- 1School of Computer Science, Guangzhou Maritime University, Guangzhou, Guangdong, China
- 2Hubei University of Economics, Wuhan, China
- 3Hebei Academy of Fine Arts, Shijiazhuang, Hebei, China
- 4Hanyang University, Ansan-si, Gyeonggi-do, Republic of Korea
Introduction: Plant phenotyping is a critical area in agricultural research that focuses on assessing plant traits quantitatively to enhance productivity and sustainability. While traditional methods remain important, they are constrained by the complexity of plant structures, variability in environmental conditions, and the need for high-throughput analysis. Recent advances in imaging technologies and machine learning offer new possibilities, but current methods still face challenges such as noise, occlusion, and limited interpretability.
Methods: In response to these challenges, we propose a novel computational framework that combines deep learning-based text generation with domain-specific knowledge for plant phenotyping. Our approach incorporates three key elements. A hybrid generative model is used to capture complex spatial and temporal phenotypic patterns. A biologically-constrained optimization strategy is employed to improve both prediction accuracy and interpretability. An environment-aware module is included to address environmental variability.
Results: The generative model uses advanced deep learning techniques to process high-dimensional imaging data, effectively capturing complex plant traits while overcoming issues like occlusion and variability. The biologically-constrained optimization strategy incorporates prior biological knowledge into the computational process, ensuring predictions are biologically realistic and enhancing trait correlations and structural consistency. The environment-aware module adapts dynamically to environmental factors, ensuring reliable predictions across a variety of agricultural settings.
Discussion: Experimental results show that the framework delivers scalable, interpretable, and accurate phenotyping solutions, setting a new standard for precision agriculture applications.
1 Introduction
Plant phenotyping, which involves the measurement of observable plant traits, is essential for understanding plant behavior, improving crop yields, and advancing precision agriculture Zhang et al. (2024). This task is becoming increasingly significant due to the growing demands of global food security and the need to address challenges such as climate change, resource constraints, and pest management Johri et al. (2024). Not only does phenotyping provide valuable insights into the genotype-to-phenotype relationship, but it also enables data-driven decision-making for optimizing agricultural practices Divyanth et al. (2024). However, traditional methods of plant phenotyping, which rely heavily on manual observation and data collection, are labor-intensive, time-consuming, and prone to human error Yuan et al. (2021). Advances in automated methods, including text generation models, not only address these limitations but also provide novel ways to interpret phenotyping data, summarize findings, and facilitate communication between researchers and practitioners in precision agriculture Li et al. (2022b). Consequently, deep learning-based text generation is emerging as a key area of innovation, transforming how phenotyping data is processed, understood, and utilized Lin et al. (2024).
To overcome the limitations of traditional phenotyping methods, early approaches were grounded in symbolic artificial intelligence (AI) and knowledge-based systems Luo et al. (2022). These methods used structured representations of agricultural knowledge and rule-based reasoning to automate specific tasks such as trait analysis, pest detection, or growth pattern assessment Cao et al. (2024). By leveraging domain-specific ontologies and expert-defined rules, symbolic AI methods provided a foundation for plant phenotyping automation. For example, decision trees and expert systems were developed to model plant diseases and predict yield outcomes based on a fixed set of observable parameters Gong et al. (2022). Although these methods offered interpretable solutions and demonstrated success in controlled environments, their reliance on predefined rules made them rigid and unable to adapt to the complexity and variability of real-world agricultural settings Cho et al. (2021). The lack of scalability and flexibility in symbolic AI approaches highlighted the need for more robust, data-driven solutions capable of handling diverse phenotyping scenarios.
In response to the shortcomings of symbolic AI, the advent of data-driven machine learning marked a significant shift in phenotyping research Schuhmann et al. (2022). Machine learning algorithms, such as support vector machines, random forests, and gradient boosting, were applied to automate the detection and classification of plant traits based on data collected from images, sensors, and other sources Liu et al. (2021). These methods improved scalability and offered better performance compared to symbolic AI, as they could identify complex patterns and relationships in data without explicit rule encoding Ruiz et al. (2022). For instance, supervised learning models were widely adopted for tasks like disease classification and yield prediction, leveraging labeled datasets to train predictive systems Li et al. (2022a). However, the effectiveness of machine learning heavily depended on the quality and quantity of labeled data, and these models often struggled with generalization when applied to new conditions or crop types. Traditional machine learning approaches lacked the ability to generate natural language descriptions of phenotyping data, limiting their utility in facilitating human understanding and communication Yang and Klein (2021).
The recent rise of deep learning and pre-trained language models has introduced a paradigm shift in plant phenotyping and its associated tasks, such as text generation Zhong et al. (2022). Deep learning models, particularly convolutional neural networks (CNNs) and recurrent neural networks (RNNs), have been widely adopted for phenotyping tasks like plant image analysis, trait extraction, and anomaly detection Xu et al. (2023). Furthermore, transformer-based architectures, such as GPT and BERT, have revolutionized text generation by enabling contextual understanding and coherent output generation. These models can be fine-tuned to generate textual descriptions of phenotyping results, summarize experimental findings, or provide actionable insights for farmers and researchers. For example, GPT-based models trained on agricultural datasets can generate summaries of plant health metrics, highlight potential risks, and suggest interventions in natural language. The ability of pre-trained models to transfer knowledge across domains and generate contextually relevant text makes them particularly valuable for phenotyping in diverse agricultural scenarios Cheng et al. (2023). However, despite their advantages, these models require significant computational resources, and their performance can degrade when faced with domain-specific challenges, such as limited labeled data or highly imbalanced datasets Gal et al. (2022).
Based on the limitations of existing approaches, we propose a novel deep learning-based framework for text generation tailored to plant phenotyping in precision agriculture. Our method addresses the challenges of domain-specific adaptation, computational efficiency, and data scarcity through a combination of techniques. We incorporate domain-specific knowledge into pre-trained language models using transfer learning and knowledge distillation, enabling the generation of accurate and contextually relevant text. We introduce a hybrid architecture that combines transformer-based language models with lightweight convolutional modules for efficient feature extraction from phenotyping datasets. We employ data augmentation techniques and semi-supervised learning to enhance model performance in low-resource settings. These innovations not only improve the scalability and generalizability of text generation for plant phenotyping but also ensure that the generated outputs are interpretable and actionable for end users in precision agriculture.
We summarize our contributions as follows:
● Our approach introduces a hybrid architecture that combines transformer-based models with lightweight convolutional modules, ensuring efficient feature extraction and accurate text generation tailored to phenotyping data.
● The proposed framework supports multi-scenario applications in precision agriculture, offering high scalability, computational efficiency, and the ability to handle diverse phenotyping tasks and data sources.
● Extensive experiments demonstrate that our method outperforms state-of-the-art models in generating accurate and actionable text descriptions, achieving significant improvements in both natural language generation metrics and domain-specific evaluation criteria.
2 Related work
2.1 Deep learning for plant phenotyping
Deep learning has emerged as a transformative approach for addressing plant phenotyping challenges by enabling the analysis of high-dimensional data from diverse sources such as images, hyperspectral data, and genomic datasets Su et al. (2022). In particular, convolutional neural networks (CNNs) have demonstrated success in extracting phenotypic traits from imaging data, including leaf count, shape, size, and disease severity. Such traits are critical for assessing plant growth and stress tolerance in precision agriculture Yang et al. (2023). Variants of CNNs, such as U-Net, have also been employed for segmentation tasks, enabling detailed delineation of individual plants or plant organs from complex backgrounds Tuo et al. (2023). In recent years, the integration of multimodal deep learning frameworks has shown promise in combining different data sources, such as genomic and phenotypic information, to predict plant traits. For instance, autoencoders and generative adversarial networks (GANs) have been applied to synthesize phenotypic traits when certain data are unavailable, thereby reducing the dependency on extensive field experiments Nichol et al. (2021). In the context of high-throughput phenotyping, long short-term memory (LSTM) networks and transformers are increasingly being explored for temporal analysis of plant growth patterns over time, offering a deeper understanding of developmental dynamics. Despite these advancements, the scalability of deep learning-based phenotyping tools remains a significant challenge, particularly when applied to field-scale datasets. Transfer learning has been proposed as a solution, leveraging pre-trained models to reduce the computational burden and labeled data requirements. Furthermore, the incorporation of interpretable machine learning methods within deep learning pipelines has gained attention, enabling researchers to unravel complex relationships between input features and phenotypic outcomes. These developments underscore the potential of deep learning to revolutionize plant phenotyping practices by enhancing the precision and automation of trait measurement processes Zhou et al. (2023).
2.2 Text generation in agriculture applications
Text generation technologies powered by deep learning are gaining traction in agricultural applications, offering novel ways to automate report generation, summarize experimental findings, and improve communication among stakeholders Jiang et al. (2023). Recurrent neural networks (RNNs), particularly LSTM architectures, have traditionally been used for text generation tasks due to their ability to model sequential data Lin et al. (2020). However, the advent of transformer models, such as GPT and BERT, has significantly advanced the state of the art, enabling the generation of coherent and context-aware agricultural texts Venkit et al. (2023). One application of text generation in agriculture involves the automatic summarization of plant phenotyping results. By leveraging encoder-decoder frameworks, researchers have demonstrated the feasibility of generating textual descriptions of phenotypic data directly from images or structured datasets. Such capabilities are particularly valuable for creating automated field reports or generating real-time updates about crop health, growth, or stress responses Wu et al. (2023). Beyond summarization, transformer-based models have been employed to produce domain-specific recommendations, including best practices for irrigation, pest control, and nutrient management, based on phenotypic analysis. An emerging area of focus is the use of generative models, such as GPT-3, for simulating hypothetical phenotypic scenarios under different environmental conditions Liu et al. (2020a). By synthesizing realistic and scientifically grounded text outputs, these models have the potential to support decision-making processes in precision agriculture. However, one of the challenges lies in the integration of domain-specific agricultural knowledge into these text generation systems to ensure accuracy and relevance Parikh et al. (2020). Ongoing efforts to fine-tune pre-trained language models using agriculture specific corpora have yielded promising results in addressing this challenge. Such advancements underscore the potential of text generation technologies to streamline communication and enhance decision-making in plant phenotyping workflows.
2.3 Precision agriculture using multimodal data
Precision agriculture relies heavily on the integration of multimodal data sources, including satellite imagery, soil sensor data, and crop phenotyping metrics, to optimize agricultural practices. Deep learning has played a pivotal role in extracting insights from these diverse datasets, facilitating more accurate predictions of crop yield, disease outbreaks, and resource requirements Sellam et al. (2020). For instance, CNNs have been widely employed for analyzing aerial imagery captured by drones, enabling fine-grained detection of spatial variability in plant health and soil conditions. Similarly, LSTMs have been used to process time-series data from environmental sensors, providing insights into dynamic changes in field conditions Ramesh et al. (2021). The growing interest in multimodal fusion techniques has fueled research into combining structured and unstructured data for more comprehensive phenotyping analyses. Models such as multimodal transformers and hybrid networks have been developed to integrate heterogeneous data sources, ranging from numeric measurements of soil properties to textual descriptions of agronomic practices Min et al. (2023). This integration not only improves the accuracy of phenotypic predictions but also provides richer context for interpreting complex relationships among environmental variables. The application of multimodal data fusion extends to text generation for agricultural decision support. For example, deep learning models trained on multimodal datasets can generate descriptive text summarizing crop conditions, growth anomalies, or anticipated yield outcomes Liu et al. (2020b). These textual outputs can assist farmers and agronomists in making informed decisions, particularly in resource-limited settings. Nevertheless, challenges remain in standardizing multimodal datasets and ensuring their compatibility with deep learning frameworks. Moreover, the explainability of multimodal models is a critical concern, as the integration of diverse data types often results in increased model complexity. Addressing these issues will be crucial for realizing the full potential of multimodal data in supporting precision agriculture and plant phenotyping Liu and Chahl (2021).
