- 1Plant Genetics and Breeding Lab, Department of Forest and Environment System, College of Forest and Environmental Science, Kangwon National University, Chuncheon, Republic of Korea
- 2Forest Technology and Management Research Center, National Institute of Forest Science, Pocheon, Republic of Korea
Larix kaempferi (Lamb.) Carrière, a valuable species for timber production and reforestation, faces challenges in large-scale seedling propagation due to its slow growth cycle and high susceptibility to environmental stressors. Early detection of drought stress is critical for preparing seedlings for harsh field conditions and for optimizing irrigation strategies. This study aimed to detect drought stress at an early stage in L. kaempferi seedlings by integrating physiological traits with image-based phenotypic measurements, with a focus on needle angle dynamics under controlled drought and irrigation conditions. The apical needle angle of one-year-old seedlings was measured using ImageJ, while seedling-level analysis was conducted using PlantCV to collect data and extract relevant parameters. Statistical analyses were performed to evaluate temporal trends and to identify growth environment and physiological traits significantly influenced by drought stress. As a result, apical needle wilting and recovery, along with seedling-level image analysis (parameter: Center of Mass(y)), exhibited significant responses to drought stress as early as Day 2. This provides a non-destructive method for early detection, preceding observable changes in physiological traits such as chlorophyll fluorescence and needle temperature that responded to drought stress by Day 6, as well as before seedling mortality occurred. Multiple regression analysis indicated that, as drought stress progressed, solar radiation and thermal-related parameters (ФNPQ and needle temperature) emerged as key predictors of needle angle variation. Image-based approaches, including RGB and thermal imaging, proved effective for real-time stress monitoring, demonstrating their practical potential for nursery applications. In summary, this study lays the groundwork for needle-based phenomic approaches using imaging techniques in nursery systems and highlights the need for further research to optimize these methods for the large-scale, cost-effective production of high-quality, drought-resilient L. kaempferi seedlings.
1 Introduction
Larix kaempferi (Lamb.) Carrière (Japanese larch) is a deciduous conifer belonging to the Pinaceae family, predominantly distributed in East Asia (Nagaike et al., 2003; Choi et al., 2021; Wu et al., 2021). In Korea, L. kaempferi is widely valued for its use as timber and as a key species for reforestation (Kim et al., 2013). However, despite its high demand, the extended larch seed production cycle of at least 2 to 3 years makes it difficult to meet demand without man-made processes (Kaliniewicz et al., 2012). Large-scale seedling production using controlled nursery systems is a viable solution to this challenge. Nonetheless, such systems remain labor-intensive and costly, necessitating improvements in production structure to ensure broader adoption.
Global warming and climate change intensify environmental stresses, significantly impacting L. kaempferi growth, physiology, and ecology (Wu et al., 2021). Among these, water is vital for all organisms, with prolonged drought stress having particularly adverse effects on plants (Trenberth et al., 2014; Sarris and Christodoulakis, 2024). In particular, seedlings, being young and vulnerable, are especially prone to drought-induced damage (Khurana and Singh, 2004; Galvez et al., 2011; Fernández et al., 2014). When transplanted from controlled nursery environments to field conditions, seedlings are exposed to severe stresses, necessitating adequate preparation (Allen et al., 2017; South et al., 2023). Priming, a method of inducing controlled stress, has been reported to enhance drought tolerance. This process involves exposing plants to mild stress conditions, such as limited water availability, to trigger adaptive responses that improve resilience to subsequent, more severe stress (Grossnickle, 2012; Abid et al., 2016; Abdallah et al., 2017; Tankari et al., 2021; Sintaha et al., 2022; Ru et al., 2022). Therefore, the development of techniques to diagnose drought stress at early stages is crucial for preparing seedlings for climate-related challenges and for implementing efficient irrigation systems alongside priming treatments.
Phenotypes refer to the observable traits of organisms, such as their physical structure, physiological processes, and responses to environmental factors. By defining and quantifying tree stress through phenotypic measurements, subjective evaluation criteria can be standardized and objectified. This data can be used to promptly assess plant health and respond effectively. The imaging-based plant phenomics, which comprehensively analyzes phenotypic traits along with genetic and environmental characteristics, has been gaining attention for its applicability across various fields (Kumar et al., 2015; Tardieu et al., 2017; Perez-Sanz et al., 2017; Pasala and Pandey, 2020). Depending on the method, high-cost equipment (e.g., 3D imaging, hyperspectral imaging) allows for precise measurements but requires technical expertise (Forsström et al., 2021; Pisek et al., 2023). On the other hand, while low-cost equipment (e.g., RGB imaging, thermal imaging) may have limitations in precision, they can still be supplemented based on their intended use and are more practical for widespread adoption (Cozzolino, 2023; Naqvi et al., 2024). In plant stress research, RGB imaging is widely used with unmanned aerial vehicles (UAVs) and thermal cameras to measure drought stress (Zhang et al., 2019a; Su et al., 2020; De Swaef et al., 2021; Chandel et al., 2022). However, early-stage stress detection is challenging using RGB-based vegetation indices, making them more suitable for field monitoring than for precise, real-time measurements at the individual plant level. Additionally, long-term monitoring studies integrating morphological parameters often face limitations in real-time stress detection (Zhang et al., 2019b; Bhusal et al., 2020, 2021; Yasin et al., 2024).
Leaf movement is a key physiological mechanism that supports plant survival, offering insights into a plant’s adaptability to its environment and tolerance to abiotic stress (Yang et al., 2023). Accordingly, leaf angle measurements offer a promising alternative for early drought stress detection (Ehleringer and Comstock, 1987; Yavas et al., 2024). In controlled nursery environments, where external factors such as wind are minimized, the utility of leaf angle measurements is expected to increase. Advancements in real-time leaf angle monitoring techniques further enhance its applicability (Kenchanmane Raju et al., 2020; Geldhof et al., 2021; Kattenborn et al., 2022; Jiang et al., 2024; Oskam et al., 2024). Recently, leaf angle has been targeted in breeding programs aimed at improving crop yield, leading to an increasing number of studies integrating leaf angle traits with agronomic research (Ji et al., 2024; Liu et al., 2024; Wu et al., 2024). However, research on the correlation between leaf angle and tree physiological traits (e.g., chlorophyll content, chlorophyll fluorescence, photosynthesis, and water status) remains limited, emphasizing the need for further investigation, particularly from the perspective of tree breeding. Moreover, tracking needle angle in conifers presents a significant challenge, highlighting the necessity for research aimed at developing more accessible and efficient measurement methods.
This study aimed to identify suitable parameters for early diagnosis of drought stress in L. kaempferi seedlings by exploring image-based changes in needle angles. Needle angle is particularly useful because it responds rapidly to changes in water availability, reflecting early physiological stress before visible symptoms such as wilting occur. This makes it an effective and non-destructive indicator for detecting drought stress at early stages. To quantify drought stress objectively, the study analyzed apical needle angles and individual seedling images, alongside greenhouse environmental parameters and tree physiological parameters, such as chlorophyll content, chlorophyll fluorescence, electrical conductivity, and needle temperature parameters.
2 Materials and methods
2.1 Plant materials
The study used 1-year-old L. kaempferi seedlings (mean height: 24.3 ± 3.3 cm, mean root collar diameter: 2.8 ± 0.4 mm), produced at the Forest Technology and Management Research Center, National Institute of Forest Science (37°45’39”N, 127°10’13”E). Seeds were submerged for 2 days in mid-April 2024 and sown at a rate of three seeds per 320 mL container filled with a growth medium (peat moss: perlite: vermiculite = 1:1:1, v/v). From April to August, the seedlings were irrigated daily with 20 L/m² using a sprinkler system. From June onward, seedlings were fertilized weekly with a 1 g/L (1,000 ppm) solution of MultiFeed 19 (19N:19P2O5:19K2O; Haifa Chemicals, Haifa, Israel) alongside irrigation. On August 8, seedlings underwent acclimatization in a greenhouse at the College of Forest and Environmental Sciences, Kangwon National University (37°52’00”N, 127°44’51”E).
2.2 Experimental conditions
The drought stress experiment was conducted over a 7-day (BD: before drought to D6: Day 6 of the experiment) period from August 16 to August 22, 2024, in a greenhouse at Kangwon National University. A total of 60 seedlings were randomly selected, with 10 seedlings per treatment chosen for soil temperature (ST) and moisture (SM) with electrical conductivity (EC) (n = 10), physiological (n =10), and image-based measurements (n =10). Treatments were as follows:
· Control (n = 30): irrigated daily with 2.35 L/container between 11:00 AM and 12:00 PM.
· Drought (n = 30): irrigated the same as the control and no irrigation from D1.
Greenhouse air temperature (AT), humidity (AH), and solar radiation (SR) were continuously monitored using Juns OL sensors (PurumBio, Suwon, Korea). Soil temperature and moisture were recorded at 30 min intervals using a Hobo micro station (H21-USB, Onset, Bourne, MA, USA) equipped with temperature (S-SMD-M005) and moisture sensors (S-TMB-M002).
