- 1Graduate School of Environmental and Life Science, Okayama University, Okayama, Japan
- 2Faculty of Agriculture, Okayama University, Okayama, Japan
- 3Faculty of Agriculture, Kyoto University, Kyoto, Japan
- 4Graduate School of Agriculture, Kyoto University, Kyoto, Japan
Rice is the most important crop species in the world, being staple food of more than 80% of people in Asia. About 80% of rice grain is composed of carbohydrates (starch), with its protein content as low as 7–8%. Therefore, increasing the protein content of rice offers way to create a stable protein source that contributes to improving malnutrition and health problems worldwide. We detected two rice lines harboring a significantly higher protein content (namely, HP5-7 and HP7-5) in the EG4 population. The EG4 strain of rice is a unique material in that the transposon mPing has high transpositional activity and high copy numbers under natural conditions. Other research indicated that mPing is abundant in the gene-rich euchromatic regions, suggesting that mPing amplification should create new allelic variants, novel regulatory networks, and phenotypic changes in the EG4 population. Here, we aimed to identify the candidate genes and/or mPing insertion sites causing high protein content by comprehensively identifying the mPing insertion sites and carrying out an RNA-seq-based transcriptome analysis. By utilizing the next-generation sequencing (NGS)-based methods, ca. 570 mPing insertion sites were identified per line in the EG4 population. Our results also indicated that mPing apparently has a preference for inserting itself in the region near a gene, with 38 genes in total found to contain the mPing insertion in the HP lines, of which 21 and 17 genes were specific to HP5-7 and HP7-5, respectively. Transcriptome analysis revealed that most of the genes related to protein synthesis (encoding glutelin, prolamin, and globulin) were up-regulated in HP lines relative to the control line. Interestingly, the differentially expressed gene (DEG) analysis revealed that the expression levels of many genes related to photosynthesis decreased in both HP lines; this suggests the amount of starch may have decreased, indirectly contributing to the increased protein content. The high-protein lines studied here are expected to contribute to the development of high protein-content rice by introducing valuable phenotypic traits such as high and stable yield, disease resistance, and abundant nutrients.
Introduction
The United Nations expects the world population to reach 9.6 billion by 2050. The current world population is 7.3 billion and its demand for protein is 202 million tons, but this is predicted to increase to 267–286 million tons in 2050 (Henchion et al., 2017). Protein is a polymer of amino acids and an indispensable component of body tissues, enzymes, hormones, etc., as well as a substantial source of essential nutrients and energy. Rice is cultivated worldwide, and 60% of the world’s population depends upon it as a staple food, with more than 80% of people in Asia eating it (Kawakatsu et al., 2008). Rice also supplies 21% of the world’s caloric intake; ca. 80% of rice consists of carbohydrates (starch), which is of great value as a staple food, yet its protein content can be as low as 7–8% (Kubota, 2016). Therefore, increasing the protein content of rice would create a stable protein source that can help to overcome malnutrition and health problems not only in Japan but also globally (Chen et al., 2018).
The endosperm of rice is composed of 70–80% starch, 7–10% protein, and 1% lipid (Martin and Fitzgerald, 2002). Rice has four types of seed storage proteins (SSP): albumin, glutelin, prolamin, and globulin. Glutelin is encoded by 15 genes and constitutes 60–80% of the total protein content and is classified into four subfamilies: GluA, GluB, GluC, and GluD (Kawakatsu et al., 2008). Prolamin, encoded by a multigene family of 34 gene copies, makes up 20–30% of total protein and may be categorized as 10 kDa prolamin (RP10), 13 kDa prolamin (RM1, RM2, RM4, and RM9), and 16 kDa prolamin (RP16) according to its molecular weight (Yamagata et al., 1982; Kawakatsu et al., 2008). Globulin is a protein representing 8–10% of total protein; it occurs as two types of polypeptides, 23–27 kDa and 16 kDa, which are structurally homologous to wheat grain glutenin called α-globulin (Ellepola et al., 2006; Kawakatsu et al., 2008). Both albumin and globulin are concentrated in the bran but polishing during the milling process removes a major portion of these proteins (Shewry, 2007). In recent years, enhancing the seed storage proteins to improve rice’s nutritive value has emerged as a key target in rice quality breeding (Jiang et al., 2014). Both the content and composition of protein is crucial to quality of rice grain and its nutritional value (Lin et al., 2005; Chen et al., 2017).
Transposable elements (TEs) are mobile genetic elements in the eukaryotic genome, now recognized as an important source of genome evolution and diversification. TEs are a major component of higher plant genomes, accounting for 35% of the rice genome (Turucotte et al., 2001). TEs may alter the expression of neighboring genes via insertion into promoter regions, or disrupt the function of protein-coding genes when inserted into the genes, or even change gene structure by altering its splicing and polyadenylation patterns (Kumar and Bennetzen, 1999; Feschotte et al., 2002; Wessler, 2006; Feschotte and Pritham, 2007; Feschotte, 2008; Butelli et al., 2012). TEs are divided into two classes according to whether their transposition involves either RNA intermediates (Class I) or DNA intermediates (Class II; Kumar and Bennetzen, 1999; Feschotte et al., 2002; Wessler, 2006; Feschotte, 2008). Class I elements are transposed by a “copy and paste” mechanism, which involves the reverse transcription of RNA and the integration of a cDNA fragment. Class II elements are excised and integrated into new genomic locations by a ‘cut and paste’ mechanism. In this respect, Miniature Inverted-repeat Transposable Elements (MITEs) are non-autonomous Class II DNA transposons of small size (< 600 bp) that harbor short terminal inverted repeats (TIRs), capable of attaining high copy numbers in eukaryotic genomes (Feschotte et al., 2002; Feschotte and Pritham, 2007). MITEs have been classified into two superfamilies based on the similarity of their TIRs and their target site duplication (TSD): Tourist-like MITEs and Stowaway-like MITEs (Feschotte et al., 2002; Feschotte and Pritham, 2007).
mPing is the first active MITE through animal and plant genomes, which was discovered independently by three different assays: long-term rice cell culture (Jiang et al., 2003), short-term anther culture (Kikuchi et al., 2003), and plants derived from gamma-irradiated seeds of the rice cultivar (Nakazaki et al., 2013). The mPing element is short (430 bp) with 15 bp TIRs and belongs to the Tourist family (Jiang et al., 2003). Because mPing has no inherent capacity for transposition, the transposase is provided by two related autonomous elements, Ping and Pong. The autonomous Ping and Pong elements are members of the PIF/Harbinger superfamily that is widespread in both plants and animals (Hancock et al., 2010). Like most members of that superfamily, Ping and Pong have two open reading frames (ORFs: ORF1 and ORF2), both of which are required for mPing transposition from one place to another on the genome (Yang et al., 2007; Hancock et al., 2010). The ORF1 protein contains a conserved Myb-like domain whose involvement in DNA binding was hypothesized (Yang et al., 2007; Hancock et al., 2010). The ORF2 encodes the transposase, which contains a putative Asp-Asp-Glu (DDE) motif that is a signature for transposase catalytic centers (Yang et al., 2007; Hancock et al., 2010).
Although mPing is clearly an active MITE, its copy numbers are relatively low, with less than 10 copies found in the subspecies indica and ~ 50 copies in the subspecies japonica, including the sequenced rice genome (Nipponbare; Naito et al., 2006). Another study revealed that mPing had amplified to over 1,000 copies in a few japonica rice strains (EG4, HEG4, and related landraces), and is still actively transposing and increasing its copy number, by about 20 copies per plant per generation, without radiation (Naito et al., 2006). A comprehensive survey of mPing insertion sites in EG4 strain revealed that mPing is enriched in euchromatic, gene-rich regions but rarely present in heterochromatic regions (Naito et al., 2009). Considering both the high activity and insertion preference of mPing in the EG4 strain, it is reasonable to envision that mPing amplification could create new allelic variants and novel regulatory networks, which may generate plants with more phenotypic diversity and/or novel phenotypic traits.
In this study, we investigated the protein content of the EG4 strain known to exhibit high mPing activity, and then used next-generation sequencing (NGS) to comprehensively analyze mPing insertion sites in the selected EG4 strains with high protein content. Furthermore, an RNA-seq analysis was performed to quantify the expression levels of genes related to protein synthesis. Using the above information, we sought to identify the mPing insertion sites and related genes responsible for high protein content of rice.
Materials and methods
Plant materials
A total of 396 lines of the EG4 population were grown in the experimental paddy field of Kyoto University, Japan. Genomic DNA was extracted from all plants by using the DNeasy Plant Mini Kit following the manufacturer’s instructions (QIAGEN, Hilden, Germany). The total RNA was extracted from immature seeds on the 7th day after flowering with three biological replicates per line using the RNeasy Plant Mini Kit according to its manufacturer’s instructions (QIAGEN, Hilden, Germany). The extracted RNA was digested with DNase (TAKARA, Shiga, Japan) to remove the remaining gDNA. The yield and quality of the extracted DNA and RNA were confirmed using a NanoDrop 2000 instrument (Thermo Fisher Scientific, Wilmington, DE, United States).
