- 1International Crop Research Center for Stress Resistance, School of Life Sciences, Guangzhou University, Guangzhou, China
- 2Crop Research Institute, Guangdong Academy of Agricultural Sciences, Guangzhou, China
- 3Nanxiong Research Institute of Guangdong Tobacco Co., Ltd., Nanxiong, China
- 4The UWA Institute of Agriculture, UWA School of Agriculture and Environment, The University of Western Australia, Perth, WA, Australia
Tobacco bacterial wilt (TBW) is a devastating soil-borne disease threatening the yield and quality of tobacco. However, its genetic foundations are not fully understood. In this study, we identified 126,602 high-quality single-nucleotide polymorphisms (SNPs) in 94 tobacco accessions using genotyping-by-sequencing (GBS) and a 94.56 KB linkage disequilibrium (LD) decay rate for candidate gene selection. The population structure analysis revealed two subpopulations with 37 and 57 tobacco accessions. Four multi-locus genome-wide association study (ML-GWAS) approaches identified 142 quantitative trait nucleotides (QTNs) in E1–E4 and the best linear unbiased prediction (BLUP), explaining 0.49–22.52% phenotypic variance. Of these, 38 novel stable QTNs were identified across at least two environments/methods, and their alleles showed significant TBW-DI differences. The number of superior alleles associated with TBW resistance for each accession ranged from 4 to 24; eight accessions had more than 18 superior alleles. Based on TBW-resistant alleles, the five best cross combinations were predicted, including MC133 × Ruyuan No. 1 and CO258 × ROX28. We identified 52 candidate genes around 38 QTNs related to TBW resistance based on homologous functional annotation and KEGG enrichment analysis, e.g., CYCD3;2, BSK1, Nitab4.5_0000641g0050, Nitab4.5_0000929g0030. To the best of our knowledge, this is the first comprehensive study to identify QTNs, superior alleles, and their candidate genes for breeding TBW-resistant tobacco varieties. The results provide further insight into the genetic architecture, marker-assisted selection, and functional genomics of TBW resistance, improving future breeding efforts to increase crop productivity.
Introduction
Tobacco (Nicotiana tabacum L.; 2n = 48) is an important cash crop in many countries, including China, and a valuable model system in genetic engineering and molecular biology. Tobacco bacterial wilt (TBW) caused by Ralstonia solanacearum is a destructive soil-borne disease in many regions worldwide (Nishi et al., 2003; Lan et al., 2014; Drake-Stowe et al., 2017). Infected tobacco plants typically exhibit symptoms such as leaf wilt, root and stem necrosis, and growth retardation, followed by plant death, which reduce yield and quality (Qian et al., 2013). TBW is prevalent in tobacco-growing countries with moist tropical or warm-temperate climates (Denny, 2006). In China, the disease occurrence has been steadily rising, reaching 15–30% in some areas (Jiang et al., 2017), posing a serious threat to tobacco production in the four main tobacco-growing regions, including 14 provinces (Li et al., 2016). Several methods, including crop rotation and soil fumigation, can reduce some economic losses from the disease; however, none provide sufficient protection (Nishi et al., 2003; Lan et al., 2014).
Tobacco bacterial wilt resistance is a quantitative trait controlled by multiple genes and/or quantitative trait loci/nucleotides (QTLs/QTNs) (Smith and Clayton, 1948; Nishi et al., 2003; Gao et al., 2010; Ni et al., 2011). It is challenging to improve TBW resistance using traditional breeding methods (Yang et al., 2012). Marker-assisted selection (MAS) is an alternative tool for combining different resistance genes/alleles into a single plant, which has been used to improve different traits in crop breeding programs (Kuchel et al., 2005; Ribaut et al., 2010; Nakaya and Isobe, 2012). It is important to identify significant QTLs/QTNs to develop superior TBW-resistant tobacco cultivars. To date, only four QTL mapping studies for TBW resistance have been conducted in bi-parental and different genetic populations, using simple sequence repeat (SSR) and amplified fragment length polymorphism (AFLP) markers (Nishi et al., 2003; Qian et al., 2013; Lan et al., 2014; Drake-Stowe et al., 2017). Nishi et al. (2003) identified one QTL with 43.8% phenotypic variance using 117 AFLP markers in 125 doubled haploid populations. Similarly, Qian et al. (2013); Lan et al. (2014), and Drake-Stowe et al. (2017) identified four, eight, and two QTLs for TBW resistance, respectively. Thus, only 15 QTLs underlying TBW resistance have been identified, which is relatively small compared to other members of the Solanaceae family (Sharma et al., 2021). Unfortunately, the identified QTLs have large genomic regions unsuitable for detecting candidate genes, and markers linked to these QTLs have limited application in tobacco breeding programs (Li et al., 2017).
With the development of next-generation sequencing (NGS) technology, genotyping-by-sequencing (GBS) has been used widely as a high-throughput and low-cost genotyping platform for discovering genome-wide single-nucleotide polymorphisms (SNPs) in many crops, including tobacco (Elshire et al., 2011; Lee et al., 2017; Sakiroglu and Brummer, 2017). Genome-wide association studies (GWAS) can use these millions of SNPs as molecular markers to screen many accessions simultaneously without needing to construct segregating populations in advance (Buckler and Thornsberry, 2002; Flint-Garcia et al., 2003; Yu et al., 2006; Wang et al., 2016; Zhang et al., 2019). For instance, Thapa et al. (2021) identified 23 and 38 QTNs associated with shoot and blossom blight resistance, respectively, using GBS markers in 273 apple accessions, while Jing et al. (2021) identified 18 QTNs related to Sclerotinia stem rot resistance in soybean. Thus, GWAS is an efficient tool for QTN identification in natural populations with high-quality SNPs to overcome the shortcomings of bi-parental QTL mapping (Zhang et al., 2019) and has great potential for discovering interrelationships among complex traits conditioned by multiple genes/alleles (Buckler and Thornsberry, 2002; Yu et al., 2006; Hyun et al., 2021; Thapa et al., 2021). However, no GWAS studies have been undertaken to detect QTNs associated with TBW resistance in tobacco. Identifying QTNs/alleles/genes related to TBW resistance is an important step for improving tobacco production.
This study assembled a panel of 94 tobacco accessions from seven countries and used GBS sequencing to identify high-density SNPs. The study aimed to: (1) analyze the SNP distribution, linkage disequilibrium (LD), and population structure using GBS data; (2) detect QTNs related to TBW resistance using GWAS; (3) identify TBW-resistant superior alleles of stable QTNs for MAS and the best parental cross combination based on superior alleles; (4) predict potential candidate genes for TBW resistance in the region of stable QTNs. The results of this study will provide information for uncovering the genetic basis of TBW resistance and facilitating MAS in tobacco breeding.
Materials and Methods
Plant Material and Phenotyping
Ninety-four tobacco accessions were obtained from the Nanxiong Scientific Research Institute of Guangdong Tobacco Company, China. These accessions came from the United States, Japan, Canada, Somalia, Australia, Zimbabwe, and China, including 90 flue-cured, two sun-cured, and two burley tobacco accessions (Supplementary Table 1).
