. The average of three biological replicates are shown; error bars depict standard deviation. Two-way ANOVA with Tukey’s test was used for statistical analysis and depicts significant differences compared to Col-0 control (*P < 0.05; **P < 0.01; ***P < 0.001).
After activation of apoplastic ROS signaling by O3, we observed higher transcript abundance of the ethylene marker gene ACS6, the SA marker gene ICS1, and the JA marker JAZ1 (Figure 5). There was no apparent influence of ABA signaling on the apoplastic ROS response since pyr/pyl1112458 displayed similar responses compared to wild type, with one exception – increased expression levels of NCED3 was only observed by O3 in pyr/pyl1112458, possibly due to some feedback mechanism when ABA signaling is impaired. The cell death marker PLA2A showed the most contrasting behavior, i.e., very high transcript abundance in response to O3, but decreased transcript levels in response to HL.
Identification of Genes Differentially Regulated Between Ozone and High Light
The transcriptional regulation of PLA2A represents an interesting case where HL and apoplastic ROS have opposite results (Figure 5). As this suggests the existence of very divergent signaling pathways, i.e., a light/chloroplast signal that leads to down-regulation and an apoplastic ROS/O3 signal that leads to up-regulation of the same gene, we searched for additional genes with this transcriptional profile and their biological context.
To identify additional genes, we re-analyzed transcriptome datasets generated with arrays or RNA-seq after HL and O3 treatments (Kleine et al., 2007; Van Aken et al., 2013; Tikkanen et al., 2014; Xu et al., 2015a,b). We used conservative selection criteria that the genes should be significantly regulated in two independent HL experiments. We identified 160 genes with significantly altered expression (Supplementary Table 3). Of these, 136 had increased transcript levels by O3 and reduced transcript levels by HL; and 24 genes with increased transcript levels by HL and reduced transcript levels by O3. We used Bayesian hierarchical cluster analysis to analyze similarities and differences between different O3 and HL experiments (Figure 6). Two main clusters were identified which represents the contrasting conditions: O3 up, HL down and vice versa.
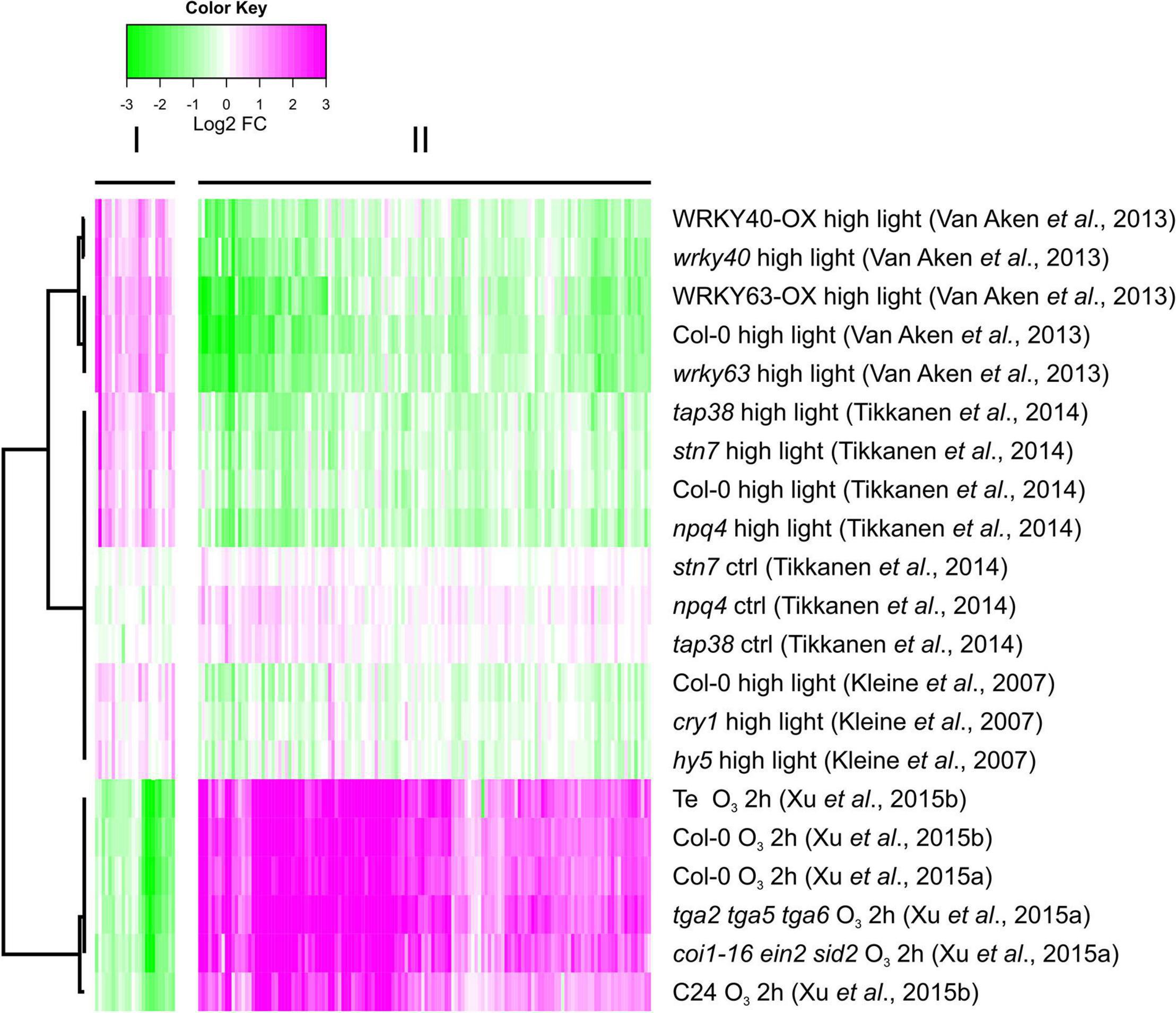
Figure 6. Genes with contrasting expression between HL and O3 – increased expression by O3, decreased expression by HL or vice versa, were identified from several O3 RNA-seq and array HL experiments. 160 genes were found (Supplementary Table 3), and subjected to bootstrapped Bayesian hierarchical clustering in log2-transformed fold changes from O3 RNA-seq data and HL array experiments. Magenta and green indicate increased and decreased expression compared with untreated or wild type plants, respectively.
The Biological Context of Genes With Contrasting Transcriptional Profiles in Response to Ozone or High Light
Next we performed GO enrichment on the genes contrasting transcriptional responses. For the 136 genes with increased transcript levels by O3 and decreased transcript levels after HL there was enrichment of multiple biological processes related to stress, pathogen responses and cell death (Supplementary Table 4). In the GO category molecular function, kinase, and signal transduction were enriched. In the list of 24 genes with decreased transcript levels by O3 and increased transcript levels by HL, there was an enrichment of biological processes related to regulation of metabolic processes, transcription and gene expression; and in molecular function there was an enrichment for transcription factors (Supplementary Table 4).
Reactive oxygen species acts as signaling molecules in defense against pathogens and in regulation of cell death. We analyzed expression levels in transcriptome datasets from several pathogen infections and lesion mimic mutants that undergo spontaneous cell death (Figure 7). For comparison, two of the HL datasets and one of the O3 samples were included. Interestingly, a majority of the genes that had increased transcript levels by O3 (and decreased transcript levels by HL) also had increased transcript levels in response to P. syringae infection, flg22 treatment and in the mutants acd11 and mkk1 mkk2 that undergo spontaneous cell death. Thus, both GO enrichment and the expression profile of genes with contrasting O3 versus HL transcriptional profiles, indicated that they have a role in defense responses, particularly related to pathogen infection and cell death. As the HL regulation of these genes were opposite to O3, pathogen and cell death, this suggests the possibility of a signal from the chloroplast that could interact with other ROS signaling pathways.