2.3 Physiological measurements
Chlorophyll index (SPAD) and fluorescence (Fm’: maximum fluorescence in light-adapted state, Fo’: minimum fluorescence in light-adapted state, Fv’/Fm’: maximum quantum yield of PSII in light-adapted state, ΦII: quantum yield of PSII, ΦNO: quantum yield of non-regulated energy dissipation, ΦNPQ: quantum yield of regulated energy dissipation, PSIact: PSI activity, PSIopen: open reaction centers in PSI, qL: fraction of open PSII reaction centers) were measured using a MultispeQ V2.0 (PhotosynQ, East Lansing, MI, USA). Measurements were repeated five times per seedling across different needle bundles every two days, starting from D2 of the experiment (between 12:00 PM and 6:00 PM). EC was monitored in real time using Juns OL sensors (PurumBio, Suwon, Korea), with electrodes attached to the seedling stem, 3 cm above the soil surface.
2.4 Image-based measurements
To collect RGB images for needle angle measurements, side-view images of control (n = 2) and drought-treated seedlings (n = 2) were captured together in a single frame (n = 4). Five images were simultaneously taken at 10 min intervals using a time lapse camera (ATL200S, Afidus, New Taipei City, Taiwan). The camera was positioned at the same height as the target pots, 60 cm away from the seedlings, with a black background placed behind the seedlings to minimize visual interference. To reduce image distortion, wide-angle, digital zoom, image stabilization, and automatic exposure optimization functions were turned off, and images were captured at a 1:1 aspect ratio. Thermal images were obtained using a thermal camera (PI 160i, Optris, Berlin, Germany) at approximately 2–3 m distance, starting from 12:00 PM, aligning with the RGB image collection.
2.5 Data processing
2.5.1 Needle angle measurements
In this study, needle angles at the apical part of seedling, which respond rapidly to drought stress, were primarily measured. The needle angle (θ) was defined as the interior angle (± 90°) formed by drawing a horizontal line from the base of the needle to its tip (Figure 1). However, since it is challenging to track a single needle consistently over time, and individual measurements may vary significantly, the apical images were divided into four quadrants. Needle angles within each quadrant were measured using ImageJ software ver. 1.53k (NIH, Bethesda, MD, USA), and the average value was taken as the resultant needle angle.
The needle angles exhibited significant changes at 9:00 AM and 6:00 PM, which led to data being collected twice per day (CM: control morning, CE: control evening, DM: drought treatment morning, DE: drought treatment evening). Seedlings that became completely discolored and wilted during the experiment were excluded from the daily data analysis (included only if the apical part is measurable). The analysis continued until at least 30 needle angle values were available from surviving seedlings in the drought treatment for RMANOVA analysis. Needle angle parameters were categorized as follows:
· STx: needle angle ranges divided into six intervals (ST1: – 90° ≤ θ < – 60°, ST2: – 60° ≤ θ < – 30°, ST3: – 30° ≤ θ < 0°, ST4: 0° ≤ θ < + 30°, ST5: + 30° ≤ θ < + 60°, ST6: + 60° ≤ θ ≤ + 90°).
· BD-M: needle angle before drought treatment – current needle angle.
· PM-M: previous needle angle – current needle angle (e.g., Day 1 evening – Day 2 morning, Day 2 morning – Day 2 evening).
· PM-M(ST): previous needle angle measured at the same time of day – current needle angle (e.g., Day 1 morning – Day 2 morning, Day 1 evening – Day 2 evening).
2.5.2 Seedling level measurements
An image analysis method using the Python-based PlantCV framework (https://plantcv.readthedocs.io/en/stable/) was applied (Gehan et al., 2017). The analysis protocol is as follows (Figure 2):
· Color correction: the range of images to be used was set, and the image colors were corrected based on a color chart. By standardizing the colors to reduce the influence of lighting, this process helped mitigate distortions of the plant in the image, enabling a more accurate analysis.
· Mask creation: a mask was created to distinguish the plant from the background, defining the range for the computer to analyze. After color correction, a suitable color range for the plant was set based on color distribution, and a new image was created that matched the plant’s shape in the original image. In the mask image, pixels within the defined color range were converted to white (255, 255, 255), while pixels outside the range were converted to black (0, 0, 0), resulting in a binary image. Noise was then removed using the fill and fill_holes functions. In this study, as each image contained four plants, an additional step was taken to create an overall mask image and then separate it into individual plant masks. The desired range (object) was defined to generate masks for each plant. This range included not only the defined rectangular area but also any connected objects outside the rectangle within the range.
· Data extraction: the prepared image and mask were compared to extract image information for the selected plants. The extracted data was based on the pixels and coordinates within the image. The range measured could be confirmed through the returned images after extraction. As traditional data extraction methods were often difficult for users to interpret, only the necessary data were selected and extracted. In this study, the extracted parameters included Area (surface area), Longest Path (longest distance passing through the center), Width (maximum horizontal length), Height (maximum vertical length), Convex Hull Area (area of the polygon connecting the outermost points), Solidity (density, calculated as the ratio of Area to Convex Hull Area), Perimeter (outline of the mask), Center of Mass(x, y) (center point of all pixels), Convex Hull Vertice (number of vertices in the polygon connecting the outermost points), and Ellipse Center(x, y) (center of the ellipse fitted to the outline) (Figure 3).
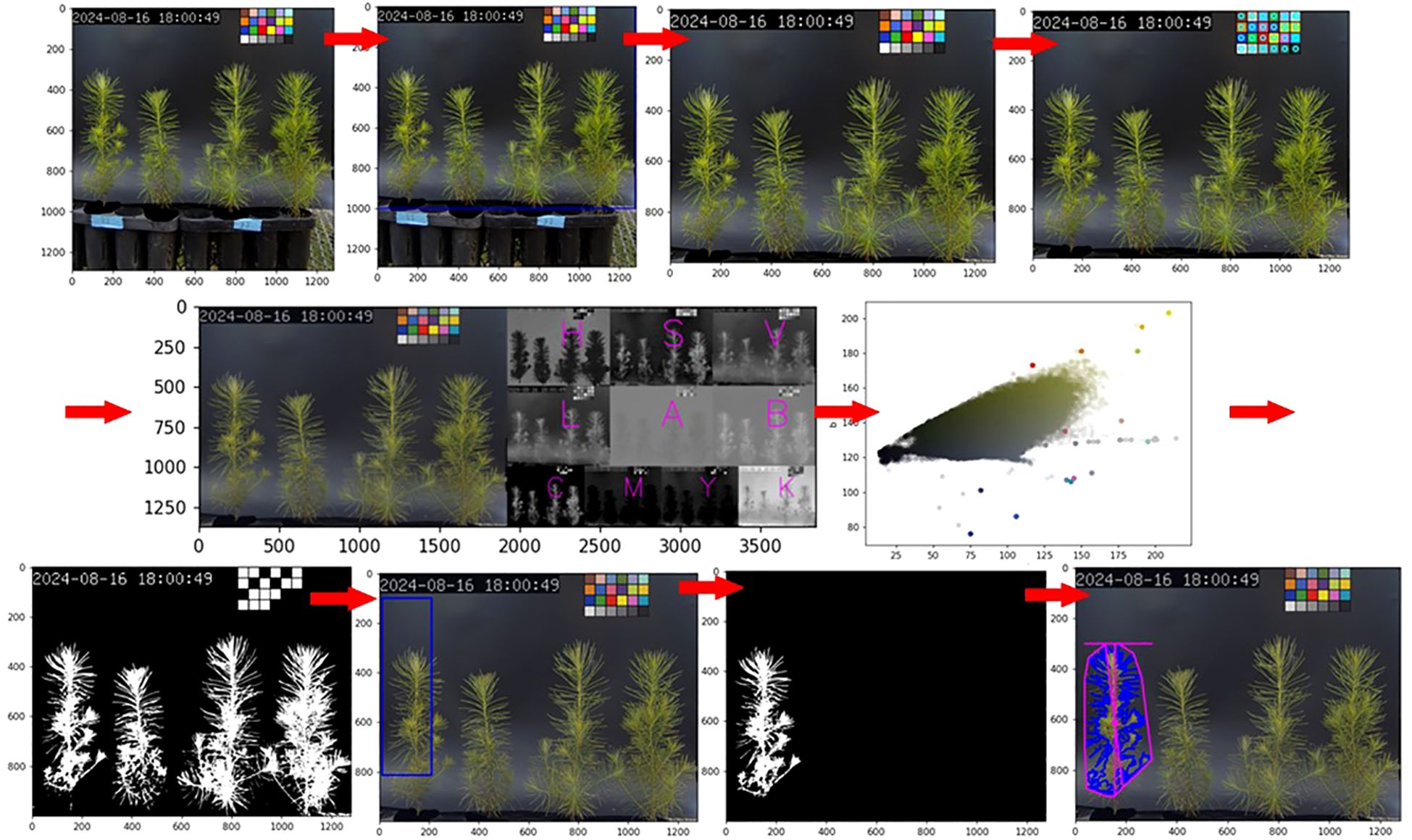
Figure 2. Seedling level parameter extraction process using PlantCV (color correction, mask creation, and data extraction). The extracted parameters were analyzed using the Kruskal-Wallis test across different days and treatments, followed by the Mann-Whitney test with Bonferroni correction for post-hoc analysis (P < 0.05).