Measurement of protein content
In this experiment, brown rice was used to quantify the protein content of rice seeds. For practical purposes, it is often desirable to use seeds post-milling to qualify their protein content. However, in this study, brown rice was instead used for two main reasons. First, it is difficult to obtain the minimum amount of brown rice required for the rice milling process. Second, an early study showed a 10% reduction in protein content after milling brown rice, but a strong positive correlation was nonetheless detected between the protein content of brown rice and white rice (Higashi et al., 1974). Hence, it was considered sufficient to quantify the protein content in seeds using brown rice.
In 2013, 396 lines of the EG4 population were cultivated for the primary screening of their protein content. To do this, four seeds per panicle from each line were crushed, and the protein content of their rice powder was measured once by the bicinchoninic acid (BCA) assay method. Based on the measurement results, 25 lines with diverse protein content values were selected and subjected to secondary screening in which total protein content was measured from brown rice by applying the improved Dumas method (Jung et al., 2003). This method burns and reduces the sample at a high temperature, and then measures the amount of nitrogen in the generated nitrogen gas (Jung et al., 2003). This method is quick, requiring just a few minutes to analyze each sample, without the use of any deleterious reagents. Protein content was measured in 700–800 mg of brown rice, three times per line, with SUMIGRAPH® (NC-TRINITY, Sumika Chemical Analysis Service, Ltd, Tokyo, Japan).
Preparation of the amplicon sequencing library for mPing insertion sites
To determine the mPing insertion sites in a comprehensive manner, flanking regions of mPing insertion sites were amplified by PCR, and the ensuing products were sequenced on an Illumina platform. An amplicon sequencing library was constructed according to previously described methods (Monden et al., 2014, 2015). First, genomic DNA was fragmented using gTUBE (~ 6 kb; Covaris Inc., MA, United States), and forked adaptors were ligated to the fragmented DNA. These forked adaptors were prepared by annealing two different oligos (Forked_Type1 and Forked_Com; Supplementary Table 1). Primary PCR amplification was performed with mPing-specific (mPing_1st) and adaptor-specific (AP2-Type1) primer combination, which used the adaptor-ligated DNA as the template (Supplementary Table 1). Nested PCR amplification was carried out using the tailed PCR primers (D501-D503 and D701-D712) with primary PCR products serving as the template. The tailed PCR primers contain the P5 or P7 sequence (Illumina) for hybridization on the sequencing flow cell, and several barcodes for multiplex sequencing. Thus, mPing-specific primers (i.e., D501–D503) consisted of a P5 sequence, a barcode sequence, and the mPing end sequence, while the adapter-specific primers (i.e., D701–D712) consisted of a P7 sequence, a barcode sequence, and an adapter sequence. The primer combinations of each sample can be found in Supplementary Table 2. The ensuing PCR products were size-selected (400–600 bp) on agarose gels and purified with a QIAquick Gel Extraction Kit (QIAGEN, Hilden, Germany). Purified products were then quantified using a Qubit fluorometer (Invitrogen, Carlsbad, CA, United States) and the size selection range was confirmed in an Agilent 2,200 TapeStation system (Agilent, Santa Clara, CA, United States). The MiSeq sequencing library was prepared by pooling equal amounts of purified barcoded products from each line.
Data analysis
The resulting paired-end reads (150 bp) were analyzed in two ways. The first method followed procedures described in our previous studies (Monden et al., 2014, 2015; Sasai et al., 2019; Hirata et al., 2020), which can detect genome-wide insertion sites of a known TE without any requirement for whole genome sequence information (Monden et al., 2014). The obtained reads were analyzed using Maser, a pipeline execution system of the Cell Innovation Program at the National Institute of Genetics2. Adaptor trimming and quality filtering (QV ≥ 30) were performed using cutadapt (Martin, 2011). Filtered reads were trimmed to a specific length that covered most of the sequences. Those reads with ≥10 identical sequences were reduced to a single sequence, in FASTA format, and then clustered using the BLAT self-alignment program (Kent, 2002) under these parameter settings: “-tileSize” = 8, “-minMatch” = 1, “-minScore” = 10, “-repMatch” = −1, and “-oneOff” = 2. This clustering analysis produced many clusters, each corresponding to a separate mPing insertion site. An optimal threshold was then set to evaluate the presence or absence of mPing insertions: if the number of reads on a given cluster at a specific insertion site comprise < 0.01% of the entire reads for that line, then mPing was considered absent from that site. This yielded genotyping information for the presence (1) versus absence (0) of mPing insertions in every line.
A second, different analytical method was adopted because the rice reference genome sequence was the first to be deciphered among staple crop species (Eckardt, 2000; Jackson, 2016). Given its subsequent improvements, a highly accurate reference genome sequence of rice is now available (Kawahara et al., 2013; Sakai et al., 2013). The 150-bp paired-end reads were mapped onto the Nipponbare reference genome sequence,1 to identify the mPing insertion sites. Galaxy/NAAC (Advanced Analysis Center, NARO) was used for the follow-up data analysis. First, low quality reads were removed with Trimmomatic (Bolger et al., 2014). After removing low quality reads, high-quality reads were aligned to the Nipponbare reference genome sequence using BWA (Galaxy Version 1.2.3) software (Li and Durbin, 2009). The number of reads within 500 bp from the start site of each aligned read was counted, using an in-house Perl script, and each site with 50 or more aligned reads were designated a mPing insertion site. The MiSeq reads for analyzing the mPing insertion sites were deposited under the accession number DDBJ: DRA014320.
Experimental validation of mPing insertion sites
To verify the existence of mPing insertions identified by the above data analysis, PCR primers were designed based on the genomic sequence flanking a mPing insertion site (Monden et al., 2009; Supplementary Table 3). Each PCR was run in 10-μl reaction volume that contained 5 μl of 2 × Gflex PCR buffer (Mg2+, dNTP plus), 0.2 μl of Tks Gflex DNA Polymerase, 1 μl of 10 μM primer (forward and reverse) and 1 μl of genomic DNA (l00 ng/μl). The cycling conditions were as follows: 94°C for 2 min; 35 cycles of 98°C for 10 s, 60°C for 30 s, and 68°C for 30 s; then 68°C for 3 min. Amplified products were visualized using electrophoresis on a 1.5% agarose gel.
RNA-sequencing
The RNA-sequencing (RNA-seq) library was sequenced using the NovaSeq system (Illumina, San Diego, CA, United States). The paired-end short reads with a read length of 150 bp were analyzed as follows. Adaptor trimming and quality filtering (QV ≥ 30) were performed using Trim Galore,2 after which the remaining clean reads were aligned to the Nipponbare reference genome sequence, by using HISAT2 (Kim et al., 2019), and their expression levels were calculated by StringTie (Pertea et al., 2015, 2016). To determine the differentially expressed genes (DEGs), the obtained expression levels were normalized and log-transformed using DESeq2 (Love et al., 2014). Principal component analysis (PCA) was implemented using the “prcomp” function. A biplot graph was visualized with “ggfortify” package in R (Tang et al., 2016). A heatmap was generated by “clustermap” in the seaborn statistical data visualization library in Python (Waskom, 2021). Gene ontology (GO) enrichment analysis was carried out using ShinyGO (Ge et al., 2020). All RNA-seq reads were deposited under the accession number DDBJ: DRA014328.
Quantitative real-time PCR
The cDNA was synthesized from the total RNA, using the ReverTra Ace® qPCR RT Master Mix (TOYOBO, Osaka, Japan). Next, the qPCR was performed in a Roche LightCycler® 480II system with KOD SYBR® qPCR Mix (TOYOBO, Osaka, Japan). The reaction solution contained 5.0 μl of qPCR Mix, 4.0 μl of cDNA and 1 μl of each primer set (10 μM per forward and reverse). The cycling conditions were as follows: 98°C for 2 min, then 45 cycles of 98°C for 10 s, 60°C for 10 s, and 68°C for 30 s. The expression levels of genes were normalized to the level of constitutive Actin expression. All primers used in the qPCR are listed in Supplementary Table 4.
Results
Evaluation of protein content in the EG4 rice population
In the primary screening step, protein content was measured using the BCA method in 396 lines of the EG4 population. Based on these results, 25 lines with diverse protein content were selected. Secondary screening was performed using the improved Dumas method, and protein content was analyzed in detail. The protein content of the randomly selected EG4 lines was 6.56 to 6.99%, while that of two lines was higher, at 7.91 ± 0.17% and 7.81 ± 0.06%, respectively (Figure 1). Because these two lines had a significantly higher protein content than randomly selected EG4 lines, both (hereon HP5-7 and HP7-5) were selected for use as high-protein lines. In addition, one line (C3-1) featuring normal protein content (6.56 ± 0.08%) was chosen as a control for the subsequent analyses (Figure 1).