The 94 accessions were planted at the Hukou experimental station in Nanxiong city in 2013, 2014, and 2015 (denoted E1, E2, and E4) and Xikou experimental station in Nanxiong city in 2014 (denoted E3) in a randomized complete block design with two replicates at each location. Each plot had 20 plants spaced 0.5 m within rows and 1.2 m between rows, with local management practices applied. We used the biochemical type III bacterial wilt pathogen race-1 strain of R. solanacearum. The inoculum was applied in early May (May 8, 2013, May 4, 2014, and May 5, 2015) using the stem puncture inoculation method. Disease ratings for each accession occurred on May 30, 2013, May 29, 2014, and May 29, 2015 using the 0–9 scale described in “China National Tobacco Pests Classification and Survey Methods (GB/T23222-2008)”: 0 = no lesions; 1 = flecks on stem or leaf wilt <1/2 leaf; 3 = lesion on <1/2 stem or leaf wilt on 1/2 to 2/3 leaf; 5 = lesion on >1/2 but not entire stem or leaf wilt on >2/3 leaf; 7 = lesion on entire stem or wilt on entire leaf, and 9 = dead plant (Figure 1). The disease index for TBW (TBW-DI) was calculated according to Lan et al. (2014):
The disease resistance of the 94 accessions was classified using a 0–100 scale following the standard method of “China National Tobacco Varieties Resistance Identification (YC/T 41-1996)”: 0 < TBW-DI ≤ 25 as highly resistant, 25 < TBW-DI ≤ 50 as moderately resistant, 50 < TBW-DI ≤ 75 as moderately susceptible, and 75 < TBW-DI ≤ 100 as highly susceptible.
Statistical Analysis of Phenotypic Data
Mean, range, standard deviation (SD), coefficient of variation (CV%), skewness, kurtosis, and analysis of variance (ANOVA) were calculated for TBW-DI of the 94 tobacco accessions in each environment using R4.0.31 software. A best linear unbiased prediction (BLUP) value of TBW-DI for each tobacco accession was calculated using the lme4 (Bates et al., 2014) statistical package of R. The mixed linear model (MLM) was applied to calculate polygenic and residual error variance components for heritability (Wang et al., 2016) as follows: y = Xα + φ + ε, where y = phenotypic vector, X = incident matrix for fixed effects, α = vector of fixed effects, φ∼MVN(0,Kσg2) = polygenic effect with a multivariate normal distribution with zero mean, and ε∼MVN(0,Iσe2) = vector of residues. Moreover, σg2, σe2, and K were used as polygenic variance, residual variance, and kinship matrix, respectively. The above two variance components were estimated from the restricted maximum likelihood (REML) method, whereas the kinship matrix was calculated from marker information (Xu, 2013). Broad-sense heritability was calculated as:
DNA Extraction and Quantification
Total genomic DNA of the 94 tobacco accessions was isolated from 0.1 g fresh young leaves. DNA extraction was performed using a NuClean Plant Genomic DNA Kit (CWBIO, Beijing, China), according to the manufacturer’s protocol. DNA quality and concentration were evaluated using 1% agarose gel electrophoresis and a NanoDrop spectrophotometer. The DNA concentration was normalized to 30 ng/uL for library construction.
Genotyping-by-Sequencing Library Construction, Sequencing, and Single-Nucleotide Polymorphism Calling
For GBS library preparation, genomic DNA was digested with the restriction enzyme ApeK1, and libraries with 250–500 bp were constructed in 96-plex using the protocols developed by Elshire et al. (2011). The GBS libraries were sequenced on an Illumina HiSeqTM 2000 instrument. Raw reads were de-multiplexed using a barcode sequence, and the adapter sequences were trimmed using the standard Illumina GA Pipeline v1.5. High-quality clean short reads were aligned to the tobacco reference genome, N. tabacum Nitab4.5 (Edwards et al., 2017), using Burrows-Wheeler Aligner (BWA, V0.7.12) software (Li and Durbin, 2009). The SNP variants were extracted using the Unified Genotyper module of GATK software (v3.4-46) in multiple samples (McKenna et al., 2010). The extracted variants were filtered using the following filter parameters: -Window 4, -filter “QD < 4.0 | | FS > 60.0 | | MQ < 40.0,” -G_filter “GQ < 20.” ANNOVAR software was used to annotate all filtered high-density SNPs (Wang et al., 2010). The GAPIT software package was used to create the kinship matrix between accessions, kinship matrix heatmap, and physical map of SNPs (Lipka et al., 2012).
Linkage Disequilibrium and Population Structure Analysis
PLINK v1.90 software (Purcell et al., 2007) was used to analyze the LD by calculating the squared correlation coefficients (R2) of SNPs, using minor allele frequency (MAF) ≥0.05 and a missing rate <20%, and the LD plot was generated using R script. The LD decay rate was observed when the average R2 decreased to half of its maximum value. The population structure for the 94 accessions was evaluated using STRUCTURE v2.3.4 (Pritchard et al., 2000). The hypothetical subgroup (K) values were set from 2 to 10, with 20,000 iterations for each run, followed by 200,000 Markov chain Monte Carlo (MCMC) replications after burn-in. According to Evanno et al. (2005), the best K was identified using STRUCTURE HARVESTER (Earl and vonHoldt, 2012). A neighbor-joining phylogenetic tree of the 94 accessions was constructed using the filtered SNPs by the Tassel 5.2 software (Bradbury et al., 2007).
Genome-Wide Association Mapping
Genome-wide association studies used SNPs with less than 20% missing data, MAF > 0.05, and sequencing depth ≥3. Four multi-locus (ML) GWAS approaches were used to identify significant QTNs, including mrMLM (Wang et al., 2016), pLARmEB (Zhang et al., 2017), ISIS EM-BLASSO (Tamba et al., 2017), and FASTmrMLM (Tamba and Zhang, 2018), while the Q and K matrix were incorporated into a MLM. These methods were implemented using the R package mrMLM (version 4.0.2).2 All multi-locus genome-wide association study (ML-GWAS) models use a modified Bonferroni; the number of markers is replaced by the effective number of markers in the correction formulas (Wang et al., 2016; Zhang et al., 2017). Two-step algorithms are involved in all these methods. In the first step, the single (SL) GWAS method scans the entire genome, with putative QTNs identified according to a less stringent threshold level. In the second step, the effects of selected markers are estimated by empirical Bayesian, the significance of the effects from zero were obtained using the likelihood ratio test, and the threshold level LOD ≥ 3 (P = 0.0002) was used to determine significant trait-associated QTNs (Wang et al., 2016; Tamba et al., 2017).
Superior Allele Analysis for Tobacco Bacterial Wilt Resistance
For this purpose, we used stable QTNs identified in multiple environments and/or by multiple GWAS methods. The resistance allele of each stable QTN was determined using code 1 for genotype and QTN effect value. If the QTN effect value is negative, the genotype with code 1 is considered the TBW-resistant superior allele; if the QTN effect value is positive, the alternative genotype is considered the TBW-resistant superior allele (Wang et al., 2016; Zhang et al., 2019). Correlation coefficients between TBW-DI and the number of superior alleles were calculated using R4.0.3 (see text footnote 1) software. The TBW superior allele percentage was calculated for each accession as the number of superior alleles divided by the total number of stable QTNs. For each QTN, the TBW superior allele percentage in the GWAS population was calculated as the number of accessions with superior alleles divided by the total number of accessions. The best parental cross combinations for tobacco breeding programs were predicted using TBW-resistant superior alleles and stable QTN information.
Prediction of Potential Candidate Genes
The search for potential candidate genes based on the stable QTNs detected by multiple methods and/or in multiple environments/BLUP was performed using the N. tabacum Nitab4.5 reference genome3, according to the genome-wide LD decay distance (Edwards et al., 2017). Next, homologous genes related to bacterial wilt in Arabidopsis were determined by BLAST analysis with 1E-30 critical E-value. These candidate genes were assigned to different biological processes related to bacterial wilt based on the function of their homologs in Arabidopsis in literature, such as WRKY transcription factors (TFs) (Cai et al., 2015; Hussain et al., 2018), ethylene-responsive factors (Gutterson and Reuber, 2004), pathogenesis-related proteins (PRs) (Kuhn et al., 2017), Cytochrome P450 family (Li et al., 2021), and brassinosteroids (Sun and Li, 2017). KEGG analysis was used to identify the functional categories (metabolic or signal transduction pathways) of predicted candidate genes, using the KOBAS v3.0 tool4, with P-value < 5% as threshold criteria (Xie et al., 2011).