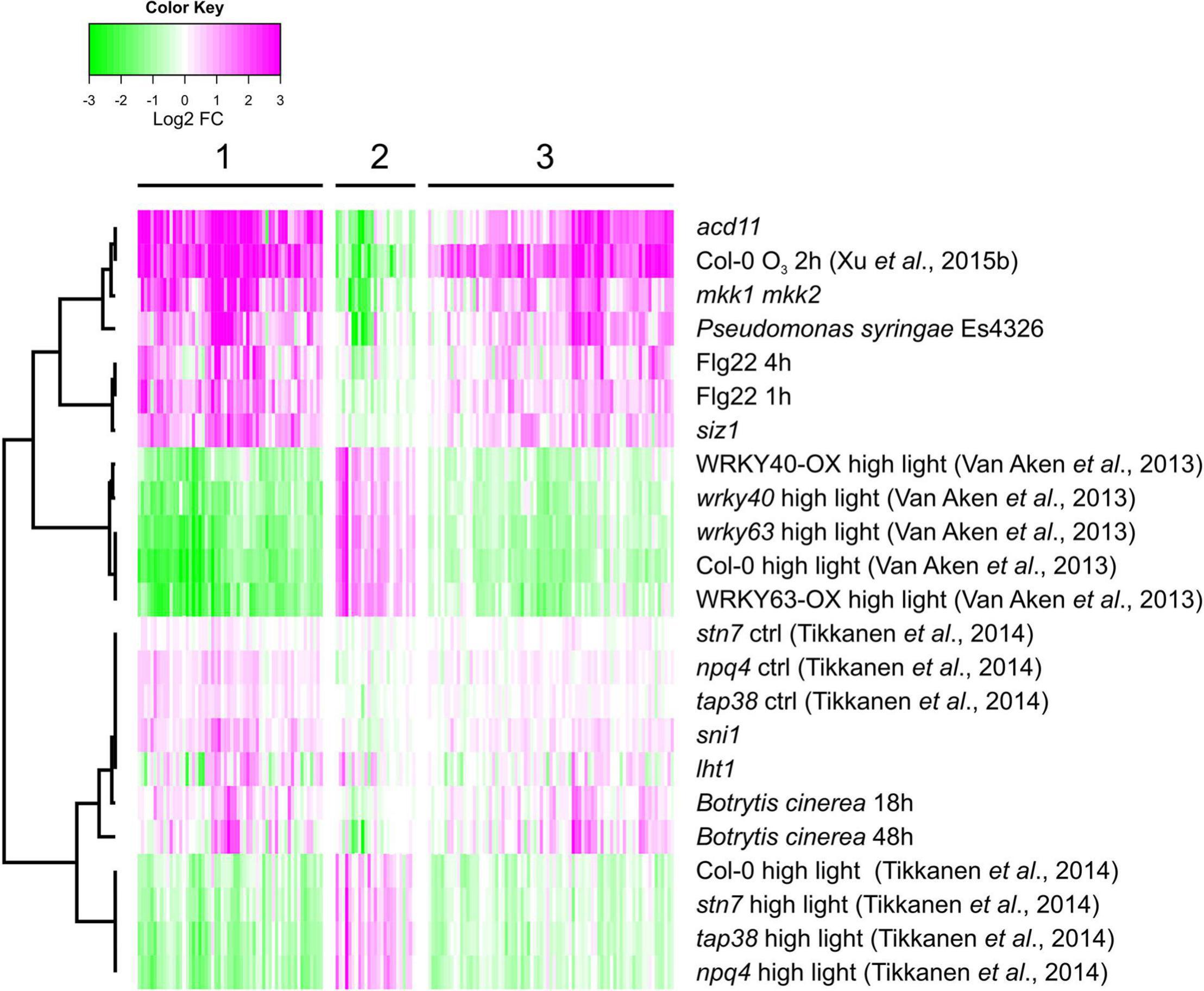
Figure 7. Pathogen regulation of genes with contrasting expression between HL and O3. Gene expression data sets include treatments with pathogens or flg22. In addition, mutants undergoing spontaneous cell death were included (see section “Materials and Methods” for full list of experiments). Genes were subjected to bootstrapped Bayesian hierarchical clustering of log2-transformed fold changes. Magenta and green indicate increased and decreased expression compared with untreated or wild type plants, respectively.
Confirmation of Genes With Contrasting Ozone and High Light Transcriptional Responses
The HL datasets used in the cluster analysis contained a variety of different time points and growth conditions (Table 1). To further support the results of the cluster analysis we selected several genes, four with increased transcript levels by O3 and decreased transcript levels by HL and one gene with decreased transcript level by O3 and increased transcript level by HL. These were tested in qPCR at 1 h time point in Col-0 and pyr/pyl112458. All five genes behaved as expected in the O3 experiment (Supplementary Figure 2). In HL the four genes with decreased expression levels were reproduced (At1g33590, At3g02410, At3g47800, and MLO2). For the last gene with increased expression level in HL (FATTY ALCOHOL OXIDASE 3 – FAO3), it had higher transcript abundance which did not reach statistical significance (Supplementary Figure 2). We included these genes in our analysis of datasets from Genevestigator (Figure 1). Also, in this analysis the selected genes behaved as expected, At1g33590, At3g02410, At3g47800, and MLO2 had decreased transcript levels and FAO3 increased transcript levels in multiple different HL datasets (Figure 1). We observed that At1g33590, At3g02410, and At3g47800 had decreased transcript levels in multiple datasets from ABA treatments (Figure 1). Results from the pyr/pyl112458 mutant suggested that HL down regulation of At1g33590 and MLO2 could require functional ABA signaling (Supplementary Figure 2).
The main focus of the meta-analysis of gene expression data was to identify genes with opposite regulation by O3 versus HL. However, we also identified genes with similar regulation of transcript abundance (Supplementary Table 5). Among genes with increased transcript levels by both treatments were JA biosynthesis and signaling genes (AOC3, LOX4, JAZ1, and JAZ6) and ROS response genes (ZAT10 and ZAT12). The expression of these genes was consistent with the qPCR results (Figures 2, 5). Numerous GO categories associated with abiotic stress, ROS signaling and HL responses but not cell death were associated with genes with increased expression levels by both O3 and HL (Supplementary Table 5).
Combined Ozone and High Light Treatments
To directly test the interaction between apoplastic ROS (O3) and chloroplast signals (HL), we used HL treatments inside our O3 chambers (growth conditions Helsinki-2 and 3, Table 1 and Figures 2, 8). For the heat stress markers genes, but not light stress marker genes, we observed synergistic effects between HL, O3, and increased temperature (Figure 2). We expanded this analysis to the hormone marker genes to get further information on potential interaction between the apoplast and chloroplast signaling pathways. Based on the GO analysis and cluster analysis (Figure 7), we also included three genes with increased transcript abundance early after pathogen treatment At1g30370, CML37, and Cbp60g (Jacob et al., 2018). In multiple datasets from Genevestigator, At1g30370 and Cbp60g [which encodes a transcription factor that regulate expression of the SA biosynthesis gene ICS1 (Wang et al., 2011)], had increased transcript levels by biotic stress and O3 and decreased transcript levels by HL (Figure 1). In contrast, CML37 had increased transcript levels by both HL and O3.