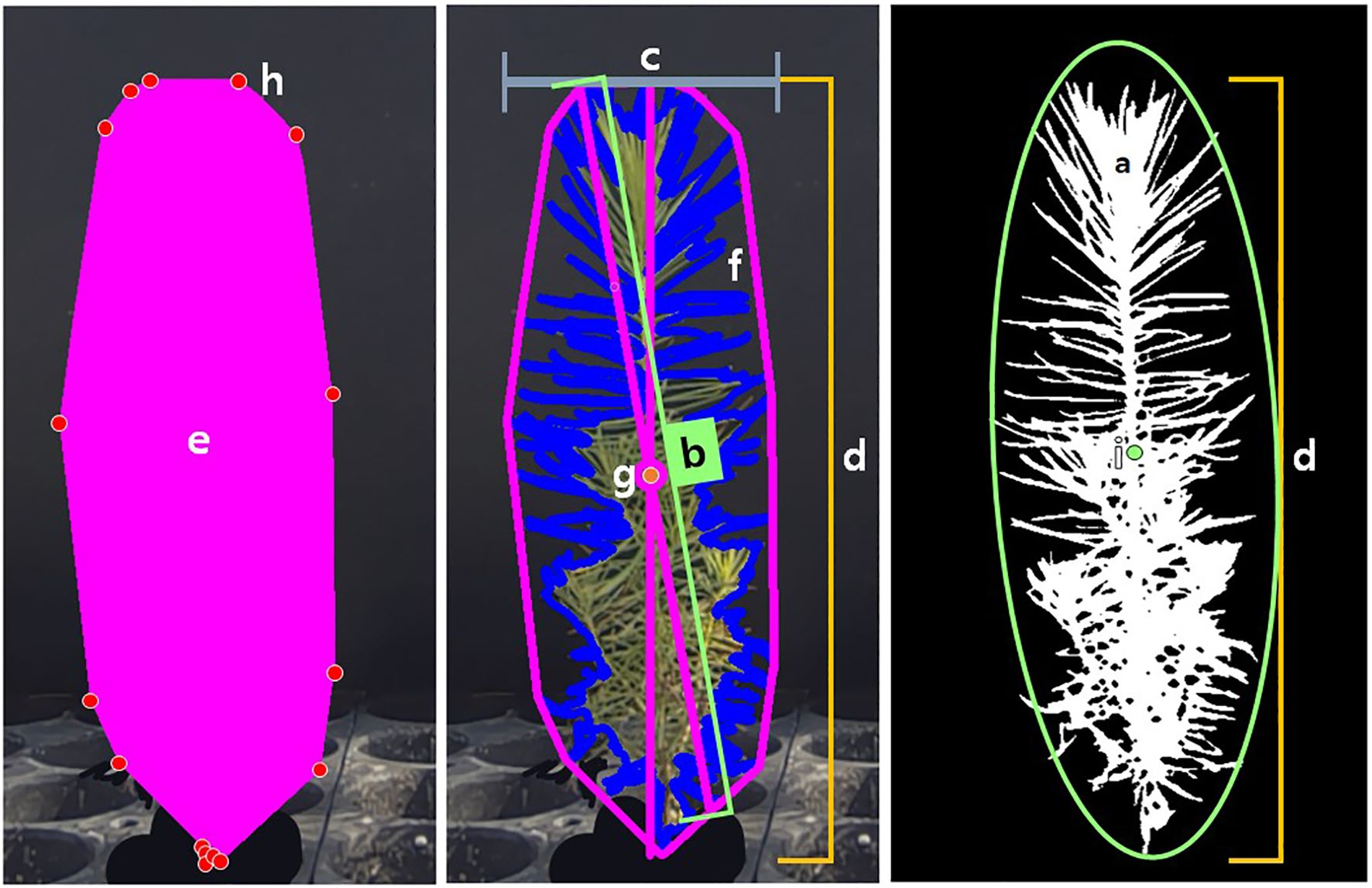
Figure 3. Criteria for seedling level parameters. (a) Area (surface area); (b) Longest Path (longest distance passing through the center); (c) Width (maximum horizontal length); (d) Height (maximum vertical length); (e) Convex Hull Area (area of the polygon connecting the outermost points); a/e: Solidity (density, calculated as the ratio of Area to Convex Hull Area); (f) Perimeter (outline of the mask); (g) Center of Mass(x,y) (center point of all pixels); (h) Convex Hull Vertice (number of vertices in the polygon connecting the outermost points); (i) Ellipse Center(x,y) (center of the ellipse fitted to the outline).
2.5.3 Needle temperature parameters
Needle temperature was measured using IR camera software PIX Connect Rel. 3.6.3046.0 (Optris, Berlin, Germany) by labeling clustered needle areas and collecting the average needle temperature within those areas. Needle (leaf) temperature parameters, including vapor pressure deficit (VPD) (Stull, 2015; Grossiord et al., 2020), crop water stress index (CWSI), and leaf temperature difference (LTD), were derived from the collected needle temperature data (Gardner et al., 1992; Zhou et al., 2021). Needle temperature parameters are calculated using the following Equations 1-6:
The meanings of each abbreviation are as follows; LVP: leaf vapor pressure, AVP: air vapor pressure, Tl: leaf temperature, Ta: air temperature, AH (RH): air humidity (relative humidity), Tlw: minimum leaf temperature, Tld: maximum leaf temperature, Taw: minimum air temperature, Tad: maximum air temperature.
2.6 Statistical analysis
A 2-way RMANOVA (repeated measures analysis of variance) was conducted for physiological traits (n = 30), whereas a 3-way RMANOVA was performed for needle angle parameters (n = 30). Post-hoc analysis was carried out using pairwise t-tests with Bonferroni correction (P < 0.05). For seedling level parameters (n ≤ 10), the Mann-Whitney U test with Bonferroni correction (P < 0.05) was used as a post-hoc analysis following the Kruskal-Wallis test. Stepwise multiple regression analysis was performed using needle angle parameters as dependent variables and the remaining parameters as independent variables. The significant predictors identified through the stepwise multiple regression analysis were further analyzed with principal component analysis (PCA) alongside the needle angle parameters. Statistical analyses, including RMANOVA, t-test, non-parametric tests, and stepwise multiple regression, were conducted using SPSS ver. 26 (IBM, Armonk, NY, USA), while PCA was performed using R ver. 3.6.1 (R Core Team, Vienna, Austria) with the ‘psych’ package for analysis and the ‘ggbiplot’ package for visualization.
3 Results
3.1 Growing conditions
During the experiment, the greenhouse conditions showed an average AH of 79.13 ± 13.88%, AT of 30.46 ± 4.72°C, and SR of 194.32 ± 233.92 W/m² (Figure 4). SM in the control remained stable at 29.48 ± 1.22%, whereas the drought treatment decreased to 13.97 ± 6.75%, showing a declining trend. ST showed no significant differences between the treatments, with 29.55 ± 4.06°C in the control and 29.45 ± 3.63°C in the drought treatment. Excluding rainfall on D5, SR and AH were higher in the morning compared to the evening, while AT exhibited the opposite trend.