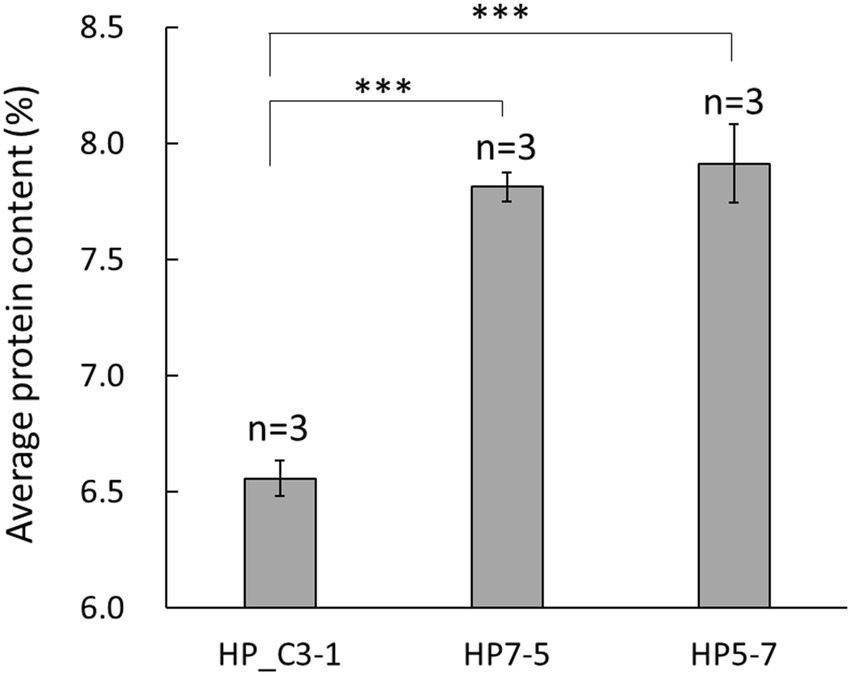
Figure 1. Average protein content in the selected EG4 lines. Protein content was evaluated using three independent plants per line. Data shown are the mean ± SD of three replicates (n = 3). A statistically significant difference between the mean values was inferred from Student’s t-test (***p < 0.001).
Sequencing and data analysis of mPing insertion sites
A total of 16,233,064 paired-end reads of 150 bp were obtained by MiSeq sequencing (min: 533,443, average: 649,323, max: 839,733 reads per line; Supplementary Table 5). After preprocessing, 2,995,488 reads remained overall (Supplementary Table 6). These reads were used for the clustering analysis with the BLAT self-alignment program (Kent, 2002), which suggested 3,268 independent insertion sites in 25 lines (Supplementary Table 6). Next, we determined the genotype (presence or absence) per insertion site and calculated the total number of insertion sites for each line. For the 25 lines, their number of mPing insertion sites averaged 573.1, ranging from 495.0 to 655.0 (Supplementary Table 7). The HP5-7 and HP7-5 (high-protein lines) had 589 and 495 mPing insertion sites, respectively, while the C3-1 line (control) had 581. These three lines were focused on subsequent analyses.
Given that a highly accurate reference genome sequence is available for rice, we also identified the mPing insertion sites in another way, by aligning the MiSeq reads to the Nipponbare reference genome sequence. This yielded 653, 538, and 617 mPing insertion sites identified in HP5-7, HP7-5, and C3-1, respectively (Table 1). Comparing the mPing insertion sites identified by the clustering-based versus the alignment-based methods in the three lines, 572 (97.1%), 478 (96.6%), and 552 (95.0%) sites were detected in common for HP5-7, HP7-5, and C3-1, respectively (Table 1). Hence, most of the insertion sites (≥ 95%) can be detected by both analytical methods: one which identified insertion sites by clustering the reads based on the sequence similarity, without reliance on the reference genome sequence, and the other doing so by aligning the reads to the rice reference genome sequence. Further, despite having aligned reads < 50 (see “Materials and methods”)—the threshold for determining the presence of absence of insertion—there were some insertion sites where those alignment reads were confirmed. Because these insertion sites were identified by the clustering-based analysis, we considered them actually present. After including these insertion sites, the total number of mPing insertion sites per line was estimated to be 580, 485, and 570 in HP5-7, HP7-5, and C3-1, respectively (Table 1).
To verify the mPing insertion sites detected above, an experimental validation was performed using several selected insertion sites. For this, PCR primers were designed based on the flanking sequences of mPing insertion sites according to our previously described methodology (Monden et al., 2009). For all insertion sites, the PCR bands of expected size were detected (Supplementary Figure 1). Therefore, the analytical methods in this study were considered highly reliable.
Comparing the mPing insertion sites identified in HP5-7, HP7-5, and C3-1, 367 of them (> 60%) were common to these three lines (Figure 2). By contrast, 141, 93, and 132 mPing insertion sites were only detected in HP5-7, HP7-5, and C3-1, respectively (Figure 2), which indicated they occurred specifically in each line. These line-specific insertion sites may have arisen very recently.
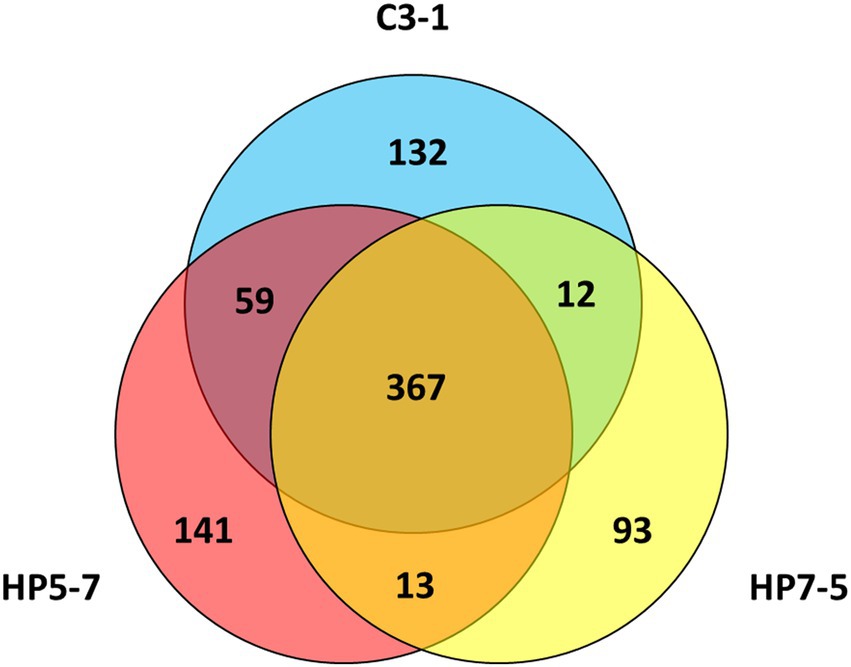
Figure 2. Venn diagram showing the number of mPing insertion sites and their overlap among the three lines.
To gain insight into the effects of mPing insertions upon genes, we investigated whether mPing insertion sites were located inside genes, near genes (within 3 kb from genes), or in intergenic regions. This revealed 14.0–14.6% of the mPing insertions positioned inside genes, 52.8–55.7% of them near genes, and 30.3–32.8% of them situated in intergenic regions (Table 2, Supplementary Table 8). The proportion of inside genes, near genes, and intergenic regions in the rice reference genome was 27.5, 31.0, and 41.5%, respectively (Supplementary Table 9); hence, the frequency of mPing insertion is significantly lower inside genes and intergenic regions and higher near genes. In addition, we investigated detailed information on mPing insertion sites inside or near genes in the rice genome (Supplementary Table 10). Of the mPing insertions located near genes, more mPing insertions were detected upstream of the gene as compared to downstream of the gene. Of the mPing insertions located inside genes, mPing is enriched in introns and 3’ UTR regions. It is possible the mPing insertion specific to the HP lines (HP5-7 and HP7-5) might affect their phenotype to increase protein content, so we investigated whether HP line-specific mPing insertions occurred inside or near genes. Of the HP5-7 specific insertion sites, 21 insertions were inside a gene and 80 insertions were near a gene (Supplementary Table 11); of the HP7-5 specific insertion sites, 17 insertions were inside a gene and 43 insertions were near a gene (Supplementary Table 12). Therefore, a total of 38 genes were detected that contained an mPing insertion unique to HP lines, and we posited they may have lost their function due to mPing insertion.