Results
Phenotypic Variation of Tobacco Bacterial Wilt in a Natural Population
The mean values for TBW-DI across 94 accessions in the four environments (E1–E4) were 52.75, 51.23, 13.34, and 72.04, with SDs of 35.24, 29.42, 16.88, and 26.47, respectively (Table 1). The CVs were >50% in all environments except E4 (36.74%), indicating the highly dispersed distribution of TBW-DI among accessions. The frequency distribution for TBW-DI in the four environments and BLUP is in Supplementary Figure 1. Skewness and kurtosis values were <1 in E1, E2, and E4, indicating that TBW-DI followed a normal distribution; in E3, TBW-DI was skewed slightly to the left (Table 1 and Supplementary Figure 1). The two-way ANOVA exhibited significant differences (P < 0.001) for genotype, environment, and genotype × environment interaction, suggesting that environmental factors also influence TBW-DI (Table 1). Moreover, the heritability estimates for TBW-DI in the four environments ranged from 61.37 to 81.36%, using residual and polygenic variances (Table 1), indicating that genetic effects play a significant role in TBW-DI variation.
Genotyping-by-Sequencing of the Test Population
We obtained 1412.73 million raw Illumina sequencing reads for the 94 accessions from the GBS library. After quality control and data filtering, 1370.27 million clean reads were generated, with an average 97.06% effective rate (Supplementary Table 2). On average, 98.63% of clean reads had a base error rate of <1% (Q20), and 95.90% of the reads had a base error rate of <0.1% (Q30), with an average GC distribution of 40.92%. Overall, 4.81–23.54 million high-quality sequencing reads were obtained per sample from clean reads, with an average of 9.76 million reads (Supplementary Table 2). Finally, 4.45–22.06 million reads were aligned to the reference genome, with an average mapping ratio of 93.01% (Supplementary Table 2).
After completing the sequencing, 938,799 SNP variants were called from GBS sequencing data using the GATK process. Among these, 573,312 SNPs were transitions, and 365,487 SNPs were transversions. The SNP data were filtered with MAF ≥ 5%, missing rate <20%, sequence depth ≥ 3 to obtain 126,602 high-quality SNPs, comprising 90,276 transitional and 36,326 transversional SNPs. SNP functional annotation revealed that most identified SNPs were located within intergenic regions (85.83%) of the genome followed by intronic regions (6.68%), coding variants (5.64%), upstream (0.95%), downstream (0.75%), and UTR regions (0.11%) (Figure 2A). Further classification of coding SNPs revealed that 54.66 and 44.56% are synonymous and non-synonymous, while stop-gain and stop-loss constituted <1% (Figure 2B). Moreover, the SNPs mentioned earlier were distributed on all 24 chromosomes of tobacco (Figure 2C), with an average of 5275.08 SNPs per chromosome (Supplementary Table 3). The maximal number of SNPs were identified on chromosome Nt17 (9583), while those with minimal numbers were on chromosome Nt02 (2966). The average marker density was approximately 24.46 kb/SNP at the genome level (Supplementary Table 3). The highest marker density (16.23 kb/SNP) was on chromosome Nt11, while the lowest marker density (37.94 kb/SNP) was on chromosome Nt15 (Supplementary Table 3). These results demonstrate the uneven distribution of markers throughout the tobacco genome.
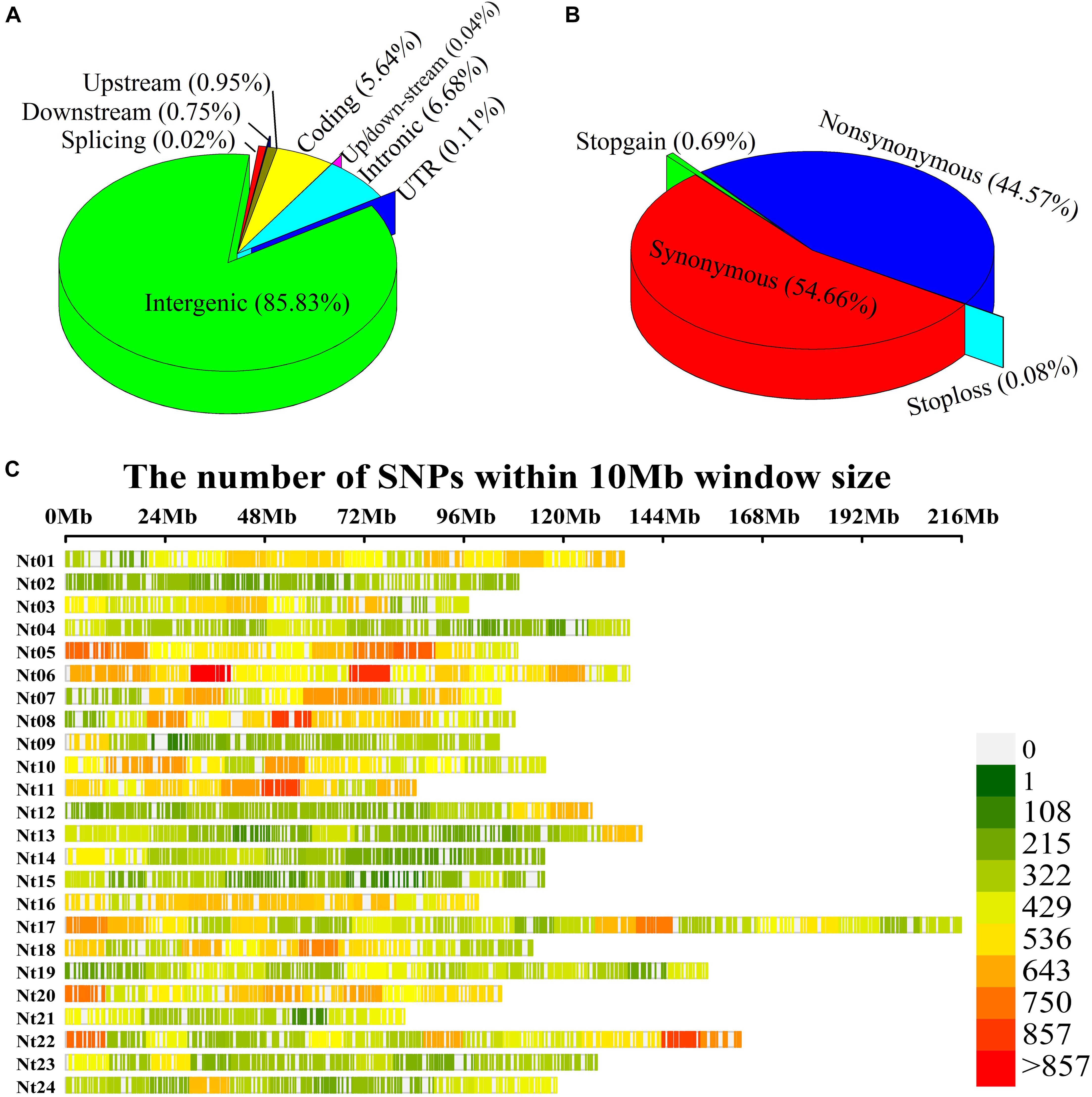
Figure 2. Single-nucleotide polymorphism (SNP) annotation, density, and distribution on 24 chromosomes of tobacco. (A) SNP variant classification; (B) Coding variant classification; (C) Tobacco physical map developed using GBS-SNPs. Y-axis represents chromosomes from Nt01 to Nt24, and X-axis represents the physical positions of the SNPs on each chromosome.