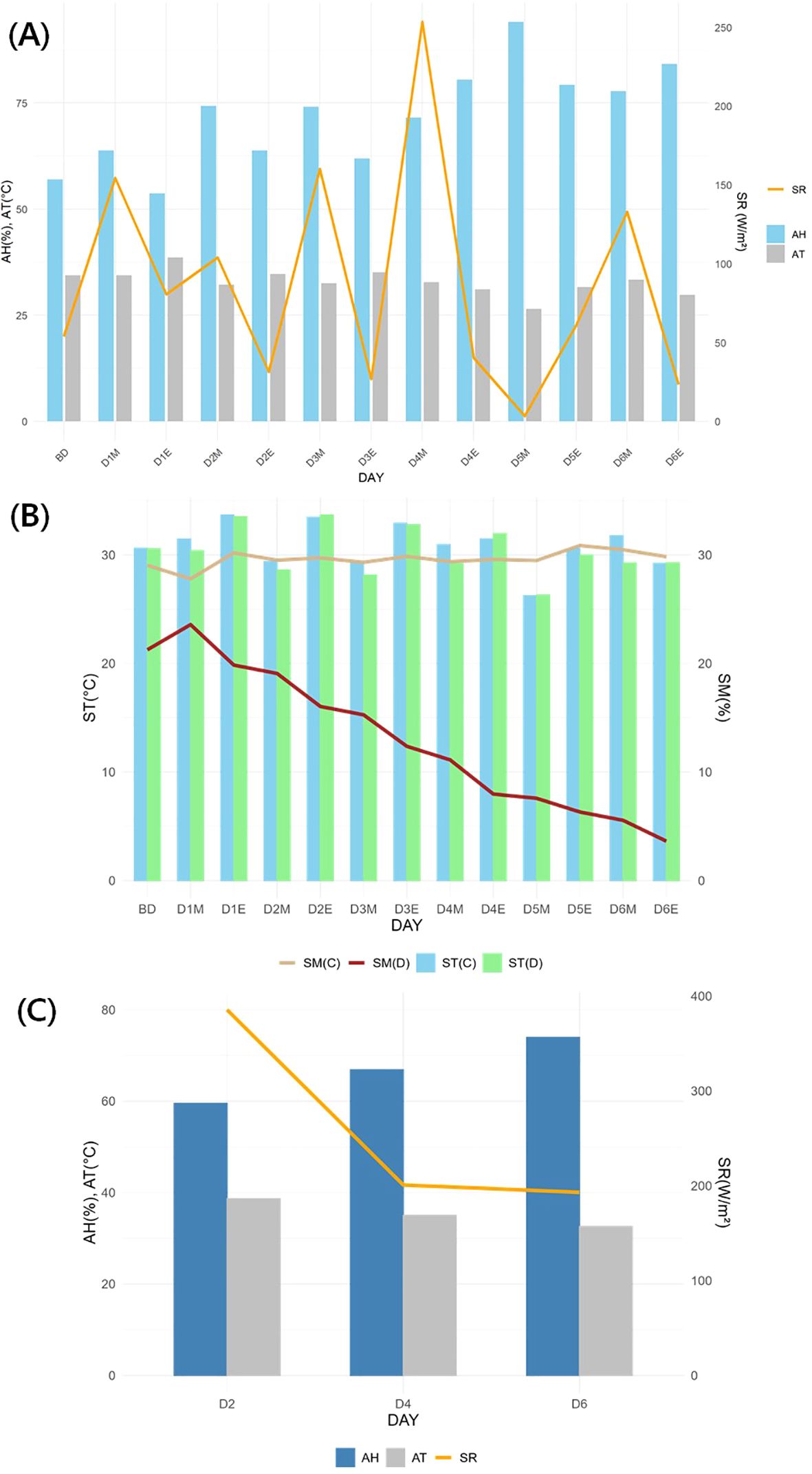
Figure 4. Greenhouse and soil environment during the experiment. (A): Temporal atmospheric environment; (B): Temporal soil environment; (C): Atmospheric environment (9:00 AM – 6:00 PM) during physiological traits measurement. AH, air humidity; AT, air temperature; SR, solar radiation; ST, soil temperature; SM, soil moisture; BD, before drought treatment; DOM, Day O of the experiment at 9:00 AM; DOE, Day O of the experiment at 6:00 PM; C, Control, D, Drought treatment.
3.2 Physiological traits
Chlorophyll fluorescence parameters that exhibited an interaction effect between day and treatment included Fm’, Fv’/Fm’, ΦII, ΦNO, and ΦNPQ (P < 0.01) (Table 1, Supplementary Table S1). No parameters exhibited a main effect of treatment alone, whereas Fv’/Fm’, ΦII, and ΦNPQ showed a main effect of day only. On D2, SR reached its highest level during the experimental period, suggesting that the control was exposed to slightly higher light and heat stress than the drought treatment (Figure 4C). On D2, significant differences between the two treatments were observed only in Fm’ (P < 0.01) and Fo’ (P < 0.001), and the control showed signs of recovery over time (Table 1, Supplementary Table S2). In contrast, on D6 in the drought treatment, a significant decrease in Fv’/Fm’ and a significant increase in ΦNPQ were observed over time, indicating drought stress. Meanwhile, SPAD showed no significant differences (P > 0.05). On the other hand, EC showed significant differences (P < 0.001) between the two treatments starting from day 2 due to root pressure and osmotic effects from direct irrigation. Needle temperature parameters tended to be more sensitive than chlorophyll fluorescence. Except for CWSI(Tl-Ta), an interaction effect between day and treatment, as well as the main effects of day and treatment, was identified. From D4, all needle temperature parameters showed significant differences (P < 0.01) between the two treatments. A clear drought stress over time was observed in LTD on D4, while VPD and CWSI(Tl) exhibited a pattern similar to chlorophyll fluorescence, appearing on the D6.
3.3 Needle angle
3.3.1 Apical vigor
The first signs of wilting were detected on D2E (evening of D2), occurring earlier than changes in chlorophyll fluorescence and needle temperature parameters (Table 2, Figure 5). During prolonged drought stress, 70% of the apical parts of the seedlings wilted in the evening but recovered by the following morning. However, these seedlings completely died within 1–2 days. In contrast, the remaining 30% of seedlings did not recover at the apical part and died without any signs of recovery.
3.3.2 RMANOVA
Unlike physiological traits, needle angle parameters showed significant differences (P < 0.001) in all interaction effects (day × time × treatment) (Supplementary Table S3). No stress response was observed in the control on D2, as indicated by the chlorophyll fluorescence results (Figure 6). The DE needle angle parameters (BD-M: 16.94 ± 43.63, PM-M: 15.75 ± 38.02, PM-M(ST): 16.15 ± 39.76) exhibited a faster response to drought stress than physiological traits, with significant differences appearing from D2E. PM-M showed a shift to a negative value (-13.47 ± 33.71) on D3M, indicating signs of recovery from wilting. When monitoring needle angle changes only in the morning, significant differences were observed starting from D4M (16.76 ± 38.33) (Figure 6C). These results suggest that needle angle can be used as a tool for detecting drought stress earlier than physiological traits.
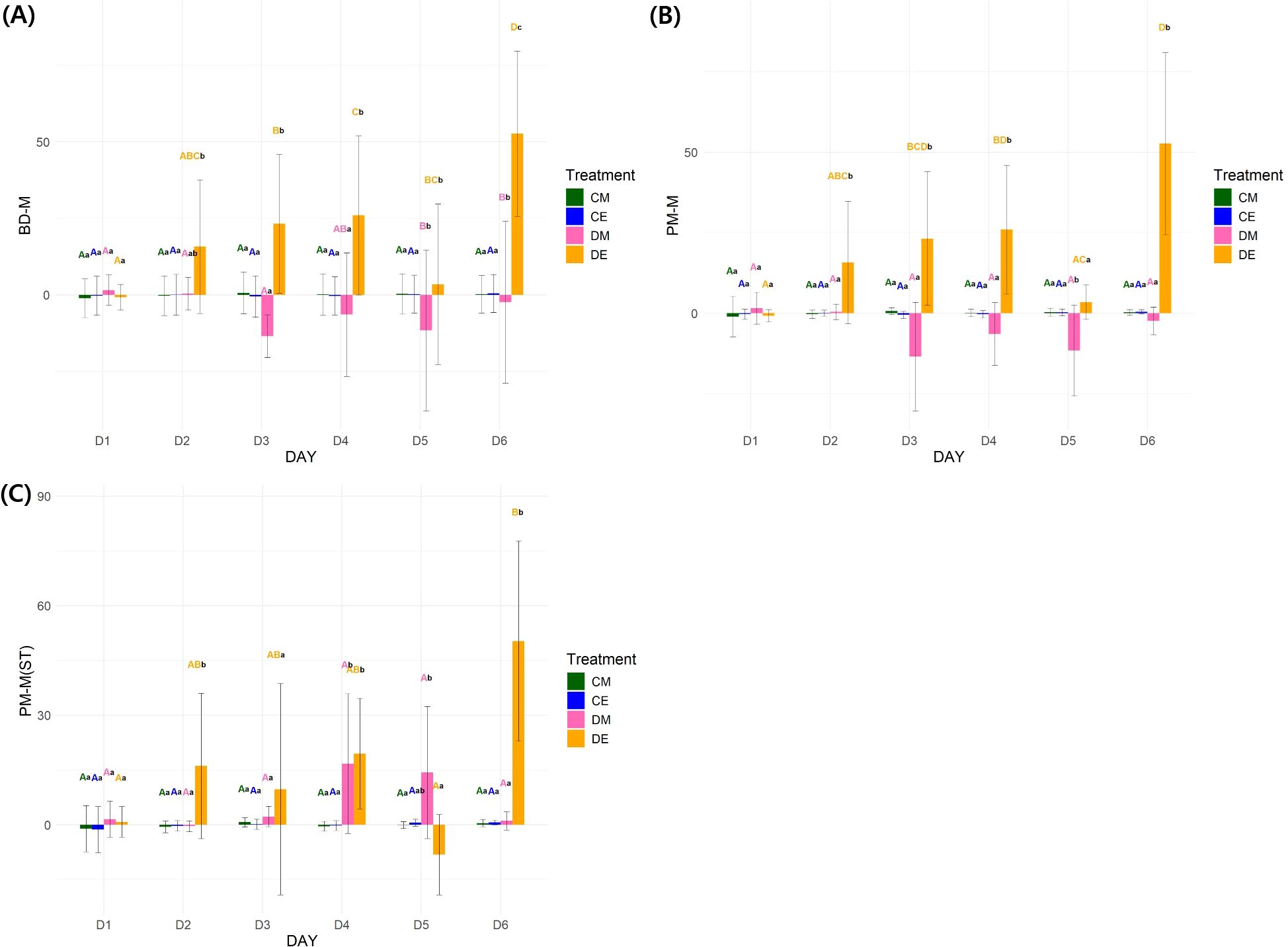
Figure 6. Statistical results of needle angle parameters. (A): Needle angle parameter (BD-M) changes over time; (B): Needle angle parameter (PM-M) changes over time; (C): Needle angle parameter (PM-M(ST)) changes over time. 3-way RMANOVA was conducted, followed by pairwise t-tests with Bonferroni correction for post-hoc analysis (n = 30) (P < 0.05). Uppercase letters: comparison among days within each treatment; Lowercase letters: comparison among treatments within each day. BD-M: needle angle before drought treatment – current needle angle; PM-M, previous needle angle – current needle angle; PM-M(ST), previous needle angle measured at the same time of day – current needle angle; CM, control morning; DM, drought treatment morning; CE, control evening; DE, drought treatment evening.