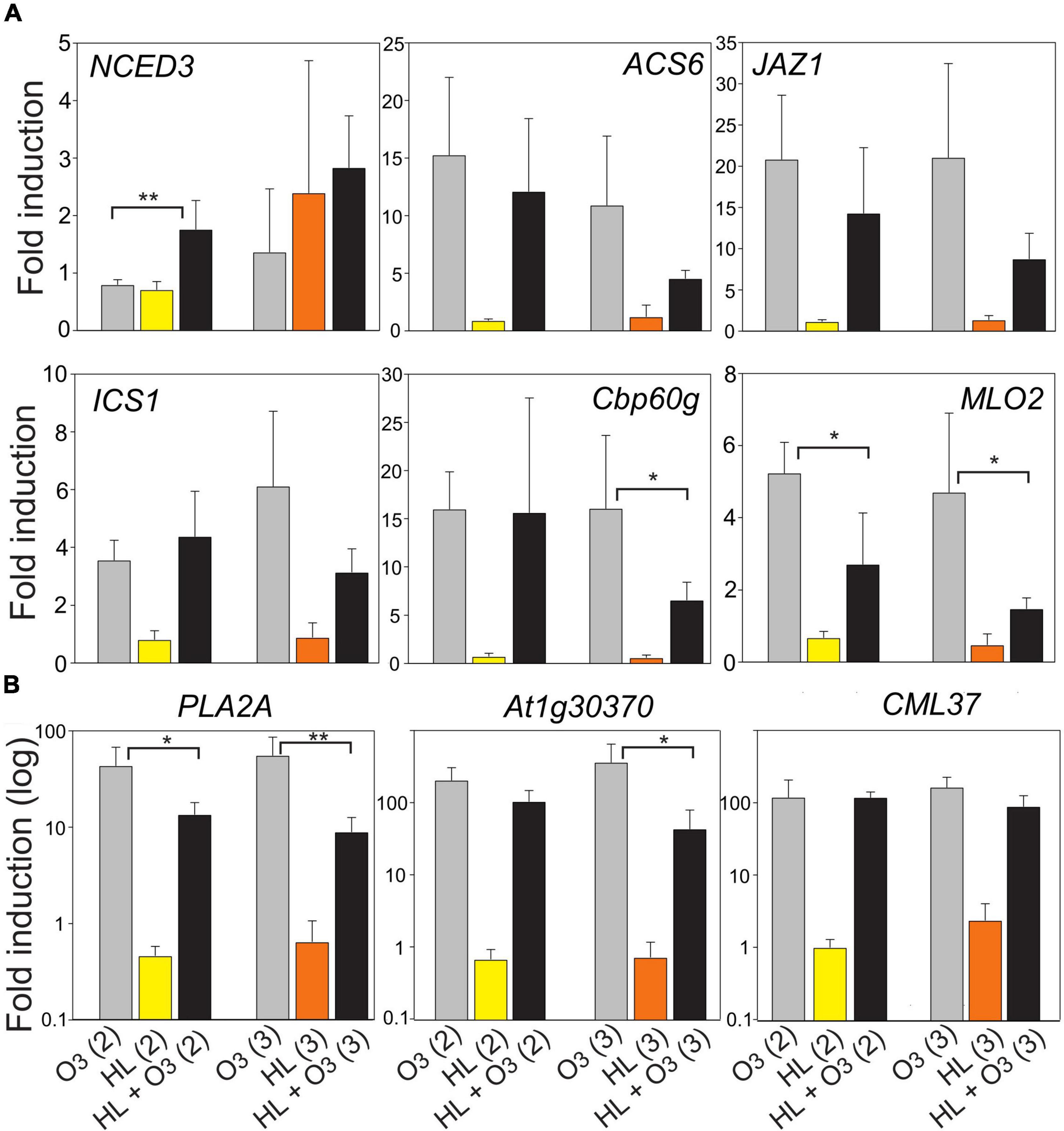
Figure 8. Fold induction of selected marker genes after 1 h HL, 1 h O3 (350 nL L– 1) or combined treatment (growth conditions Helsinki-2 and Helsinki-3, Table 1). Panel (A) has normal scale and panel (B) log10 scale, to better visualize the large differences in transcript levels between samples. The average of four biological replicates are shown; error bars depict standard deviation. The t-test was used for statistical analysis and depicts significant differences between O3 and combined O3 + HL (*P < 0.05; **P < 0.01).
In the combined treatments, there appeared to be a stronger effect when there was also an additional increase in temperature (growth condition Helsinki-3) for the marker genes Cbp60g, MLO2, PLA2A, and At1g30370, in which the combined treatment led to significant reduction in transcript levels compared to O3 alone (Figure 8). This significant reduction in combined treatment was also observed for growth condition Helsinki-2 and the marker genes MLO2 and PLA2A (Figure 8), where the heat component was minimal (Table 1). In addition, there was a trend toward lower transcript levels in combined treatments for ACS6 and JAZ1, although this did not reach statistical significance. We conclude that a signal pathway initiated from the chloroplast (HL treatment) can down regulate transcript levels for pathogen related genes as a single treatment (Figures 1, 7 and Supplementary Figure 2), but also in the combined treatment where this chloroplast signal can modulate and partially block the signaling initiated from the apoplast (O3) (Figure 8).
Regulation of Cell Death After Combined Ozone + High Light
A characteristic response to O3 in sensitive plants is accumulation of ROS leading to induction of cell death (Wohlgemuth et al., 2002). To test the relevance of the interaction between O3 and HL, and if HL modulates O3 cell death, we measured cell death in Col-0 at 3 and 6 h after the combined treatment (Figure 9A, growth condition Helsinki-3). We quantified cell death as ion leakage and observed an increase in cell death only at 6 h in the combined treatment.
