- Inner Mongolia Key Laboratory of Grassland Ecology and the Candidate State Laboratory of Ministry of Science and Technology, School of Ecology and Environment, Inner Mongolia University, Hohhot, China
Thymus mongolicus is a common medicinal and edible plant in grasslands of northern China. However, previous studies were limited to the production and utilization value of T. mongolicus, and only few studies have paid attention to the changes in the functional traits of wild T. mongolicus on a large scale. Therefore, this study examined 145 T. mongolicus specimens collected from northern China. By measuring 10 functional traits, including leaf length, leaf width, and leaf area, we analyzed the interplay between functional traits as a response to changes in environmental factors. Significant differences were observed between the eastern and western populations of T. mongolicus. Functional traits exhibited pronounced latitudinal and longitudinal gradients. With increasing latitude and longitude, T. mongolicus tended to change its functional traits to adapt to environmental changes. In high-temperature and high-rainfall environments, T. mongolicus developed larger leaf areas and longer leaves. Random forest analysis identified temperature within the latitudinal and longitudinal context as the primary driver of functional trait variation. Furthermore, interdependencies among functional traits were evident, with specific traits—such as stem length, leaf width, and leaf area—emerging as central to the adaptive process. These findings elucidate the mechanisms and key factors underlying the functional trait adaptation of T. mongolicus, providing critical insights for the breeding of region-specific varieties in China.
1 Introduction
Functional traits are the most intuitive way to study the response of plants to environmental changes and have always been an important research direction in ecology (Cornelissen et al., 2003; Reich et al., 2003). Over the past two decades, research on functional traits has spanned multiple levels—ranging from physiological to organ-level processes, from individual plants to ecosystems, and from aboveground to belowground interactions—extending into diverse ecological disciplines (Sobral, 2021). The main research areas are as follows: (1) the internal relationships and trade-offs among functional traits (Jin et al., 2024; Zhang et al., 2024); (2) the distribution patterns and variation in traits along environmental gradients (Hulshof et al., 2013; Wieczynski et al., 2019); (3) responses of traits to disturbances such as drought, fire, and grazing (Kerr et al., 2023; Wang et al., 2023); (4) studies on functional diversity (Díaz and Cabido, 2001); and (5) the mechanisms underlying species coexistence (Mello et al., 2020). The leaf traits of woody plants are closely related to changes in environmental factors such as light and precipitation. In temperate regions, woody plants usually have larger leaves and a higher specific leaf area (LA) to improve the efficiency of photosynthesis in a longer growing season. In arid or low-light environments, these plants tend to exhibit smaller leaves and a lower specific LA to reduce water evaporation and enhance drought resistance. The regulation of this trait enables woody plants to effectively respond to environmental changes and maintain survival and stability under diverse ecological conditions (Vilà et al., 2015). In addition to woody plants, bryophytes and herbaceous plants also showed similar regular changes (Candeias and Fraterrigo, 2020; Souza et al., 2020).
Leaves represent the most evolutionarily plastic organ in plants, playing vital roles in photosynthesis, respiration, and evapotranspiration—key processes for maintaining plant life (Koyama et al., 2012). Leaf size reflects a plant’s light-capture efficiency, carbon acquisition capacity, and environmental adaptations, revealing a trade-off between carbon assimilation and water use (Onoda et al., 2012). This trade-off is central to regulating leaf temperature and photosynthetic efficiency under varying climatic conditions, forming a cornerstone of plant ecological strategies. Leaf morphological characteristics serve as intuitive indicators of plant adaptability to specific habitats (Henn et al., 2018). The dynamic changes in leaf shape reflect how plants respond to environmental challenges, showcasing fundamental ecological behaviors and strategies (Chitwood and Sinha, 2016; Fritz et al., 2018). For instance, the same plant can produce different leaf shapes across distinct environments, with each shape tailored to specific adaptive functions (Li et al., 2019). Stem is the main transport network for carrying leaves. The length of the stem may directly determine the number of leaves, LA, and other functional traits. As a “bridge” connecting both aboveground leaves and underground roots, the stem plays a key role in plant nutrient transport (Huang et al., 2016; Miao et al., 2023; Sun et al., 2019). Additionally, because the stem of thyme can grow adventitious roots and expand the living area of self-growth, the length of the stem reflects the expansion ability of T. mongolicus.
Thymus mongolicus (Ronniger) Ronniger, a member of the Lamiaceae family, is a perennial small semi-shrub. Its slender, woody stems bear opposite leaves that are small, narrow, and oval-shaped, with surfaces covered in small glands that secrete essential oils with a strong aroma. The flowers of T. mongolicus are small, dense, and light purple or pink, with occasional white variants. These flowers bloom in spring or summer, featuring a long flowering period and significant ornamental value (Sun et al., 2023). The natural distribution of T. mongolicus Shanxi, Hebei, Inner Mongolia, and other regions in China (Li and Hedge, 1994). It is widely distributed in various habitats such as sandy land, grassland, understory and forest-grass transition zone. These regions and habitats have significant differences in environmental factors such as altitude, precipitation, and temperature. In the field sampling survey conducted in this study, the functional traits of T. mongolicus in different regions showed significant differences. However, the mechanism underlying the changes in functional traits needs further exploration. The functional traits of plants are primarily influenced by light, temperature, and water, with different environmental conditions driving diverse adaptation strategies. The relationships between “trait-environment” and “trait-trait” interactions illustrate the principles of optimal adaptation for plant growth in natural conditions. However, previous studies have focused more on the medicinal and edible research of T. mongolicus, limited research has explored the large-scale adaptation of T. mongolicus functional traits to environmental factors or how these traits are internally balanced. This study collected T. mongolicus samples from 145 sites across northern China to address two key scientific questions: (1) How do the functional traits of T. mongolicus adapt to vary across heterogeneous environments? (2) How are T. mongolicus functional traits internally each other during heterogeneous environmental adaptation?
2 Materials and methods
2.1 T. mongolicus collection and transplanting
From 2022 to 2023, we collected T. mongolicus samples from 145 sampling sites across Inner Mongolia, Shanxi, Hebei, and Liaoning. The T. mongolicus at each sampling point was considered a material, and 8 individual plants were collected from each material. In this study, 145 sites were numbered one by one, according to the order of sampling, numbered from Thy1 to Thy145. The sampling sites were spaced at least 30 km apart to avoid spatial redundancy. To minimize duplication, samples were collected from individuals at least 5 m apart. For each site, we recorded latitude, longitude, altitude, habitat characteristics, and associated companion species. Because the field sampling lasted too long, the field measurement led to a large difference in the measurement time between different materials, resulting in differences in functional traits. To maintain a consistent measurement time and environment, the collected plants were placed in pots, watered, and then immediately transplanted to the Grassland Ecosystem Research Station at Inner Mongolia University (Xilinhot, coordinates 44°10′4″N, 116°28′56.8″E). All samples were maintained under uniform field management, with regular weeding but no fertilization, in preparation for subsequent uniform measurements. According to the location of the sampling points, we divided the sampling area into eastern and western regions, and there were significant differences between the east, west and center environmental factors (Supplementary Table S1).
2.2 Measurement of functional traits
In July 2024, we measured 10 functional traits for each plant in 145 samples (Table 1). To prevent experimental errors due to time-of-measurement variability, all samples were processed on the same day. Plant height was measured, and four branches from each plant—healthy, pest-free, and of uniform length—were selected for analysis. These branches were stored in water-sealed bags under refrigeration. Stem lengths were measured using an electronic vernier caliper. For leaf traits, we selected the three largest pairs of opposite leaves on each branch, ensuring they were healthy and pest-free. LA, leaf length, and leaf width (LW) were measured using ImageJ software, with the data being exported to Excel (Microsoft 2021) for further analysis. The remaining leaves on each stem were counted, along with the number and lengths of stem nodes, measured with a vernier caliper. Leaf shape factor was calculated using a defined formula: lSF=(4π*0.8WL)/(L+W)2, where L is leaf length (LL) and W is LW.