3.3.3 Multiple regression analysis
The STx(M) and BD-M(M) models demonstrated high explanatory power (R² > 0.5) compared to the evening needle angles (Tables 3, 4). The evening wilting-morning recovery cycle caused variability, leading to lower consistency across seedlings. Significant predictors for the STx(M) model included ΦNPQ, LTD, AH(P), and SR, while BD-M(M) model identified ΦNPQ, SR, AH(P), and CWSI(Tl) as significant predictors. Among the two models, ΦNPQ exhibited the highest standardized coefficient beta (> 0.5), indicating its dominant influence on needle angles. In contrast, SM was not identified as a significant predictor. Additionally, needle temperature parameters consistently emerged as significant predictors across all 8 models, highlighting their role in detecting early heat dissipation responses induced by drought stress. However, the Durbin-Watson values were close to 1 (indicating positive autocorrelation), suggesting a decrease in the independence of residuals, which could potentially affect the reliability and validity of the regression models.
3.3.4 PCA
On D2E, drought treatment formed a distinct group, with needle angle parameters showing the greatest influence (Figure 7). By D4, clear group separations were observed between control and drought treatments, regardless of time of day. On D6, the morning and evening groups of control were similar, whereas drought treatment groups showed distinct differences. These results indicate significant diurnal variations in apical vigor under continuous drought stress. The cumulative variance explained by PC1 and PC2 remained below 60%, suggesting complex and non-linear interactions among parameters.
3.4 Seedling level measurements
3.4.1 Non-parametric tests
In BD-M, Longest Path and Height showed significant differences (P < 0.05) between the control and drought treatments from D4 until the end of the experiment (Table 5). In DE, Center of Mass(y) was able to detect drought stress from D2. In PM-M, Convex Hull Area showed significant differences (P < 0.05) among the four treatments from D4 until the end of the experiment, with differences also observed between DM and DE (Supplementary Table S4). Center of Mass(y) exhibited significant differences (P < 0.05) among the four treatments from D3 to D5, and differences were also observed between DM and DE. Unlike BD-M, PM-M did not show significant differences (P > 0.05) over time. In PM-M(ST), significant differences (P < 0.05) among the four treatments and the drought treatment were observed at D3 or D4, but no persistent differences were observed afterward (Table 6). Center of Mass(y) and Ellipse Center(y) showed significant differences over time in CE and DE (P < 0.01). Notably, Center of Mass(y) in DE showed a continuous difference from D2 to the end of the experiment compared to D1. This suggests that the wilting of the apical part in the evening also affected seedling level parameters. Overall, distinct trends were observed depending on the needle angle parameter, and Center of Mass(y), which reflects changes in seedling height, was identified as the most suitable seedling level parameter for detecting drought stress.
3.4.2 Multiple regression analysis
Unlike the RMANOVA results, no significant predictors were identified in DE for constructing a regression model. In contrast, a regression model was successfully constructed in DM, but its R² values remained below 0.3, considerably lower than those of the needle angles (Table 7). SR was identified as a significant predictor in both the Height and Center of Mass(y) models. Likewise, SR also emerged as a significant predictor in the apical needle angle-based STx(M) and BD-M(M) models, highlighting its sensitive influence on needle angles under drought stress (Table 3).
4 Discussion
4.1 Physiological traits
Physiological measurements successfully identified drought stress non-destructively in seedlings before visible needle discoloration. The study results demonstrated that different physiological parameters vary in their effectiveness in detecting specific stress conditions. Chlorophyll fluorescence showed particularly sensitive to light and heat stress in the control, likely due to exposure to high light conditions. Over time, the drought treatment exhibited a stress response due to the absence of irrigation, whereas no such response was observed in the control.
Heat stress damages the protein structure of PSII complexes and directly affects the structural stability of the photosynthetic mechanism, leading to a sharp response in chlorophyll fluorescence (Allakhverdiev et al., 2008). Excessive light also induces the formation of reactive oxygen species (ROS), leading to PSII damage, as evidenced by higher Fm’ and Fo’ values in the control compared to the drought treatment and an increase in ΦNPQ in the drought treatment on D2 (Murata et al., 2007). Previous studies have reported Fv/Fm decreases under heat stress in various crop species, highlighting the sensitivity of chlorophyll fluorescence to heat stress (Guo et al., 2006; Carmo-Silva and Salvucci, 2012; Barboričová et al., 2022).
In contrast, mild drought stress has been reported to have minimal impact on Fv/Fm, suggesting that chlorophyll fluorescence parameters may exhibit a delayed response under short-term drought conditions when soil moisture is still available (Baker and Rosenqvist, 2004). This aligns with the study results, where the main effect was observed over time rather than between treatments, as drought stress did not appear immediately.
Needle temperature parameters showed no indication of light or heat stress in the control. Meanwhile, VPD calculated with air temperature as a factor was lower on D2 than on D4 in the drought treatment, indicating the influence of high temperatures that day. During drought conditions, plants regulate stomatal activity to maintain internal water levels, making them relatively less sensitive to light and heat stress (Chaves et al., 2009; Martínez-Vilalta and Garcia-Forner, 2017). The structural traits of L. kaempferi needles, such as stomatal area and density, reduce their efficiency in heat dissipation through transpiration compared to broadleaf species. This is consistent with LTD showing a rapid stress response on D4. Therefore, thermal imaging holds great potential for detecting drought stress and improving irrigation management in L. kaempferi nurseries.
EC also emerged as the earliest physiological response to drought stress, consistent with water potential and osmotic effects reported in previous studies (Yang et al., 2016). Park et al. (2019) noted that dynamic environmental conditions, such as cloudy days, were reflected in EC measurements. This suggests that EC could be a valuable tool for real-time monitoring of drought stress and other unusual cultivation conditions. However, direct irrigation in soil may have led to over- or underestimation of drought stress, highlighting the need for further studies using sprinkler irrigation to enhance accuracy.
4.2 Comparative analysis with needle angle-based phenotypes
Image-based measurements of apical vigor allowed earlier drought stress detection compared to physiological traits. The apical part, positioned at the seedling tip, is the last to receive water through xylem transport and is structurally and physiologically more sensitive to water deficits. Apical needles are thinner, more vulnerable to turgor loss, and exposed to direct sunlight, which accelerates wilting under drought stress (Gebauer et al., 2015; Nadal et al., 2020; Jahan et al., 2023). Stepwise multiple regression analysis confirmed that SR was the most influential factor for both apical needle angles and seedling level parameters. Although the study results did not show an immediate stress response to strong SR in needle angles, its impact was evident in the needle angle RMANOVA results through the significant interaction between day and treatment. Changes in needle angles reflect physiological adaptation strategies to optimize photosynthesis while minimizing heat stress (Nilsen and Forseth, 2018). Under drought conditions, heat and light stress synergistically exacerbate damage, leading to rapid needle wilting in L. kaempferi (Kim et al., 2022). This aligns with findings suggesting that shading treatments can mitigate drought-induced damage and that this species is more susceptible to high temperatures than drought (Kim et al., 2024). Thus, when drought stress is induced by the absence of irrigation, SR becomes a key factor in monitoring needle angle changes, particularly when drought period (day and time) is considered as a variable. This suggests its importance in determining the duration of needle angle recovery and maintaining stability, as well as optimizing irrigation timing.
4.3 Applicability of image analysis methods at seedling level
As mentioned in the introduction, while real-time leaf angle analysis systems have been developed, they are often species-specific and require further optimization for L. kaempferi. The continuous growth pattern of needles along the stem poses challenges in distinguishing the apical part through imaging. In particular, the young stems of L. kaempferi, being less lignified, may show structural irregularities that further complicate analysis. Therefore, seedling level image analysis improved practicality by providing objective parameters for seedling morphology that cannot be measured manually. Furthermore, expanding the analysis from the seedling level to the container level could allow for image-based assessment of seedlings within a single area, enabling its application for drought stress diagnosis.
The results of this study showed that changes in the height (y-axis) of L. kaempferi significantly affect the canopy shape in images. This suggests a broad applicability for simultaneously monitoring growth parameters such as height growth. Additionally, since L. kaempferi is sensitive to heat, integrating thermal imaging could help identify irrigation blind spots in greenhouse environments, facilitating the development of a dynamic and efficient irrigation management system. Such imaging systems are expected to effectively analyze seedling growth and physiological status, contributing to the production of high-quality L. kaempferi.