Transcriptome analysis
To investigate how mPing insertion affected gene expression, and how the latter is to protein content, RNA-seq-based transcriptome analysis was carried out using immature seeds 7 days post-pollination. In the PCA of expression levels of 37,871 genes, the HP5-7, HP7-5, and C3-1 samples clustered separately in the PCA biplot, while their three replicates per line were close to one another (Figure 3). This indicated the expression patterns of plants belonging to the same line were similar, but differed for plants among the lines. Interestingly, HP5-7 and HP7-5 were distributed in the positive direction of the first principal component (PC1) whereas C3-1 was distributed in the negative direction of PC1 (Figure 3). Accordingly, PC1 was inferred as the axis that reflected protein content of rice. Along PC2, however, HP7-5 and HP5-7 were distributed opposite directions (Figure 3); hence, PC2 was designated an axis that explaining features other than protein content. As expected, principal component scores of seed storage protein genes, when plotted, were strongly biased in the positive direction of PC1 (Figure 3). Therefore, we investigated the expression levels of the genes related to protein synthesis in HP5-7, HP7-5, and C3-1 based on the RNA-seq data.
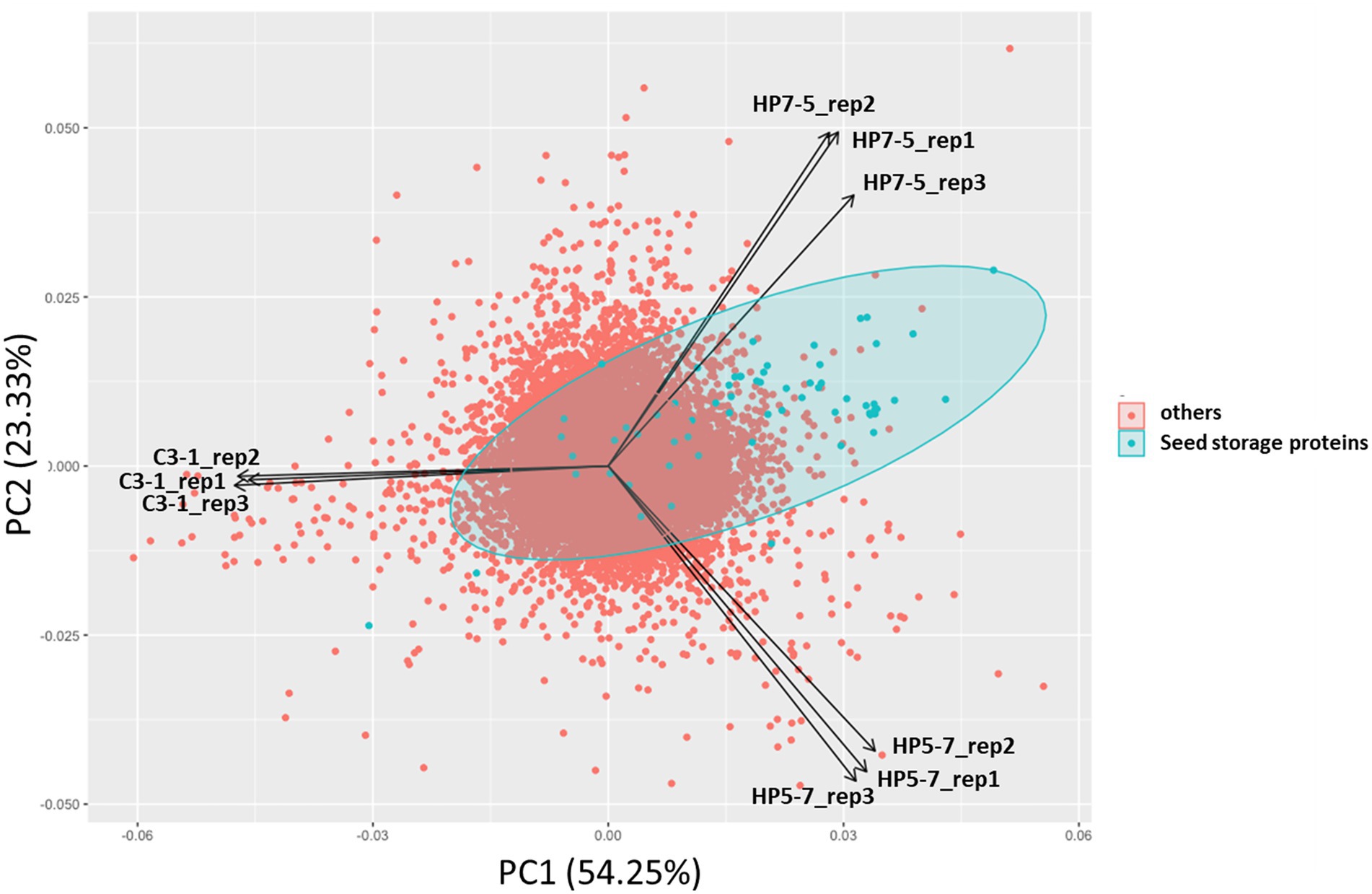
Figure 3. Results of principal component analysis (PCA) based on the expression levels of 37,871 genes. Principal component scores of seed storage protein genes are plotted as green dots.
The RNA-seq results showed that most of the genes encoding prolamin, glutelin, and globulin were clearly up-regulated in HP5-7 and HP7-5 compared to C3-1 (Figure 4; Supplementary Figure 2). To confirm those results, three genes, respectively, encoding prolamin, glutelin and globulin were randomly selected and subjected to qRT-PCR. Significant differences in expression were confirmed for all genes, and all validated genes had expression patterns similar to those of the RNA-seq data (Figure 5).
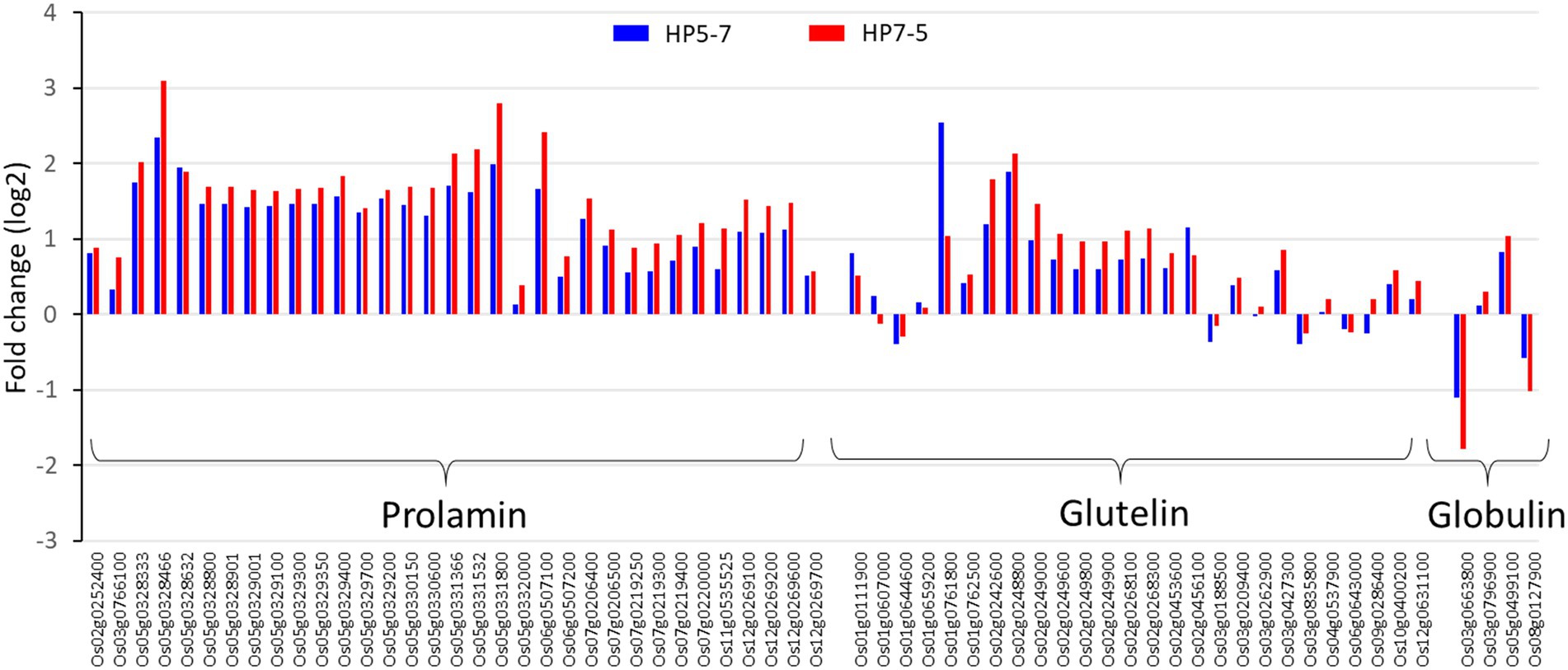
Figure 4. Expression levels of the genes encoding glutelin, prolamin, and globulin, based on the RNA-seq results. The log2[fold-change] value was calculated by comparison with the expression levels of C3-1 (the control).