2.3 Environmental factor extraction
Plant functional traits adapt to environmental conditions over the long term. Accordingly, we selected 10 stable environmental factors for predictive modeling (Table 2). These factors were categorized into spatial, topographic, and climatic variables. Longitude, latitude, and altitude were derived from GPS data, while the remaining factors were sourced from WorldClim (https://www.worldclim.org/).
2.4 Data analysis
The data analysis in this study was conducted using the R programming language (R version 4.4.1; R Core Team, 2023). The mean (mean), standard deviation, and coefficient of variation (CV) were calculated with the dplyr package (Wickham et al., 2023). Principal component analysis (PCA) was performed using the factoextra package (Kassambara and Mundt, 2020), while correlation analysis between functional traits and environmental factors was carried out with the pheatmap package (Kolde, 2019). The interpretation of PCA results for functional traits was guided by a scree plot. Elbow analysis and Gap statistics were employed to determine the optimal number of clusters. Functional correlation analysis was conducted with the corrplot package (Taiyun and Viliam, 2024). A relational network was visualized using the igraph package, retaining edges with correlations above 0.5 (Csardi and Nepusz, 2006). A random forest model was used to evaluate the importance of environmental factors in shaping functional traits. The randomForest (Liaw and Wiener, 2002) and rfPermute (Archer, 2023) packages were utilized for model construction and prediction. Unlike the standard randomForest function, the rfPermute function was chosen for its permutation-based approach to variable importance, yielding more robust results. The model was configured with 500 trees and 1,000 bootstrap iterations to enhance predictive accuracy. Model confidence and significance were tested using the A3 package (Fortmann-Roe, 2015), yielding p-values of < 0.05, which confirmed the reliability of the predictions. Map data utilized in this study were sourced from the Alibaba Cloud data visualization platform.
3 Result
3.1 Descriptive statistics of functional traits
In LN, the distribution of most materials was concentrated in the range of 25-50 and 50-75; in LA, the LA values of most materials were distributed between 12-15 and 15-18; in LL, the range of 8-12 contains the vast majority of materials; in LW, the range of 2.5-3.5 is the most concentrated; the distribution peak of LSF appears at 1.8. In the L:W ratio, the distribution of most materials was concentrated in the range of 2.5-3.0 (Supplementary Figure S1). The height distribution of most materials was in the range of 4.0-6.0 and 6.0-8.0; the range of 20-40 contains the most materials; the SN value of most materials was 8; the SNL values were distributed in the range of 0-10, covering the vast majority of materials (Supplementary Figure S2). Additionally, the CV of functional traits of T. mongolicus varied significantly across different regions, indicating that thyme exhibits considerable differentiation in its functional traits in response to regional variations (Supplementary Table S2).
3.2 Cluster analysis of the functional traits of T. mongolicus
This study utilized PCA to examine the functional traits of T. mongolicus from two perspectives. First, PCA was conducted based on the spatial distribution of sampling points. The first two principal components of the gravel map explained 98.8% of the total variance, indicating a strong interpretative capacity for functional traits (Supplementary Figure S3). The results revealed a clear differentiation of functional traits between the eastern and western regions, with central region traits bridging the two without clear separation (Figure 1).
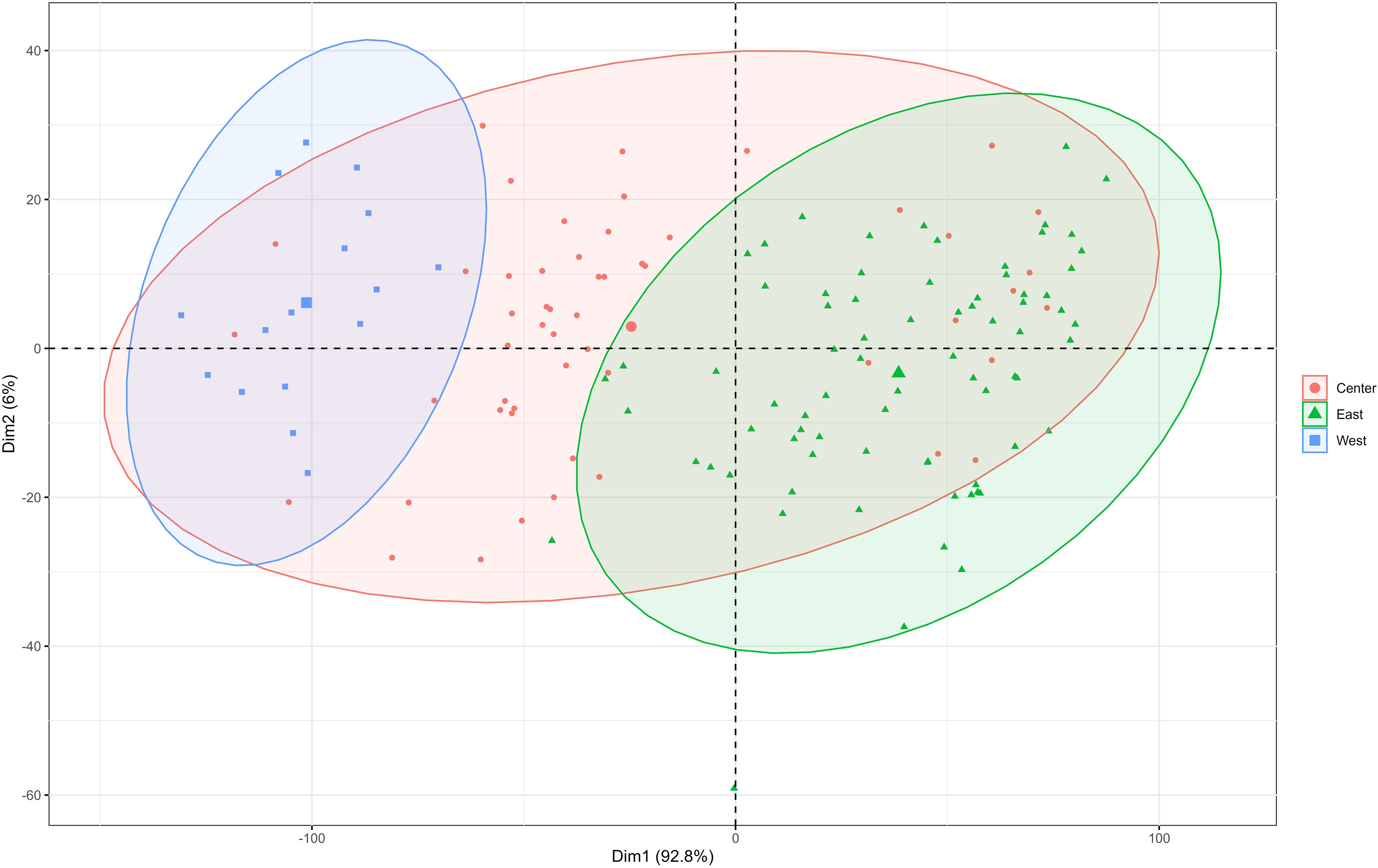
Figure 1. PCA was performed on the functional traits of T. mongolicus according to the sampling points.
Subsequently, PCA was performed on the functional traits to determine the optimal clustering, identified as four clusters using elbow and GAP analyses (Supplementary Figure S4). The results indicated that the 145 materials could be grouped into four clusters based on their functional traits. The PCA results showed significant overlap between Clusters 1 and 4, as well as between Clusters 2 and 3. This suggests that Cluster 1 and Cluster 4 share more similarities, while Cluster 2 and Cluster 3 are more similar to each other. However, there is no overlap between Cluster 1 and Cluster 5, indicating a significant difference between them (Figure 2; Supplementary Figure S5). Based on the principal component loadings for each axis, it can be seen that LA, LW, Height, SL, and SNL have higher loadings on PC1, while LL, LSF, and L:W have higher loadings on PC2. The loading of LL is the highest on both axes, indicating that LL plays a crucial role in the functional trait variation of T. mongolicus.