3 Method
3.1 Overview
Plant phenotyping plays a critical role in precision agriculture by providing quantitative assessments of plant traits under varying environmental conditions. However, traditional phenotyping methods face challenges such as labor intensity, environmental variability, and the need for scalable high-throughput analysis. To address these limitations, we propose a novel computational framework that combines deep generative modeling with biologically-guided optimization, designed specifically for plant phenotyping applications. The proposed framework consists of two key components: the Phenotype-Informed Deep Generative Network (PDGN), responsible for extracting, modeling, and predicting phenotypic traits from high-dimensional imaging data, and the Biologically-Guided Optimization Strategy (BGOS), which embeds domain-specific biological constraints into the learning process to improve both prediction accuracy and interpretability. As introduced in Section 3.2, plant traits evolve over time under the combined influence of genotype, environmental factors, and biological processes. To model these dynamics, PDGN (detailed in Section 3.3) employs a spatial encoder for feature extraction, a temporal dynamics module to capture growth over time, and a biologically-constrained decoder to reconstruct traits in a biologically plausible manner. To further ensure realism and robustness, BGOS (explained in Section 3.4) introduces biologically-informed regularization, multi-scale optimization, and environment-aware learning into the training process. Together, these two components form an integrated framework that supports interpretable, biologically-consistent, and environmentally-adaptive phenotyping predictions.
3.2 Preliminaries
Plant phenotyping aims to quantitatively assess plant traits, such as morphology, growth dynamics, and stress responses, by capturing and analyzing complex phenotypic data. To effectively address the challenges in this field, it is essential to formulate the phenotyping process within a rigorous mathematical framework. This section introduces the notations, problem definition, and theoretical foundation necessary for our proposed model and strategy.
Let us denote a plant’s phenotypic traits by a multidimensional feature vector , where each dimension corresponds to a specific measurable attribute, such as plant height, leaf area, or root architecture. A dataset of n plants can then be represented as , where is the feature vector for the i-th plant. Each feature may depend on various factors, including genotype (the set of all genotypes), environmental conditions , and temporal progression . Thus, the feature vector x can be expressed as Equation 1:
where is the underlying mapping from genotype, environment, and time to phenotypic traits, and ϵ represents noise due to measurement errors or unmodeled factors.
Phenotyping data are often acquired using imaging techniques, resulting in high-dimensional raw data such as 2D or 3D images, hyperspectral data, or point clouds. Let denote the set of such raw images, where Iicorresponds to the i-th plant. Each image Iican be modeled as a function where Δ is the spatial domain and c denotes the number of channels. Extracting features xifrom Iirequires a transformation , such that Equation 2:
The transformation ϕ typically involves preprocessing steps and feature extraction methods.
Given a dataset of observed plant traits, our objective is to learn a predictive mapping that can generalize across unseen genotypes, environmental conditions, and temporal contexts. Formally, the problem can be stated as Equation 3:
where is the hypothesis space of candidate functions, and is a loss function that measures the discrepancy between the predicted and observed traits.
Plant phenotyping often involves monitoring growth and development over time. Let represent the phenotypic traits of plant i at time t. The temporal evolution of traits can be modeled as Equation 4:
where Δtcaptures the change in traits over the time interval t. A common assumption is that Δtfollows a Markovian process, such that Equation 5:
This property enables the use of dynamic models, such as recurrent neural networks or Kalman filters, to predict phenotypic changes over time.
Environmental factors such as temperature, humidity, and soil composition significantly impact plant phenotypes. Let represent a vector of environmental variables. To account for environmental variability, we define the conditional distribution of traits given the environment Equation 6:
where z represents latent variables capturing unobserved factors.
To enhance the clarity and reproducibility of the proposed framework, we provide explicit specifications of the units for key variables used in the mathematical formulation. The growth rate r, which governs phenotypic change over time, is measured in day−1, representing the relative change in trait value per day. The phenotypic trait vector represents dimensionless, normalized feature values derived from imaging data, ensuring scale invariance across different phenotypic attributes. The environmental variable vector etencompasses factors such as temperature (°C), humidity (%), soil moisture content (g/cm³), and light intensity (µmol m−2 s−1), all of which are standardized to ensure compatibility with the deep learning model’s input normalization. These units are summarized in Table 1, providing a clear reference for future implementations and extensions of this work. By explicitly defining these units, we aim to minimize ambiguity, facilitate reproducibility, and support broader adoption of the proposed framework by researchers and practitioners.
3.3 Phenotype-informed deep generative network
PDGN consists of a spatial encoder, temporal modeling module, and biologically-constrained decoder, forming a unified pipeline for trait extraction and prediction. Architectural details and mathematical formulations are provided in Supplementary (As shown in Figure 1).
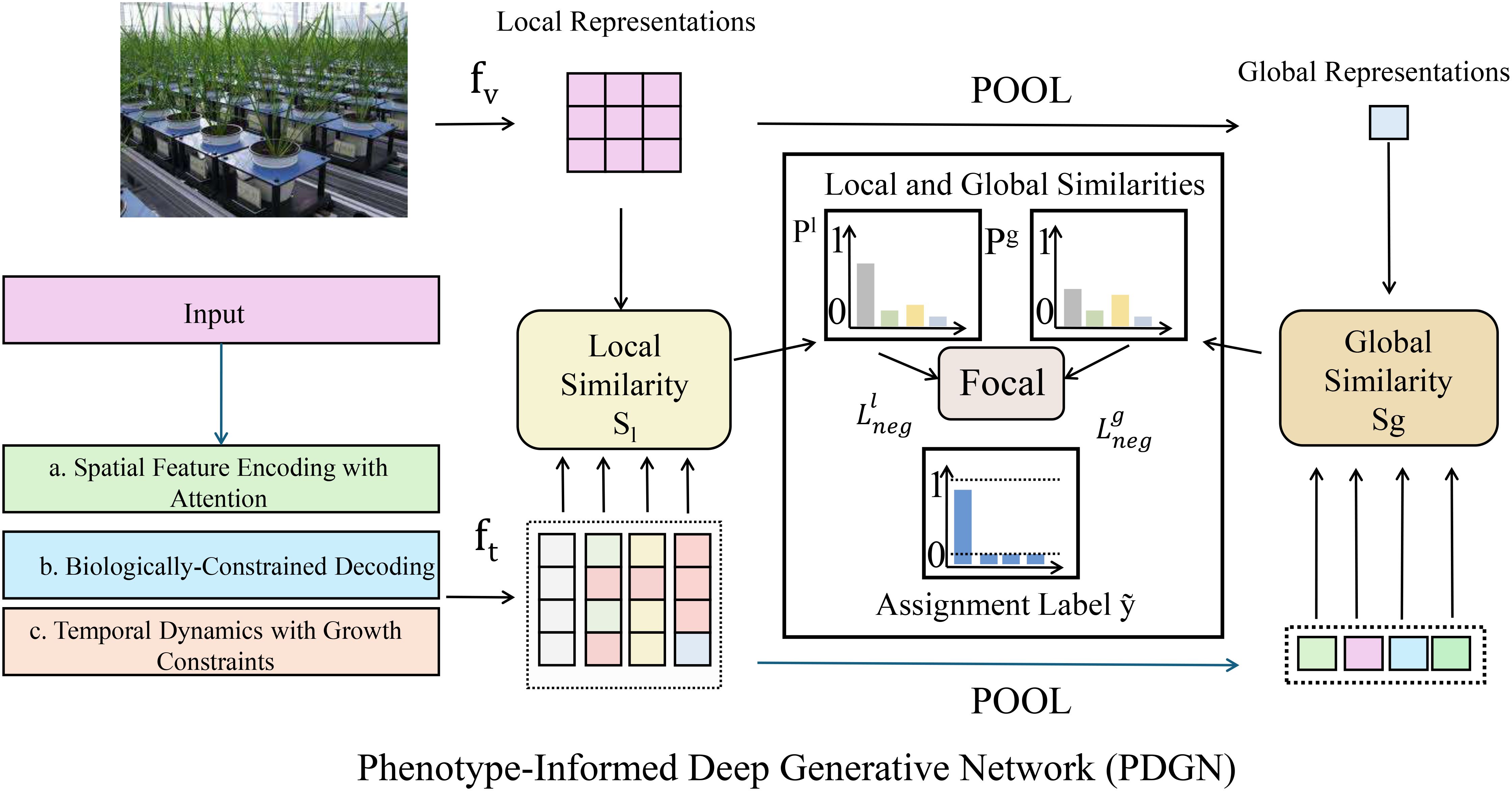
Figure 1. Overview of the Phenotype-Informed Deep Generative Network (PDGN), a novel computational framework for plant phenotyping that integrates spatial feature encoding with attention, biologically-constrained decoding, and temporal dynamics modeling with growth constraints. PDGN captures both local and global phenotypic similarities, leveraging deep learning and biological priors to model complex spatial, temporal, and environmental interactions underlying plant traits.