Population Structure, Linkage Disequilibrium, and Kinship Analysis
A total of 126,602 high-density SNPs were used to define the subgroups/subpopulations within the panel of 94 tobacco accessions. Delta K (K = 1–10) analysis revealed two subpopulations (selected K = 2) comprising 37 (39.40%) and 57 (60.60%) tobacco accessions, respectively (Figures 3A,B). Each subpopulation comprised accessions from different ecological zones (Figure 3B), indicating that the division of two subpopulations was not related to their geographical origins. Furthermore, a neighbor-joining phylogenetic tree was conducted based on their genetic distances derived from the SNP differences in these accessions. The population could be divided into two subpopulations (Figure 3C), and the phylogenetic analysis agreed well with the clustering results in STRUCTURE. The squared correlation coefficient (r2) values were calculated for all SNP pairs to determine LD decay. The r2-values decreased rapidly with increasing physical distance between pairwise SNPs (Figure 3D). The overall LD decay distance for all chromosomes was estimated at ∼94.56 kb, where r2 = 0.381 decreased to half its maximum value (Figure 3D). Moreover, the pairwise relative kinship coefficients showed a lower level of genetic relatedness among 94 tobacco accessions (Figure 3E).
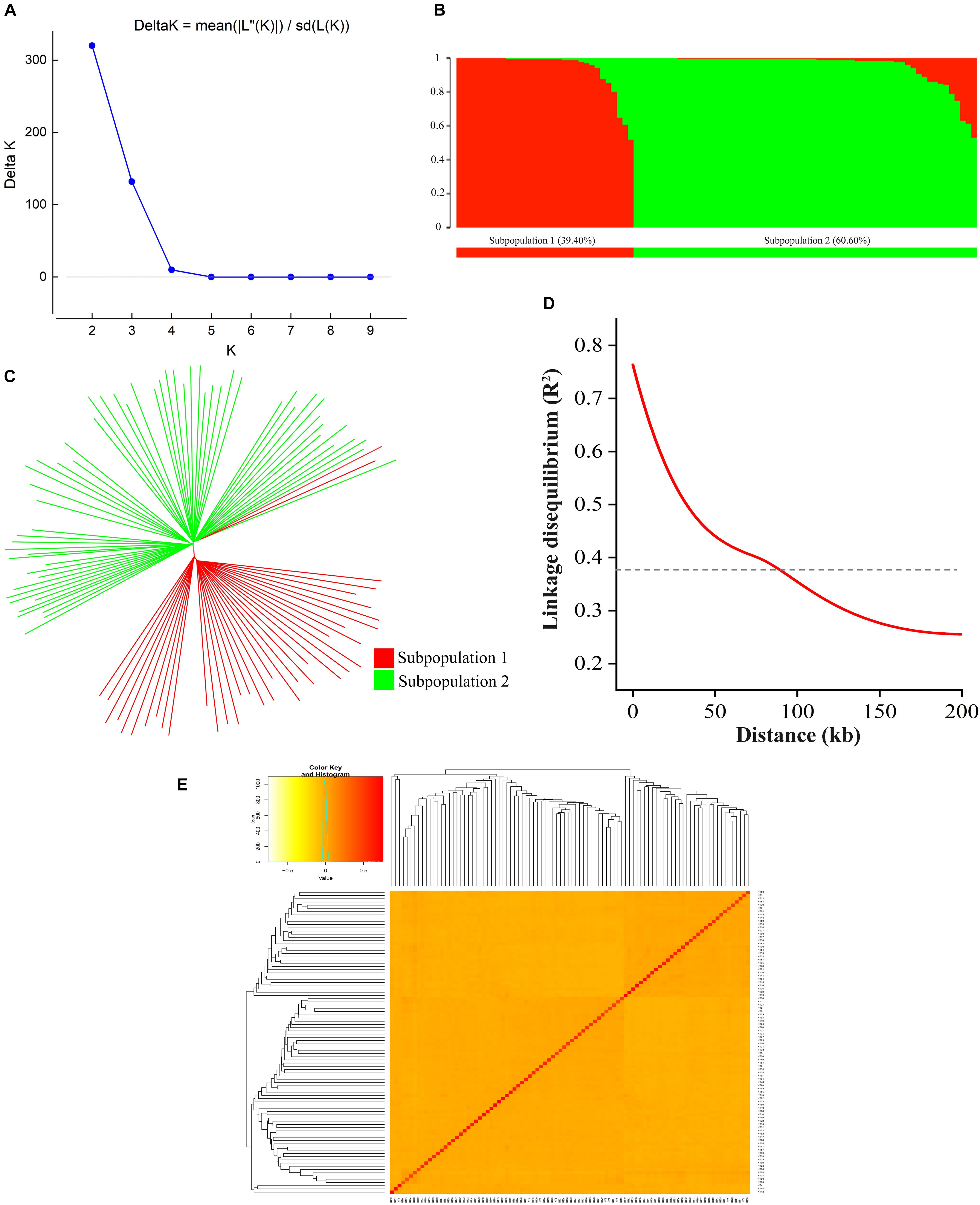
Figure 3. Population structure, phylogenetic tree, LD decay, and kinship of 94 tobacco accessions. (A) Relationship between K and Delta K, and determination of subpopulations using Delta method of Evanno et al. (2005); (B) Distribution of accessions into subgroups: red and green bars indicate subgroup I and subgroup II, respectively; (C) Neighbor-joining phylogenetic tree based on Nei’s genetic distances; (D) Entire genome LD decay of the population; (E) Heatmap of kinship matrix of 94 accessions.
Identification of Quantitative Trait Nucleotides by Multi-Locus Genome-Wide Association Study Methods
The four multi-locus methods identified 142 significant QTNs associated with TBW resistance based on LOD scores ≥3 in the four environments and BLUP (Figure 4 and Supplementary Figure 2). Of these, 26, 34, 38, 26, and 28 QTNs were detected in E1, E2, E3, E4, and BLUP, respectively, explaining 8.18, 7.95, 7.03, 7.93, and 8.22% of the phenotypic variation (PVE) on average (range 0.49–22.52%) (Table 2). The corresponding LOD scores ranged from 3.20 to 12.41, 3.02 to 14.19, 3.01 to 15.20, 3.02 to 13.23, and 3.11 to 13.26 (Supplementary Figure 2). Among the 142 QTNs, 7–12, 5–13, 8–17, and 10–13 QTNs were identified using FASTmrMLM, ISIS EM-BLASSO, mrMLM, and pLARmEB, respectively, in E1–E4 and BLUP (Table 2). The corresponding PVE values ranged from 1.56 to 22.52, 1.14 to 19.16, 0.63 to 20.32, and 0.49 to 19.26, and LOD values ranged from 3.02 to 12.11, 3.10 to 9.56, 3.02 to 14.18, and 3.01 to 15.20 (Table 2). Significant QTNs were disseminated on 24 chromosomes, with more than eight QTNs located on chromosomes 1, 4, 7, 10, 17, 20, and 22 (Supplementary Figure 2). Additionally, the QTNs identified in this study were not located in or overlapped with the genomic region of previously reported QTLs for bacterial wilt resistance in tobacco.