3.3 Correlation analysis of the functional traits of T. mongolicus
Further analysis explored the correlations between the functional traits and environmental factors of T. mongolicus. Longitude and latitude exhibited significant positive correlations with the length-to-width (L:W) ratio and significant negative correlations with other functional traits. Slope, mean annual temperature (MAT), and maximum and minimum temperatures also showed significant negative correlations with L:W. Other functional shape indicators correlated negatively with latitude and longitude but showed significant positive correlations with geographic and climatic factors to varying degrees. Altitude, MAT, and GR had particularly strong effects on functional traits such as LA, LL, LW, and plant height, whereas slope and aspect showed no significant correlations (Figure 3).
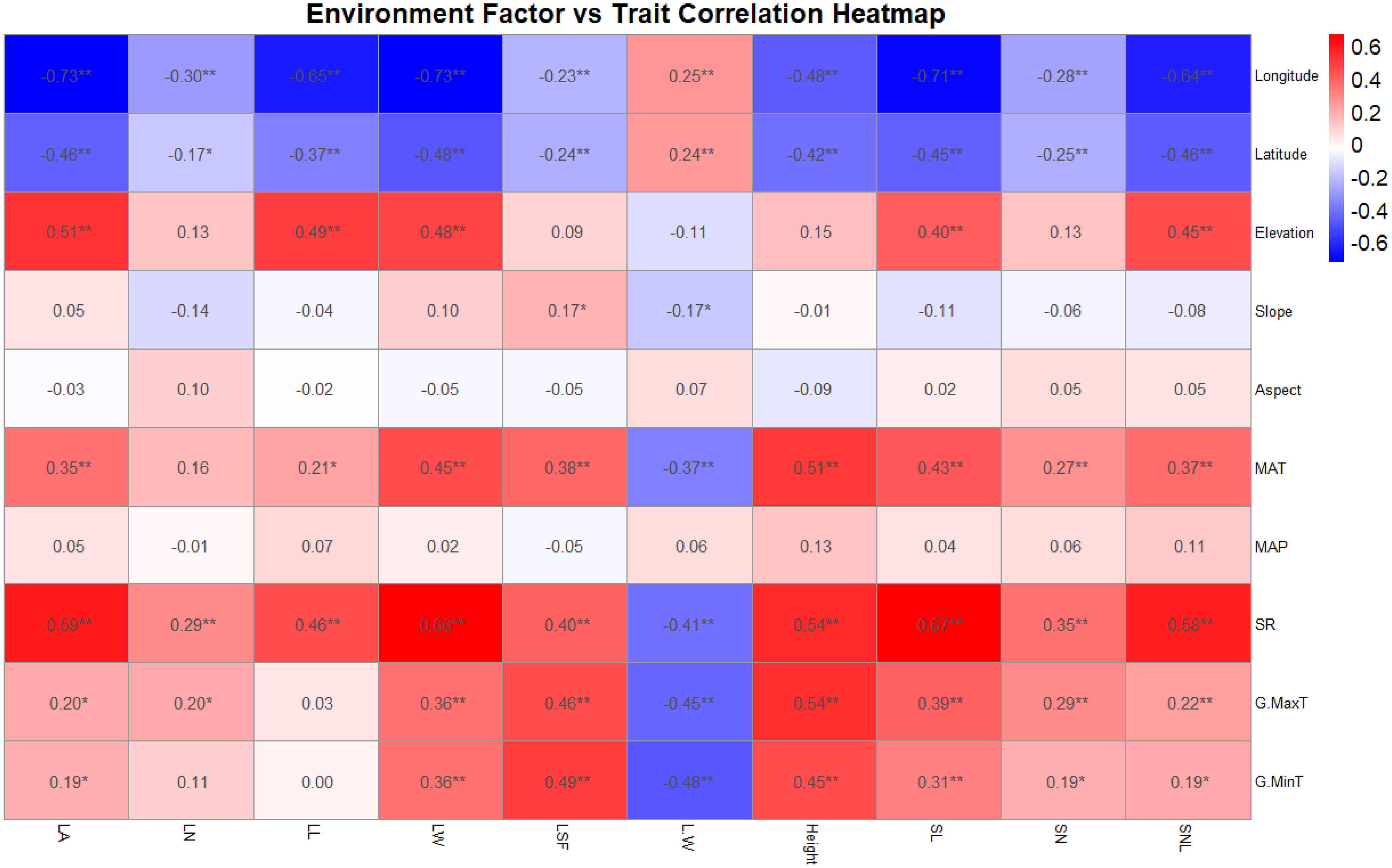
Figure 3. Heat map analysis of functional traits and environmental factors of T. mongolicus. LN, leaf number per branch; LA: leaf area; LL, leaf length; LW, leaf width; LSF, leaf shape factor; L:W, leaf length:leaf width; SL, stem length; SN, stem node number; SNL, stem node length; MAT, mean annual temperature; MAP, mean annual precipitation; SR, solar radiation; G.MaxT, growing maximum temperature; G.MinT, growing minimum temperature. The number in the figure represents the correlation coefficient, *P < 0.05; **P < 0.01.
Finally, autocorrelation analysis highlighted strong interrelationships among the functional traits of T. mongolicus. LA exhibited significant positive correlations with LL and LW, while plant height and SL were similarly correlated. Other indicators also showed substantial interdependence, underscoring the intrinsic linkages between functional traits of T. mongolicus (Figure 4). In addition to the significant correlation between functional traits, there were also significant correlations between different types of environmental factors, indicating that there were also significant synergistic changes between environmental factors (Supplementary Figure S6).