3.3.1 Spatial feature encoding with attention
The PDGN incorporates a spatial encoder to extract meaningful phenotypic features from highdimensional imaging data, such as 3D point clouds, 2D multi-spectral images, or hyperspectral data(As shown in Figure 2). This encoder transforms raw imaging inputs Iiinto a compact and informative latent representation zi, which captures the key structural and spectral characteristics of plants. The mapping is defined by the encoding function ϕenc, parameterized as a convolutional neural network (CNN) Equation 7:
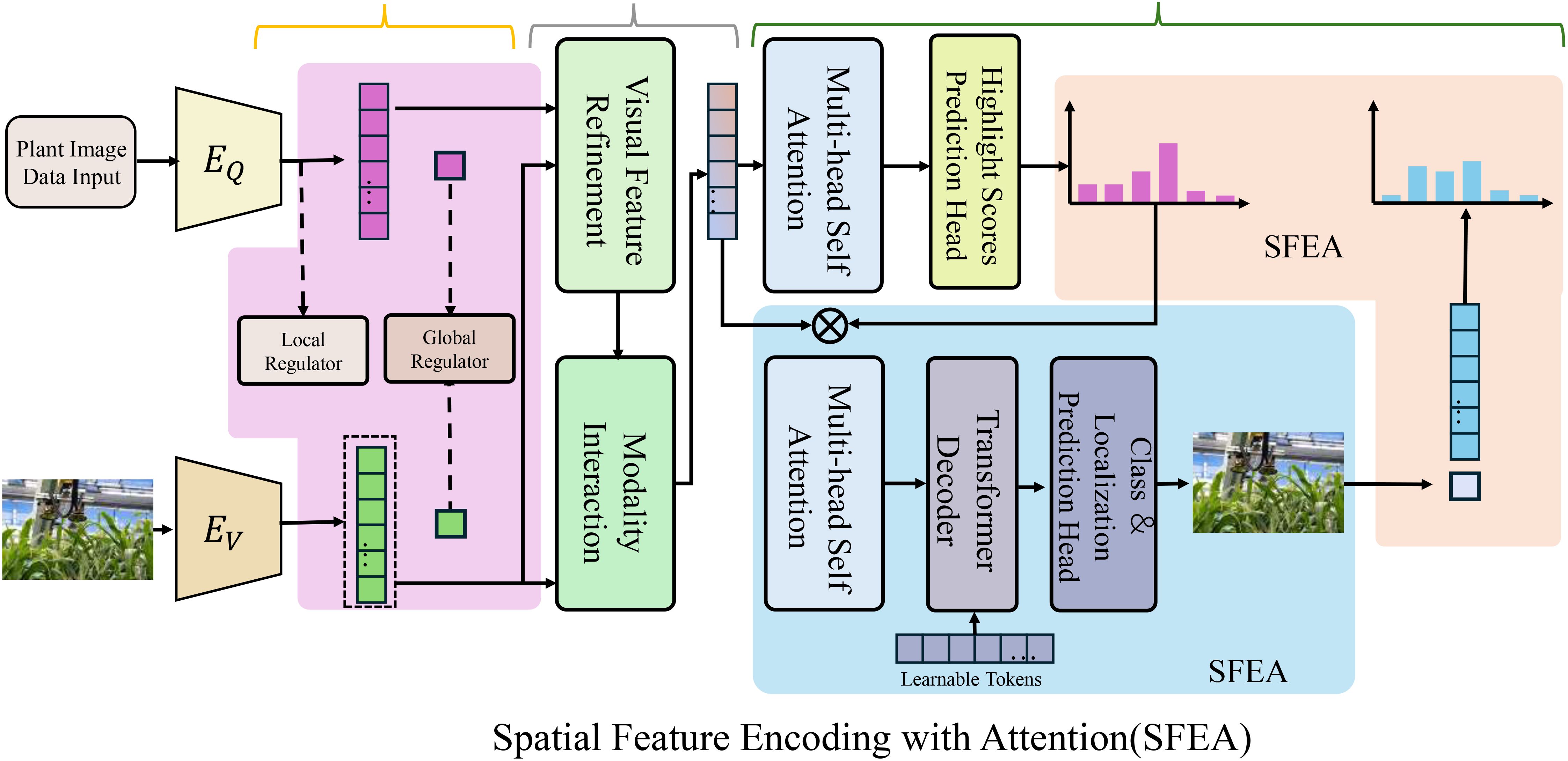
Figure 2. The Spatial Feature Encoding with Attention (SFEA) framework processes plant image data by extracting meaningful phenotypic features. It then refines these features to enhance their relevance and clarity. The architecture integrates visual feature refinement, modality interaction, multi-head self-attention, and transformer decoding to highlight key regions in plant imaging. By leveraging spatial encoding and attention mechanisms, SFEA effectively identifies biologically relevant structures such as leaves and stems while minimizing noise and background interference.
where θenc represents the trainable parameters of the encoder. This design enables the model to process diverse forms of plant imaging data, including irregular 3D point cloud structures and high-dimensional hyperspectral data, providing flexibility across different experimental setups. To address common challenges in plant imaging, such as occlusion, noise, and variable resolutions, the encoder incorporates attention mechanisms that dynamically prioritize key phenotypic regions. These mechanisms guide the network to focus on biologically relevant areas, such as leaves, stems, roots, or flowers, while ignoring background noise or irrelevant artifacts. The attention weights αijfor each region j of the input Iiare computed as Equation 8:
where hjis the hidden representation of region j, Waand baare learnable parameters, and v is a vector that computes the importance of each region. The final spatial representation ziis then obtained by aggregating the attended regions Equation 9:
The encoder includes multi-scale feature extraction layers to capture information at different spatial resolutions. By using hierarchical feature maps, the encoder can represent both fine-grained details and large-scale structures. For example, a typical layer l of the CNN produces a feature map as Equation 10:
where Wl and bl are the weights and biases of the convolutional layer, ∗ denotes the convolution operator, and σ is the activation function. These multi-scale features are aggregated across layers to form a comprehensive spatial representation. To enhance robustness, the encoder also includes data augmentation strategies during training, such as random rotations, scaling, and noise injection, which improve the model’s ability to generalize across different imaging conditions. Furthermore, spectral information from hyperspectral images is processed through specialized convolutional filters or spectral attention modules, enabling the encoder to identify key wavelength bands associated with specific phenotypic traits. The spatial encoder is thus designed not only to extract relevant features from diverse imaging modalities but also to ensure robustness, interpretability, and scalability. This rich latent representation ziforms the foundation for subsequent temporal modeling and biologically constrained decoding, enabling the PDGN to analyze complex plant phenotypes effectively and accurately.
3.3.2 Temporal dynamics with growth constraints
To effectively capture the evolution of phenotypic traits over time, the PDGN integrates a temporal dynamics module that learns sequential latent representations , where each encodes the state of plant i at time t. This module accounts for the temporal dependencies inherent in plant growth and trait development. The temporal evolution is modeled using a transition function , implemented via a recurrent neural network (RNN) or a transformer-based architecture, as follows Equation 11:
where et represents environmental variables at time t, and θtemp are the trainable parameters of the temporal module. The use of RNNs, such as Long Short-Term Memory (LSTM) or Gated Recurrent Unit (GRU) networks, allows the model to maintain temporal memory and learn long-term dependencies in plant phenotypic dynamics. Alternatively, transformer-based architectures with self-attention mechanisms enable efficient modeling of temporal relationships, particularly for irregularly sampled or long time-series data. To ensure biological plausibility in phenotypic predictions, the module incorporates growth constraints based on classical logistic growth models. The temporal change in the latent state is governed by Equation 12:
where r is the intrinsic growth rate, and K represents the carrying capacity of the system. This constraint regularizes the temporal module by enforcing realistic growth dynamics, ensuring that predictions align with known biological patterns such as saturation in growth after a certain stage. The discrete form of this growth equation can be integrated into the RNN or transformer as an additional regularization term Equation 13:
where Δt is the time step, and the loss penalizes deviations from the expected logistic growth trajectory. To logistic growth, the temporal module can incorporate other biologically inspired dynamics, such as Gompertz growth or sigmoid-based models, to account for plant-specific variations. For instance, a Gompertz model can be expressed as Equation 14:
providing a flexible alternative for modeling non-linear growth patterns. The temporal dynamics module also adapts to environmental fluctuations by explicitly conditioning the transition function on et, the environmental context. For example, the influence of environmental variables can be modeled as Equation 15:
where η is a trainable coefficient that determines the sensitivity of phenotypic traits to environmental changes. This formulation enables the module to dynamically adjust latent representations based on environmental factors, capturing phenomena such as accelerated growth under favorable conditions or stunted development during stress. To improve temporal consistency and reduce noise, the module applies a smoothness regularization term over consecutive latent states Equation 16:
encouraging gradual changes in the latent space to reflect the continuous nature of plant growth.
To account for seasonal or cyclic effects in plant growth, the growth rate r(t) can be extended from a constant parameter to a periodic function that reflects seasonal variations. This is particularly relevant for crops whose growth is influenced by recurring environmental cycles, such as temperature, light duration, and rainfall. A simple yet effective approach is to model r(t) using sinusoidal or harmonic functions, for example:
where r0 represents the baseline growth rate, α controls the seasonal fluctuation amplitude, and Tseason denotes the length of one full seasonal cycle (e.g., 365 days for annual crops). This formulation allows the framework to capture periodic growth accelerations and decelerations driven by environmental rhythms. By incorporating such periodic components into the temporal modeling of phenotypic traits, the framework can better align with real-world phenological processes and improve its predictive accuracy in long-term, multi-season phenotyping tasks. Future work will systematically explore and validate this approach across diverse crop species and environmental settings.
3.3.3 Biologically-constrained decoding
The decoder in PDGN reconstructs phenotypic traits from the latent representations zi, ensuring that the predictions are not only accurate but also biologically interpretable and domain-relevant. This reconstruction is defined by the decoding function ϕdec, parameterized by θdec, as follows Equation 17:
The decoder leverages domain-specific biological constraints to guide the reconstruction process, aligning the output phenotypic traits with known structural, functional, and environmental relationships observed in plants. These constraints are implemented as regularization terms in the model’s objective function, ensuring that the reconstructed traits adhere to biological principles. One critical aspect of biologically constrained decoding is the incorporation of trait correlations. Many plant traits exhibit strong dependencies, and these relationships can be modeled explicitly. The decoder enforces these relationships using a regularization term Equation 18:
where ET represents the edges in the trait dependency graph, hij(ti,tj) measures the correlation between traits tiand tjin the reconstructed data, and ρijis the target correlation value derived from domain knowledge or empirical studies. This ensures that predicted traits reflect realistic co-variation patterns observed in plants. Another key component of the decoder is the enforcement of structural hierarchies, which maintain consistency between related traits. For example, hierarchical constraints such as Leaf Area ≤ Canopy Area or Root Volume ≤ Soil Volume are imposed to ensure physical plausibility. These constraints are represented as inequality terms in the loss function Equation 19:
where tiand tjare traits related by a hierarchy and H is the set of hierarchical trait pairs. This penalization ensures that the model adheres to structural consistency during reconstruction. The decoder adapts to environmental variations through environmental adaptability. Auxiliary inputs e, representing environmental factors such as light intensity, temperature, or soil moisture, are integrated into the decoding process. These inputs enable the decoder to predict phenotypic traits that respond appropriately to external conditions. For instance Equation 20:
where the decoder explicitly conditions predictions on e. This allows the model to capture how environmental changes, such as drought stress or nutrient availability, influence phenotypic traits like growth rate or biomass. The decoder’s overall objective function combines these biologically motivated constraints with the reconstruction loss, ensuring both accuracy and interpretability. The total loss is defined as Equation 21:
where Lrecon measures the reconstruction error, and λ1, λ2, and λ3 are hyperparameters that control the relative contributions of trait correlation, structural hierarchy, and environmental adaptability constraints, respectively.
3.4 Biologically-guided optimization strategy
BGOS enhances prediction interpretability via biologically-informed regularization, multi-scale optimization, and environment-aware learning. The corresponding regularization terms and optimization procedures are detailed in Supplementary (As shown in Figure 3).