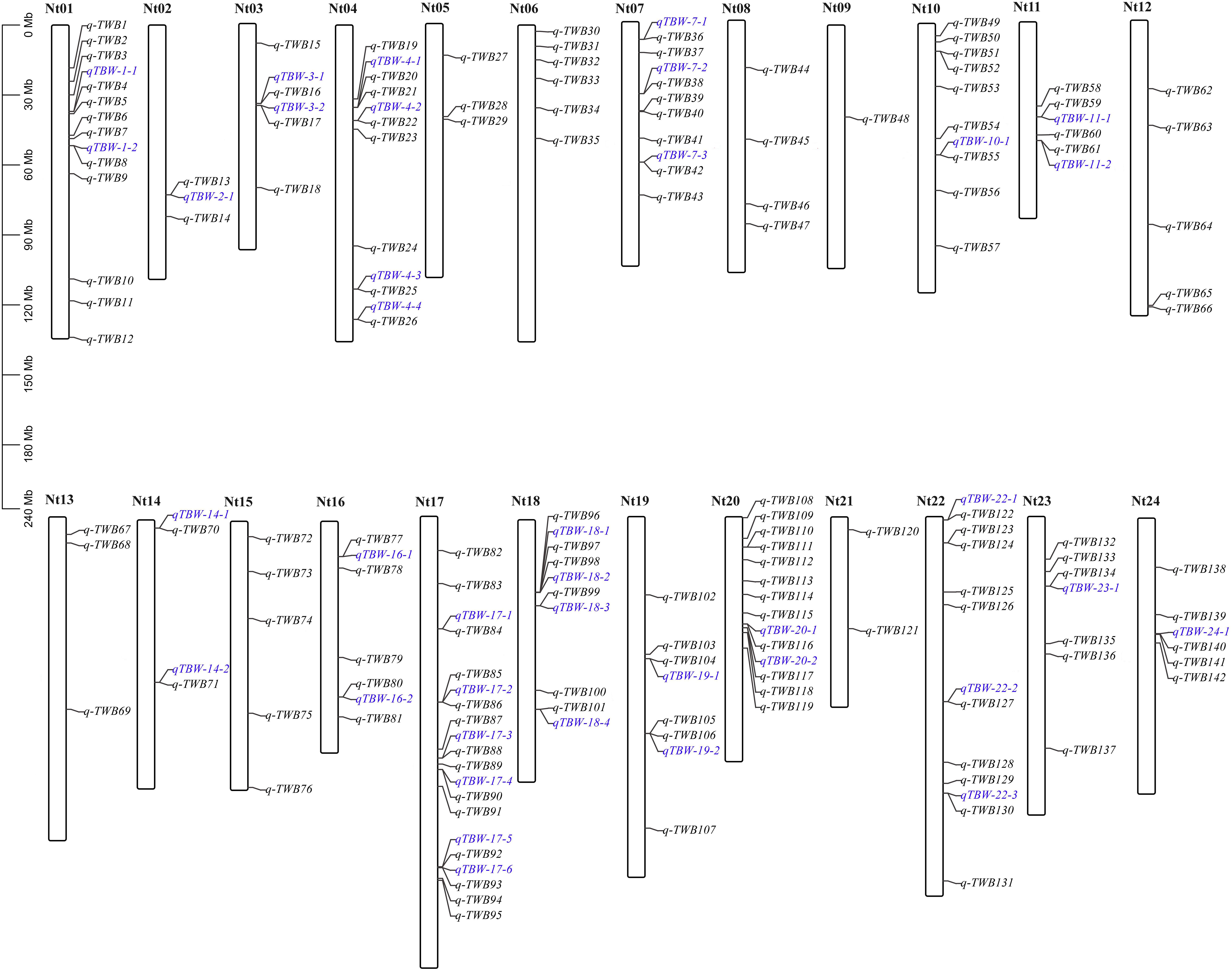
Figure 4. Genetic map based on identified QTNs. The black font represents QTNs identified in E1–E4 and BLUP using four ML-GWAS approaches. The blue font represents QTNs repeatedly identified across at least two environments and/or two ML-GWAS methods.

Table 2. Summary of QTNs detected in multiple environments and BLUP model using four ML-GWAS methods.
Environment- and Method-Stable Quantitative Trait Nucleotides for Tobacco Bacterial Wilt Resistance
Two types of QTNs were defined as stable QTNs: those detected in at least two environments/BLUP (environment-stable) and/or by at least two ML-GWAS models (method-stable). In this study, 38 QTNs were identified as stable for TBW resistance (Table 3 and Figures 4, 5A–C), of which nine were environment-stable (Table 3 in bold and Figure 5B), 37 were method-stable (Table 3 and Figure 5C), and eight were co-detected as environment-stable and method-stable. For example, qTBW-20-1 was detected in E2, E4, and BLUP by three methods, with LOD and PVE values ranging from 3.10 to 9.43 and 8.05 to 19.98, respectively. Likewise, six stable QTNs, qTBW-7-1, qTBW-7-2, qTBW-11-1, qTBW-18-3, qTBW-18-4, and qTBW-19-1 were identified in two environments using two, three, two, four, four, and two ML-GWAS methods; their respective LOD values were 4.55–7.55, 3.49–12.60, 3.37–3.78, 3.86–13.27, 3.10–5.40, and 4.78–14.18, and PVE values were 8.42–17.26, 5.79–16.87, 0.49–1.17, 4.77–14.83, 3.91–12.75, and 4.03–13.37 (Table 3). Three QTNs (qTBW-2-1, qTBW-14-2, and qTBW-17-1) were detected by all four multi-locus methods in one environment, and eight QTNs (qTBW-3-1, qTBW-10-1, qTBW-14-1, qTBW-17-4, qTBW-19-2, qTBW-22-3, qTBW-23-1, and qTBW-24-1) were detected by three methods in one environment. Interestingly, only one QTN (qTBW-22-1) was identified by a single method in two environments, with LOD 4.06–5.48 and PVE 7.03–11.16 (Table 3).