However, measuring with 2D-RGB images alone may lack precision, highlighting the need to explore cost-effective solutions to address this limitation. Color correction issues may arise due to variations in light intensity, potentially leading to inconsistencies in color recognition even under the same stress conditions. Therefore, it is necessary to refine the standard light source calibration for color correction or develop analytical images in a way that minimizes errors. As a method to enhance camera performance, Liu et al. (2021) introduced a real-time, precise stress measurement technique at the individual plant level using RGB-D (depth) imaging. This approach was used to assess plant height and aboveground biomass in Toona sinensis seedlings subjected to drought stress.
The leaf projection function (G function) have been introduced to evaluate the spatial arrangement of leaves, analyze light environments, and model the structural characteristics of plants, enabling a detailed analysis of plant-light interactions (Pisek et al., 2011; Liu et al., 2021). Integrating the G function into a smart nursery system would enable the adjustment of appropriate spacing between seedlings and leaf arrangement, enhancing light utilization and facilitating the design of an optimal tree structure.
Meanwhile, in this study, although the apical part did not wither, partial canopy dieback or leaf abscission was observed. A rapid decrease in needle has been reported as a key factor accelerating the forest dieback process (Sangüesa-Barreda et al., 2023). The needle trace method (NTM) is a technique that quantifies the dynamics of needle production by analyzing cross-sections or longitudinal sections of the stem based on traces of needle attachment (Pouttu and Dobbertin, 2000; Drenkhan et al., 2006; Sangüesa-Barreda et al., 2023). Similarly, an image analysis technique for detecting partial canopy dieback or leaf abscission could serve as a drought tolerance assessment parameter for the production of high-quality seedlings. Furthermore, this technique is expected to function as a critical monitoring tool for adjusting the intensity of irrigation and priming treatments.
By integrating and optimizing various methods, early detection of drought stress at the seedling level can be achieved while also facilitating diverse tree physiological analyses, supporting the cultivation of high-quality seedlings.
4.4 Limitations and future studies
In this study, while the correlation between physiological traits and needle angles was analyzed, difficulties arose in perfectly synchronizing physiological data and needle angle measurements at the same seedling and time point. Addressing this issue will be essential for achieving more precise analyses in the future. Additionally, direct irrigation was applied to the control soil instead of using sprinklers, which excluded factors such as environmental conditions or dew formation on the needle that could influence needle angles. For seedling level analysis, an adequate sample size of surviving seedlings is necessary to ensure sufficient statistical power for parametric tests. Therefore, future studies should aim to develop experiments in large-scale nursery settings to improve practical applicability. Moreover, the period during which apical vigor recovers and seedling survival stabilizes may vary depending on weather conditions during the growing season or individual plant characteristics. This highlights the need for additional research to determine whether growth is sustained after apical wilting and recovery at different stages of the growing season. If growth continues, it could facilitate more efficient irrigation strategies while also leveraging the priming effects induced by stress treatments, further optimizing seedling resilience and resource management.
5 Conclusion
This study demonstrated the potential of needle angle-based phenotypic measurements for the early detection of drought stress in L. kaempferi seedlings. Overall, tree physiological traits detected drought stress on D6. Chlorophyll fluorescence effectively detected light and heat stress, whereas needle temperature parameters were more sensitive to drought stress. Needle angles provided the earliest indicators of drought stress, with significant changes observed by D2, even before physiological symptoms were evident. Apical needle wilting occurred in the evening and recovered the following morning, with most seedlings showing mortality within 1–2 days. Seedling level image analysis validated these findings, Center of Mass(y) as significant parameter for drought stress monitoring. Combining apical and seedling level parameters enhances the applicability of image-based methods for stress detection. These methods can be further integrated with thermal imaging to optimize irrigation systems and monitor seedling growth dynamically. Future advancements in imaging techniques are expected to enhance precision and broaden the scope of phenotypic measurements in nursery systems. This study lays the groundwork for developing efficient tools for monitoring drought stress, combining physiological and phenotypic approaches, to support the production of resilient, high-quality L. kaempferi seedlings.
Data availability statement
The original contributions presented in the study are included in the article/Supplementary Material. Further inquiries can be directed to the corresponding authors.
Author contributions
UJ: Conceptualization, Data curation, Formal analysis, Investigation, Methodology, Software, Supervision, Validation, Visualization, Writing – original draft, Writing – review & editing. DK: Data curation, Formal analysis, Investigation, Software, Validation, Visualization, Writing – review & editing. SK: Formal analysis, Investigation, Writing – review & editing. SHH: Funding acquisition, Resources, Writing – review & editing. EJC: Conceptualization, Project administration, Supervision, Writing – review & editing.
Funding
The author(s) declare that financial support was received for the research and/or publication of this article. This study was funded by the National Institute of Forest Science, grant number SC0300-2023-01-2024.
Conflict of interest
The authors declare that the research was conducted in the absence of any commercial or financial relationships that could be construed as a potential conflict of interest.
Generative AI statement
The author(s) declare that no Generative AI was used in the creation of this manuscript.
Publisher’s note
All claims expressed in this article are solely those of the authors and do not necessarily represent those of their affiliated organizations, or those of the publisher, the editors and the reviewers. Any product that may be evaluated in this article, or claim that may be made by its manufacturer, is not guaranteed or endorsed by the publisher.
Supplementary material
The Supplementary Material for this article can be found online at: https://www.frontiersin.org/articles/10.3389/fpls.2025.1550748/full#supplementary-material
References
Abdallah, M. B., Methenni, K., Nouairi, I., Zarrouk, M., Youssef, N. B. (2017). Drought priming improves subsequent more severe drought in a drought-sensitive cultivar of olive cv. Chétoui. Sci. Hortic. 221, 43–52. doi: 10.1016/j.scienta.2017.04.021
Abid, M., Tian, Z., Ata-Ul-Karim, S. T., Liu, Y., Cui, Y., Zahoor, R., et al. (2016). Improved tolerance to post-anthesis drought stress by pre-drought priming at vegetative stages in drought-tolerant and-sensitive wheat cultivars. Plant Physiol. Biochem. 106, 218–227. doi: 10.1016/j.plaphy.2016.05.003
Allakhverdiev, S. I., Kreslavski, V. D., Klimov, V. V., Los, D. A., Carpentier, R., Mohanty, P. (2008). Heat stress: an overview of molecular responses in photosynthesis. Photosynth. Res. 98, 541–550. doi: 10.1007/s11120-008-9331-0
Allen, K. S., Harper, R. W., Bayer, A., Brazee, N. J. (2017). A review of nursery production systems and their influence on urban tree survival. Urban For. Urban Green 21, 183–191. doi: 10.1016/j.ufug.2016.12.002
Baker, N. R., Rosenqvist, E. (2004). Applications of chlorophyll fluorescence can improve crop production strategies: an examination of future possibilities. J. Exp. Bot. 55, 1607–1621. doi: 10.1093/jxb/erh196