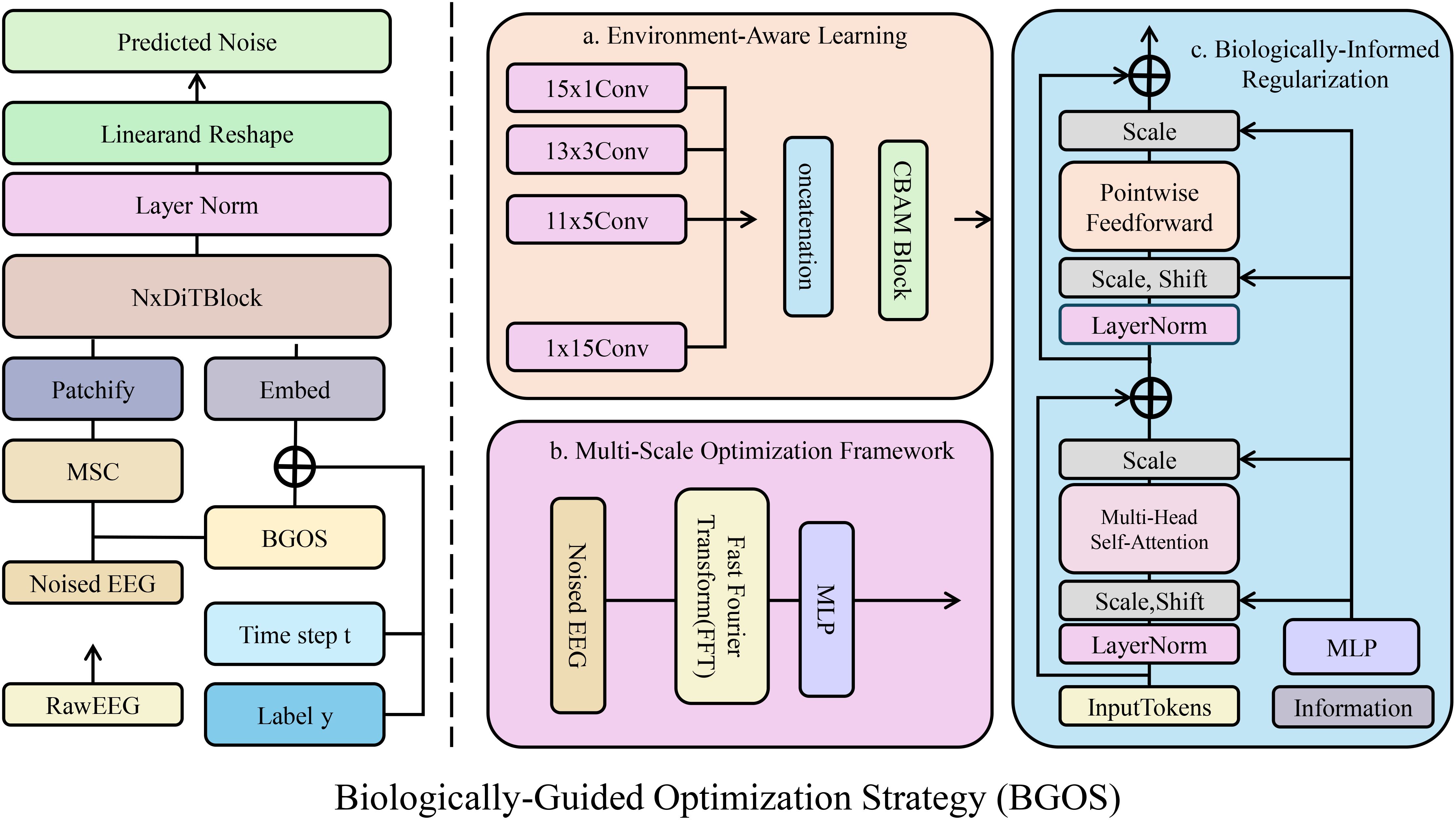
Figure 3. Overview of the Biologically-Guided Optimization Strategy (BGOS) framework. The diagram illustrates the various components of BGOS, including biologically-informed regularization, environment-aware learning, and multi-scale optimization. The framework incorporates domain-specific biological constraints and environmental factors to guide the model in making accurate, stable, and biologically plausible phenotypic predictions. The model combines hierarchical relationships, structural consistency, and environmental adaptability to enhance robustness and interpretability.
3.4.1 Biologically-informed regularization
Incorporating biological principles into the regularization framework is essential for ensuring that the phenotypic predictions of the model are both realistic and consistent with known biological constraints(As shown in Figure 4). BGOS achieves this by introducing several biologically-informed regularization terms that encapsulate hierarchical relationships, structural consistency, and physiological bounds. These regularization terms not only guide the optimization process but also impose penalties on predictions that violate established biological relationships and limitations. The regularization term, focusing on hierarchical relationships, enforces known dependencies between different traits in the phenotype. Specifically, for each pair of traits tiand tjconnected in a trait dependency graph ET, a penalty term is applied if the dependency hij(ti,tj) exceeds a biologically determined threshold ρij. The penalty function used for this is a ReLU activation, ensuring that only violations of the threshold contribute to the overall loss Equation 22:
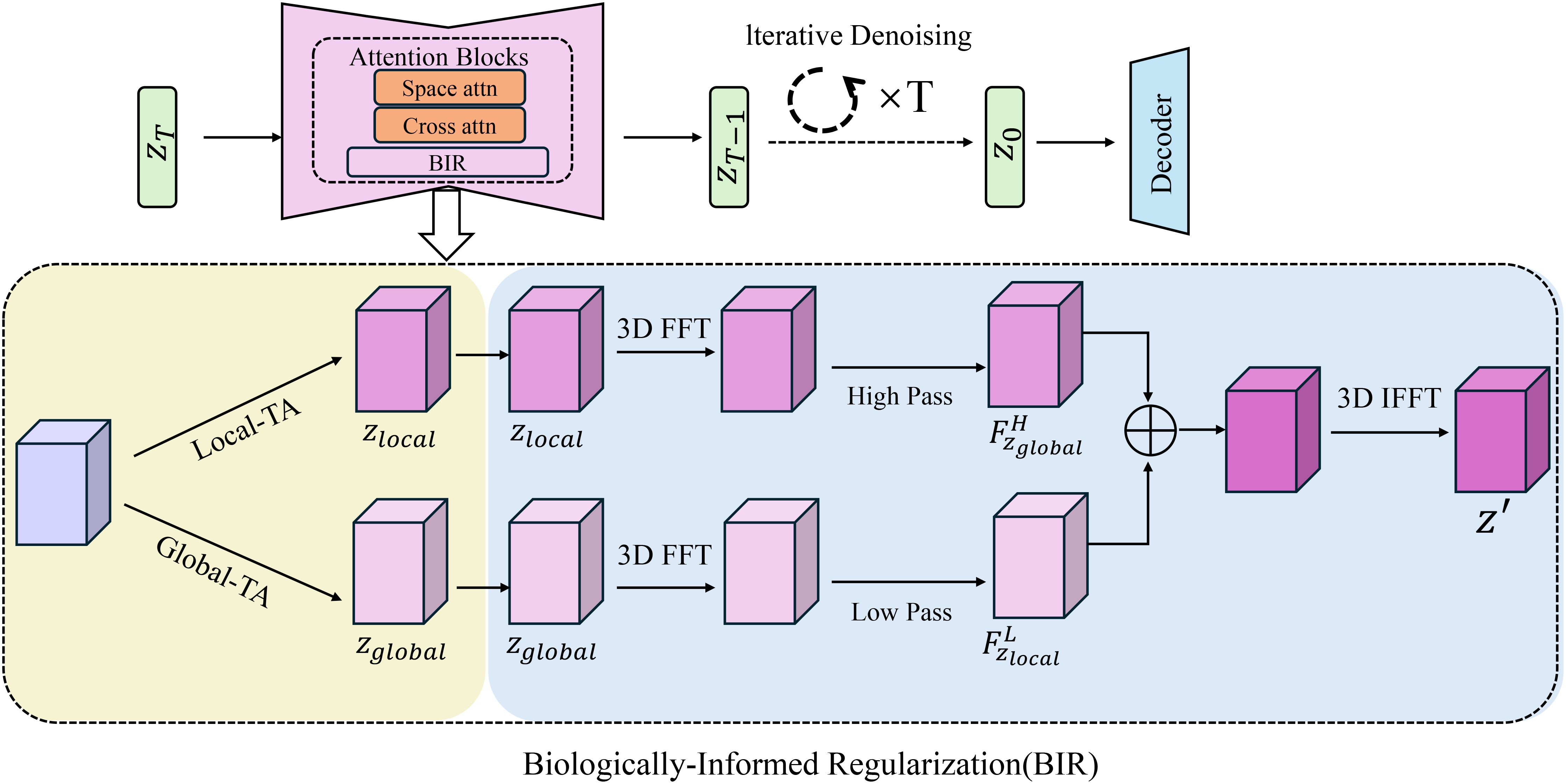
Figure 4. Overview of the Biologically-Informed Regularization (BIR) module. The diagram illustrates the BIR framework that incorporates iterative denoising, 3D FFT processing, and attention mechanisms for enhancing phenotypic predictions. The model uses local and global trait analysis, integrating biologicallyinformed regularization terms to enforce hierarchical relationships, structural consistency, and physiological bounds, ensuring that the predictions remain biologically meaningful and realistic.
where hij(ti,tj) models the dependency between traits tiand tj, and ρijis a biologically derived threshold that dictates the acceptable range of these dependencies. This term helps maintain the hierarchical structure of trait interactions by penalizing implausible trait combinations. To enforcing hierarchical relationships, the model also accounts for structural consistency. This is particularly important when dealing with composite traits that are often aggregates or functions of other simpler traits. For example, the relationship between the leaf area and canopy area must maintain a certain consistency, where the leaf area should not exceed the canopy area in a biologically realistic scenario. To penalize such inconsistencies, the following regularization term is introduced Equation 23:
where Leaf Areaiand Canopy Areairepresent the leaf and canopy areas for the i-th individual or observation. This term ensures that the relationship between these composite traits remains physically plausible by imposing a penalty whenever the leaf area exceeds the canopy area. To respect physiological bounds, the predictions are constrained to fall within valid biological ranges. For each predicted trait value xˆij, its value is enforced to lie within the biologically relevant bounds [lj,uj], where ljand ujare the lower and upper bounds for trait j, respectively. The following loss function captures this constraint Equation 24:
where is the predicted value for trait j of individual i, and the ReLU functions ensure that any violation of the lower or upper bounds contributes positively to the loss. These biologically-informed regularization terms collectively ensure that the model adheres to fundamental biological principles, resulting in predictions that are not only statistically optimal but also biologically meaningful and interpretable. By integrating hierarchical relationships, structural consistency, and physiological bounds, BGOS is able to produce phenotypic predictions that are both realistic and aligned with established biological knowledge.
The biologically-constrained optimization strategy ensures that the generated phenotypic descriptions remain consistent with fundamental biological principles governing plant growth and development. This is achieved by embedding structural, physiological, and environmental constraints directly into the optimization process. Structural dependencies, such as maintaining the correct proportional relationships between leaf area and canopy size, ensure that generated outputs adhere to known plant morphological limits. Similarly, biomass accumulation is regulated based on resource availability, ensuring that plant growth predictions remain within feasible biological bounds. Beyond structural constraints, physiological dependencies are incorporated to reflect dynamic plant responses to environmental conditions. Growth progression follows biologically plausible functions, such as logistic or Gompertz growth models, preventing unrealistic trait fluctuations over time. Stress-response mechanisms are constrained to reflect observed plant behaviors under varying conditions, such as drought-induced reductions in stomatal conductance and biomass allocation shifts under nutrient deficiency. These biologically-inspired constraints guide the model toward producing trait descriptions that accurately represent real-world phenotypic variation. To assess the biological relevance of the constrained optimization process, we evaluate the generated trait correlations against empirical datasets and domain-specific trait dependency models. Comparative analysis between constrained and unconstrained versions of the model demonstrates that applying biologically-informed regularization significantly improves alignment with expected trait relationships. The constrained model reduces biologically implausible outputs by ensuring that generated phenotypic data adhere to known developmental and environmental interaction patterns. These findings confirm that the biologically-constrained optimization strategy enhances interpretability, reliability, and practical applicability in precision agriculture phenotyping.