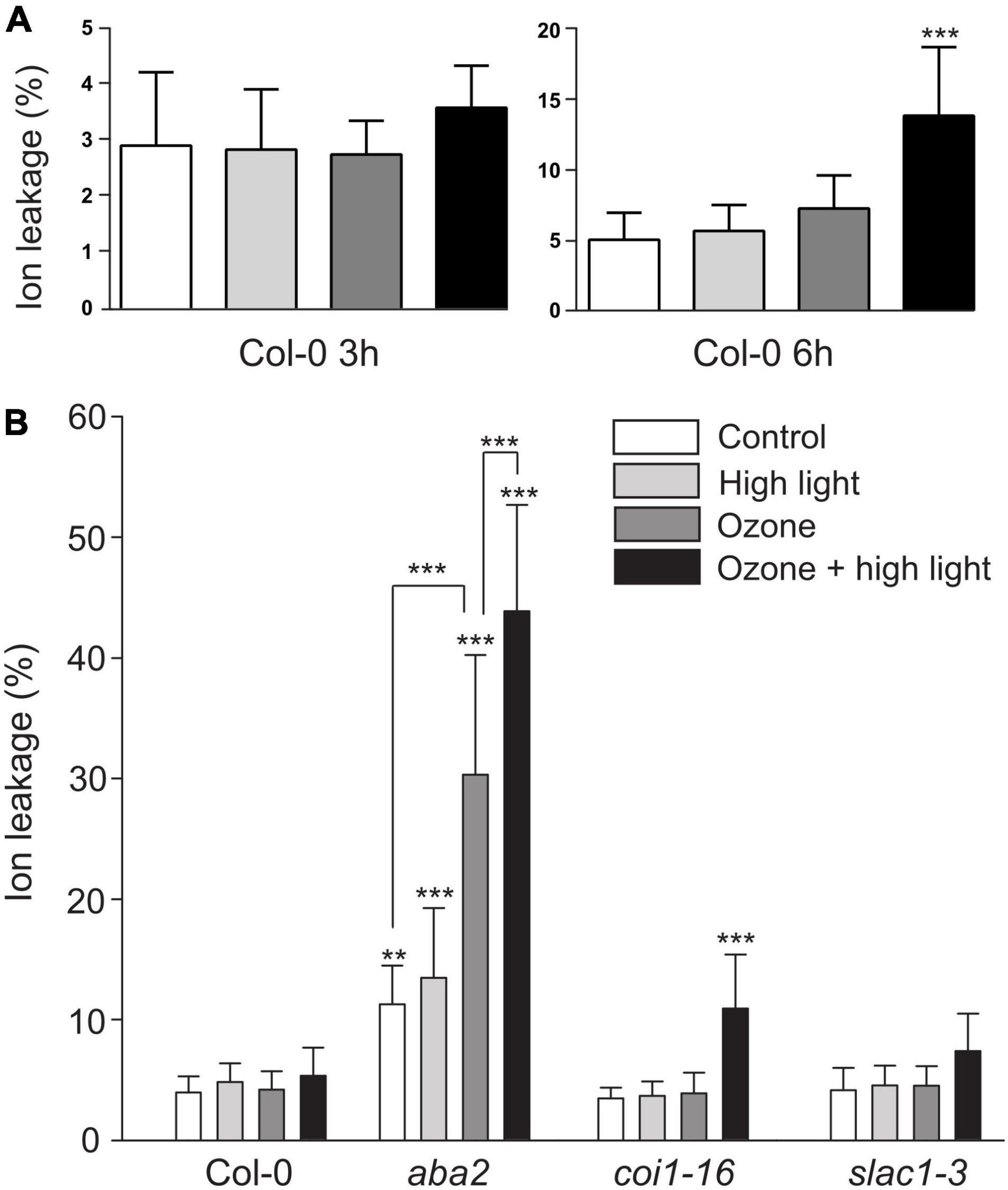
Figure 9. Cell death after combined O3 and HL. (A) Cell death quantified with ion leakage after 3 or 6 h of HL, O3 (350 nL L– 1) or combined treatment (growth condition Helsinki-3). (B) Plants treated with 2 h of HL, O3 or combined treatment followed by 4 h in control conditions and quantification of cell death. The average of three biological replicates are shown (N = 15); error bars depict standard deviation. One-way or two-way ANOVA with Tukey’s test was used for statistical analysis and depicts significant differences compared to Col-0 control (*P < 0.05; **P < 0.01; ***P < 0.001).
Jasmonic acid is a regulator of O3 cell death (Xu et al., 2015a), and ABA a regulator of HL cell death. To test the role of these hormones in the O3 + HL interaction we used aba2, a strong ABA biosynthesis mutant and coi1-16, a mutant for the JA receptor. As an additional control, we included the O3 sensitive slac1 as this mutant is O3 sensitive independent of hormone signaling due to more open stomata that allow high O3 uptake (Vahisalu et al., 2008). In a first trial, the combined treatment severely damaged all three mutants, preventing precise quantification of cell death. Therefore, we used a shorter 2 h treatment plus recovery at 4 h in control conditions followed by quantification of cell death. In this scheme with a lower O3 dose, mutants that previously were shown to be O3 sensitive [slac1, coi1 (Vahisalu et al., 2008; Xu et al., 2015a)], did not show damage by O3 alone (Figure 9B). In contrast, aba2 showed higher ion leakage already in control conditions, possibly due to the permeable cuticle of ABA deficient mutants (Cui et al., 2016). The aba2 mutant was also highly O3 sensitive, which might be related to the very high stomatal conductance of this mutant (Merilo et al., 2018). In the combined treatment, HL + raised temperature increased the damaging effects of O3 in aba2 and coi1 (Figure 9). Thus, a combined stress treatment cause more damage than O3 alone.
Discussion
The Role of Reactive Oxygen Species in Plant Signal Networks
Signaling pathways in plants are highly responsive to ROS produced in different cellular compartments, but the mechanisms underlying appropriate ROS-induced responses upon biotic and abiotic challenges are only starting to emerge (Castro et al., 2021). The ROS signaling network is integrated with the stress hormone signaling network: in O3 (apoplastic ROS), SA and ethylene promotes cell death and JA is protective (Xu et al., 2015a); in HL induced cell death, JA and SA promotes cell death (Laloi and Havaux, 2015; Beaugelin et al., 2019). However, assigning specific and clear-cut roles for hormones is often an over simplification, since growth conditions, genetic background, or stress severity can change the extent of cell death. For example, SA can also protect against O3 (Xu et al., 2015b) and HL induced cell death (Lv et al., 2015). Most likely, the balance between different signaling pathways determines the outcome of a stress treatment.
As plants in nature are likely to experience multiple altered growth conditions simultaneously, using experimental set-ups where two stress conditions interact can further help to understand the priority and interaction between different signaling pathways. Meta-analysis of array and RNA-seq data from different stress combinations show that all stress combinations tested so far result in transcriptome changes that are unique for each specific stress combination (Zandalinas et al., 2019a). This indicates that plants are highly responsive to changes in the environment with corresponding activation of signaling pathways that integrate multiple sources of signals. Site specific ROS production could be one of the ways that plants use to activate different signaling pathways; demonstrated by methylene blue sensitivity mutants, which show impaired HL regulation of 1O2 regulated genes but not H2O2/O2.– regulated genes (Shao et al., 2013).
This study further illustrates that signals from different subcellular compartments have different signaling roles, since O3 and HL had very different outcomes on expression of several marker genes (Figures 1, 5). In particular, the cell death marker PLA2A showed opposite regulation – increased transcript levels by O3 and decreased transcript levels by HL. Additional genes with contrasting expression profiles in O3 versus HL were identified from transcriptome datasets and were enriched for pathogen response genes (Figure 7). To directly evaluate the interaction between apoplast and chloroplast signaling, we used combined O3 + HL treatments. While O3 had no effect on HL marker genes, there was a consistent inhibitory effect of HL on O3 regulated genes associated with cell death and pathogen responses (Figure 8). This emphasizes that: (1) apoplastic and chloroplastic ROS activate distinct signaling pathways; (2) at least one signal initiated from HL converge with the apoplastic ROS signal to regulate changes in transcript levels for genes related to pathogen infection and cell death.