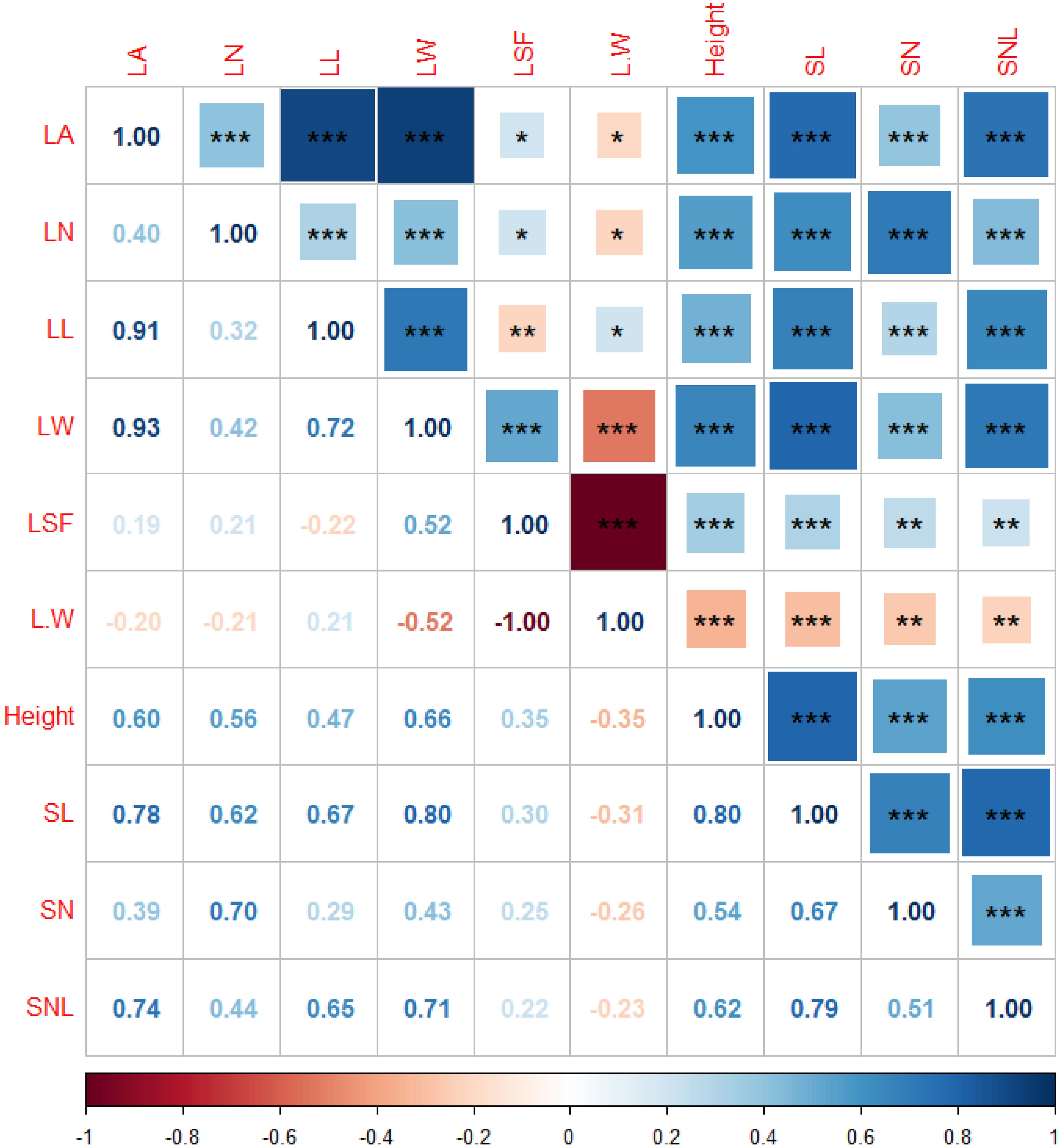
Figure 4. Autocorrelation analysis of the functional traits of T. mongolicus. LN, leaf number per branch; LA, leaf area; LL, leaf length; LW, leaf width; LSF, leaf shape factor; L:W, leaf length:leaf width; SL, stem length; SN, stem node number; SNL, stem node length. *P < 0.05; **P < 0.01, *** P < 0.001.
A network analysis of 10 functional trait indicators was performed, using correlations above 0.5 as the threshold for node connections. The L:W had correlations below 0.5 with all other traits and was thus excluded from the network. In the network, traits such as LW, LA, SNL, SL, and plant height had a higher number of connections than average, indicating their central roles. In contrast, traits such as LL, LN, and SN had fewer connections. SL had the highest connectivity, with seven nodes, whereas LSF had the least, with only one node (Figure 5).
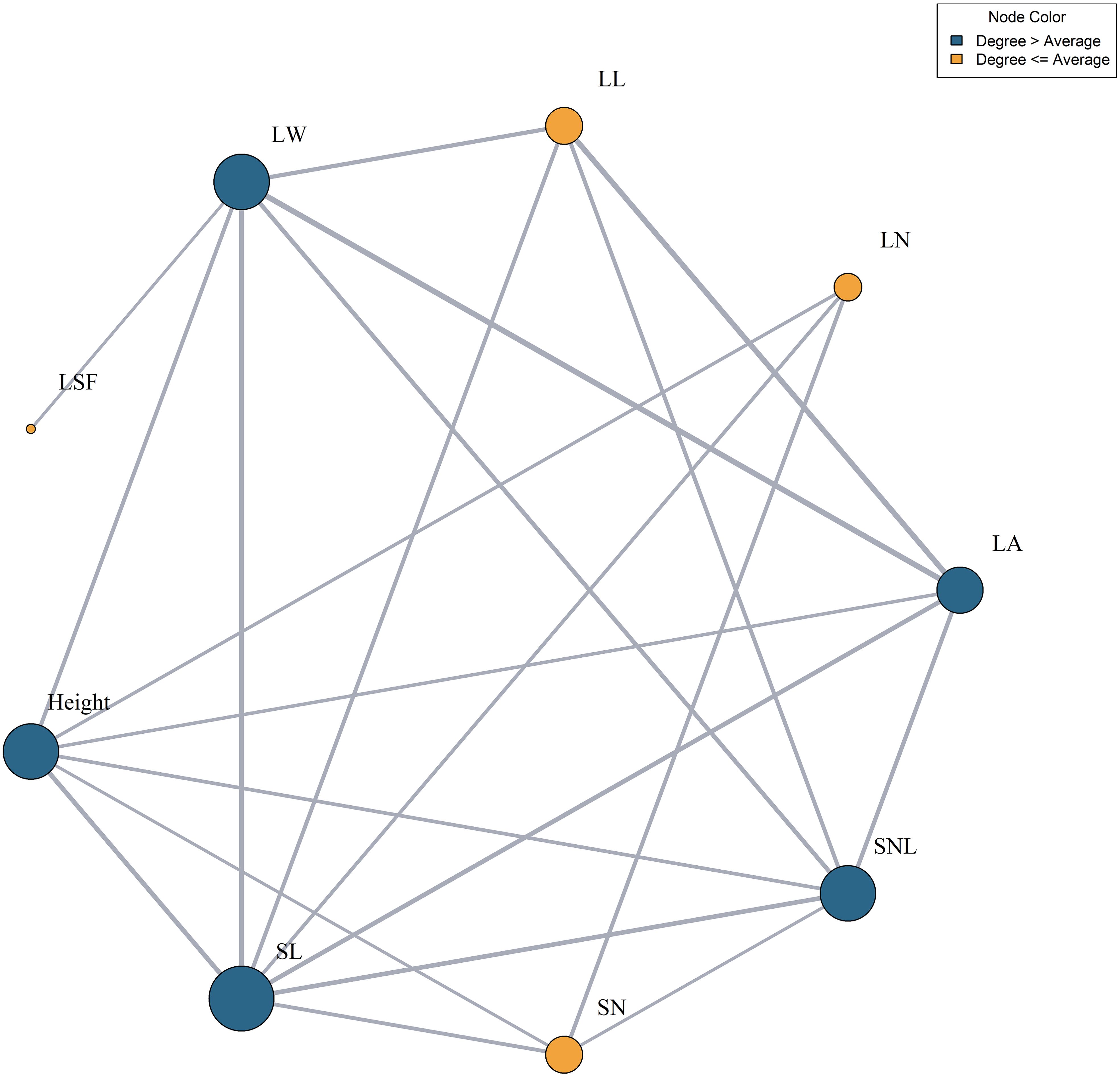
Figure 5. Functional trait relationship network. LN, leaf number per branch; LA, leaf area; LL, leaf length; LW, leaf width; LSF, leaf shape factor; SL, stem length; SN, stem node number; SNL, stem node length. Dark blue indicates that the connectivity is greater than the average connectivity, and yellow indicates that the connectivity is lower than the average connectivity. The larger the circle, the higher the degree of connection.
3.4 Importance prediction of environmental factors
We used a random forest model to predict the contribution and significance of environmental factors to functional traits. In LN, latitude and longitude were the most significant factors. In LA, latitude, longitude, SR and elevation were the most significant factors. In LL, latitude, longitude, elevation, SR, MAT and mean annual precipitation (MAP) were the most significant factors. In LW, all environmental factors except aspect, MAP and slope were significant. Similarly, in LSF, all factors except elevation, slope, and aspect were significant. In L:W, all factors except MAP, elevation, slope and aspect showed notable importance (Figure 6).