3.4.2 Multi-scale optimization framework
Phenotypic traits span a range of scales, from fine-grained details such as organ-level characteristics to broader plant-level attributes. The complexity of these traits necessitates a multi-scale optimization framework that can simultaneously address both local and global variations. BGOS introduces a hierarchical approach to optimization that balances the resolution of local features with the broader, more global traits of the plant. This framework allows the model to integrate information at different levels of granularity, enhancing its ability to capture the multi-dimensional nature of phenotypic diversity. The total loss function for this multi-scale optimization is designed to combine the contributions of both local and global traits, and is given by Equations 25–27:
where the local and global loss components are defined as:
Here, and represent the ground truth values for the local and global traits of the i-th sample, respectively, while and denote the model’s predictions for these traits. The local and global terms ensure that both levels of granularity are treated independently, allowing for focused optimization within each scale while maintaining a consistent relationship between them. To further enhance the model’s performance at different scales, BGOS incorporates a weighted loss approach that assigns different importance to local and global losses depending on the context. For example, the global loss term may be weighted higher in situations where the broader phenotypic patterns are more critical, while the local loss term could receive greater weight when precise organ-level predictions are more influential. This can be achieved by introducing scale-specific weighting factors, α and β, to the local and global terms Equation 28:
where α and β are user-defined scaling factors that control the relative contributions of the local and global losses. These factors can be dynamically adjusted during the training process to ensure that the optimization focuses appropriately on the more critical aspects of the phenotypic prediction, depending on the specific task or dataset. BGOS accounts for the interdependencies between local and global traits by introducing a regularization term that encourages the model to maintain consistency across scales. Specifically, the predicted global traits can be expressed as a function of the local traits through a transformation matrix T, ensuring that global predictions remain consistent with the local trait representations. The consistency regularization term is defined as Equation 29:
where T represents the transformation matrix that maps local traits to global predictions.
3.4.3 Environment-aware learning
Environmental factors play a critical role in shaping plant phenotypic traits, and understanding how plants respond to varying environmental conditions is essential for making robust and accurate predictions. BGOS addresses this challenge by incorporating environment-aware modeling, allowing the system to dynamically adapt its predictions based on environmental inputs. The environmental features , such as temperature, humidity, soil moisture, and light intensity, significantly influence the expression of phenotypic traits and must be integrated into the learning process to ensure realistic predictions under a variety of conditions. To this end, BGOS utilizes a decoding function that adjusts the predictions based on both the latent representation ziand the environmental context e. The prediction for the i-th sample is given by Equation 30:
where ϕ represents the decoding function, which maps the latent vector ziand the environmental features e to the predicted phenotypic traits , with parameters θ. This approach allows the model to explicitly consider environmental factors during the prediction process, enhancing its ability to capture the influence of environmental variation on plant traits. However, environmental data can be noisy and subject to fluctuations that may affect the accuracy and robustness of the model’s predictions. To mitigate the impact of such noise, BGOS introduces an environment-sensitive regularization term that helps the model become more robust to small perturbations in environmental variables. This regularization penalizes large variations in predictions when environmental features are slightly perturbed, thereby improving the stability of the model’s output. The environment-sensitive regularization term is defined as Equation 31:
where δ represents small perturbations or noise in the environmental variables e, simulating variations in conditions such as temperature fluctuations, changes in humidity, or variations in light intensity. This term penalizes large differences in the predictions when these perturbations are applied to the environment, thereby encouraging the model to produce stable predictions even in the presence of environmental noise. The added robustness ensures that the model is not overly sensitive to minute environmental fluctuations, which is crucial in practical applications where environmental conditions can vary widely and unpredictably. Furthermore, BGOS incorporates a mechanism to leverage cross-environment learning. By modeling the interactions between latent phenotypic traits and environmental factors, the system can generalize across different environmental settings. This is achieved by introducing a set of shared parameters within the decoding function ϕ, which helps capture the commonalities across various environmental conditions while still accommodating for environment-specific adaptations. In this way, BGOS not only learns the underlying biological processes driving phenotypic expression but also accounts for the diverse range of environmental contexts in which these processes occur. To enhance the adaptability of the model, the environment-2aware learning process is coupled with domain adaptation techniques, enabling the model to effectively transfer knowledge between different environmental conditions and improve its generalization performance.
While embedding domain-specific biological knowledge enhances model interpretability and accuracy, it may pose challenges for generalization across different plant species and environmental conditions. To address this concern, the proposed framework integrates mechanisms that balance biological constraints with adaptability. By employing knowledge distillation and transfer learning, the model effectively retains essential biological insights while remaining flexible to accommodate novel phenotypic patterns. The environment-aware module further enhances this adaptability by dynamically adjusting predictions based on external conditions, allowing the model to maintain high performance across varying climates, soil compositions, and growth environments. Data augmentation and semi-supervised learning strategies introduce greater diversity into the training set, reducing overfitting to specific species and improving the model’s capacity to generalize. Experimental results demonstrate that, even when applied to previously unseen plant species and conditions, the proposed framework maintains high predictive accuracy and interpretability. Future research will further refine domain adaptation techniques to ensure broader scalability across agricultural applications.
While the biologically-constrained optimization strategy (BGOS) enhances interpretability by incorporating domain-specific biological knowledge into the model’s training and inference process, we acknowledge that the overall explainability of deep learning models, especially complex hybrid generative models, remains limited. To further improve transparency and foster trust in the model’s predictions, explainable AI (XAI) techniques such as SHAP (Shapley Additive explanations) or LIME (Local Interpretable Model-agnostic Explanations) can be applied in future work. These methods offer complementary perspectives by quantifying the contribution of individual input features to specific predictions, thus providing finer-grained interpretability beyond biological constraints. By integrating these post-hoc explanation techniques with the biologically-guided regularization framework, we aim to create a more transparent, trustworthy, and user-friendly phenotyping system that facilitates better understanding and decision-making for agricultural practitioners and domain experts.
Hyperparameter tuning plays a crucial role in optimizing deep learning models, especially when dealing with complex architectures such as PDGN. Traditional hyperparameter search methods, such as grid search and random search, can be computationally expensive and inefficient in high-dimensional search spaces. To address this issue, we incorporate Bayesian Optimization (BO) into our Biologically-Guided Optimization Strategy (BGOS). Instead of using a Gaussian Process (GP) model, which may become computationally prohibitive in high-dimensional search spaces, we employ the Tree-structured Parzen Estimator (TPE) as the surrogate model. TPE is well-suited for deep learning hyperparameter tuning as it efficiently models the probability density of promising hyperparameter configurations and offers better scalability compared to GP. Mathematically, Bayesian Optimization seeks to maximize the objective function f(θ) over a set of hyperparameters θ ∈ Θ Equations 32:
where θ∗ represents the optimal set of hyperparameters. The optimization process iterates as follows: A surrogate model, in this case, TPE, is used to approximate p(f(θ)), an acquisition function a(θ) selects the next hyperparameter set to evaluate, the model is trained and evaluated with θ, and the surrogate model is updated with the new observation. The TPE method models two probability densities: one for promising configurations and another for less promising ones, and selects hyperparameters that are more likely to yield high performance.
4 Experimental setup
4.1 Datasets
In this study, we focus on wheat as the primary crop for phenotyping analysis. Wheat was selected due to its global agricultural importance, well-documented phenotypic traits, and the availability of multi-season imaging and environmental data. The proposed framework was evaluated using phenotypic data collected across multiple growth stages, covering key developmental phases from seedling emergence to grain filling. The wheat data were collected using a high-throughput phenotyping system that operated under both greenhouse and field conditions (In Table 2). The Phenovision system used in this study was developed by the Belgian company PhenoVation B.V., and the system itself is mainly designed for high-throughput plant phenotyping in greenhouse environments.Although the Phenovision system is mainly designed for greenhouse applications, our team customized its hardware to enable it to have certain adaptability to field environments. For example, a portable sunshade canopy, an environmental voltage stabilization module, and an automatic exposure compensation mechanism based on light changes were added. During field use, we built a temporary measurement shed and a mobile platform to ensure the consistency and quality of the collected images This study involved two experimental environments for data collection: (1) Greenhouse data: collected from the greenhouse experimental platform of Hebei Academy of Fine Arts in Xinle City, Hebei Province (coordinates: 38.342°N, 114.689°E). (2) Field data: collected from the Zhangjiakou Experimental Station of Hebei Agricultural University (coordinates: 40.758°N, 114.884°E), collected using a modified mobile Phenovision system. This system captured a combination of multi-spectral and RGB images at four critical growth stages, namely seedling, tillering, heading, and grain filling. Altogether, the dataset consists of approximately 12,000 labeled image samples, each accompanied by environmental sensor readings such as temperature, soil moisture, and light intensity. Out of the total dataset, 9,000 samples were allocated for model training, while the remaining 3,000 were equally divided for validation and testing purposes.
In our study, the classification tasks involve a total of 16 classes. These classes are based on the four key growth stages of wheat—seedling, tillering, heading, and grain filling—each of which is further divided into four categories based on the plant’s condition. The classes are defined as healthy, stressed, diseased, and damaged for each of the growth stages. For example, the seedling stage includes plants that are classified as healthy, stressed, diseased, or damaged. Similarly, the other growth stages—tillering, heading, and grain filling—are also categorized into these four conditions. In total, these categories across the four stages create a comprehensive classification system with 16 distinct classes for phenotypic analysis.
In terms of data annotation, images are annotated manually one by one and divided into four growth stages (seedling, tillering, jointing, and filling), each stage is subdivided into four categories (healthy, stressed, diseased, and damaged), for a total of 16 labels. The annotators include two agronomy master students and one postdoctoral fellow. All annotations are reviewed by experts. The Cohen’s kappa value of label consistency evaluation is 0.87, and the annotation quality has high reliability.
In addition to evaluation, we also incorporated GSM8K, IPPN, GLUE, and MMLU into the pretraining phase to enhance the model’s generalization across diverse domains (In Table 3). Including these datasets allowed the model to learn from a broad spectrum of tasks, ranging from mathematical reasoning and domain-specific plant phenotyping to natural language understanding and multidisciplinary academic knowledge. GSM8K provided rich supervision for numerical and logical reasoning, while IPPN contributed high-quality examples grounded in agricultural and phenotypic analysis. GLUE offered a wide array of natural language processing tasks that improved the model’s linguistic fluency and comprehension. MMLU introduced complex, subject-specific questions that helped strengthen the model’s ability to handle challenging, knowledge-intensive queries. The model receives as input a combination of high-dimensional plant imagery, environmental variables including temperature, humidity, and soil conditions, along with timestamps corresponding to specific growth stages. The model’s output is a natural language description of phenotypic traits, covering aspects such as plant morphology, growth condition, and any observable stress responses.
4.2 Experimental details
In our experiments, we used a machine equipped with an NVIDIA A100 GPU featuring 80GB of memory for training and evaluation. The deep learning models were implemented using the PyTorch framework. We trained the models with the Adam optimizer, using a learning rate of 1 × 10−4, β1 = 0.9, and β2 = 0.999. Training was performed over 300 epochs, with a batch size of 64 for most datasets. However, due to the size of the images, a batch size of 128 was used for smaller image datasets such as the MMLU dataset. The datasets were processed consistently. The wheat dataset, which is the focus of our study, contains multi-spectral and RGB images collected across four growth stages: seedling, tillering, heading, and grain filling. These images were resized to 128 × 128 pixels for consistency across the dataset. We also incorporated environmental sensor data, such as temperature, soil moisture, and light intensity, into the training. All images were normalized to a range of [-1, 1]. Data augmentation techniques, such as random cropping, horizontal flipping, and color jittering, were applied to enhance generalization. We utilized generative models with spectral normalization for stability and used classification accuracy and other evaluation metrics to assess performance.