The Impact of Different Growth Conditions on Plant Stress Responses
A challenge in interpretation of results from plant stress studies is the variety of different growth conditions used in research with Arabidopsis. In one of the few studies that directly attempted to replicate similar growth conditions in ten different laboratories, revealed significant changes in growth and metabolite profiles due to subtle variations in growth conditions (Massonnet et al., 2010). For HL experiments, considerably different growth conditions and HL treatments have been used (Table 1), which in turn is likely to give difference in molecular responses measured as altered transcript levels (Figure 3). These experiments used a wide range of plant ages and different light periods (constant light, 16:8, 12:12, or 8:16 light/dark). Another critical factor in HL experiments is the potential increase in temperature associated with the HL treatment. For example, the HL marker gene APX2 show very high transcript levels when the HL treatment is combined with increased temperature (Jung et al., 2013; Huang et al., 2019). Even small increases in temperature are monitored by plants through PhyB and PIF4 (PHYTOCHROME INTERACTING FACTOR 4), and PIF4 acts a negative regulator of transcript levels for pathogen defense related genes (Legris et al., 2016; Gangappa et al., 2017). Analysis of HL RNA-seq data from contrasting growth conditions (Table 1 and Figure 3), gives further support for both a robust response to HL and growth condition specific HL responses. We suggest that a robust molecular response to HL stress require a core set of transcription factors to execute the transcriptional regulation. We found consistent up-regulation of HsfA2 (and to lesser extent HsfA3) in all data-sets for early time points from 8 min to 3.5 h; and up-regulation of NAC13, DREB2A, and BBX32 in all data-sets from 30 min to 7 h. This is consistent with the proposed role for HsfA2/HsfA3 as key positive regulators for early HL responses (Jung et al., 2013); and the newly established role for BBX32 as a key negative regulator of HL responses (Alvarez-Fernandez et al., 2021). BBX32 was proposed to act to down-regulate pathogen defense related genes after HL exposure (Alvarez-Fernandez et al., 2021), consistent with our cluster analysis which identified genes up-regulated by O3 treatments, but down-regulated by HL treatment (Figures 1, 7). NAC13 has not previously been associated with HL stress, but this transcription factors is a key regulator of mitochondrial retrograde signaling (De Clercq et al., 2013; Shapiguzov et al., 2019). Its consistent up-regulation across all time points from 30 min to 7 h, suggest that the HL stress response require coordinated responses from both the chloroplast and mitochondria. DREB2A has been extensively characterized for its role in drought stress responses, and it also acts as a regulator of heat stress responses (Sakuma et al., 2006). Accordingly, the consistent up-regulation of DREB2A, HsfA2, and HsfA3 across many time points, suggest their coordinated function to regulate the expression of heat shock proteins.
As changes in the light environment may be one of the most common experiences by plants in nature, it is perhaps not surprising that transcriptional responses to HL is integrated with growth conditions. HL stress has been studied in combination with other stresses including heat (Balfagón et al., 2019), drought (Giraud et al., 2008), and heat, salt and chloroplast ROS generated from methyl viologen (Zandalinas et al., 2021b); where the combined treatments increased the amount of damage compared to single treatments. Higher concentration of O3 in the troposphere is significantly correlated with both increase in temperature and sunshine hours (Tiwari et al., 2008). The impact of O3 pollution on yield of agriculturally important species, including wheat and rice, is higher in field experiments than in pot experiments, pointing toward interactions between O3 and other unknown cues from the environment (Feng et al., 2022). Further identification of signals from the environment that modulate O3 responses is crucial to protect against yield losses from O3 pollution, which can be as high as 30% in wheat (Feng et al., 2022). Here we showed that O3 + HL led to more damage than O3 alone in Arabidopsis (Figure 9), this observation makes it possible to use this model plant to better understand how O3 interacts with other environmental factors including light (Juran et al., 2021).
Retrograde signaling from the chloroplast involve multiple signaling molecules (Leister, 2019). ABA is critical for plant drought and cold responses, and is an important signaling molecule in response to HL based on several evidences. HL treatment led to increased expression of ABA biosynthesis enzymes and production of ABA (Galvez-Valdivieso et al., 2009). The ABA biosynthesis double mutant nced3 nced5 showed increased damage after HL treatment (Huang et al., 2019), and HL induction of APX2 and ELIP2 was impaired in ABA biosynthesis and signaling mutants (Fryer et al., 2003; Galvez-Valdivieso et al., 2009; Figure 4). However, the role of ABA in HL signaling also appears to be cell specific and influenced by environmental factors (Gorecka et al., 2014). In contrast, for the marker genes tested here (Figure 5), ABA does not appear to regulate O3 transcriptional responses.
Plants constantly face different signals from the environment that needs to be integrated with developmental programs. Here we have shown that HL activates signaling that can inhibit signaling initiated from the apoplast, which could be used by the plant to prioritize between potentially conflicting defense responses.
Data Availability Statement
The datasets presented in this study can be found in online repositories. The names of the repositories and accession numbers can be found in the Materials and Methods, and in Supplementary Table 2.
Author Contributions
MB conceived and designed the experiments and wrote the manuscript. EX, MT, and MB performed the experiments. EX, MT, FS, SK, and MB analyzed the data. All authors read and approved the final manuscript.
Funding
This work was supported by the Academy of Finland (#135751, #140981, and #273132 to MB), the Centre of Excellence (CoE) in the Molecular Biology of Primary Producers (2014-2019) (decision #271832 and 307335); and the Academy of Finland’s “Thriving Nature” research profiling action.
Conflict of Interest
The authors declare that the research was conducted in the absence of any commercial or financial relationships that could be construed as a potential conflict of interest.
Publisher’s Note
All claims expressed in this article are solely those of the authors and do not necessarily represent those of their affiliated organizations, or those of the publisher, the editors and the reviewers. Any product that may be evaluated in this article, or claim that may be made by its manufacturer, is not guaranteed or endorsed by the publisher.
Acknowledgments
We thank Gema Flores Andaluz for assistance with RNA isolation; Jaakko Kangasjärvi, Cezary Waszczak Alexey Shapiguzov, Michael Wrzaczek, and Julia Krasensky-Wrzaczek for comments on the manuscript.
Supplementary Material
The Supplementary Material for this article can be found online at: https://www.frontiersin.org/articles/10.3389/fpls.2022.883002/full#supplementary-material
Supplementary Figure 1 | Genes co-expressed with APX2, ELIP2, and ZAT12 were identified with the Arabidopsis Coexpression Tool and displayed in a cladogram (Zogopoulos et al., 2021).
Supplementary Figure 2 | Relative expression scaled to the Col-0 control (set to 1), after 1 h HL (growth condition Turku, Table 1) or 1 h O3 (350 nL L–1, growth condition Helsinki-1) in Col-0 and pyr/pyl112458. The average of three biological replicates are shown; error bars depict standard deviation. Two-way ANOVA with Tukey’s test was used for statistical analysis and depicts significant differences compared to Col-0 control (*P < 0.05; **P < 0.01; ***P < 0.001).
Supplementary Table 1 | Primers used in this study.
Supplementary Table 2 | Differentially expressed genes in HL RNA-seq datasets and the overlap of differentially expressed genes, corresponding to the Venn diagram analysis in Figure 3.
Supplementary Table 3 | Genes with increased expression by O3 and decreased expression by HL (or vice versa), corresponding to the cluster analysis in Figures 6, 7.
Supplementary Table 4 | Gene Ontology (GO) analysis of genes with increased expression by O3 and decreased expression by HL (or vice versa).
Supplementary Table 5 | Genes with increased expression by O3 and HL, or decreased expression by O3 and HL.