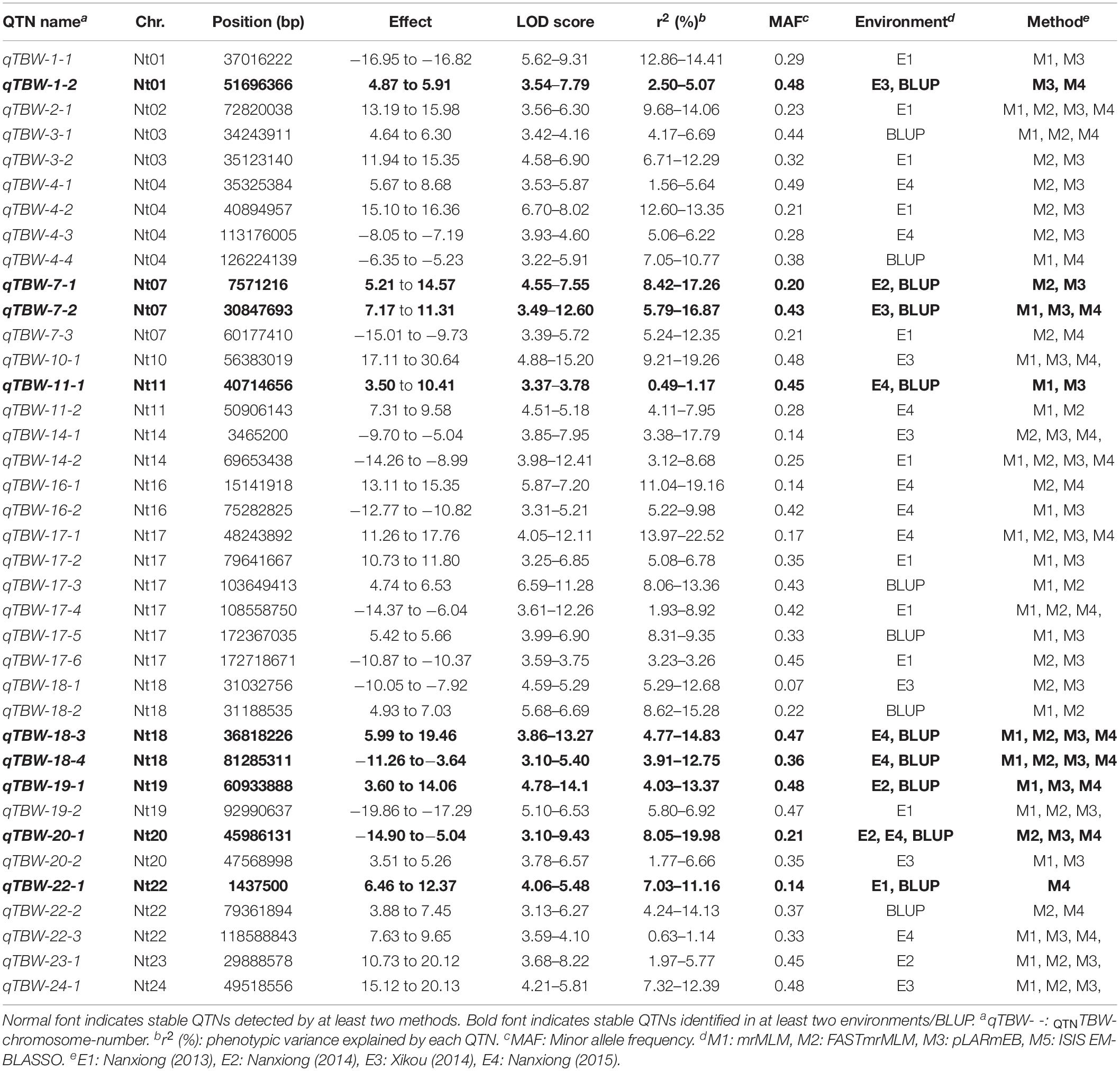
Table 3. Significant QTNs for TBW resistance detected by multiple ML-GWAS methods and/or in multiple environments/BLUP.
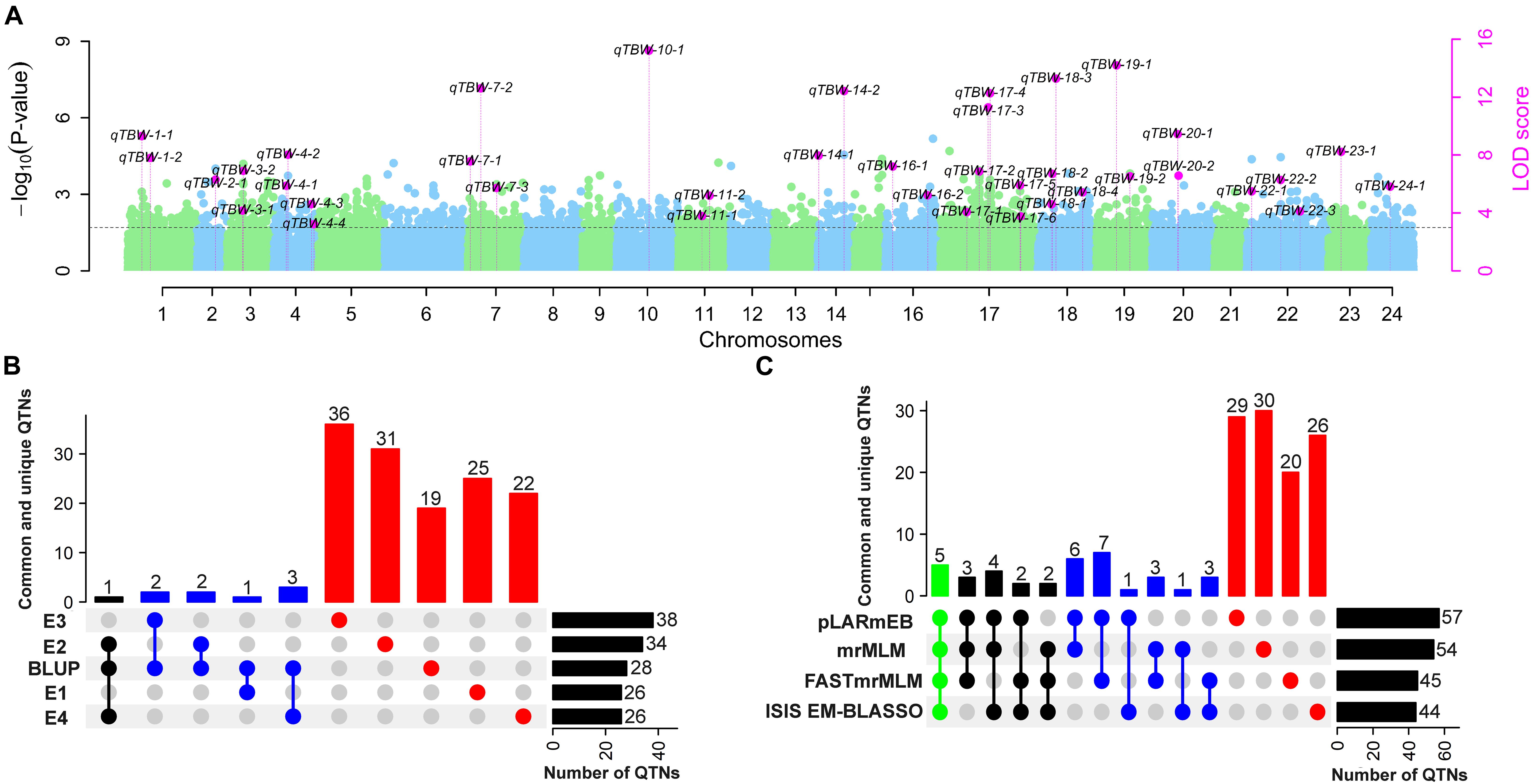
Figure 5. Number of stable QTNs detected for TBW resistance using different ML-GWAS methods in four environments and their BLUP values. (A) Pink dots represent stable QTNs identified by more than two methods and/or two environments/BLUP. Light blue and green dots represent the –log10 (P-value) of 126,602 markers on 24 chromosomes in the first step of ML-GWAS methods. The threshold level LOD ≥ 3 (P = 0.0002) was used to determine significant QTNs and estimated by empirical Bayesian in the second step of ML-GWAS methods; (B) Common and unique QTNs identified in E1–E4 and BLUP; (C) Common and unique QTNs detected by mrMLM, FastmrMLM, pLARmEB, and ISIS EM-BLASSO. The connected circles below the histogram show overlapping QTNs; green, black, and blue represent four, three, and two overlapping QTNs, respectively, while red represents unique QTNs. Horizontal bars show the total number of QTN set sizes.
Identification of Superior Alleles
The 38 stable QTNs were used to identify superior alleles for TBW resistance using QTN effect values. Thirty-eight superior alleles were identified and significantly (P < 0.05) differed from the alternative alleles (Supplementary Table 4). The TBW-DI values for the accessions with superior alleles ranged from 31.73 to 46.91, while those for the alternative alleles ranged from 47.1 to 72.44 (Supplementary Table 4). For example, qTBW-14-2 had CC as a superior allele and TT as an alternative allele, and TBW-DI values of 44.22 and 58.04, respectively. Similarly, three stable QTNs, qTBW-20-2, qTBW-22-3, and qTBW-23-1, had TT, CC, and TT superior alleles with TBW-DI values <45 (Supplementary Table 4). Moreover, all TBW-DI values for superior alleles of the 38 stable QTNs were <47; according to the disease index, these alleles are considered TBW-resistant superior alleles. A negative correlation was detected between the number of superior alleles and TBW-DI (r = −0.83, p ≤ 1 × 10–5) (Figure 6A). A similar trend was found between the number of superior alleles and TBW-DI in E1 (−0.53, p ≤ 1 × 10–5), E2 (r = −0.72, p ≤ 1 × 10–4), E3 (r = −0.61, p ≤ 1 × 10–6), E4 (r = −0.63, p ≤ 1 × 10–4), and BLUP (r = −0.81, p ≤ 1 × 10–5) (Figures 6B–F). Based on these results, the superior alleles can be used in MAS for TBW resistance in tobacco.