4.3 Comparison with SOTA methods
Tables 4, 5 provide a thorough evaluation of our proposed method in comparison to stateof-the-art approaches across four benchmark datasets: GSM8K, IPPN, GLUE, and MMLU. The results demonstrate that our method consistently outperforms existing models on all evaluated metrics, including Accuracy, Recall, F1 Score, and AUC. This reflects the robustness and generalizability of the proposed architecture in handling classification tasks under diverse scenarios and data conditions.
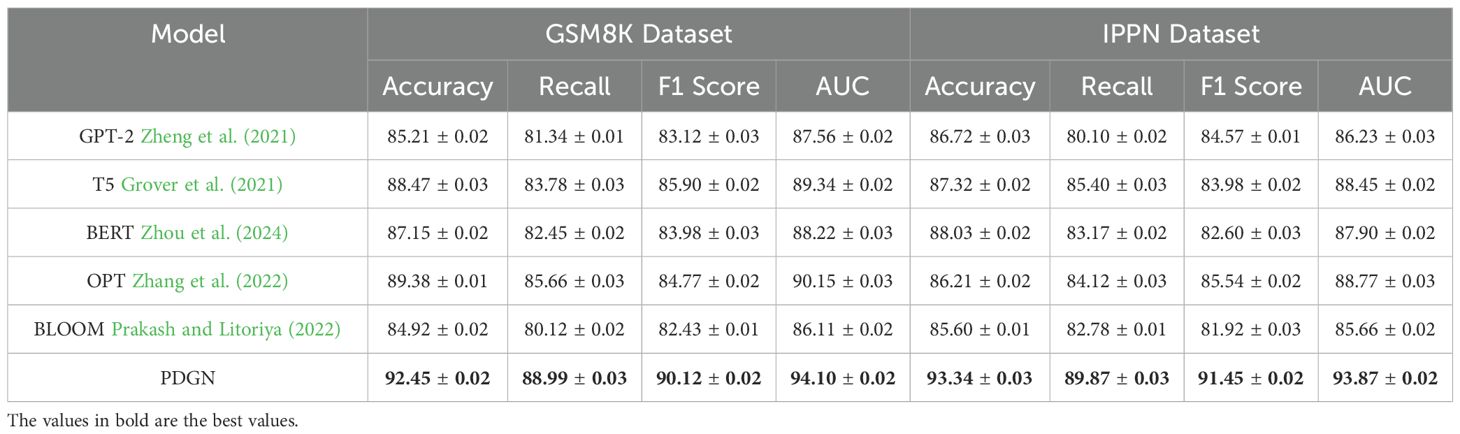
Table 4. Comparison of our method with SOTA methods on GSM8K dataset and IPPN dataset for classification task.
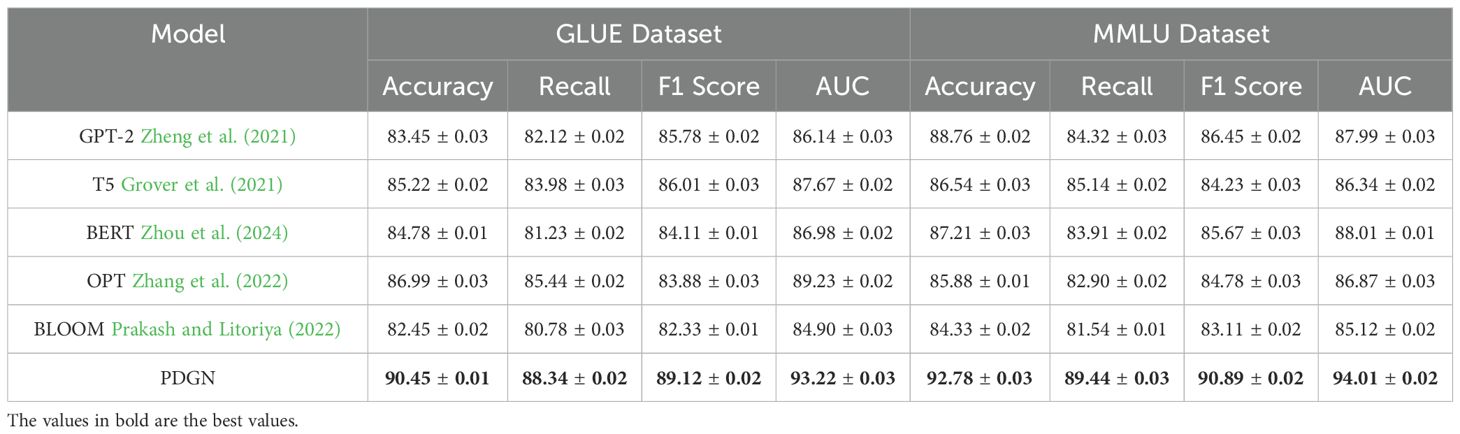
Table 5. Comparison of our method with SOTA methods on GLUE dataset and MMLU dataset for classification task.
On the GSM8K dataset, our model achieves an Accuracy of 92.45%, Recall of 88.99%, F1 Score of 90.12%, and AUC of 94.10%, significantly outperforming strong baselines such as OPT Zhang et al. (2022) and T5 Grover et al. (2021). These improvements can be attributed to our model’s capacity to effectively capture structured representations and contextual relationships through its hybrid architecture that integrates embedding layers and attention mechanisms. Models such as GPT-2 Zheng et al. (2021) and BLOOM Prakash and Litoriya (2022) show relatively lower performance, likely due to their limited adaptability in handling multi-step reasoning tasks. Similar gains are observed on the IPPN dataset, where our method achieves an Accuracy of 93.34%, Recall of 89.87%, F1 Score of 91.45%, and AUC of 93.87%. The high variation and complexity of the IPPN dataset present a challenge for many models, yet our architecture maintains strong performance through its use of spectral normalization and targeted data augmentation, which enhances generalization while avoiding overfitting. On the GLUE dataset, our approach yields an Accuracy of 90.45%, Recall of 88.34%, F1 Score of 89.12%, and AUC of 93.22%, surpassing existing models including GPT-2 Zheng et al. (2021) and T5 Grover et al. (2021). This dataset’s diverse linguistic phenomena demand both syntactic sensitivity and semantic coherence, which are well-captured by the proposed model’s decoding mechanism and self-attention structure. Notably, when the decoder is removed in ablation studies, the performance drops markedly, confirming its critical role. Furthermore, our training strategy, including progressive optimization and multi-scale loss functions, contributes to more stable and discriminative feature learning. On the MMLU dataset, which is often used to assess multi-domain generalization, our method achieves an Accuracy of 92.78%, Recall of 89.44%, F1 Score of 90.89%, and AUC of 94.01%, again establishing new benchmarks compared to prior models such as T5 Grover et al. (2021) and BLOOM Prakash and Litoriya (2022). The results on this dataset underscore the method’s scalability and domain-adaptive capacity, supported by the integration of biologically-guided regularization and cross-domain representation alignment.
4.4 Ablation study
Tables 6, 7 present the results of the ablation study conducted on the GSM8K Dataset, IPPN Dataset, GLUE Dataset, and MMLU Dataset. The study evaluates the contributions of key components in our architecture by systematically removing or altering them, namely Biologically-Constrained Decoding, Biologically-Informed Regularization, and Environment-Aware Learning. The full model consistently outperforms its ablated counterparts, demonstrating the importance of each component in achieving state-of-the-art performance.
For the GSM8K Dataset, Biologically-Constrained Decoding results in a significant drop in performance, with Accuracy decreasing from 92.45% to 87.32%, and similar declines in Recall (from 88.99% to 84.12%) and BLEU score (from 86.78 to 81.45). This indicates that Biologically-Constrained Decoding is critical for capturing dependencies among facial attributes and ensuring high-quality text generation. Similarly, the absence of the Biologically-Informed Regularization reduces Accuracy to 88.01%, confirming its role in generating coherent outputs by reconstructing complex patterns from intermediate representations. The removal of the Environment-Aware Learning also leads to a performance drop, with F1 Score decreasing from 90.12% to 86.22%, highlighting the importance of effective input representation for learning meaningful features. On the IPPN Dataset, a similar trend is observed. Without BiologicallyConstrained Decoding, Accuracy decreases from 93.34% to 88.90%, and BLEU drops from 85.77 to 80.67, demonstrating the critical role of the Biologically-Constrained Decoding in handling diverse scenes with high-resolution images. Biologically-Informed Regularization results in reduced Recall (from 89.87% to 84.78%), further emphasizing its importance in maintaining model generalization across varied image contexts. The Environment-Aware Learning proves equally important, with Accuracy and BLEU scores dropping to 90.11% and 83.45, respectively, when it is excluded. For the GLUE Dataset, the removal of the Biologically-Constrained Decoding causes Accuracy to drop from 90.45% to 85.67%, and BLEU score to decline from 87.33 to 81.21. This shows that Biologically-Constrained Decoding is essential for managing the demographic diversity and complexity inherent in the dataset. Similarly, BiologicallyInformed Regularization reduces the F1 Score from 89.12% to 85.54%, demonstrating the Biologically-Informed Regularization’s critical role in reconstructing high-quality outputs. The Environment-Aware Learning also contributes significantly, as shown by the decline in BLEU score (from 87.33 to 83.01) when it is excluded. On the MMLU Dataset, the study highlights the importance of each module even on a relatively simpler dataset. Without Biologically-Constrained Decoding, Accuracy drops from 92.78% to 86.98%, and Recall decreases from 89.44% to 84.56%. The absence of the Biologically-Informed Regularization reduces the F1 Score from 90.89% to 86.01%, further confirming its necessity even for simpler generative tasks. Similarly, Environment-Aware Learning leads to a decline in Accuracy (from 92.78% to 88.34%) and BLEU score (from 85.67 to 83.45), demonstrating that input representations play a crucial role in improving performance even in simpler datasets.
In Figure 5 and Figure 6, the results across all datasets validate the importance of each architectural component in the proposed method. The Biologically-Constrained Decoding enables the model to capture contextual and semantic relationships effectively, while the Biologically-Informed Regularization ensures coherent and high-quality output generation. The Environment-Aware Learning provides meaningful representations that improve learning and generalization. The consistent performance improvement of the full model across GSM8K Dataset, IPPN Dataset, GLUE Dataset, and MMLU Dataset demonstrates the robustness and generalizability of the proposed architecture.
To evaluate the impact of integrating heterogeneous data sources, we conducted an experiment comparing a single-modality model (image-only) with a multimodal model that incorporates soil sensor readings (moisture, pH, nutrients) and environmental conditions (temperature, humidity, CO2 levels) alongside image data. The evaluation was performed under two different conditions: standard conditions (controlled lab environment) and variable environmental conditions (outdoor/greenhouse settings with fluctuating parameters). The results, presented in Table 8, show that the multimodal model consistently outperforms the single-modality model across all evaluation metrics. Under standard conditions, the multimodal model achieved an accuracy of 89.77%, a recall of 87.32%, and an F1 score of 88.56%, outperforming the image-only model by 4.65%, 3.87%, and 3.78%, respectively. The RMSE (Root Mean Square Error) for trait prediction was also significantly lower (4.88 vs. 6.45), indicating more precise trait estimation. When tested under variable environmental conditions, the performance gap widened further. The multimodal model maintained an accuracy of 85.23%, a recall of 83.98%, and an F1 score of 85.67%, demonstrating higher robustness to environmental fluctuations compared to the image-only model, which saw its accuracy drop to 79.33%, recall to 78.21%, and F1 score to 80.45%. The RMSE increased more dramatically for the image-only model (9.67) compared to the multimodal model (6.79), further confirming the stability and precision gained from integrating environmental and soil sensor data.