Footnotes
- ^ www.valoya.com
- ^ https://www.qbaseplus.com/
- ^ http://arabidopsis.info/affy/link_to_iplant.html
- ^ http://www.ncbi.nlm.nih.gov/geo
- ^ http://www.ebi.ac.uk/arrayexpress/
References
Alvarez-Fernandez, R., Penfold, C. A., Galvez-Valdivieso, G., Exposito-Rodriguez, M., Stallard, E. J., Bowden, L., et al. (2021). Time-series transcriptomics reveals a BBX32-directed control of acclimation to high light in mature Arabidopsis leaves. Plant J. 107, 1363–1386. doi: 10.1111/tpj.15384
Balfagón, D., Sengupta, S., Gómez-Cadenas, A., Fritschi, F. B., Azad, R., Mittler, R., et al. (2019). Jasmonic acid is required for plant acclimation to a combination of high light and heat stress. Plant Physiol. 181, 1668–1682. doi: 10.1104/pp.19.00956
Balfagon, D., Zandalinas, S. I., Mittler, R., and Gomez-Cadenas, A. (2020). High temperatures modify plant responses to abiotic stress conditions. Physiol. Plant. 170, 335–344. doi: 10.1111/ppl.13151
Beaugelin, I., Chevalier, A., D’Alessandro, S., Ksas, B., Novak, O., Strnad, M., et al. (2019). OXI1 and DAD regulate light-induced cell death antagonistically through jasmonate and salicylate levels. Plant Physiol. 180, 1691–1708. doi: 10.1104/pp.19.00353
Bechtold, U., Richard, O., Zamboni, A., Gapper, C., Geisler, M., Pogson, B., et al. (2008). Impact of chloroplastic- and extracellular-sourced ROS on high light-responsive gene expression in Arabidopsis. J. Exp. Bot. 59, 121–133. doi: 10.1093/jxb/erm289
Castro, B., Citterico, M., Kimura, S., Stevens, D. M., Wrzaczek, M., and Coaker, G. (2021). Stress-induced reactive oxygen species compartmentalization, perception and signalling. Nat. Plants 7, 403–412. doi: 10.1038/s41477-021-00887-0
Corchete, L. A., Rojas, E. A., Alonso-Lopez, D., De Las Rivas, J., Gutierrez, N. C., and Burguillo, F. J. (2020). Systematic comparison and assessment of RNA-seq procedures for gene expression quantitative analysis. Sci. Rep. 10:19737. doi: 10.1038/s41598-020-76881-x
Crisp, P. A., Ganguly, D. R., Smith, A. B., Murray, K. D., Estavillo, G. M., Searle, I., et al. (2017). Rapid recovery gene downregulation during excess-light stress and recovery in Arabidopsis. Plant Cell 29, 1836–1863. doi: 10.1105/tpc.16.00828
Cui, F. Q., Brosche, M., Lehtonen, M. T., Amiryousefi, A., Xu, E. J., Punkkinen, M., et al. (2016). Dissecting abscisic acid signaling pathways involved in cuticle formation. Mol. Plant. 9, 926–938. doi: 10.1016/j.molp.2016.04.001
De Clercq, I., Vermeirssen, V., Van Aken, O., Vandepoele, K., Murcha, M. W., Law, S. R., et al. (2013). The membrane-bound NAC transcription factor ANAC013 functions in mitochondrial retrograde regulation of the oxidative stress response in Arabidopsis. Plant Cell 25, 3472–3490. doi: 10.1105/tpc.113.117168
Du, Z., Zhou, X., Ling, Y., Zhang, Z. H., and Su, Z. (2010). agriGO: a GO analysis toolkit for the agricultural community. Nucleic Acids Res. 38, W64–W70. doi: 10.1093/nar/gkq310
Feng, Z. Z., Xu, Y. S., Kobayashi, K., Dai, L. L., Zhang, T. Y., Agathokleous, E., et al. (2022). Ozone pollution threatens the production of major staple crops in East Asia. Nat. Food 3, 47–56. doi: 10.1038/s43016-021-00422-6
Ferreyra, M. L. F., Serra, P., and Casati, P. (2021). Recent advances on the roles of flavonoids as plant protective molecules after UV and high light exposure. Physiol. Plant. 173, 736–749. doi: 10.1111/ppl.13543
Fichman, Y., Zandalinas, S. I., Sengupta, S., Burks, D., Myers, R. J., Azad, R. K., et al. (2020). MYB30 orchestrates systemic reactive oxygen signaling and plant acclimation. Plant Physiol. 184, 666–675. doi: 10.1104/pp.20.00859
Frenkel, M., Kulheim, C., Jankanpaaa, H. J., Skogstrom, O., Dall’Osto, L., Agren, J., et al. (2009). Improper excess light energy dissipation in Arabidopsis results in a metabolic reprogramming. BMC Plant Biol. 9:12. doi: 10.1186/1471-2229-9-12
Fryer, M. J., Ball, L., Oxborough, K., Karpinski, S., Mullineaux, P. M., and Baker, N. R. (2003). Control of ascorbate peroxidase 2 expression by hydrogen peroxide and leaf water status during excess light stress reveals a functional organisation of Arabidopsis leaves. Plant J. 33, 691–705. doi: 10.1046/j.1365-313X.2003.01656.x
Gadjev, I., Vanderauwera, S., Gechev, T. S., Laloi, C., Minkov, I. N., Shulaev, V., et al. (2006). Transcriptomic footprints disclose specificity of reactive oxygen species signaling in Arabidopsis. Plant Physiol. 141, 436–445. doi: 10.1104/pp.106.078717
Galvez-Valdivieso, G., Fryer, M. J., Lawson, T., Slattery, K., Truman, W., Smirnoff, N., et al. (2009). The high light response in Arabidopsis involves ABA signaling between vascular and bundle sheath cells. Plant Cell 21, 2143–2162. doi: 10.1105/tpc.108.061507
Gangappa, S. N., Berriri, S., and Kumar, S. V. (2017). PIF4 coordinates thermosensory growth and immunity in Arabidopsis. Curr. Biol. 27, 243–249. doi: 10.1016/j.cub.2016.11.012
Gautier, L., Cope, L., Bolstad, B. M., and Irizarry, R. A. (2004). affy - analysis of Affymetrix GeneChip data at the probe level. Bioinformatics 20, 307–315. doi: 10.1093/bioinformatics/btg405
Giraud, E., Ho, L. H. M., Clifton, R., Carroll, A., Estavillo, G., Tan, Y. F., et al. (2008). The absence of alternative oxidase1a in Arabidopsis results in acute sensitivity to combined light and drought stress. Plant Physiol. 147, 595–610. doi: 10.1104/pp.107.115121
Gollan, P. J., Tikkanen, M., and Aro, E. M. (2015). Photosynthetic light reactions: integral to chloroplast retrograde signalling. Curr. Opin. Plant Biol. 27, 180–191. doi: 10.1016/j.pbi.2015.07.006
Gonzalez-Guzman, M., Pizzio, G. A., Antoni, R., Vera-Sirera, F., Merilo, E., Bassel, G. W., et al. (2012). Arabidopsis PYR/PYL/RCAR receptors play a major role in quantitative regulation of stomatal aperture and transcriptional response to abscisic acid. Plant Cell 24, 2483–2496. doi: 10.1105/tpc.112.098574
Gorecka, M., Alvarez-Fernandez, R., Slattery, K., McAusland, L., Davey, P. A., Karpinski, S., et al. (2014). Abscisic acid signalling determines susceptibility of bundle sheath cells to photoinhibition in high light-exposed Arabidopsis leaves. Philos. Trans. R. Soc. B. Biol. Sci. 369:20130234. doi: 10.1098/rstb.2013.0234
Hayami, N., Sakai, Y., Kimura, M., Saito, T., Tokizawa, M., Iuchi, S., et al. (2015). The responses of Arabidopsis early light-induced protein2 to ultraviolet B, high light, and cold stress are regulated by a transcriptional regulatory unit composed of two elements. Plant Physiol. 169, 840–855. doi: 10.1104/pp.15.00398