Barboričová, M., Filaček, A., Vysoká, D. M., Gašparovič, K., Živčák, M., Brestič, M. (2022). Sensitivity of fast chlorophyll fluorescence parameters to combined heat and drought stress in wheat genotypes. Plant Soil Environ. 68, 309–316. doi: 10.17221/87/2022-PSE
Bhusal, N., Lee, M., Han, A. R., Han, A., Kim, H. S. (2020). Responses to drought stress in Prunus sargentii and Larix kaempferi seedlings using morphological and physiological parameters. For. Ecol. Manage. 465, 118099. doi: 10.1016/j.foreco.2020.118099
Bhusal, N., Lee, M., Lee, H., Adhikari, A., Han, A. R., Han, A., et al. (2021). Evaluation of morphological, physiological, and biochemical traits for assessing drought resistance in eleven tree species. Sci. Total Environ. 779, 146466. doi: 10.1016/j.scitotenv.2021.146466
Carmo-Silva, A. E., Salvucci, M. E. (2012). The temperature response of CO2 assimilation, photochemical activities and Rubisco activation in Camelina sativa, a potential bioenergy crop with limited capacity for acclimation to heat stress. Planta 236, 1433–1445. doi: 10.1007/s00425-012-1691-1
Chandel, N. S., Rajwade, Y. A., Dubey, K., Chandel, A. K., Subeesh, A., Tiwari, M. K. (2022). Water stress identification of winter wheat crop with state-of-the-art AI techniques and high-resolution thermal-RGB imagery. Plants 11, 3344. doi: 10.3390/plants11233344
Chaves, M. M., Flexas, J., Pinheiro, C. (2009). Photosynthesis under drought and salt stress: regulation mechanisms from whole plant to cell. Ann. Bot. 103, 551–560. doi: 10.1093/aob/mcn125
Choi, S. H., Lee, S. H., Shin, Y. J., Cho, W., Lee, M. H., Kim, J. H. (2021). Ecological structure of larix kaempferi in national park. Korean J. Environ. Ecol. 35, 609–620. doi: 10.13047/KJEE.2021.35.6.609
Cozzolino, D. (2023). Advantages and limitations of using near infrared spectroscopy in plant phenomics applications. Comput. Electron. Agric. 212, 108078. doi: 10.1016/j.compag.2023.108078
De Swaef, T., Maes, W. H., Aper, J., Baert, J., Cougnon, M., Reheul, D., et al. (2021). Applying RGB-and thermal-based vegetation indices from UAVs for high-throughput field phenotyping of drought tolerance in forage grasses. Remote Sens. 13, 147. doi: 10.3390/rs13010147
Drenkhan, R., Kurkela, T., Hanso, M. (2006). The relationship between the needle age and the growth rate in Scots pine (Pinus sylvestris): a retrospective analysis by needle trace method (NTM). Eur. J. For. Res. 125, 397–405. doi: 10.1007/s10342-006-0131-9
Ehleringer, J. R., Comstock, J. (1987). “Leaf absorptance and leaf angle: mechanisms for stress avoidance,” in Plant response to stress: functional analysis in Mediterranean ecosystems. Eds. Tenhunen, J. D., Catarino, F. M., Lange, O. L., Oechel, W. C. (Springer, Heidelberg, Berlin), 55–76. doi: 10.1007/978-3-642-70868-8
Fernández, M. E., Gyenge, J. E., Varela, S., de Urquiza, M. (2014). Effects of the time of drought occurrence within the growing season on growth and survival of Pinus ponderosa seedlings. Trees 28, 745–756. doi: 10.1007/s00468-014-0986-1
Forsström, P. R., Hovi, A., Ghielmetti, G., Schaepman, M. E., Rautiainen, M. (2021). Multi-angular reflectance spectra of small single trees. Remote Sens. Environ. 255, 112302. doi: 10.1016/j.rse.2021.112302
Galvez, D. A., Landhäusser, S. M., Tyree, M. T. (2011). Root carbon reserve dynamics in aspen seedlings: does simulated drought induce reserve limitation? Tree Physiol. 31, 250–257. doi: 10.1093/treephys/tpr012
Gardner, B. R., Nielsen, D. C., Shock, C. C. (1992). Infrared thermometry and the crop water stress index. I. History, theory, and baselines. J. Prod. Agric. 5, 462–466. doi: 10.2134/jpa1992.0462
Gebauer, R., Volařík, D., Urban, J., Børja, I., Nagy, N. E., Eldhuset, T. D., et al. (2015). Effects of prolonged drought on the anatomy of sun and shade needles in young Norway spruce trees. Ecol. Evol. 5, 4989–4998. doi: 10.1002/ece3.1766
Gehan, M. A., Fahlgren, N., Abbasi, A., Berry, J. C., Callen, S. T., Chavez, L., et al. (2017). PlantCV v2: Image analysis software for high-throughput plant phenotyping. PeerJ 5, e4088. doi: 10.7717/peerj.4088
Geldhof, B., Pattyn, J., Eyland, D., Carpentier, S., Van de Poel, B. (2021). A digital sensor to measure real-time leaf movements and detect abiotic stress in plants. Plant Physiol. 187, 1131–1148. doi: 10.1093/plphys/kiab407
Grossiord, C., Buckley, T. N., Cernusak, L. A., Novick, K. A., Poulter, B., Siegwolf, R. T., et al. (2020). Plant responses to rising vapor pressure deficit. New Phytol. 226, 1550–1566. doi: 10.1111/nph.16485
Grossnickle, S. C. (2012). Why seedlings survive: influence of plant attributes. New For. 43, 711–738. doi: 10.1007/s11056-012-9336-6
Guo, Y. P., Zhou, H. F., Zhang, L. C. (2006). Photosynthetic characteristics and protective mechanisms against photooxidation during high temperature stress in two citrus species. Sci. Hortic. 108, 260–267. doi: 10.1016/j.scienta.2006.01.029
Jahan, E., Sharwood, R. E., Tissue, D. T. (2023). Effects of leaf age during drought and recovery on photosynthesis, mesophyll conductance and leaf anatomy in wheat leaves. Front. Plant Sci. 14. doi: 10.3389/fpls.2023.1091418
Ji, X., Gao, Q., Zhuang, Z., Chang, F., Peng, Y. (2024). WGCNA analysis of the effect of exogenous BR on leaf angle of maize mutant lpa1. Sci. Rep. 14, 5238. doi: 10.1038/s41598-024-55835-7
Jiang, S., Wu, X., Wang, Q., Pei, Z., Wang, Y., Jin, J., et al. (2024). Auto-LIA: The automated vision-based leaf inclination angle measurement system improves monitoring of plant physiology. Plant Phenomics 6, 0245. doi: 10.1111/2041-210X.13968
Kaliniewicz, Z., Markowski, P., Anders, A., Rawa, T., Liszewski, A., Fura, S. (2012). Correlations between the germination capacity and selected attributes of European larch seeds (Larix decidua Mill.). Techn. Sc. 15, 229–242. Available at: https://www.researchgate.net/publication/266851975_CORRELATIONS_BETWEEN_THE_GERMINATION_CAPACITY_AND_SELECTED_ATTRIBUTES_OF_EUROPEAN_LARCH_SEEDS_LARIX_DECIDUA_MILL.
Kattenborn, T., Richter, R., Guimarães-Steinicke, C., Feilhauer, H., Wirth, C. (2022). AngleCam: Predicting the temporal variation of leaf angle distributions from image series with deep learning. Methods Ecol. Evol. 13, 2531–2545. doi: 10.1111/2041-210X.13968
Kenchanmane Raju, S. K., Adkins, M., Enersen, A., Santana de Carvalho, D., Studer, A. J., Ganapathysubramanian, B., et al. (2020). Leaf Angle eXtractor: A high-throughput image processing framework for leaf angle measurements in maize and sorghum. Appl. Plant Sci. 8, e11385. doi: 10.1002/aps3.11385
Khurana, E., Singh, J. S. (2004). Germination and seedling growth of five tree species from tropical dry forest in relation to water stress: impact of seed size. J. Trop. Ecol. 20, 385–396. doi: 10.1017/S026646740400135X
Kim, S. M., An, J. H., Lim, Y. K., Pee, J. H., Kim, G. S., Lee, H. Y., et al. (2013). Ecological changes of the Larix kaempferi plantations and the restoration effects confirmed from the results. Korean J. Ecol. Environ. 46, 241–250. doi: 10.11614/KSL.2013.46.2.241
Kim, G. J., Jo, H., Cho, M. S., Noh, N. J., Han, S. H., Khamzina, A., et al. (2024). Photosynthetic responses of Larix kaempferi and Pinus densiflora seedlings are affected by summer extreme heat rather than by extreme precipitation. Sci. Rep. 14, 5250. doi: 10.1038/s41598-024-56120-3
Kim, H., Jo, H., Kim, G. J., Kim, H. S., Son, Y. (2022). Effects of spring warming and drought events on the autumn growth of larix kaempferi seedlings. Water 14, 1962. doi: 10.3390/w14121962
Kumar, J., Pratap, A., Kumar, S. (2015). “Plant phenomics: an overview,” in Phenomics in Crop Plants: Trends, Options and Limitations. Eds. Kumar, J., Pratap, A., Kumar, S. (Springer, New Delhi), 1–10. doi: 10.1007/978-81-322-2226-2_1
Liu, W., Li, Y., Liu, J., Jiang, J. (2021). Estimation of plant height and aboveground biomass of Toona sinensis under drought stress using RGB-D imaging. Forests 12, 1747. doi: 10.3390/f12121747
Liu, L., Zhao, L., Liu, Y., Zhu, Y., Chen, S., Yang, L. (2024). Transcription factor OsWRKY72 controls rice leaf angle by regulating LAZY1-mediated shoot gravitropism. Plant Physiol. 195, 1586–1600. doi: 10.1093/plphys/kiae159
Martínez-Vilalta, J., Garcia-Forner, N. (2017). Water potential regulation, stomatal behaviour and hydraulic transport under drought: deconstructing the iso/anisohydric concept. Plant Cell Environ. 40, 962–976. doi: 10.1111/pce.12846