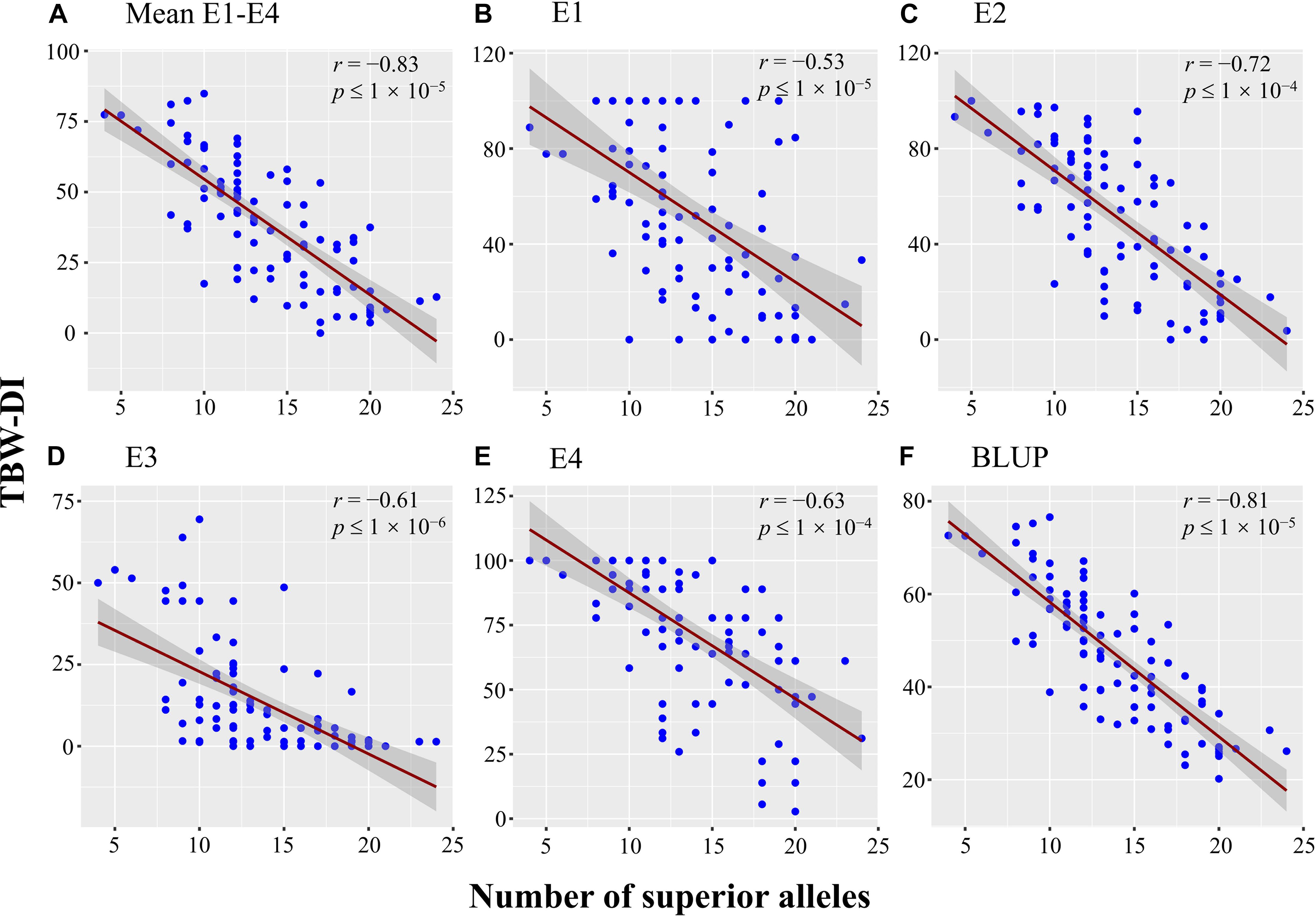
Figure 6. Scatter plot with fitted regression lines and 95% confidence interval bands, showing a negative correlation between TBW-DI in (A) Mean E1–E4, (B) E1, (C) E2, (D) E3, (E) E4, (F) BLUP and number of alleles. The E1, E2, E3, and E4 denote the populations planted in Nanxiong (2013), Nanxiong (2014), Xikou (2014), and Nanxiong (2015), respectively. The X-axis represents the number of superior alleles, while the Y-axis corresponds to the TBW-DI.
Distribution of Superior Alleles and Prediction of Best Cross Combination for Tobacco Bacterial Wilt Resistance
The number of TBW-resistant superior alleles for each stable QTN in the 94 accessions ranged from 1 (1.06%) to 91 (96.81%). Among the 38 stable QTNs, 11 had >50% superior alleles, and 27 had <50% superior alleles; only four had >80% superior alleles (Supplementary Table 4). The number of superior alleles for each accession ranged from 4 (10.52%) to 24 (63.15%); 15 accessions had >50% superior alleles, and 79 accessions had <50% superior alleles. In addition, K326, Ruyuan No. 1, MC133, C176, ROX28, CO258, H66B, and RG17 had 24, 21, 20, 20, 20, 20, 18, and 18 TBW-resistant superior alleles. These accessions can be used in tobacco breeding programs to increase the number of superior alleles for TBW resistance in one cultivar. For example, a cross between K326 (24 TBW-resistant superior alleles) and C176 (20 TBW-resistant superior alleles) could produce offspring with 30 superior alleles. Based on this information, we predicted the following best parental cross combinations: K326 × C176, MC133 × H66B, MC133 × Ruyuan No. 1, CO258 × ROX28, and CO258 × RG17.
Candidate Genes Underlying Stable Quantitative Trait Nucleotides for Tobacco Bacterial Wilt Resistance
The genomic regions (±95 kb around the associated QTNs) of QTN-linked candidate genes were adopted according to the genome-wide LD decay distance (about 94.5 kb) in this study. As a result, 642 genes were presented in the above regions of the 38 stable QTNs, according to N. tabacum reference genome Nitab4.5, of which 489 were homologous in Arabidopsis. These 489 genes belonged to different functional categories: stress and defense-related, unknown functional families, hormonal signaling, transcription, translation, transporter, and cell metabolism. Further, the Kyoto encyclopedia of genes and genomes (KEGG, see text footnote 4) analysis of the above 642 genes indicated that 74 genes were involved in 19 KEGG pathways (adjusted P-value ≤ 0.05), including flavonoid biosynthesis, glutathione metabolism, MAPK signaling pathway, phenylpropanoid biosynthesis, phosphatidylinositol signaling system, gingerol biosynthesis, and plant–pathogen interactions. Finally, 52 genes were considered potential candidate genes associated with disease resistance in plants (Supplementary Table 5) based on functional annotation, homologous to known genes, and pathway enrichment analysis. For example, candidate gene Nitab4.5_0002694g0030 underlying the stable QTN qTBW-3-2 was homologous to AT4G34050 (CCoAOMT1), which annotates caffeoyl-CoA O-methyltransferase and phenylpropanoid in Arabidopsis. Similarly, Nitab4.5_0000274g0070 and Nitab4.5_0000123g0350, located near qTBW-17-3 and qTBW-24-1, respectively, corresponded with Arabidopsis thaliana genes AT5G15130 (WRKY72) and AT2G40890 (CYP98A3) involved in diarylheptanoid, stilbenoid, gingerol biosynthesis, phenylpropanoid biosynthesis, and flavonoid biosynthesis (Supplementary Table 5). Therefore, these candidate genes may regulate tobacco growth to increase plant defense and disease resistance.