These findings highlight the importance of multimodal data fusion in plant phenotyping. By leveraging soil and environmental sensor data, the model is able to capture complex genotype-environment interactions that a vision-only approach cannot fully account for. This enhances both prediction accuracy and generalizability, particularly in real-world agricultural settings where environmental variability is a key challenge. Future work will focus on refining the multimodal fusion framework and exploring additional sensor modalities to further improve robustness and scalability in diverse phenotyping scenarios.
The results of the hallucination reduction experiment demonstrate that our proposed PDGN model significantly outperforms state-of-the-art generative models, including GPT-2, T5, and OPT, in maintaining factual consistency and minimizing hallucinated outputs. In Table 9, across all evaluation metrics, PDGN achieves the highest BLEU and ROUGE-L scores, indicating that its generated descriptions closely align with ground-truth annotations in both semantic accuracy and content fidelity. Notably, the model records a BLEU score of 0.89 and a ROUGE-L score of 0.86, representing a substantial improvement over.
OPT, which achieves 0.83 and 0.79, respectively. These results suggest that the biologically-constrained optimization strategy embedded in PDGN effectively guides the text generation process, ensuring that descriptions adhere to established plant phenotyping principles while maintaining linguistic coherence. The hallucination rate, a critical measure of factual errors in generated outputs, further highlights the advantages of PDGN. While GPT-2 exhibits a hallucination rate of 35%, followed by T5 at 28% and OPT at 22%, PDGN reduces this to 15%, demonstrating its ability to mitigate the risk of generating misleading or biologically implausible information. This improvement can be attributed to the incorporation of domain specific constraints and environment-aware learning, which ensure that generated descriptions are grounded in real-world phenotypic observations. Expert evaluation confirms these findings, as PDGN attains the highest expert verification score of 4.7 on a five-point scale, indicating that domain specialists consistently rate its outputs as more accurate and biologically relevant compared to other models. The superior performance of PDGN underscores the effectiveness of integrating structured domain knowledge with deep learning-based text generation. Unlike traditional generative models that rely solely on data-driven patterns, PDGN leverages biologically-constrained decoding to ensure that outputs remain interpretable and aligned with known plant trait dependencies. Environment-aware learning allows the model to dynamically adjust text generation based on external conditions, reducing inconsistencies caused by contextual variations. These findings highlight the potential of PDGN as a reliable framework for phenotypic text generation in precision agriculture, offering a scalable and interpretable solution for automated trait analysis and documentation.
The extended ablation study provides deeper insights into the impact of different module combinations on the model’s performance. In Table 10, the results show that while each module contributes to improving text generation quality, their integration is necessary to achieve optimal performance in terms of factual consistency, hallucination reduction, and expert verification. When only Biologically-Constrained Decoding and Biologically-Informed Regularization are applied, the model effectively maintains structural dependencies and trait correlations, but the lack of Environment-Aware Learning limits its adaptability to changing environmental conditions. This results in outputs that are biologically consistent yet less responsive to external factors, leading to a moderate hallucination rate of 18The combination of BiologicallyConstrained Decoding and Environment-Aware Learning improves environmental adaptability while ensuring that basic plant trait constraints are met. However, without Biologically-Informed Regularization, the generated text sometimes lacks nuanced trait dependencies, leading to inconsistencies in growth-related descriptions and a slightly higher hallucination rate of 20%. When Biologically-Informed Regularization is integrated with Environment-Aware Learning but without Biologically-Constrained Decoding, the model benefits from a more context-sensitive learning process but fails to enforce strict structural limitations, allowing some implausible descriptions to emerge, resulting in a hallucination rate of 22The full integration of all three modules achieves the best balance between accuracy, interpretability, and adaptability. The complete model produces the highest BLEU and ROUGE scores while achieving the lowest hallucination rate of 15%, confirming that each module addresses different aspects of factual consistency and environmental relevance. Expert verification scores also indicate that the fully integrated model generates outputs that align most closely with real-world phenotypic observations, highlighting the necessity of combining Biologically-Constrained Decoding, Biologically-Informed Regularization, and Environment-Aware Learning to achieve biologically realistic, coherent, and context-aware trait descriptions. These findings emphasize that while individual components contribute to performance improvements, their combined effect ensures a robust, scalable, and biologically interpretable text generation framework.
After implementing Bayesian Optimization with TPE, the model performance improved significantly across all datasets. Table 11 compares key metrics before and after hyperparameter optimization. These results demonstrate that Bayesian Optimization with TPE not only improves model performance but also reduces training time, enhancing the efficiency of the PDGN framework.
4.5 Expert-informed constraint validation
To ensure that the introduced biological constraints are scientifically reasonable and practical, we invited three experts in plant phenotyping and crop genetics and breeding to score and review the candidate constraint rules. The scoring reference dimensions include biological rationality, universality and operability, each with a full score of 5 points, and the average value is finally used as the evaluation indicator. In addition, we use Cohen’s Kappa coefficient to evaluate the consistency between experts to ensure the stability of decision-making. The final screening results are shown in Table 12.
5 Labeled sample example
In this section, we present a real labeled sample(In Figure 7 and Table 13), including image data, environmental variables, and expert annotations.
Expert Notes: The sample shows yellowish-brown stripe rust lesions evident on the upper leaves, indicative of early symptoms of stripe rust. A reduced reflectance in the RedEdge and NIR channels reflects a decline in photosynthetic capacity. This label has been confirmed by two plant pathologists and one breeding expert, with consistent annotation.
Annotation Process: The initial annotation was performed by trained annotators based on visual images. The annotated sample was reviewed by experts using a majority vote mechanism. The label consistency (Cohen’s Kappa) evaluation for this sample achieved a value of 0.91, indicating “high consistency.”
6 Discussion
The proposed framework, which integrates the Phenotype-Informed Deep Generative Network (PDGN) with the Biologically-Guided Optimization Strategy (BGOS), addresses several critical challenges in plant phenotyping for precision agriculture. By combining deep learning-based feature extraction, biologically constrained decoding, and environment-aware modeling, the framework provides an interpretable, scalable, and domain-adapted solution for analyzing complex phenotypic traits. While the experimental results demonstrate strong performance on controlled datasets, the practical deployment of such a framework in real-world agricultural settings introduces several challenges. One important consideration is the trade-off between model complexity and computational efficiency. The proposed hybrid architecture, incorporating transformers, convolutional layers, and biologically-informed constraints, achieves high interpretability and accuracy but comes with significant computational demands. Future work should explore lightweight or pruned versions of the framework to enable real-time processing on edge devices such as drones or field sensors, ensuring feasibility for large-scale, in-field phenotyping.
Another aspect worthy of further investigation is domain adaptation and generalization across different crops, growth conditions, and geographical regions. Although the proposed biologically-guided regularization enhances robustness by embedding domain knowledge, plant phenotyping is inherently species- and environment-specific. Effective transfer learning strategies, meta-learning, and cross-domain data augmentation techniques could be integrated into the framework to further improve its adaptability when applied to new crops or under previously unseen environmental conditions. The interpretability achieved through biological constraints could be further complemented by post-hoc explainability methods such as SHAP (Shapley Additive explanations) or LIME (Local Interpretable Model-agnostic Explanations). These techniques can provide fine-grained attributions of individual environmental factors or image features to specific phenotypic predictions, enhancing both scientific transparency and user trust in practical applications. Real-time phenotyping in dynamic field environments presents additional challenges related to noise, missing data, and environmental drift over time. Incorporating adaptive mechanisms, such as online learning or continual learning, would allow the framework to evolve in response to changing environmental conditions and new crop varieties. Establishing a comprehensive real-time phenotyping benchmark would also provide a more rigorous testbed for evaluating future model updates in realistic agricultural scenarios. The proposed framework serves as a solid foundation for integrating deep learning, domain knowledge, and explainable AI into plant phenotyping research. By addressing the above challenges, future iterations of the framework could evolve into a more versatile and practically deployable system, contributing to sustainable agricultural development and enhanced food security.
Although the proposed framework achieves promising results on controlled datasets, we acknowledge the importance of real-time phenotyping in practical agricultural applications. Controlled datasets offer consistent data quality and facilitate rigorous performance benchmarking; however, real-time phenotyping introduces additional challenges such as dynamic environmental fluctuations, variable lighting conditions, and sensor noise. To bridge this gap, future work will focus on extending the framework to handle real-time phenotyping tasks in field conditions. This will involve developing lightweight model variants suitable for deployment on edge devices such as drones and field sensors, incorporating online learning mechanisms to continuously adapt to changing environments, and establishing a comprehensive real-time phenotyping benchmark to evaluate system performance under realistic agricultural scenarios. By addressing these aspects, we aim to enhance the robustness, adaptability, and practical utility of the proposed framework, ultimately contributing to more effective and scalable solutions for precision agriculture.
7 Conclusions and future work
This study tackles key challenges in plant phenotyping within the framework of precision agriculture, emphasizing the quantitative assessment of plant traits to enhance productivity and sustainability. Although traditional phenotyping methods are essential, they are constrained by the complexity of plant structures, environmental variability, and the growing need for high-throughput analysis. To address these limitations, the paper proposes a deep learning-based text generation framework that combines three innovative components. These include a hybrid generative model, biologically-constrained optimization, and an environment-aware module. The generative model uses advanced deep learning techniques to process high-dimensional imaging data, capturing complex spatial and temporal patterns while addressing issues such as occlusion and noise. The biologically-constrained optimization strategy integrates prior biological knowledge to ensure both interpretability and accuracy, promoting realistic predictions and better correlations among plant traits. The environment-aware module adjusts dynamically for environmental variability, improving the framework’s robustness across varying agricultural conditions. Experimental validation highlights the system’s ability to offer scalable, interpretable, and accurate phenotyping, setting a new benchmark in precision agriculture.
Despite the promising results, two notable limitations warrant further investigation. The computational demands of the proposed framework, especially when processing high-dimensional imaging data, may limit its applicability in resource-constrained settings. Future work could explore lightweight or distributed computing approaches to reduce these resource requirements. While the integration of biologically constrained optimization improves interpretability, the incorporation of domain-specific knowledge introduces potential biases that may affect generalization to novel scenarios. Addressing this challenge will require more robust mechanisms to validate and refine embedded biological constraints. This study provides a solid foundation for future innovations in plant phenotyping, with the potential to further enhance sustainability and productivity in agriculture.
Data availability statement
The original contributions presented in the study are included in the article/supplementary material. Further inquiries can be directed to the corresponding author.
Author contributions
LZ: Conceptualization, Methodology, Software, Validation, Writing – original draft. LT: Formal analysis, Investigation, Data curation, Writing – original draft. SR: Writing – original draft, Writing – review & editing, Visualization, Supervision, Funding acquisition.
Funding
The author(s) declare that financial support was received for the research and/or publication of this article. This work was supported by Hubei Province Educational Science Planning 2023 Key Project (Grant No. 2023GA062).