Heberle, H., Meirelles, G. V., da Silva, F. R., Telles, G. P., and Minghim, R. (2015). InteractiVenn: a web-based tool for the analysis of sets through venn diagrams. BMC Bioinformatics 16:169. doi: 10.1186/s12859-015-0611-3
Hellemans, J., Mortier, G., De Paepe, A., Speleman, F., and Vandesompele, J. (2007). qBase relative quantification framework and software for management and automated analysis of real-time quantitative PCR data. Genome Biol. 8:R19. doi: 10.1186/gb-2007-8-2-r19
Hruz, T., Laule, O., Szabo, G., Wessendorp, F., Bleuler, S., Oertle, L., et al. (2008). Genevestigator v3: a reference expression database for the meta-analysis of transcriptomes. Adv. Bioinform. 2008:420747. doi: 10.1155/2008/420747
Huang, J., Zhao, X., and Chory, J. (2019). The Arabidopsis transcriptome responds specifically and dynamically to high light stress. Cell Rep. 29, 4186–4199.e. doi: 10.1016/j.celrep.2019.11.051
Jacob, F., Kracher, B., Mine, A., Seyfferth, C., Blanvillain-Baufume, S., Parker, J. E., et al. (2018). A dominant-interfering camta3 mutation compromises primary transcriptional outputs mediated by both cell surface and intracellular immune receptors in Arabidopsis thaliana. New Phytol. 217, 1667–1680. doi: 10.1111/nph.14943
Jung, H. S., Crisp, P. A., Estavillo, G. M., Cole, B., Hong, F. X., Mockler, T. C., et al. (2013). Subset of heat-shock transcription factors required for the early response of Arabidopsis to excess light. Proc. Natl. Acad. Sci. U.S.A. 110, 14474–14479. doi: 10.1073/pnas.1311632110
Juran, S., Grace, J., and Urban, O. (2021). Temporal changes in ozone concentrations and their impact on vegetation. Atmosphere 12:82. doi: 10.3390/atmos12010082
Kallio, M. A., Tuimala, J. T., Hupponen, T., Klemela, P., Gentile, M., Scheinin, I., et al. (2011). Chipster: user-friendly analysis software for microarray and other high-throughput data. BMC Genomics 12:507. doi: 10.1186/1471-2164-12-507
Karpinski, S., Reynolds, H., Karpinska, B., Wingsle, G., Creissen, G., and Mullineaux, P. (1999). Systemic signaling and acclimation in response to excess excitation energy in Arabidopsis. Science 284, 654–657. doi: 10.1126/science.284.5414.654
Kleine, T., Kindgren, P., Benedict, C., Hendrickson, L., and Strand, A. (2007). Genome-wide gene expression analysis reveals a critical role for CRYPTOCHROME1 in the response of arabidopsis to high irradiance. Plant Physiol. 144, 1391–1406. doi: 10.1104/pp.107.098293
Krasensky-Wrzaczek, J., and Kangasjarvi, J. (2018). The role of reactive oxygen species in the integration of temperature and light signals. J. Exp. Bot. 69, 3347–3358. doi: 10.1093/jxb/ery074
Laloi, C., and Havaux, M. (2015). Key players of singlet oxygen-induced cell death in plants. Front. Plant Sci. 6:39. doi: 10.3389/fpls.2015.00039
Legris, M., Klose, C., Burgie, E. S., Rojas, C. C., Neme, M., Hiltbrunner, A., et al. (2016). Phytochrome B integrates light and temperature signals in Arabidopsis. Science 354, 897–900. doi: 10.1126/science.aaf5656
Leister, D. (2019). Piecing the puzzle together: the central role of reactive oxygen species and redox hubs in chloroplast retrograde signaling. Antioxid. Redox Signal. 30, 1206–1219. doi: 10.1089/ars.2017.7392
Lim, S. D., Kim, S. H., Gilroy, S., Cushman, J. C., and Choi, W. G. (2019). Quantitative ROS bioreporters: a robust toolkit for studying biological roles of ROS in response to abiotic and biotic stresses. Physiol. Plant. 165, 356–368. doi: 10.1111/ppl.12866
Littlejohn, G. R., Breen, S., Smirnoff, N., and Grant, M. (2021). Chloroplast immunity illuminated. New Phytol. 229, 3088–3107. doi: 10.1111/nph.17076
Lv, F. F., Zhou, J., Zeng, L. Z., and Xing, D. (2015). beta-cyclocitral upregulates salicylic acid signalling to enhance excess light acclimation in Arabidopsis. J. Exp. Bot. 66, 4719–4732. doi: 10.1093/jxb/erv231
Massonnet, C., Vile, D., Fabre, J., Hannah, M. A., Caldana, C., Lisec, J., et al. (2010). Probing the reproducibility of leaf growth and molecular phenotypes: a comparison of three arabidopsis accessions cultivated in ten laboratories. Plant Physiol. 152, 2142–2157. doi: 10.1104/pp.109.148338
Merilo, E., Yarmolinsky, D., Jalakas, P., Parik, H., Tulva, I., Rasulov, B., et al. (2018). Stomatal VPD response: there is more to the story than ABA. Plant Physiol. 176, 851–864. doi: 10.1104/pp.17.00912
Michelet, L., and Krieger-Liszkay, A. (2012). Reactive oxygen intermediates produced by photosynthetic electron transport are enhanced in short-day grown plants. Biochim. Biophys. Acta 1817, 1306–1313. doi: 10.1016/j.bbabio.2011.11.014
Palma, K., Thorgrimsen, S., Malinovsky, F. G., Fiil, B. K., Nielsen, H. B., Brodersen, P., et al. (2010). Autoimmunity in Arabidopsis acd11 is mediated by epigenetic regulation of an immune receptor. PLoS Pathog. 6:e1001137. doi: 10.1371/journal.ppat.1001137
Phua, S. Y., De Smet, B., Remacle, C., Chan, K. X., and Van Breusegem, F. (2021). Reactive oxygen species and organellar signaling. J. Exp. Bot. 72, 5807–5824. doi: 10.1093/jxb/erab218
Putri, G. H., Anders, S., Pyl, P. T., Pimanda, J. E., and Zanini, F. (2022). Analysing high-throughput sequencing data in Python with HTSeq 2.0. Bioinformatics 38, 2943–2945. doi: 10.1093/bioinformatics/btac166
Ritchie, M. E., Phipson, B., Wu, D., Hu, Y. F., Law, C. W., Shi, W., et al. (2015). limma powers differential expression analyses for RNA-sequencing and microarray studies. Nucleic Acids Res. 43:e47. doi: 10.1093/nar/gkv007
Robinson, M. D., McCarthy, D. J., and Smyth, G. K. (2010). edgeR: a Bioconductor package for differential expression analysis of digital gene expression data. Bioinformatics 26, 139–140. doi: 10.1093/bioinformatics/btp616
Sakuma, Y., Maruyama, K., Qin, F., Osakabe, Y., Shinozaki, K., and Yamaguchi-Shinozaki, K. (2006). Dual function of an Arabidopsis transcription factor DREB2A in water-stress-responsive and heat-stress-responsive gene expression. Proc. Natl. Acad. Sci. U.S.A. 103, 18822–18827. doi: 10.1073/pnas.0605639103
Seyednasrollah, F., Laiho, A., and Elo, L. L. (2015). Comparison of software packages for detecting differential expression in RNA-seq studies. Brief. Bioinform. 16, 59–70. doi: 10.1093/bib/bbt086
Shao, N., Duan, G. Y., and Bock, R. (2013). A mediator of singlet oxygen responses in Chlamydomonas reinhardtii and Arabidopsis identified by a luciferase-based genetic screen in algal cells. Plant Cell 25, 4209–4226. doi: 10.1105/tpc.113.117390