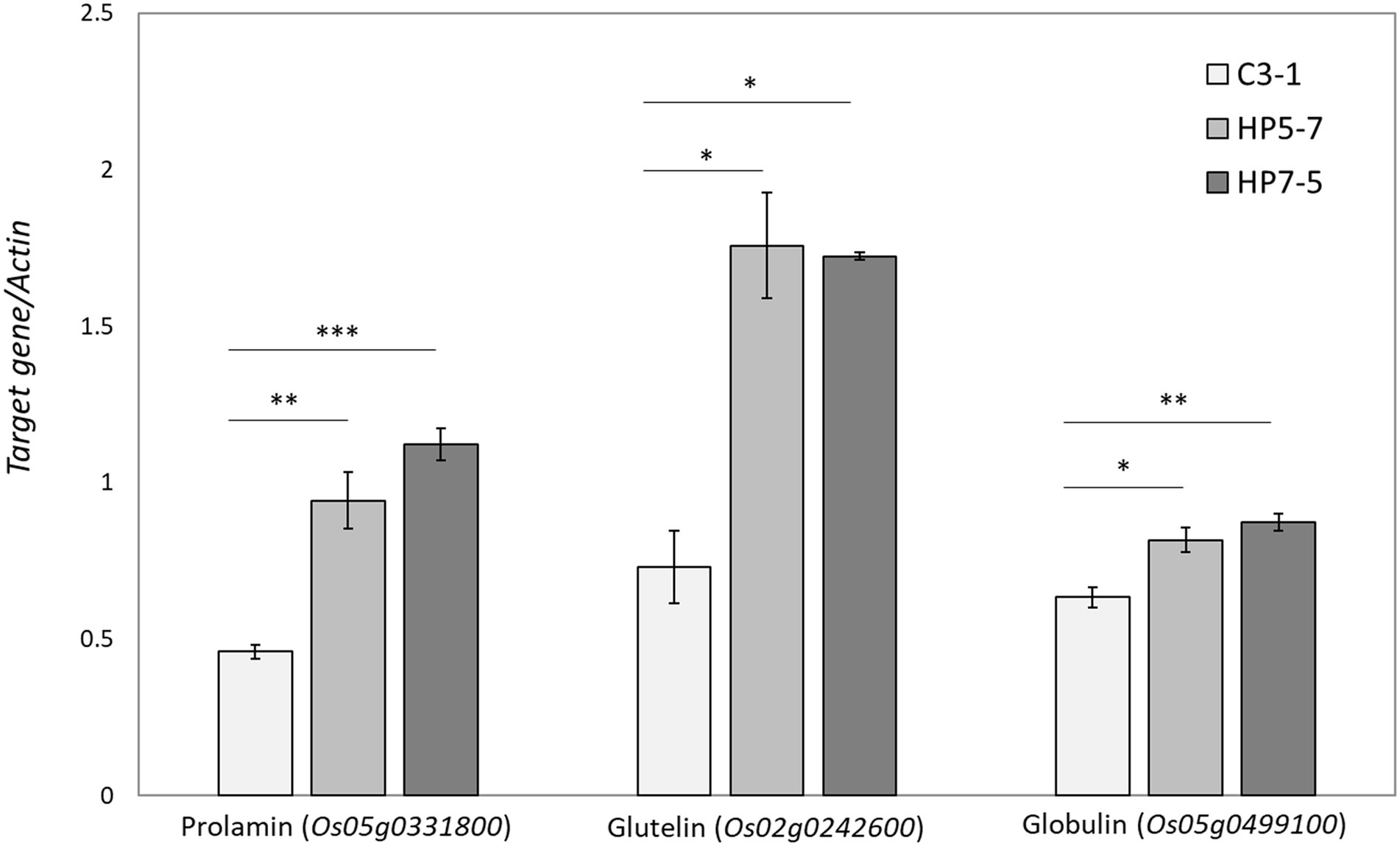
Figure 5. Validation of expression patterns of the selected three genes by qRT-PCR. The expression level of each gene was calculated relative to that of the Actin gene that served as an internal standard. Data shown are the mean ± SD of three replicates. A statistically significant difference between the mean values was inferred from Student’s t-test (*p < 0.05, **p < 0.01, and ***p < 0.001).
Using eXpress and edgeR software tools, differential expression analysis for HP5-7 versus C3-1 revealed 568 up-regulated and 1,910 down-regulated DEGs in HP5-7 (Supplementary Figure 3). Likewise, there were 550 up-regulated and 2,043 down-regulated DEGs in HP7-5 versus C3-1 (Supplementary Figure 3). These results revealed that approximately 80% of DEGs were down-regulated in the HP lines when compared to C3-1. Hierarchical clustering and heatmap expression analyses were then performed using the 1,278 DEGs commonly detected in the HP lines (Supplementary Figure 4). Evidently, expression patterns of these DEGs clearly differed between the C3-1 and both HP lines, whereas those of HP5-7 and HP7-5 were much more similar (Supplementary Figure 4). Compared with C3-1, there were 106 DEGs whose expression was significantly increased in the HP lines (orange cluster in Supplementary Figure 4) and 128 DEGs whose expression was significantly decreased in the HP lines (green cluster in Supplementary Figure 4). We performed a GO enrichment analysis, using ShinyGO, to functionally annotate these DEGs. The top-enriched GO terms for the up-regulated genes in HP lines were “UTP metabolic process,” “UTP biosynthetic process,” “GTP metabolic process,” “GTP biosynthetic process,” and “Guanosine-containing compound biosynthetic process” (Supplementary Figure 5). On the other hand, the top-enriched GO terms for the down-regulated genes in HP lines were “Photosynthesis, light harvesting in photosystem I,” “Regulation of photosynthesis, dark reduction,” “Regulation of reductive pentose-phosphate cycle,” “Negative regulation of reductive pentose-phosphate cycle,” and “Photosynthesis, light harvesting” (Figure 6). This indicated a tendency for decreased expression levels of photosynthesis-related genes in the HP lines, suggesting that the amount of starch, a major product of photosynthesis, might be reduced in the HP lines.
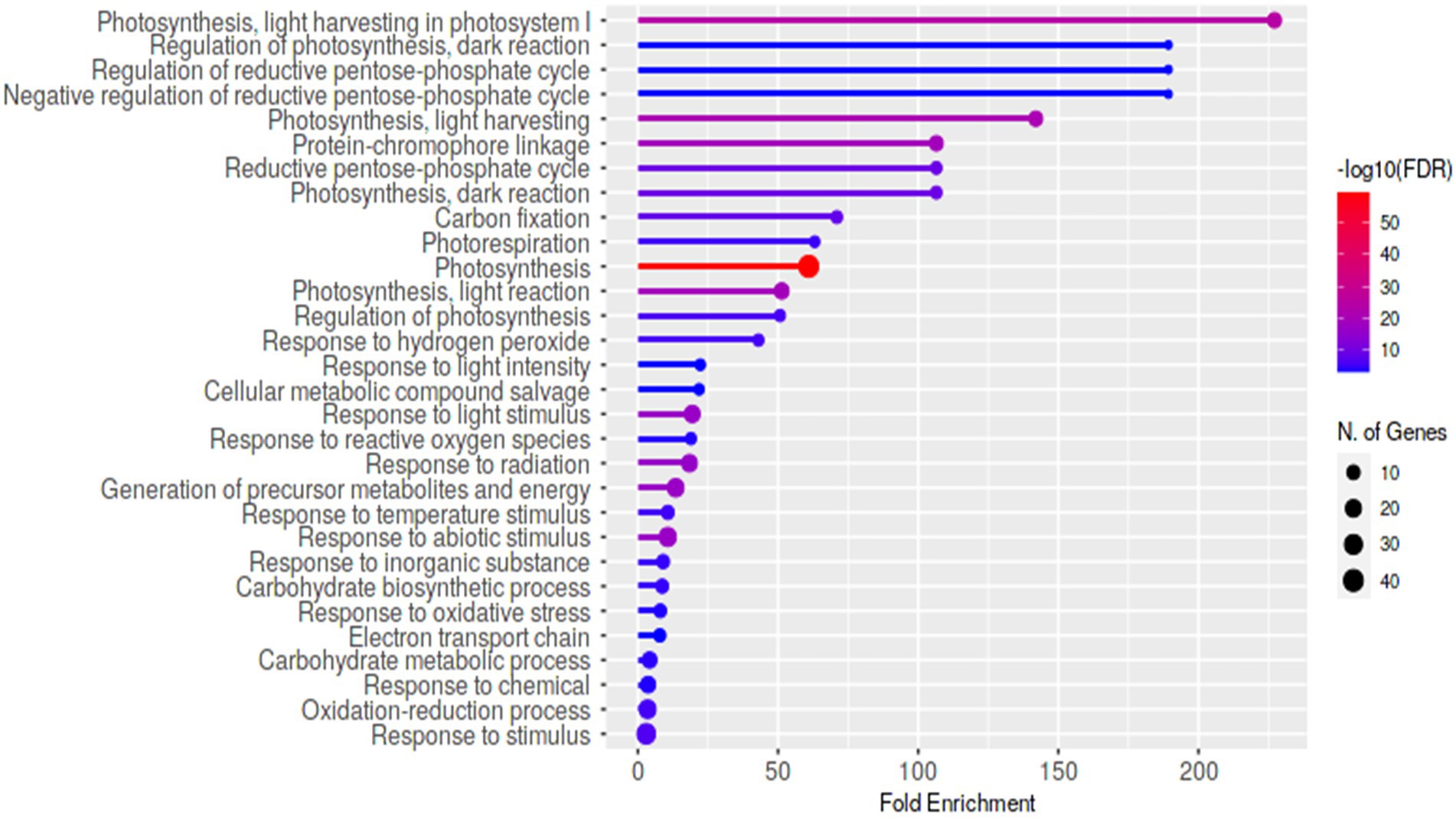
Figure 6. The enriched Gene Ontology (GO) terms of the identified 1,278 DEGs commonly detected in the HP lines. The top-ranked GO terms for the down-regulated DEGs in HP lines compared with C3-1.