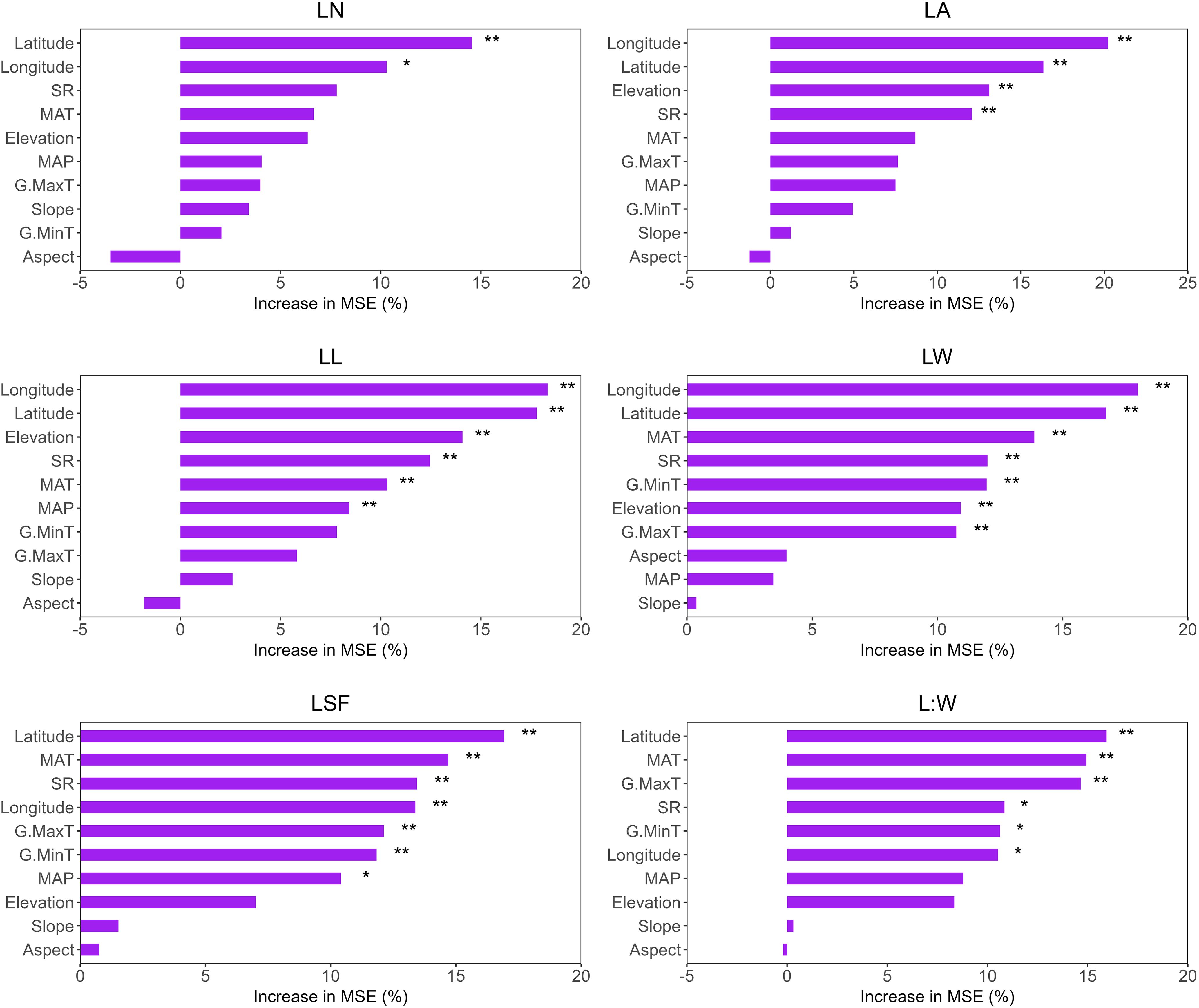
Figure 6. Random Forest model was used to predict the relative importance and ranking of environmental factors on the functional traits of T. mongolicus. MAT, mean annual temperature; MAP, mean annual precipitation; SR, solar radiation; G.MaxT, growing maximum temperature; G.MinT, growing minimum temperature. LN, leaf number per branch; LA, leaf area; LL, leaf length; LW, leaf width; LSF, leaf shape factor; L:W, leaf length:leaf width. *P < 0.05; **P < 0.01.
Our results indicate that, apart from elevation, slope, and aspect, other environmental factors substantially influenced plant height. In SL, longitude, latitude, SR, and G.MinT were the primary contributors. For SN, all factors except longitude, elevation, MAP, slope, and aspect had a significant impact. For SNL, longitude, latitude, and SR showed high contributions (Figure 7). Overall, the random forest analysis demonstrated that latitude, longitude, and temperature-related factors were key predictors across all models.
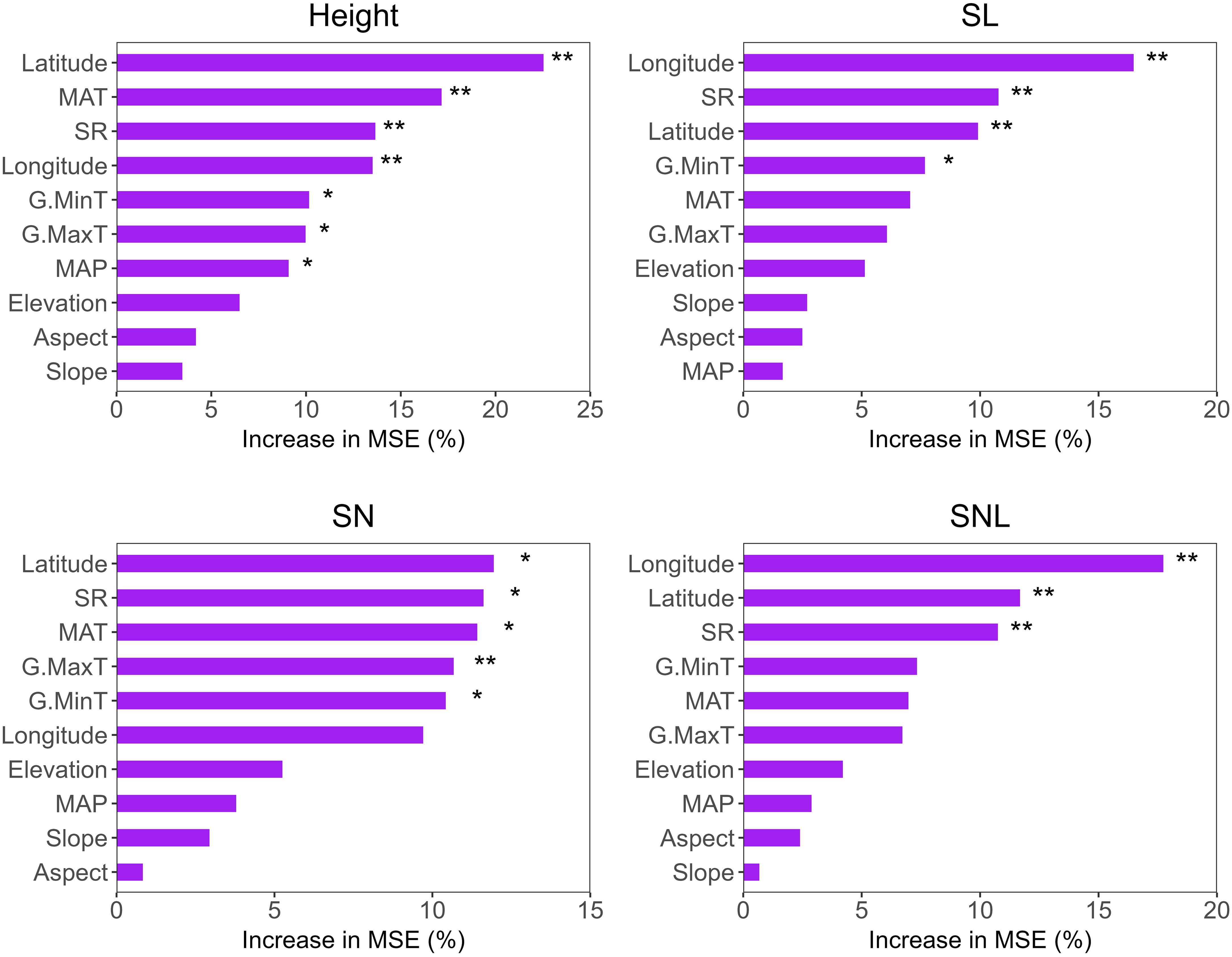
Figure 7. Random Forest model was used to predict the relative importance and ranking of environmental factors on the functional traits of T. mongolicus. MAT, mean annual temperature; MAP, mean annual precipitation; SR, solar radiation; G.MaxT, growing maximum temperature; G.MinT, growing minimum temperature. SL, stem length; SN, stem node number; SNL, stem node length. *P < 0.05; **P < 0.01.