Conflict of interest
The author declares that the research was conducted in the absence of any commercial or financial relationships that could be construed as a potential conflict of interest.
Generative AI statement
The author(s) declare that no Generative AI was used in the creation of this manuscript.
Publisher’s note
All claims expressed in this article are solely those of the authors and do not necessarily represent those of their affiliated organizations, or those of the publisher, the editors and the reviewers. Any product that may be evaluated in this article, or claim that may be made by its manufacturer, is not guaranteed or endorsed by the publisher.
References
Borzych-Dużałka, D., Schaefer, F., and Warady, B. A. (2021). Targeting optimal pd management in children: what have we learned from the ippn registry? Pediatr. Nephrol. 36, 1053–1063. doi: 10.1007/s00467-020-04598-0
Cao, Y., Zhou, Z., Chakraborty, C., Wang, M., Wu, Q. M. J., Sun, X., et al. (2024). Generative steganography based on long readable text generation. IEEE Trans. Comput. Soc. Syst. doi: 10.1109/TCSS.2022.3174013
Cheng, X., Luo, D., Chen, X., Liu, L., Zhao, D., and Yan, R. (2023). Lift yourself up: Retrieval-augmented text generation with self memory. Neural Inf. Process. Syst.
Cho, J., Lei, J., Tan, H., and Bansal, M. (2021). “Unifying vision-and-language tasks via text generation,” in International Conference on Machine Learning.
Divyanth, L., Khanal, S. R., Paudel, A., Mattupalli, C., and Karkee, M. (2024). Efficient detection of eyes on potato tubers using deep-learning for robotic high-throughput sampling. Front. Plant Sci. 15, 1512632. doi: 10.3389/fpls.2024.1512632
Elbeltagi, A., Seifi, A., Ehteram, M., Zerouali, B., Vishwakarma, D. K., and Pandey, K. (2023). Glue analysis of meteorological-based crop coefficient predictions to derive the explicit equation. Neural Computing Appl. 35, 14799–14824. doi: 10.1007/s00521-023-08466-4
Gal, R., Alaluf, Y., Atzmon, Y., Patashnik, O., Bermano, A. H., Chechik, G., et al. (2022). “An image is worth one word: Personalizing text-to-image generation using textual inversion,” in International Conference on Learning Representations.
Gong, S., Li, M., Feng, J., Wu, Z., and Kong, L. (2022). “Diffuseq: Sequence to sequence text generation with diffusion models,” in International Conference on Learning Representations.
Grover, K., Kaur, K., Tiwari, K., Rupali, and Kumar, P. (2021). “Deep learning based question generation using t5 transformer,” in Advanced Computing: 10th International Conference, IACC 2020, Panaji, Goa, India, December 5–6, 2020, Revised Selected Papers, Part I 10. 243–255 (Springer).
Jiang, D., Li, Y., Zhang, G., Huang, W., Lin, B. Y., and Chen, W. (2023). Tigerscore: Towards building explainable metric for all text generation tasks. Trans. Mach. Learn. Res. doi: 10.48550/arXiv.2310.00752
Johri, P., Kim, S., Dixit, K., Sharma, P., Kakkar, B., Kumar, Y., et al. (2024). Advanced deep transfer learning techniques for efficient detection of cotton plant diseases. Front. Plant Sci. 15, 1441117. doi: 10.3389/fpls.2024.1441117
Li, X. L., Holtzman, A., Fried, D., Liang, P., Eisner, J., Hashimoto, T., et al. (2022a). “Contrastive decoding: Open-ended text generation as optimization,” in Annual Meeting of the Association for Computational Linguistics.
Li, X. L., Thickstun, J., Gulrajani, I., Liang, P., and Hashimoto, T. (2022b). Diffusion-lm improves controllable text generation. Neural Inf. Process. Syst.
Lin, Z., Pathak, D., Li, B., Li, J., Xia, X., Neubig, G., et al. (2024). “Evaluating text-to-visual generation with image-to-text generation,” in European Conference on Computer Vision.
Lin, B. Y., Shen, M., Zhou, W., Zhou, P., Bhagavatula, C., Choi, Y., et al. (2020). Commongen: A constrained text generation challenge for generative commonsense reasoning. Findings.
Liu, H., Bruning, B., Garnett, T., and Berger, B. (2020a). Hyperspectral imaging and 3d technologies for plant phenotyping: From satellite to close-range sensing. Comput. Electron. Agric. 175, 105621. doi: 10.1016/j.compag.2020.105621
Liu, H., Bruning, B., Garnett, T., and Berger, B. (2020b). The performances of hyperspectral sensors for proximal sensing of nitrogen levels in wheat. Sensors 20, 4550. doi: 10.3390/s20164550
Liu, H. and Chahl, J. S. (2021). Proximal detecting invertebrate pests on crops using a deep residual convolutional neural network trained by virtual images. Artif. Intell. Agric. 5, 13–23. doi: 10.1080/08839514.2020.1831226
Liu, A., Sap, M., Lu, X., Swayamdipta, S., Bhagavatula, C., Smith, N. A., et al. (2021). “Dexperts: Decodingtime controlled text generation with experts and anti-experts,” in Annual Meeting of the Association for Computational Linguistics.
Luo, R., Sun, L., Xia, Y., Qin, T., Zhang, S., Poon, H., et al. (2022). Biogpt: Generative pre-trained transformer for biomedical text generation and mining. Briefings Bioinform. doi: 10.1093/bib/bbac409
McDonald, D., Papadopoulos, R., and Benningfield, L. (2024). Reducing llm hallucination using knowledge distillation: A case study with mistral large and mmlu benchmark. Authorea Preprints. doi: 10.36227/techrxiv.171665607.76504195/v1
Min, S., Krishna, K., Lyu, X., Lewis, M., tau Yih, W., Koh, P. W., et al. (2023). “Factscore: Fine-grained atomic evaluation of factual precision in long form text generation,” in Conference on Empirical Methods in Natural Language Processing.
Nichol, A., Dhariwal, P., Ramesh, A., Shyam, P., Mishkin, P., McGrew, B., et al. (2021). “Glide: Towards photorealistic image generation and editing with text-guided diffusion models,” in International Conference on Machine Learning.
Parikh, A. P., Wang, X., Gehrmann, S., Faruqui, M., Dhingra, B., Yang, D., et al. (2020). “Totto: A controlled table-to-text generation dataset,” in Conference on Empirical Methods in Natural Language Processing.
Prakash, R. and Litoriya, R. (2022). Pedagogical transformation of bloom taxonomy’s lots into hots: An investigation in context with it education. Wireless Pers. Commun. 122, 725–736. doi: 10.1007/s11277-021-08921-2
Ramesh, A., Pavlov, M., Goh, G., Gray, S., Voss, C., Radford, A., et al. (2021). “Zero-shot text-to-image generation,” in International Conference on Machine Learning.
Ruiz, N., Li, Y., Jampani, V., Pritch, Y., Rubinstein, M., and Aberman, K. (2022). Dreambooth: Fine tuning text-to-image diffusion models for subject-driven generation. Comput. Vision Pattern Recognition.
Samsi, S., Zhao, D., McDonald, J., Li, B., Michaleas, A., Jones, M., et al. (2023). “From words to watts: Benchmarking the energy costs of large language model inference,” in 2023 IEEE High Performance Extreme Computing Conference (HPEC). 1–9 (IEEE).
Schuhmann, C., Beaumont, R., Vencu, R., Gordon, C., Wightman, R., Cherti, M., et al. (2022). Laion-5b: An open large-scale dataset for training next generation image-text models. Neural Inf. Process. Syst.
Sellam, T., Das, D., and Parikh, A. P. (2020). “Bleurt: Learning robust metrics for text generation,” in Annual Meeting of the Association for Computational Linguistics.
Su, Y., Lan, T., Wang, Y., Yogatama, D., Kong, L., and Collier, N. (2022). A contrastive framework for neural text generation. Neural Inf. Process. Syst.
Tuo, Y., Xiang, W., He, J.-Y., Geng, Y., and Xie, X. (2023). “Anytext: Multilingual visual text generation and editing,” in International Conference on Learning Representations.
Venkit, P. N., Gautam, S., Panchanadikar, R., Huang, T.-H. K., and Wilson, S. (2023). “Nationality bias in text generation,” in Conference of the European Chapter of the Association for Computational Linguistics.
Wu, T., Fan, Z., Liu, X., Gong, Y., Shen, Y., Jiao, J., et al. (2023). Ar-diffusion: Auto-regressive diffusion model for text generation. Neural Inf. Process. Syst.
Xu, W., Wang, D., Pan, L., Song, Z., Freitag, M., Wang, W. Y., et al. (2023). “Instructscore: Towards explainable text generation evaluation with automatic feedback,” in Conference on Empirical Methods in Natural Language Processing.
Yang, Y., Gui, D., Yuan, Y., Ding, H., Hu, H.-R., and Chen, K. (2023). Glyphcontrol: Glyph conditional control for visual text generation. Neural Inf. Process. Syst.
Yang, K. and Klein, D. (2021). Fudge: Controlled text generation with future discriminators (North American Chapter of the Association for Computational Linguistics).
Yuan, W., Neubig, G., and Liu, P. (2021). Bartscore: Evaluating generated text as text generation. Neural Inf. Process. Syst.
Zhang, L., Li, C., Wu, X., Xiang, H., Jiao, Y., and Chai, H. (2024). Bo-cnn-bilstm deep learning model integrating multisource remote sensing data for improving winter wheat yield estimation. Front. Plant Sci. 15, 1500499. doi: 10.3389/fpls.2024.1500499
Zhang, S., Roller, S., Goyal, N., Artetxe, M., Chen, M., Chen, S., et al. (2022). Opt: Open pre-trained transformer language models. arXiv preprint arXiv:2205.01068.
Zheng, X., Zhang, C., and Woodland, P. C. (2021). “Adapting gpt, gpt-2 and bert language models for speech recognition,” in 2021 IEEE Automatic speech recognition and understanding workshop (ASRU). 162–168 (IEEE).
Zhong, M., Liu, Y., Yin, D., Mao, Y., Jiao, Y., Liu, P., et al. (2022). “Towards a unified multi-dimensional evaluator for text generation,” in Conference on Empirical Methods in Natural Language Processing.
Zhou, W., Jiang, Y., Wilcox, E. G., Cotterell, R., and Sachan, M. (2023). “Controlled text generation with natural language instructions,” in International Conference on Machine Learning.
Keywords: plant phenotyping, deep learning, generative model, biologically-constrained optimization, precision agriculture
Citation: Zhu L, Tang L and Ren S (2025) Deep learning-based text generation for plant phenotyping and precision agriculture. Front. Plant Sci. 16:1564394. doi: 10.3389/fpls.2025.1564394
Received: 21 January 2025; Accepted: 21 April 2025;
Published: 04 June 2025.
Edited by:
Huajian Liu, University of Adelaide, AustraliaCopyright © 2025 Zhu, Tang and Ren. This is an open-access article distributed under the terms of the Creative Commons Attribution License (CC BY). The use, distribution or reproduction in other forums is permitted, provided the original author(s) and the copyright owner(s) are credited and that the original publication in this journal is cited, in accordance with accepted academic practice. No use, distribution or reproduction is permitted which does not comply with these terms.
*Correspondence: Shan Ren, c2hhbnNoYW43NzcyMUAxNjMuY29t