Differentially expressed genes and mPing insertion sites
Finally, to investigate the relationships between gene expression levels of DEGs and mPing insertion sites in the HP lines, we extracted those DEGs containing mPing insertions or near them (within 3 kb of a gene). Of the DEGs in HP5-7 and HP7-5, an mPing insertion was detected near 12 and 10 DEGs, respectively, and one of these DEGs (Os3g0758551) was common to both lines (Supplementary Table 13). In addition, for two DEGs (Os08g0233900 and Os08g0260400), the mPing insertion inside the gene were detected in HP7-5 (Supplementary Table 13). Against the number of mPing insertions near or inside genes (404 and 326 mPing insertions in HP5-7 and HP7-5, respectively; Table 2), only 23 DEGs have mPing insertions near or inside them. Those results indicated that most of the mPing insertions did not affect the expression levels of neighboring genes. Intriguingly, the expression levels of most DEGs with or near an mPing insertion were down-regulated, with only two DEGs (Os10g0532150 and Os04g0415100) near an mPing insertion found up-regulated (Supplementary Table 13). This suggested mPing insertions are more likely to reduce the expression levels of neighboring genes when affecting gene expression. The gene annotations of these DEGs were examined carefully; unfortunately, among them no candidate gene governing the high protein content of HP lines was found.
Discussion
In this study, two high protein lines (i.e., HP5-7 and HP7-5) were screened from EG4 rice population, and candidate genes and/or mPing insertion sites related to high protein content were explored by genome-wide mPing insertion sites and an RNA-seq based transcriptome analysis. mPing has high transpositional activity and high copy numbers in EG4 and related rice strains under natural conditions (Naito et al., 2009). Considering that mPing tends to be inserted into gene-rich euchromatic regions, it is reasonable to suppose that altered gene expression and/or a gene knockout via mPing insertion and associated phenotypic changes are likely to occur in the EG4 population. In screening for high-protein content lines from that population based on two independent approaches (primary screening by the BCA assay and secondary screening by the improved Dumas method), we detected two lines (HP5-7 and HP7-5) characterized by high protein content. Utilizing an NGS-based method, a genome-wide analysis of mPing insertion sites in EG4 population was completed. These results uncovered approximately 570 mPing insertion sites per line for the 25 EG4 lines having variable protein content. The transcriptome results revealed that most of the genes related to storage protein content—encoding glutelin, prolamin, and globulin—were up-regulated in HP lines compared to the control line. We found a total of 38 genes containing an mPing insertion that were restricted to the HP lines, consisting of 21 and 17 genes specific to HP5-7 and HP7-5, respectively (Supplementary Tables 11, 12). Focusing on the DEGs, a total of 23 DEGs were detected to have mPing insertions inside or near them (Supplementary Table 13). These genes and/or DEGs may be responsible for causing the high protein content in the two selected HP lines. Further research is needed to find genes and/or mutations governing that high protein content.
Rice is a typical self-fertilizing crop, and its genome has been fixed over generations. Generally, the phenotype of these self-fertilizing plants has rarely changed during cultivation, resulting in a uniform population. TEs are considered a pivotal factor for inducing somatic mutations, nucleotide changes, and phenotypic variation in self-fertilizing plants. In the EG4 population, mPing is known to be actively transposing and capable of producing approximately 20 new copies per generation without any particular stress (Naito et al., 2006), which should lead to the generation of new allelic mutations and regulatory networks. This study comprehensively investigated the mPing insertion sites of several lines from the EG4 population by using the NGS platform. From the rice reference genome sequence, we categorized three regions: inside genes, near genes (within 3 kb from a gene) and intergenic regions, whose corresponding proportions of mPing insertion sites in the 12 rice chromosomes were 27.5, 31.0, and 41.5%, respectively (Supplementary Table 9). Yet 14.0–14.6%, 52.8–55.7% and 30.3–32.8% of mPing insertion sites were, respectively, located inside genes, near genes, and intergenic regions (Table 2; Supplementary Table 8). Our results suggest mPing is more apt to insert itself into the region near a gene. These results are consistent with other studies finding mPing enriched in euchromatic, gene-rich regions yet infrequent in heterochromatic regions (Naito et al., 2009, 2014).
Previous studies have analyzed mPing insertion sites by a variety of methods. Before the advent of NGS, the copy number of mPing was estimated using an experimental method called transposon display (Naito et al., 2006; Takagi et al., 2007). Transposon display, a modified method of amplified fragment length polymorphism (AFLP), has been used to generate and display hundreds of genomic fragments that flank specific transposable elements (Casa et al., 2000). To know the sequence information of a flanking region for the insertion site, the amplified products of interest were excised and purified from polyacrylamide gels. After cloning the purified products, these were individually sequenced piecemeal, using the Sanger method (Naito et al., 2006). But the advent of NGS technology now makes it possible to analyze the mPing insertion sites comprehensively at once. Naito et al. (2009) was the first to report on the identification of genome-wide mPing insertion sites using high-throughput sequencing technology in EG4 rice and related strains. That paper identified mPing insertion sites by amplifying the flanking DNA fragments of mPing insertion sites by applying vectorette PCR (Arnold and Hodgson, 1991) and pyrosequencing in the Roche 454 platform. Later, Chen et al. (2019) also characterized mPing insertion sites in EG4 and related strains, by using high-throughput short-reads sequencing data. In that paper, the authors analyzed the whole-genome sequencing reads obtained from the Illumina platform using a tool they developed, RelocaTE2 (Chen et al., 2017). RelocaTE2 detects the insertion sites of a known TE using resequencing data, by searching junction reads which contain parts of the TE sequence and parts of the unique host genomic sequence (Chen et al., 2017). In Chen et al. (2019), the copy number of mPing was estimated to be 437 in EG4. By contrast, our study found an average of 573.1 (min: 495, max: 655) mPing insertion sites identified in each line derived from the EG4 population (Supplementary Table 7). Therefore, our methods can detect more mPing insertion sites than that previous study. Moreover, we applied and compared two different analytical methods, clustering-based and alignment-based, to identify the mPing insertion sites. More than 95% of mPing insertion sites were identified by both methods in the tested three lines (Table 1). These identified insertion sites were amplified as expected by PCR and verified experimentally, confirming our analytical methods are highly effective and reliable.
In this study, we aimed to identify the causal genes underpinning the high-protein phenotype in the HP lines (HP5-7 and HP7-5). Unfortunately, we could not detect any candidate genes. Nevertheless, most of the genes encoding glutelin, prolamin, and globulin were clearly up-regulated in both HP lines (Figure 4). Accordingly, it seems the expression of multiple genes may contribute to the greater protein content, rather than one major gene per se being responsible for increasing the protein content. In addition, given the reduced expression of many genes related to photosynthesis, as inferred from the DEG analysis (Figure 6), the starch content likely decreased in HP5-7 and HP7-5. Photosynthesis is a process whereby plants convert light energy into chemical energy; the former is used to convert water, carbon dioxide, and minerals into oxygen and energy-rich organic compounds. In most green plants, carbohydrates, especially starch and the sugar sucrose, are the direct, major organic products of photosynthesis. In a previous study, nitrogen fertilization reduced the expression of genes related to starch synthesis and decreased the storage starch content, while increasing the expression of genes related to amino acid biosynthesis and increasing the storage protein content, implying a trade-off between protein and starch synthesis (Midorikawa et al., 2014). Therefore, a decreased starch synthesis in the HP lines may have increased protein content. When we quantified the starch content in rice landraces varying in their protein content, a strong negative correlation was clearly confirmed between the starch and protein content (unpublished data). Based on the above, we posit that the expression of those genes involved in photosynthesis may indirectly contribute to the improved protein content of rice. In the near future, we plan to further investigate both HP lines, in terms of their starch and protein synthesis, which should point to the network of causal genes responsible for their high protein content. Still, for unknown reasons, many DEGs were down-regulated in the HP lines compared with C3-1 (Supplementary Figure 3). It is interesting that thousands of DEGs were detected, even though HP lines and C3-1 have the same genetic background derived from the EG4 strain. Of the detected DEGs, 0.48% (12/2,478) and 0.39% (10/2,593) of DEGs have an mPing insertion within 3 kb in HP5-7 and HP7-5, respectively, which indicates that the vast majority of DEGs were not affected by an mPing insertion. Accordingly, researchers should also consider the possibility that other factors besides mPing might cause an increase in the protein content of HP lines of rice.