4 Discussion
4.1 Analysis of the differential variation in the functional traits of T. mongolicus
Plant functional traits are shaped by a combination of genetic regulation and external environmental influences (Maugarny-Calès and Laufs, 2018). To reduce the differences in functional traits caused by different sampling times, this study adopted the method of transplanting field materials into a homogeneous garden to maintain consistency in sampling time and measurement methods to reduce external errors and improve the accuracy of the experiment. Our results revealed significant variations in the functional traits of across regions, with pronounced differences between sampling sites. This variability likely stems from two main factors. First, because thyme is a species that relies on insect pollination and has a short seed dispersal distance, limited gene flow between different regions may lead to genetic differentiation at a large scale, resulting in differences in functional traits, thus promoting the differentiation of regional characteristics (Abraham et al., 2018; Campolo et al., 2016). Second, functional traits reflect the plant’s long-term adaptation to environmental conditions (Fritz et al., 2018). The considerable environmental heterogeneity across the study area—including variations in habitat, topography, and climate—has driven significant differences in functional traits as T. mongolicus adapts to local conditions.
Our results demonstrated that the functional traits of T. mongolicus across different sites exhibited a high CV. The CV is a key metric for assessing the degree of variation in functional traits within a population and serves as an important indicator of the population’s capacity to adapt to environmental changes (Moller et al., 2023). The high CV observed in T. mongolicus reflects its robust adaptability to environmental variability and diversity. Furthermore, this high CV suggests that T. mongolicus possesses significant ecological resilience and adaptability, enabling it to cope effectively with environmental pressures. These findings align with its known biological traits of drought resistance, cold tolerance, and resilience to nutrient-poor conditions (Martin et al., 2017).
4.2 Relationship between functional traits and the environmental gradient
In our study, latitude and longitude showed a significant negative correlation with the remaining nine indicators, except for the L:W ratio. This is consistent with previous research, which found that functional traits exhibited significant adaptability to latitudinal and longitudinal gradients (Chang et al., 2024; Zhou et al., 2022). Our results also revealed a significant positive correlation between the functional traits of T. mongolicus and both MAT and solar radiation (SR). Temperature is a critical factor influencing plant growth and development as it determines photosynthetic efficiency and growth rates (Schellenberger Costa et al., 2017; Lu et al., 2016). In the Northern Hemisphere, temperature decreases significantly with increasing latitude, and plants adapt to these environmental gradients through specific functional trait combinations (Wright et al., 2004). For example, as latitude increases and temperatures decline, lower accumulated temperatures and shorter growing seasons inhibit plant growth. In response to colder climates, T. mongolicus adjusts its traits to minimize heat loss and enhance photosynthetic capacity. For instance, in our study, with increasing latitude, LW narrowed and LA decreased. Slender leaves helped minimize heat loss (Guerin et al., 2012). Additionally, plant height decreases to reduce heat dissipation, a response to both shorter growth cycles and lower accumulated temperatures (Moles et al., 2009). This results in a general decline in functional traits at higher latitudes compared to lower latitudes. Similarly, in northern China, where temperature decreases with increasing longitude, the functional trait trends along the longitude gradient mirror those along the latitude gradient. Additionally, a significant positive correlation was observed between LA and elevation. This may be due to the increase in altitude, resulting in more direct and strong sunlight. In such an environment, plants may increase the surface area involved in photosynthesis by increasing LA and may absorb more sunlight to ensure sufficient energy supply (Sharma et al., 2024; Wei et al., 2021).
The L:W reflects the plant’s environmental adaptation strategy. The slender leaf shape is more effective at retaining heat and water compared to the wider leaf shape (Schellenberger Costa et al., 2017). Our study found that the L:W ratio of T. mongolicus increased significantly with longitude, and the leaf shape was slender. Indicating an evolutionary trend toward reducing water loss and conserving heat in response to colder and drier environments (Fan et al., 2020). Conversely, L:W exhibited a significant negative correlation with temperature and rainfall. In warmer and wetter environments, plants adapt by dissipating heat more efficiently and improving water use efficiency (Toscano et al., 2019; Yavas et al., 2024). At lower latitudes, broader leaves help maintain temperature and water balance, thereby enhancing photosynthetic capacity.
4.3 Synergistic correlation among functional traits
Plants function as a continuum, with various organs interconnected to perform distinct functions, cooperate, influence each other, and collectively regulate life activities. To adapt to diverse environments, plant organs interact dynamically, forming an “optimal collocation” (Guittar et al., 2016; He et al., 2020). Our research identified SL as the trait with the highest number of nodes and the strongest associations with other functional traits. This prominence arises because the stem serves as the primary conduit for nutrient transport and a structural base for leaf growth (Zhang et al., 2020). These attributes directly determine the number of leaves, nutrient availability during leaf development, and the integration of T. mongolicus leaves with other organs. Consequently, SL exhibits more nodes compared to other functional traits.
Among leaf attributes, LW and LA have demonstrated higher-than-average node counts. This is because LW and LA are highly plastic traits that frequently change in response to environmental conditions, enhancing adaptation. Changes in these traits significantly influence photosynthetic capacity and nutrient acquisition, which, in turn, impact other functional traits of T. mongolicus (Gong et al., 2020; Zhang et al., 2019). The observed strong correlations between LW, LA, and environmental factors—especially MAT—further support this conclusion.
5 Conclusion
Our findings revealed that T. mongolicus exhibited differentiation in functional traits to adapt to varied environments, leading to significant trait variation across different locations. In this adaptive process, the temperature gradient associated with latitude and longitude was a key factor influencing functional traits. Furthermore, multiple traits interacted closely during adaptation, with SL, LW, and LA playing critical roles. These results elucidated the variation pattern of T. mongolicus in northern China and identified key factors driving functional trait variation. Such insights established a solid foundation for future breeding efforts targeting T. mongolicus.
Data availability statement
The original contributions presented in the study are included in the article/Supplementary Material. Further inquiries can be directed to the corresponding author.
Author contributions
WM: Conceptualization, Methodology, Software, Writing – original draft, Writing – review & editing. HZ: Conceptualization, Methodology, Writing – review & editing. HXZ: Data curation, Investigation, Writing – review & editing. WZ: Investigation, Writing – review & editing. YC: Writing – review & editing, Investigation. WR: Funding acquisition, Writing – review & editing.
Funding
The author(s) declare that financial support was received for the research and/or publication of this article. This work was financially supported by the Major Special Foundation of Science and Technology Plan of Inner Mongolia (Nos. 2023YFSH0025, 2021ZD00804) and the project for Young talent scientists of Inner Mongolia (No. NMGIRT2316).
Acknowledgments
This study is grateful to Grassland Ecosystem Research Station at Inner Mongolia University for providing experimental sites.
Conflict of interest
The authors declare that the research was conducted in the absence of any commercial or financial relationships that could be construed as a potential conflict of interest.
Generative AI statement
The author(s) declare that no Generative AI was used in the creation of this manuscript.