Murata, N., Takahashi, S., Nishiyama, Y., Allakhverdiev, S. I. (2007). Photoinhibition of photosystem II under environmental stress. Biochim. Biophys. Acta - Bioenerg. 1767, 414–421. doi: 10.1016/j.bbabio.2006.11.019
Nadal, M., Roig-Oliver, M., Bota, J., Flexas, J. (2020). Leaf age-dependent elastic adjustment and photosynthetic performance under drought stress in Arbutus unedo seedlings. Flora 271, 151662. doi: 10.1016/j.flora.2020.151662
Nagaike, T., Hayashi, A., Abe, M., Arai, N. (2003). Differences in plant species diversity in Larix kaempferi plantations of different ages in central Japan. For. Ecol. Manage. 183, 177–193. doi: 10.1016/S0378-1127(03)00105-1
Naqvi, R. Z., Farooq, M., Zahid, R., Imran, I., Mahmood, M. A., Awan, M. J. A., et al. (2024). “Recent advances in plant phenomics and speed breeding for climate-smart agriculture,” in Plant Speed Breeding and High-throughput Technologies. Ed. Chen, J. T. (CRC Press, Boca Raton, FL), 82–103. doi: 10.1201/b23372-5
Nilsen, E. T., Forseth, I. N. (2018). “The role of leaf movements for optimizing photosynthesis in relation to environmental variation,” in The leaf: a platform for performing photosynthesis. Eds. Adams, III, W. W., Terashima, I. (Springer, Cham, Switzerland), 401–423. doi: 10.1007/978-3-319-93594-2_14
Oskam, L., Snoek, B. L., Pantazopoulou, C. K., van Veen, H., Matton, S. E., Dijkhuizen, R., et al. (2024). A low-cost open-source imaging platform reveals spatiotemporal insight into leaf elongation and movement. Plant Physiol. 195, 1866–1879. doi: 10.1093/plphys/kiae097
Park, H. J., Park, J. H., Park, K. S., Son, J. E. (2019). Evaluating plant stress conditions in paprika by comparing internal electrical conductivity, photosynthetic response, and sap flow. Hortic. Environ. Biotechnol. 60, 41–48. doi: 10.1007/s13580-018-0105-0
Pasala, R., Pandey, B. B. (2020). Plant phenomics: High-throughput technology for accelerating genomics. J. Biosci. 45, 111. doi: 10.1007/s12038-020-00083-w
Perez-Sanz, F., Navarro, P. J., Egea-Cortines, M. (2017). Plant phenomics: An overview of image acquisition technologies and image data analysis algorithms. Gigascience 6, gix092. doi: 10.1093/gigascience/gix092
Pisek, J., Borysenko, O., Janoutová, R., Homolová, L. (2023). Estimation of coniferous shoot structure by high precision blue light 3D photogrammetry scanning. Remote Sens. Environ. 291, 113568. doi: 10.1016/j.rse.2023.113568
Pisek, J., Ryu, Y., Alikas, K. (2011). Estimating leaf inclination and G-function from leveled digital camera photography in broadleaf canopies. Trees 25, 919–924. doi: 10.1007/s00468-011-0566-6
Pouttu, A., Dobbertin, M. (2000). Needle-retention and density patterns in Pinus sylvestris in the Rhone Valley of Switzerland: comparing results of the needle-trace method with visual defoliation assessments. Can. J. For. Res. 30, 1973–1982. doi: 10.1139/x00-12
Ru, C., Hu, X., Chen, D., Wang, W., Song, T. (2022). Heat and drought priming induce tolerance to subsequent heat and drought stress by regulating leaf photosynthesis, root morphology, and antioxidant defense in maize seedlings. Environ. Exp. Bot. 202, 105010. doi: 10.1016/j.envexpbot.2022.105010
Sangüesa-Barreda, G., Gazol, A., Camarero, J. J. (2023). Drops in needle production are early-warning signals of drought-triggered dieback in Scots pine. Trees 37, 1137–1151. doi: 10.1007/s00468-023-02412-6
Sarris, D., Christodoulakis, D. (2024). Topographic and climatic effects on Pinus halepensis sl growth at its drought tolerance margins under climatic change. J. For. Res. 35, 102. doi: 10.1007/s11676-024-01755-1
Sintaha, M., Man, C. K., Yung, W. S., Duan, S., Li, M. W., Lam, H. M. (2022). Drought stress priming improved the drought tolerance of soybean. Plants 11, 2954. doi: 10.3390/plants11212954
South, D. B., Starkey, T. E., Lyons, A. (2023). Why healthy pine seedlings die after they leave the nursery. Forests 14, 645. doi: 10.3390/f14030645
Stull, R. B. (2015). Practical meteorology: an algebra-based survey of atmospheric science (Vancouver: University of British Columbia). doi: 10.14288/1.0300441
Su, J., Coombes, M., Liu, C., Zhu, Y., Song, X., Fang, S., et al. (2020). Machine learning-based crop drought mapping system by UAV remote sensing RGB imagery. Unmanned Syst. 8, 71–83. doi: 10.1142/S2301385020500053
Tankari, M., Wang, C., Ma, H., Li, X., Li, L., Soothar, R. K., et al (2021). Drought priming improved water status, photosynthesis and water productivity of cowpea during post-anthesis drought stress. Agric. Water Manag. 245, 106565. doi: 10.1016/j.agwat.2020.106565
Tardieu, F., Cabrera-Bosquet, L., Pridmore, T., Bennett, M. (2017). Plant phenomics, from sensors to knowledge. Curr. Biol. 27, R770–R783. doi: 10.1016/j.cub.2017.05.055
Trenberth, K. E., Dai, A., van der Schrier, G., Jones, P. D., Barichivich, J., Briffa, K. R., et al. (2014). Global warming and changes in drought. Nat. Clim. Change. 4, 17–22. doi: 10.1038/nclimate2067
Wu, C., Chen, D., Shen, J., Sun, X., Zhang, S. (2021). Estimating the distribution and productivity characters of Larix kaempferi in response to climate change. J. Environ. Manage. 280, 111633. doi: 10.1016/j.jenvman.2020.111633
Wu, Y., Zhang, Y., Zhuang, Z., Ji, X., Bian, J., Xian, J., et al. (2024). Phenotypic investigation and RNA-seq of KN1 involved in leaf angle formation in maize (Zea mays L.). Int. J. Mol. Sci. 25, 3180. doi: 10.3390/ijms25063180
Yang, X., Li, R., Jablonski, A., Stovall, A., Kim, J., Yi, K., et al. (2023). Leaf angle as a leaf and canopy trait: Rejuvenating its role in ecology with new technology. Ecol. Lett. 26, 1005–1020. doi: 10.1111/ele.14215
Yang, L., Teskey, R. O., Sun, H. (2016). The effects of rehydration time on pressure-volume curves of Larix gmelinii and Fraxinus mandshurica in Northeast China. For. Sci. 62, 385–391. doi: 10.5849/forsci.15-155
Yasin, S., Zavala-García, F., Niño-Medina, G., Rodríguez-Salinas, P. A., Gutiérrez-Diez, A., Sinagawa-García, S. R., et al. (2024). Morphological and Physiological Response of Maize (Zea mays L.) to Drought Stress during Reproductive Stage. Agronomy 14, 1718. doi: 10.3390/agronomy14081718
Yavas, I., Jamal, M. A., Ul Din, K., Ali, S., Hussain, S., Farooq, M. (2024). Drought-induced changes in leaf morphology and anatomy: overview, implications and perspectives. Pol. J. Environ. Stud. 33, 1517–1530. doi: 10.15244/pjoes/174476
Zhang, L., Niu, Y., Zhang, H., Han, W., Li, G., Tang, J., et al. (2019a). Maize canopy temperature extracted from UAV thermal and RGB imagery and its application in water stress monitoring. Front. Plant Sci. 10. doi: 10.3389/fpls.2019.01270
Zhang, Y., Yu, T., Ma, W., Tian, C., Sha, Z., Li, J. (2019b). Morphological and physiological response of Acer catalpifolium Rehd. Seedlings to water and light stresses. Glob. Ecol. Conserv. 19, e00660. doi: 10.1016/j.gecco.2019.e00660
Keywords: Larix kaempferi, forest nursery, drought stress monitoring, early detection, leaf angle, phenomics
Citation: Jeong U, Kim D, Kim S, Han SH and Cheong EJ (2025) Needle angle dynamics as a rapid indicator of drought stress in Larix kaempferi (Lamb.) Carrière: advancing non-destructive imaging techniques for resilient seedling production. Front. Plant Sci. 16:1550748. doi: 10.3389/fpls.2025.1550748
Received: 24 December 2024; Accepted: 10 April 2025;
Published: 12 May 2025.
Edited by:
Silvia Traversari, National Research Council (CNR), ItalyReviewed by:
Sonia Cacini, Council for Agricultural and Economics Research (CREA), ItalyMansour Ghorbanpour, Arak University, Iran
Copyright © 2025 Jeong, Kim, Kim, Han and Cheong. This is an open-access article distributed under the terms of the Creative Commons Attribution License (CC BY). The use, distribution or reproduction in other forums is permitted, provided the original author(s) and the copyright owner(s) are credited and that the original publication in this journal is cited, in accordance with accepted academic practice. No use, distribution or reproduction is permitted which does not comply with these terms.
*Correspondence: Eun Ju Cheong, ZWpjaGVvbmdAa2FuZ3dvbi5hYy5rcg==; Seung Hyun Han, Zm9yZXN0aHNoQGtvcmVhLmty
†ORCID: Ukhan Jeong, https://orcid.org/0000-0002-6861-4207
Dohee Kim, orcid.org/0009-0007-1804-510X
Sohyun Kim, orcid.org/0009-0002-1763-0749
Seung Hyun Han, orcid.org/0000-0002-8180-2915
Eun Ju Cheong, orcid.org/0000-0002-2576-5435