Conclusion
In this study, we selected two rice lines (HP5-7 and HP7-5) with high protein content from the unique EG4 population in which mPing is actively transposing under natural conditions, and analyzed their mPing insertion sites and their transcriptome. Given that the mPing insertion sites identified by NGS were confirmed experimentally, we consider our analytical methods to be highly effective and reliable. Many of the detected mPing insertion sites were positioned near the gene (i.e., within 3 kb), which suggests that mPing tends to affect the transcription activity of those genes. Transcriptomics revealed that most genes encoding glutelin, prolamin, and globulin were up-regulated in both HP5-7 and HP7-5 lines. Conversely, many genes had expression levels that were lower in the HP lines than the control C3-1 line, and most of the DEGs near mPing insertion sites were also down-regulated. In particular, there tends to be reduced expression of photosynthesis-related genes in the HP lines, which suggests that decreased starch content may contribute to greater protein content. In the future, we plan to identify the causal genes responsible for the high protein content of HP lines, by considering the involvement of genes related to photosynthesis and starch synthesis. Further, we anticipate the high-protein lines detected here could lead to the development of high protein rice cultivars by introducing valuable traits such as high and stable yield, disease resistance, and rich nutrient content.
Data availability statement
The datasets presented in this study can be found in the DDBJ repository, accession numbers DRA014320 and DRA014328.
Author contributions
TY and YM conceived and designed the experiments. YM, HT, and TY conducted the experiments and data analysis to identify the mPing insertion sites. RF and TY performed the transcriptome experiments and analyzed that data. EK and KM measured the protein content of rice seeds. SS performed the experiments that validated the mPing insertion sites and transcriptome analysis. KY cultivated the experiments’ rice plants. YM wrote the manuscript. All authors contributed to the article and approved the submitted version.
Funding
This work was supported by the Japanese Society for the Promotion of Science (JSPS) [KAKENHI, grant number 22H02311 (to TY)] and by the Japanese Science and Technology (JST) agency [COI grant number JPMJCE1307 (to TY)].
Acknowledgments
We would like to express our thanks to Tsuyoshi Tanaka (NARO) for providing the sequence information of the rice reference genome, and to Tao Watanabe (Graduate School of Agriculture Kyoto University) for generating the gene expression heatmap. Computations were partially performed on the NIG supercomputer at ROIS National Institute of Genetics.
Conflict of interest
The authors declare that the research was conducted in the absence of any commercial or financial relationships that could be construed as a potential conflict of interest.
Publisher’s note
All claims expressed in this article are solely those of the authors and do not necessarily represent those of their affiliated organizations, or those of the publisher, the editors and the reviewers. Any product that may be evaluated in this article, or claim that may be made by its manufacturer, is not guaranteed or endorsed by the publisher.
Supplementary material
The Supplementary material for this article can be found online at: https://www.frontiersin.org/articles/10.3389/fpls.2022.969582/full#supplementary-material
Footnotes
1. ^http://cell-innovation.nig.ac.jp/index_en.html(Accessed July 17, 2019).
2. ^https://rapdb.dna.affrc.go.jp/download/irgsp1.html
3. ^https://www.bioinformatics.babraham.ac.uk/projects/trim_galore/
References
Arnold, C., and Hodgson, I. J. (1991). Vectorette PCR: a novel approach to genomic walking. PCR Methods Appl. 1, 39–42. doi: 10.1101/gr.1.1.39
Bolger, A. M., Lohse, M., and Usadel, B. (2014). Trimmomatic: a flexible trimmer for Illumina sequence data. Bioinformatics 30, 2114–2120. doi: 10.1093/bioinformatics/btu170
Butelli, E., Licciardello, C., Zhang, Y., Liu, J., Mackey, S., Bailey, P., et al. (2012). Retrotransposons control fruit-specific, cold-dependent accumulation of anthocyanins in blood oranges. Plant Cell 24, 1242–1255. doi: 10.1105/tpc.111.095232
Casa, A. M., Brouwer, C., Nagel, A., Wang, L., Zhang, Q., Kresovich, S., et al. (2000). The MITE family heartbreaker (Hbr): molecular markers in maize. Proc. Natl. Acad. Sci. U. S. A. 97, 10083–10089. doi: 10.1073/pnas.97.18.10083
Chen, L., Lu, L., Benjamin, J., Diaz, S., Hancock, C. N., Jason, E. S., et al. (2019). Tracking the origin of two genetic components associated with transposable element bursts in domesticated rice. Nat. Commun. 10:641. doi: 10.1038/s41467-019-08451-3
Chen, P., Shen, Z., Ming, L., Li, Y., Dan, W., Lou, G., et al. (2018). Genetic basis of variation in rice seed storage protein (albumin, globulin, prolamin, and glutelin) content revealed by genome-wide association analysis. Front. Plant Sci. 9:612. doi: 10.3389/fpls.2018.00612
Chen, J., Wrightsman, T. R., Wessler, S. R., and Stajich, J. E. (2017). RelocaTE2: A high resolution transposable element insertion site mapping tool for population resequencing. PeerJ. 5:e2942. doi: 10.7717/peerj.2942
Eckardt, N. A. (2000). Sequencing the rice genome. Plant Cell 12, 2011–2017. doi: 10.1105/tpc.12.11.2011
Ellepola, S., Choi, S., Phillips, D., and Ma, C. (2006). Raman spectroscopic study of rice globulin. J. Cereal Sci. 43, 85–93. doi: 10.1016/j.jcs.2005.06.006
Feschotte, C. (2008). Transposable elements and the evolution of regulatory networks. Nat. Rev. Genet. 9, 397–405. doi: 10.1038/nrg2337
Feschotte, C., Jiang, N., and Wessler, S. R. (2002). Plant transposable elements: where genetics meets genomics. Nat. Rev. Genet. 3, 329–341. doi: 10.1038/nrg793
Feschotte, C., and Pritham, E. J. (2007). DNA transposons and the evolution of eukaryotic genomes. Annu. Rev. Genet. 41, 331–368. doi: 10.1146/annurev.genet.40.110405.090448
Ge, S. X., Jung, D., and Yao, R. (2020). ShinyGO: A graphical gene-set enrichment tool for animals and plants. Bioinformatics 36, 2628–2629. doi: 10.1093/bioinformatics/btz931
Hancock, C. N., Zhang, F., and Wessler, S. R. (2010). Transposition of the tourist-MITE mPing in yeast: an assay that retains key features of catalysis by the class 2 PIF/harbinger superfamily. Mob. DNA 1:5. doi: 10.1186/1759-8753-1-5
Henchion, M., Hayes, M., Mullen, A. M., Fenelon, M., and Tiwari, B. (2017). Future protein supply and demand: strategies and factors influencing a sustainable equilibrium. Foods 6:6. doi: 10.3390/foods6070053
Higashi, T., Kushibuchi, K., and Ito, R. (1974). Studies on breeding for high protein rice: I. Protein content of different rice varieties and their relations with some agronomic traits including yield. Japan. J. Breed. 24, 88–96.