Publisher’s note
All claims expressed in this article are solely those of the authors and do not necessarily represent those of their affiliated organizations, or those of the publisher, the editors and the reviewers. Any product that may be evaluated in this article, or claim that may be made by its manufacturer, is not guaranteed or endorsed by the publisher.
Supplementary material
The Supplementary Material for this article can be found online at: https://www.frontiersin.org/articles/10.3389/fpls.2025.1596849/full#supplementary-material
References
Abraham, E. M., Aftzalanidou, A., Ganopoulos, I., Osathanunkul, M., Xanthopoulou, A., Avramidou, E., et al. (2018). Genetic diversity of Thymus sibthorpii Bentham in mountainous natural grasslands of Northern Greece as related to local factors and plant community structure. Ind. Crops Prod. 111, 651–659. doi: 10.1016/j.indcrop.2017.11.038
Archer, E. (2023). rfPermute: Estimate Permutation p-Values for Random Forest Importance Metrics (R package version 2.5.2). Available at: https://CRAN.R-project.org/package=rfPermute.
Campolo, O., Zappala, L., Malacrino, A., Laudani, F., and Palmeri, V. (2016). Bees visiting flowers of Thymus longicaulis (Lamiaceae). Plant Biosyst. 150, 1182–1188. doi: 10.1080/11263504.2015.1009190
Candeias, M. and Fraterrigo, J. (2020). Trait coordination and environmental filters shape functional trait distributions of forest understory herbs. Ecol. Evol. 10, 14098–14112. doi: 10.1002/ece3.7000
Chang, W., Song, Q., Zheng, X., Li, C., Wang, L., Li, H., et al. (2024). Leaf trait variations and correlations across four forests with similar mean annual precipitation in northern China. Ecol. Indic. 165, 112199. doi: 10.1016/j.ecolind.2024.112199
Chitwood, D. H. and Sinha, N. R. (2016). Evolutionary and environmental forces sculpting leaf development. Curr. Biol. 26, 297–R306. doi: 10.1016/j.cub.2016.02.033
Cornelissen, J. H. C., Lavorel, S., Garnier, E., Díaz, S., Buchmann, N., Gurvich, D. E., et al. (2003). A handbook of protocols for standardised and easy measurement of plant functional traits worldwide. Aust. J. Bot. 51, 335. doi: 10.1071/bt02124
Csardi, G. and Nepusz, T. (2006). The igraph software package for complex network research._InterJournal_, *Complex Systems*. Available online at: https://igraph.org.
Díaz, S. and Cabido, M. (2001). Vive la difference: plant functional diversity matters to ecosystem processes. Trends Ecol. Evolut. 16, 646–655. doi: 10.1016/s0169-5347(01)02283-2
Fan, X., Yan, X., Qian, C., Bachir, D. G., Yin, X., Sun, P., et al. (2020). Leaf size variations in a dominant desert shrub, Reaumuria soongarica, adapted to heterogeneous environments. Ecol. Evolut. 10, 10076–10094. doi: 10.1002/ece3.6668
Fortmann-Roe, S. (2015). Consistent and clear reporting of results from diverse modeling techniques: The A3 Method. J. Stat. Soft. 66, 1–23. doi: 10.18637/jss.v066.i07
Fritz, M. A., Rosa, S., and Sicard, A. (2018). Mechanisms underlying the environmentally induced plasticity of leaf morphology. Front. Genet. 9. doi: 10.3389/fgene.2018.00478
Gong, H. D., Cui, Q. J., and Gao, J. (2020). Latitudinal, soil and climate effects on key leaf traits in northeastern China. Global Ecol. Conservat. 22. doi: 10.1016/j.gecco.2020.e00904
Guerin, G. R., Wen, H., and Lowe, A. J. (2012). Leaf morphology shift linked to climate change. Biol. Lett. 8, 882–886. doi: 10.1098/rsbl.2012.0458
Guittar, J., Goldberg, D., Klanderud, K., Telford, R. J., and Vandvik, V. (2016). Can trait patterns along gradients predict plant community responses to climate change? Ecology 97, 2791–2801. doi: 10.1002/ecy.1500
He, N., Li, Y., Liu, C., Xu, L., Li, M., Zhang, J., et al. (2020). Plant trait networks: Improved resolution of the dimensionality of adaptation. Trends Ecol. Evolut. 35, 908–918. doi: 10.1016/j.tree.2020.06.003
Henn, J. J., Buzzard, V., Enquist, B. J., Halbritter, A. H., Klanderud, K., Maitner, B. S., et al. (2018). Intraspecific trait variation and phenotypic plasticity mediate alpine plant species response to climate change. Front. Plant Sci. 9. doi: 10.3389/fpls.2018.01548
Huang, Y., Lechowicz, M. J., Price, C. A., Li, L., Wang, Y., and Zhou, D. (2016). The underlying basis for the trade-off between leaf size and leafing intensity. Funct. Ecol. 30, 199–205. doi: 10.1111/1365-2435.12491
Hulshof, C. M., Violle, C., Spasojevic, M. J., Mcgill, B., Damschen, E., Harrison, S., et al. (2013). Intra-specific and inter-specific variation in specific leaf area reveal the importance of abiotic and biotic drivers of species diversity across elevation and latitude. J. Vegetat. Sci. 24, 921–931. doi: 10.1111/jvs.12041
Jin, Y., Ye, Q., Liu, X., Liu, H., Gleason, S. M., He, P., et al. (2024). Precipitation, solar radiation, and their interaction modify leaf hydraulic efficiency–safety trade-off across angiosperms at the global scale. New Phytol. 244, 2267–2277. doi: 10.1111/nph.20213
Kassambara, A. and Mundt, F. (2020). _factoextra: Extract and visualize the results of multivariate data analyses (Rpackage version 1.0.7). Available at: https://CRAN.R-project.org/package=factoextra.
Kerr, K. L., Fickle, J. C., and Anderegg, W. R. L. (2023). Decoupling of functional traits from intraspecific patterns of growth and drought stress resistance. New Phytol. 239, 174–188. doi: 10.1111/nph.18937
Kolde, R. (2019). _pheatmap: pretty heatmaps_ (R package version 1.0.12). Available at: https://CRAN.R-project.org/package=pheatmap.
Koyama, K., Hidaka, Y., and Ushio, M. (2012). Dynamic scaling in the growth of a non-branching plant, Cardiocrinum cordatum. PloS One 7. doi: 10.1371/journal.pone.0045317
Li, X. W. and Hedge, I. C. (1994). Flora of China: lamiaceae Vol. 17 (Science Press, Beijing: Missouri Botanical Garden Press), 186–188.
Li, G. J., Hu, S. Q., Hou, H. W., and Kimura, S. (2019). Heterophylly: Phenotypic plasticity of leaf shape in aquatic and amphibious plants. Plants-Basel 8. doi: 10.3390/plants8100420
Liaw, A. and Wiener, M. (2002). Classification and Regression by randomForest Vol. *2* (_R News_), 18–22. Available at: https://CRAN.R-project.org/doc/Rnews/.