Shapiguzov, A., Vainonen, J. P., Hunter, K., Tossavainen, H., Tiwari, A., Jarvi, S., et al. (2019). Arabidopsis RCD1 coordinates chloroplast and mitochondrial functions through interaction with ANAC transcription factors. Elife 8:e43284. doi: 10.7554/eLife.43284
Simkova, K., Moreau, F., Pawlak, P., Vriet, C., Baruah, A., Alexandre, C., et al. (2012). Integration of stress-related and reactive oxygen species-mediated signals by Topoisomerase VI in Arabidopsis thaliana. Proc. Natl. Acad. Sci. U.S.A. 109, 16360–16365. doi: 10.1073/pnas.1202041109
Tikkanen, M., and Aro, E. M. (2014). Integrative regulatory network of plant thylakoid energy transduction. Trends in Plant Sci. 19, 10–17. doi: 10.1016/j.tplants.2013.09.003
Tikkanen, M., Gollan, P. J., Mekala, N. R., Isojarvi, J., and Aro, E. M. (2014). Light-harvesting mutants show differential gene expression upon shift to high light as a consequence of photosynthetic redox and reactive oxygen species metabolism. Philos. Trans. R. Soc. B. Biol. Sci. 369:20130229. doi: 10.1098/rstb.2013.0229
Tiwari, S., Rai, R., and Agrawal, M. (2008). Annual and seasonal variations in tropospheric ozone concentrations around Varanasi. Int. J. Remote Sens. 29, 4499–4514. doi: 10.1080/01431160801961391
Vaahtera, L., Brosche, M., Wrzaczek, M., and Kangasjarvi, J. (2014). Specificity in ROS signaling and transcript signatures. Antioxid. Redox Signal. 21, 1422–1441. doi: 10.1089/ars.2013.5662
Vahisalu, T., Kollist, H., Wang, Y. F., Nishimura, N., Chan, W. Y., Valerio, G., et al. (2008). SLAC1 is required for plant guard cell S-type anion channel function in stomatal signalling. Nature 452, 487–U415. doi: 10.1038/nature06608
Vainonen, J. P., and Kangasjarvi, J. (2015). Plant signalling in acute ozone exposure. Plant Cell Environ. 38, 240–252. doi: 10.1111/pce.12273
Van Aken, O., Zhang, B. T., Law, S., Narsai, R., and Whelan, J. (2013). AtWRKY40 and AtWRKY63 modulate the expression of stress-responsive nuclear genes encoding mitochondrial and chloroplast proteins. Plant Physiol. 162, 254–271. doi: 10.1104/pp.113.215996
Wang, L., Tsuda, K., Truman, W., Sato, M., Nguyen, L. V., Katagiri, F., et al. (2011). CBP60g and SARD1 play partially redundant critical roles in salicylic acid signaling. Plant J. 67, 1029–1041. doi: 10.1111/j.1365-313X.2011.04655.x
Waszczak, C., Carmody, M., and Kangasjarvi, J. (2018). Reactive oxygen species in plant signaling. Ann. Rev. Plant Biol. 69, 209–236. doi: 10.1146/annurev-arplant-042817-040322
Wohlgemuth, H., Mittelstrass, K., Kschieschan, S., Bender, J., Weigel, H. J., Overmyer, K., et al. (2002). Activation of an oxidative burst is a general feature of sensitive plants exposed to the air pollutant ozone. Plant Cell Environ. 25, 717–726. doi: 10.1046/j.1365-3040.2002.00859.x
Wrzaczek, M., Brosche, M., Salojarvi, J., Kangasjarvi, S., Idanheimo, N., Mersmann, S., et al. (2010). Transcriptional regulation of the CRK/DUF26 group of receptor-like protein kinases by ozone and plant hormones in Arabidopsis. BMC Plant Biol. 10:95. doi: 10.1186/1471-2229-10-95
Xu, E. J., Vaahtera, L., and Brosche, M. (2015a). Roles of defense hormones in the regulation of ozone-induced changes in gene expression and cell death. Mol. Plant 8, 1776–1794. doi: 10.1016/j.molp.2015.08.008
Xu, E. J., Vaahtera, L., Horak, H., Hincha, D. K., Heyer, A. G., and Brosche, M. (2015b). Quantitative trait loci mapping and transcriptome analysis reveal candidate genes regulating the response to ozone in Arabidopsis thaliana. Plant Cell Environ. 38, 1418–1433. doi: 10.1111/pce.12499
Zabala, M. D. T., Littlejohn, G., Jayaraman, S., Studholme, D., Bailey, T., Lawson, T., et al. (2015). Chloroplasts play a central role in plant defence and are targeted by pathogen effectors. Nat. Plants 1:15074. doi: 10.1038/nplants.2015.74
Zandalinas, S. I., Fichman, Y., Devireddy, A. R., Sengupta, S., Azad, R. K., and Mittler, R. (2020). Systemic signaling during abiotic stress combination in plants. Proc. Natl. Acad. Sci. U.S.A. 117, 13810–13820. doi: 10.1073/pnas.2005077117
Zandalinas, S. I., Fritschi, F. B., and Mittler, R. (2019a). Signal transduction networks during stress combination. J. Exp. Bot. 71, 1734–1741. doi: 10.1093/jxb/erz486
Zandalinas, S. I., Fritschi, F. B., and Mittler, R. (2021a). Global warming, climate change, and environmental pollution: recipe for a multifactorial stress combination disaster. Trends Plant Sci. 26, 588–599. doi: 10.1016/j.tplants.2021.02.011
Zandalinas, S. I., Sengupta, S., Burks, D., Azad, R. K., and Mittler, R. (2019b). Identification and characterization of a core set of ROS wave-associated transcripts involved in the systemic acquired acclimation response of Arabidopsis to excess light. Plant J. 98, 126–141. doi: 10.1111/tpj.14205
Zandalinas, S. I., Sengupta, S., Fritschi, F. B., Azad, R. K., Nechushtai, R., and Mittler, R. (2021b). The impact of multifactorial stress combination on plant growth and survival. New Phytol. 230, 1034–1048. doi: 10.1111/nph.17232
Zhang, S. R., Apel, K., and Kim, C. H. (2014). Singlet oxygen-mediated and EXECUTER-dependent signalling and acclimation of Arabidopsis thaliana exposed to light stress. Philos. Trans. R. Soc. B. Biol. Sci. 369:20130227. doi: 10.1098/rstb.2013.0227
Keywords: ozone, cell death, signal interaction, high light, abscisic acid, Arabidopsis
Citation: Xu E, Tikkanen M, Seyednasrollah F, Kangasjärvi S and Brosché M (2022) Simultaneous Ozone and High Light Treatments Reveal an Important Role for the Chloroplast in Co-ordination of Defense Signaling. Front. Plant Sci. 13:883002. doi: 10.3389/fpls.2022.883002
Received: 24 February 2022; Accepted: 06 June 2022;
Published: 07 July 2022.
Edited by:
Vicent Arbona, Jaume I University, SpainReviewed by:
Thomas Roach, University of Innsbruck, AustriaPavel Kerchev, Mendel University in Brno, Czechia
Copyright © 2022 Xu, Tikkanen, Seyednasrollah, Kangasjärvi and Brosché. This is an open-access article distributed under the terms of the Creative Commons Attribution License (CC BY). The use, distribution or reproduction in other forums is permitted, provided the original author(s) and the copyright owner(s) are credited and that the original publication in this journal is cited, in accordance with accepted academic practice. No use, distribution or reproduction is permitted which does not comply with these terms.
*Correspondence: Mikael Brosché, bWlrYWVsLmJyb3NjaGVAaGVsc2lua2kuZmk=
†Present address: Enjun Xu, Institute of Botany, Chinese Academy of Sciences, Beijing, China