Hirata, C., Waki, T., Shimomura, K., Wada, T., Tanaka, S., Ikegami, H., et al. (2020). DNA markers based on retrotransposon insertion polymorphisms can detect short DNA fragments for strawberry cultivar identification. Breed. Sci. 70, 231–240. doi: 10.1270/jsbbs.19116
Jiang, N., Bao, Z., Zhang, X., Hirochika, H., Eddy, S. R., McCouch, S. R., et al. (2003). An active DNA transposon family in rice. Nature 421, 163–167. doi: 10.1038/nature01214
Jiang, C., Cheng, Z., Zhang, C., Yu, T., Zhong, Q., Shen, J. Q., et al. (2014). Proteomic analysis of seed storage proteins in wild rice species of the Oryza genus. Proteome Sci. 12:51. doi: 10.1186/s12953-014-0051-4
Jung, S., Rickert, D. A., Deak, N. A., Aldin, E. D., Recknor, J., Johnson, L. A., et al. (2003). Comparison of kjeldahl and dumas methods for determining protein contents of soybean products. J. Amer. Oil Chem. Soc. 80:1169. doi: 10.1007/s11746-003-0837-3
Kawahara, Y., de la Bastide, M., Hamilton, J. P., Kanamori, H., McCombie, W. R., Ouyang, S., et al. (2013). Improvement of the Oryza sativa Nipponbare reference genome using next generation sequence and optical map data. Rice 6:4. doi: 10.1186/1939-8433-6-4
Kawakatsu, T., Yamamoto, M. P., Hirose, S. Y., and Takaiwa, F. (2008). Characterization of a new rice glutelin gene GluD-1 expressed in the starchy endosperm. J. Exp. Bot. 59, 4233–4245. doi: 10.1093/jxb/ern265
Kent, W. J. (2002). BLAT—the BLAST-like alignment tool. Genome Res. 12, 656–664. doi: 10.1101/gr.229202
Kikuchi, K., Terauchi, K., Wada, M., and Hirano, H. Y. (2003). The plant MITE mPing is mobilized in anther culture. Nature 421, 167–170. doi: 10.1038/nature01218
Kim, D., Paggi, J. M., Park, C., and Salzberg, S. L. (2019). Graph-based genome alignment and genotyPing with HISAT2 and HISAT-genotype. Nat. Biotechnol. 37, 907–915. doi: 10.1038/s41587-019-0201-4
Kubota, M. (2016). Novel physiological functions of rice protein. J. Jpn. Soc. Nutr. Food Sci. 69, 283–288. doi: 10.4327/jsnfs.69.283
Kumar, A., and Bennetzen, J. L. (1999). Plant retrotransposons. Annu. Rev. Genet. 33, 479–532. doi: 10.1146/annurev.genet.33.1.479
Li, H., and Durbin, R. (2009). Fast and accurate short read alignment with burrows-wheeler transform. Bioinformatics 25, 1754–1760. doi: 10.1093/bioinformatics/btp324
Lin, S. K., Chang, M. C., Tsai, Y. G., and Lur, H. S. (2005). Proteomic analysis of the expression of proteins related to rice quality during caryopsis development and the effect of high temperature on expression. Proteomics 5, 2140–2156. doi: 10.1002/pmic.200401105
Love, M. I., Huber, W., and Anders, S. (2014). Moderated estimation of fold change and dispersion for RNA-seq data with DESeq2. Genome Biol. 15:550. doi: 10.1186/s13059-014-0550-8
Martin, M. (2011). Cutadapt removes adapter sequences from high-throughput sequencing reads. EMBnet J. 17, 10. doi: 10.14806/ej.17.1.200
Martin, M., and Fitzgerald, M. A. (2002). Proteins in rice grains influence cooking properties. J. Cereal Sci. 36, 285–294. doi: 10.1006/jcrs.2001.0465
Midorikawa, K., Kuroda, M., Terauchi, K., Hoshi, M., Ikenaga, S., Ishimaru, Y., et al. (2014). Additional nitrogen fertilization at heading time of rice down-regulates cellulose synthesis in seed endosperm. PLoS One 9:e98738. doi: 10.1371/journal.pone.0098738
Monden, Y., Hara, T., Okada, Y., Jahana, O., Kobayashi, A., Tabuchi, H., et al. (2015). Construction of a linkage map based on retrotransposon insertion polymorphisms in sweet potato via high-throughput sequencing. Breed. Sci. 65, 145–153. doi: 10.1270/jsbbs.65.145
Monden, Y., Naito, K., Okumoto, Y., Saito, H., Oki, N., Tsukiyama, T., et al. (2009). High potential of a transposon mPing as a marker system in japonica × japonica cross in rice. DNA Res. 16, 131–140. doi: 10.1093/dnares/dsp004
Monden, Y., Yamamoto, A., Shindo, A., and Tahara, M. (2014). Efficient DNA fingerprinting based on the targeted sequencing of active retrotransposon insertion sites using a bench-top high-throughput sequencing platform. DNA Res. 21, 491–498. doi: 10.1093/dnares/dsu015
Naito, K., Cho, E., Yang, G., Campbell, M. A., Yano, K., Okumoto, Y., et al. (2006). Dramatic amplification of a rice transposable element during recent domestication. Proc. Natl. Acad. Sci. U. S. A. 103, 17620–17625. doi: 10.1073/pnas.0605421103
Naito, K., Monden, Y., Yasuda, K., Saito, H., and Okumoto, Y. (2014). mPing: the bursting transposon. Breed. Sci. 64, 109–114. doi: 10.1270/jsbbs.64.109
Naito, K., Zhang, F., Tsukiyama, T., Saito, H., Hancock, C. N., Richardson, A. O., et al. (2009). Unexpected consequences of a sudden and massive transposon amplification on rice gene expression. Nature 461, 1130–1134. doi: 10.1038/nature08479
Nakazaki, T., Okumoto, Y., Horibata, A., Yamahira, S., Teraishi, M., Nishida, H., et al. (2013). Mobilization of a transposon in the rice genome. Nature 421, 170–172. doi: 10.1038/nature01219
Pertea, M., Kim, D., Pertea, G. M., Leek, J. T., and Salzberg, S. L. (2016). Transcript-level expression analysis of RNA-seq experiments with HISAT, StringTie and Ballgown. Nat. Protoc. 11, 1650–1667. doi: 10.1038/nprot.2016.095
Pertea, M., Pertea, G. M., Antonescu, C. M., Chang, T. C., Mendell, J. T., and Salzberg, S. L. (2015). StringTie enables improved reconstruction of a transcriptome from RNA-seq reads. Nat. Biotech. 33, 290–295. doi: 10.1038/nbt.3122
Sakai, H., Lee, S. S., Tanaka, T., Numa, H., Kim, J., Kawahara, Y., et al. (2013). Rice annotation project database (RAP-DB): an integrative and interactive database for rice genomics. Plant Cell Physiol. 54:e6. doi: 10.1093/pcp/pcs183
Sasai, R., Tabuchi, H., Shirasawa, K., Kishimoto, K., Sato, S., Okada, Y., et al. (2019). Development of molecular markers associated with resistance to Meloidogyne incognita by performing quantitative trait locus analysis and genome-wide association study in sweet potato. DNA Res. 26, 399–409. doi: 10.1093/dnares/dsz018
Shewry, P. R. (2007). Improving the protein content and composition of cereal grain. J. Cereal Sci. 46, 239–250. doi: 10.1016/j.jcs.2007.06.006
Takagi, K., Ishikawa, N., Maekawa, M., Tsugane, K., and Iida, S. (2007). Transposon display for active DNA transposons in rice. Genes Genet. Syst. 82, 109–122. doi: 10.1266/ggs.82.109
Tang, Y., Horikoshi, M., and Li, W. (2016). Ggfortify: unified Interface to visualize statistical result of popular R packages. R J. 8, 474–485. doi: 10.32614/RJ-2016-060
Turucotte, K., Srinivsan, S., and Bureau, T. (2001). Survey of transposable elements from rice genomic sequences. Plant J. 25, 169–179. doi: 10.1046/j.1365-313x.2001.00945.x
Waskom, M. L. (2021). Seaborn: statistical data visualization. J. Open Source Soft. 6:3021. doi: 10.21105/joss.03021
Wessler, S. R. (2006). Transposable elements and the evolution of eukaryotic genomes. Proc. Natl. Acad. Sci. U. S. A. 103, 17600–17601. doi: 10.1073/pnas.0607612103
Yamagata, H., Sugimoto, T., Tanaka, K., and Kasai, Z. (1982). Biosynthesis of storage proteins in develoPing rice seeds. Plant Physiol. 70, 1094–1100. doi: 10.1104/pp.70.4.1094
Keywords: amplicon sequencing, protein, rice, RNA-seq, starch, transposon
Citation: Monden Y, Tanaka H, Funakoshi R, Sunayama S, Yabe K, Kimoto E, Matsumiya K and Yoshikawa T (2022) Comprehensive survey of transposon mPing insertion sites and transcriptome analysis for identifying candidate genes controlling high protein content of rice. Front. Plant Sci. 13:969582. doi: 10.3389/fpls.2022.969582
Edited by:
Gennady I. Karlov, All-Russia Research Institute of Agricultural Biotechnology, RussiaReviewed by:
Wen Yao, Henan Agricultural University, ChinaPeijian Cao, Zhengzhou Tobacco Research Institute of CNTC, China
Copyright © 2022 Monden, Tanaka, Funakoshi, Sunayama, Yabe, Kimoto, Matsumiya and Yoshikawa. This is an open-access article distributed under the terms of the Creative Commons Attribution License (CC BY). The use, distribution or reproduction in other forums is permitted, provided the original author(s) and the copyright owner(s) are credited and that the original publication in this journal is cited, in accordance with accepted academic practice. No use, distribution or reproduction is permitted which does not comply with these terms.
*Correspondence: Takanori Yoshikawa, eW9zaGlrYXdhLnRha2Fub3JpLjVzQGt5b3RvLXUuYWMuanA=