Lu, X., Zhou, G., Wang, Y., and Song, X. (2016). Effects of changing precipitation and warming on functional traits of zonal Stipa plants from inner Mongolian grassland. J. Meteorol. Res. 30, 412–425. doi: 10.1007/s13351-016-5091-5
Martin, A. R., Rapidel, B., Roupsard, O., Van den Meersche, K., Virginio, E. D., Barrios, M., et al. (2017). Intraspecific trait variation across multiple scales: the leaf economics spectrum in coffee. Funct. Ecol. 31, 604–612. doi: 10.1111/1365-2435.12790
Maugarny-Calès, A. and Laufs, P. (2018). Getting leaves into shape: a molecular, cellular, environmental and evolutionary view. Development 145. doi: 10.1242/dev.161646
Mello, F. N. A., Estrada-Villegas, S., Defilippis, D. M., and Schnitzer, S. A. (2020). Can functional traits explain plant coexistence? A case study with tropical lianas and trees. Diversity-Basel 12. doi: 10.3390/d12100397
Miao, F., Yu, X., Tang, X., Liu, X., Tang, W., Zhao, Y., et al. (2023). The Responses of Stem and Leaf Functional Traits of Medicago sativa and Bromus inermis to Different Mixed Planting Patterns. Agronomy-Basel 13. doi: 10.3390/agronomy13112733
Moles, A. T., Warton, D. I., Warman, L., Swenson, N. G., Laffan, S. W., Zanne, A. E., et al. (2009). Global patterns in plant height. J. Ecol. 97, 923–932. doi: 10.1111/j.1365-2745.2009.01526.x
Moller, C., March-Salas, M., Kuppler, J., De Frenne, P., and Scheepens, J. F. (2023). Intra-individual variation in Galium odoratum is affected by experimental drought and shading. Annal. Bot. 131, 411–422. doi: 10.1093/aob/mcac148
Onoda, Y., Richards, L., and Westoby, M. (2012). The importance of leaf cuticle for carbon economy and? mechanical strength. New Phytol. 196, 441–447. doi: 10.1111/j.1469-8137.2012.04263.x
R Core, T. (2023). R: A language and environment for statistical computing. R Foundation for Statistical Computing. Available online at: https://www.R-project.org.
Reich, P. B., Wright, I. J., Cavender-Bares, J., Craine, J. M., Oleksyn, J., Westoby, M., et al. (2003). The evolution of plant functional variation: Traits, spectra, and strategies. Int. J. Plant Sci. 164, 143–S164. doi: 10.1086/374368
Schellenberger Costa, D., Classen, A., Ferger, S., Helbig-Bonitz, M., Peters, M., Böhning-Gaese, K., et al. (2017). Relationships between abiotic environment, plant functional traits, and animal body size at Mount Kilimanjaro, Tanzania. PloS One 12. doi: 10.1371/journal.pone.0174157
Sharma, P., Rathee, S., Ahmad, M., Siddiqui, M. H., Alamri, S., Kaur, S., et al. (2024). Leaf functional traits and resource use strategies facilitate the spread of invasive plant Parthenium hysterophorus across an elevational gradient in western Himalayas. BMC Plant Biol. 24. doi: 10.1186/s12870-024-04904-0
Sobral, M. (2021). All traits are functional: An evolutionary viewpoint. Trends Plant Sci. 26, 674–676. doi: 10.1016/j.tplants.2021.04.004
Souza, J. P. S., Silva, M. P. P., and Pôrto, K. C. (2020). Spatial distribution of functional traits of bryophytes along environmental gradients in an Atlantic Forest remnant in north-eastern Brazil. Plant Ecol. Diversity 13, 93–104. doi: 10.1080/17550874.2019.1709227
Sun, J., Wang, M., Lyu, M., Niklas, K. J., Zhong, Q., Li, M., et al. (2019). Stem and leaf growth rates define the leaf size vs. number trade-off. Aob Plants 11. doi: 10.1093/aobpla/plz063
Sun, M. Y., Zhang, Y. N., Bai, H. T., Sun, G. F., Zhang, J. Z., and Shi, L. (2023). Population diversity analyses provide insights into key horticultural traits of Chinese native thymes. Horticult. Res. 10. doi: 10.1093/hr/uhac262
Taiyun, W. and Viliam, S. (2024). R package ‘corrplot’: Visualization of a correlation matrix (Version 0.95). Available online at: https://github.com/taiyun/corrplot.
Toscano, S., Ferrante, A., and Romano, D. (2019). Response of mediterranean ornamental plants to drought stress. Horticulturae 5. doi: 10.3390/horticulturae5010006
Vilà-Cabrera, A., Martínez-Vilalta, J., and Retana, J. (2015). Functional trait variation along environmental gradients in temperate and Mediterranean trees. Global Ecol. Biogeogr. 24, 1377–1389. doi: 10.1111/geb.12379
Wang, C. A., Li, X., Lu, X. M., Wang, Y., and Bai, Y. F. (2023). Intraspecific trait variation governs grazing-induced shifts in plant community above- and below-ground functional trait composition. Agric. Ecosyst. Environ. 346. doi: 10.1016/j.agee.2023.108357
Wei, G.-W., Chen, Y.-H., Sun, X.-S., Matsubara, S., Luo, F.-L., and Yu, F.-H. (2021). Elevation-dependent selection for plasticity in leaf and root traits of Polygonumhydropiper in response to flooding. Environ. Exp. Bot. 182. doi: 10.1016/j.envexpbot.2020.104331
Wickham, H., François, R., Henry, L., Müller, K., and Vaughan, D. (2023). ._dplyr: A grammar of data manipulation_ (R package version 1.1.4). Available at: https://CRAN.R-project.org/package=dplyr.
Wieczynski, D. J., Boyle, B., Buzzard, V., Duran, S. M., Henderson, A. N., Hulshof, C. M., et al. (2019). Climate shapes and shifts functional biodiversity in forests worldwide. Proc. Natl. Acad. Sci. U.S.A. 116, 587–592. doi: 10.1073/pnas.1813723116
Wright, I. J., Reich, P. B., Westoby, M., Ackerly, D. D., Baruch, Z., Bongers, F., et al. (2004). The worldwide leaf economics spectrum. Nature 428, 821–827. doi: 10.1038/nature02403
Yavas, I., Jamal, M. A., Ul Din, K., Ali, S., Hussain, S., and Farooq, M. (2024). Drought-induced changes in leaf morphology and anatomy: Overview, implications and perspectives. Polish J. Environ. Stud. 33. doi: 10.15244/pjoes/174476
Zhang, C. C., Gu, R., Lin, L. X., and Russo, S. E. (2024). Functional traits and ecological niches as correlates of the interspecific growth-mortality trade-off among seedlings of 14 tropical tree species. Funct. Ecol. 38, 1888–1901. doi: 10.1111/1365-2435.14624
Zhang, X., He, X., Gao, J., and Wang, L. (2019). Latitudinal and climate effects on key plant traits in Chinese forest ecosystems. Glob. Ecol. Conserv. 17. doi: 10.1016/j.gecco.2019.e00527
Zhang, J., He, N., Liu, C., Xu, L., Chen, Z., Li, Y., et al. (2020). Variation and evolution of C:N ratio among different organs enable plants to adapt to N-limited environments. Glob. Change Biol. 26, 2534–2543. doi: 10.1111/gcb.14973
Keywords: adaptability, functional traits, Thymus mongolicus, environmental gradient, leaf
Citation: Mi W, Zheng H, Zhang H, Zhang W, Chi Y and Ren W (2025) Changes in the functional traits of Thymus mongolicus along environmental gradients and factors influencing the traits of Northern China. Front. Plant Sci. 16:1596849. doi: 10.3389/fpls.2025.1596849
Received: 20 March 2025; Accepted: 28 April 2025;
Published: 20 May 2025.
Edited by:
Mehrdad Zarafshar, Linnaeus University, SwedenReviewed by:
Chenni Zhou, Sichuan University of Science and Engineering, ChinaSara Pedraza, University of California Los Angeles, United States
Copyright © 2025 Mi, Zheng, Zhang, Zhang, Chi and Ren. This is an open-access article distributed under the terms of the Creative Commons Attribution License (CC BY). The use, distribution or reproduction in other forums is permitted, provided the original author(s) and the copyright owner(s) are credited and that the original publication in this journal is cited, in accordance with accepted academic practice. No use, distribution or reproduction is permitted which does not comply with these terms.
*Correspondence: Weibo Ren, cndlaWJvMjAyMkAxNjMuY29t