Discussion
To study the mechanism of TBW resistance in tobacco plants, GWAS is a useful tool for dissecting the genetic basis and candidate genes for the natural variations in a targeted quantitative trait (Zhang et al., 2019). Here, four ML-GWAS methods were used to analyze TBW-DI and BLUP values using 126,602 high-density SNP markers. We identified 38 stable QTNs, superior alleles, and 52 candidate genes associated with TBW resistance. The markers associated with TBW resistance can be used to develop resistant varieties.
Population Selection for Association Mapping
To gain some insight, we evaluated 94 tobacco accessions for TBW in four different environments at two locations; the variance components indicated that TBW is affected by environmental conditions. The broad-sense heritability for TBW-DI was moderate (61–81%) and differed between environments (Table 1). These observations are similar to other studies in tobacco (Nishi et al., 2003; Qian et al., 2013; Lan et al., 2014), including some heritability problems (Gao et al., 2010).
Genetic diversity in modern tobacco cultivars is low (Wernsman, 1999; Leng et al., 2010); only a few genotypes are ancestors of most cultivars. Thus, it is challenging to assemble a natural population with rich genetic diversity. However, based on the 126,602 SNPs, we found 24.46 kb/SNP in the whole genome (Supplementary Table 3 and Figure 2C) and higher coverage density than reported elsewhere (Leng et al., 2010; Wang et al., 2021). We also found an LD decay distance of ∼94.56 kb (Figure 3D), much smaller than that reported by Fricano et al. (2012). STRUCTURE analysis identified two subpopulations in the panel (Figure 3B), and clustering results showed that the genetic background of 94 accessions is diverse and complex, consistent with most studies on tobacco (Fricano et al., 2012; Dadras et al., 2014; Wang et al., 2021). There are high genome-wide SNP variations in the panel used in this study that are suitable for association mapping (Mackay and Powell, 2007; Wang et al., 2009; Ikram et al., 2020).
Statistical Power of Multi-Locus Genome-Wide Association Methods and Significance of Stable Quantitative Trait Nucleotides
The four ML-GWAS methods—FASTmrMLM, ISIS EM-BLASSO, mrMLM, and pLARmEB—identified 45, 44, 54, and 57 significant QTNs for TBW-DI, with small to large effects (Table 2). While the statistical power of QTN detection has improved, after controlling the polygenic background, most small-effect QTNs of complex traits are not captured by SL-GWAS methods (Zhang et al., 2019). However, ML-GWAS studies have shown that these methods have high-resolution power; e.g., Hou et al. (2018) used SL- and ML-GWAS models to identify 20 QTNs related to the drought stress response using mrMLM, but only three by EMMAX, suggesting that the ML-GWAS methods are more powerful. Likewise, Su et al. (2018) reported that multi-locus methods are robust and more potent than MLM method.
In this study, 38 QTNs were identified in more than two environments and/or ML-GWAS models (Table 3 and Figures 4, 5); nine were considered environment-stable, 37 were considered method-stable, and eight were considered both. In previous studies, environmental-stable QTNs have gained more attention than method-stable QTNs (Zhang et al., 2019), but recent studies have shown that QTNs detected using multiple methods are also reliable (Cui et al., 2018; Li et al., 2018; Peng et al., 2018; Chaurasia et al., 2020; Ikram et al., 2020). For example, 42 QTNs related to salt stress in wheat were detected using multiple methods (Chaurasia et al., 2020). Hence, environment-stable and method-stable QTNs are more reliable for breeding programs, with similar results reported in other crop plants (Zhang et al., 2019), including soybean (Ikram et al., 2020), maize (Ma et al., 2018), wheat (Chaurasia et al., 2020; Danakumara et al., 2021), and rice (Verma et al., 2021). In addition, the 38 stable QTNs identified in our study are considered novel as they are not located in the genomic region of previously reported QTLs for TBW.
Application of Superior Alleles in Breeding Programs
Tobacco cultivars exhibit low genetic diversity, and their existing alleles may not improve TBW resistance (Qian et al., 2013; Wang et al., 2021). New alleles identified through germplasm screening will improve TBW resistance. Marker-assisted breeding has dramatically improved breeding efficiency. The alleles of stable QTNs significantly differed, with 4–24 superior alleles for TBW resistance found in the 94 accessions (Supplementary Table 4). Eight resistant genotypes were identified with superior alleles for TBW resistance that can be used to breed highly resistant varieties. The best cross combinations were identified based on these superior alleles for TBW resistance, similar to previous studies for complex traits in different crops (Wang et al., 2006; Zeng et al., 2017). The concept of molecular design breeding (Peleman and Van Der Voort, 2003; Zeng et al., 2017) was used by Tian and his co-workers to successfully selected the LYP9 rice variety with high yield and quality by transferring several alleles into the new cultivar (Tian et al., 2009; Zeng et al., 2017).
Candidate Genes for Tobacco Bacterial Wilt Resistance
The identification of candidate genes associated with quantitatively inherited traits is challenging in genetic research. The present study identified 52 candidate genes underlying the 38 stable QTNs, based on homology with Arabidopsis and KEGG pathways for plant defense and disease resistance (Supplementary Table 5). The gene Nitab4.5_0002694g0030 encodes the caffeoyl-CoA O-methyltransferase-like protein that may prevent TBW by regulating the phenylpropanoid pathway and lignin production (Yang et al., 2017). Nitab4.5_0001039g0060 is homologous to CYCD3;2 in Arabidopsis (Supplementary Table 5), and CYCD3 genes appear to be positive regulators of plant resistance because mutations in the target gene conferred increased disease susceptibility to plant pathogens (Hamdoun et al., 2016). Similarly, Nitab4.5_0000337g0220 encodes BR-signaling kinase 1 (BSK1), and a bsk1-1 mutation displayed enhanced susceptibility to a range of pathogens, demonstrating that BSK1 plays an important role in plant immunity (Shi et al., 2013). BSK1 is a substrate of the brassinosteroid receptor BRI1 and plays a critical role in brassinosteroid signaling to regulate plant immunity (Tang et al., 2008; Sun and Li, 2017). Most of the candidate genes (Nitab4.5_0000430g0170, Nitab4.5_0000016g0210, Nitab4.5_0002576g0050, Nitab4.5_0000553g0050, and Nitab4.5_0002890g0050) were involved in signaling pathways, and their homologous genes (MKK9, bZIP65, AT1G17345, EPF2, and EMB14, respectively) in A. thaliana play a significant role in plant disease resistance (Feys and Parker, 2000; Eshraghi et al., 2014; Liu and Lam, 2019). Nitab4.5_0000641g0050—involved in the glutathione metabolism pathway and glutathione—is the most abundant antioxidant in cells and crucial for life processes. It protects DNA, biomolecules, and proteins against oxidative damage, which favored resistance against environmental stresses (Freeman et al., 2004; Li et al., 2021) and increased resistance in eggplant after infection with R. solanacearum (Avinash et al., 2017). TFs are essential regulatory genes in plants, and WRKY TFs play a significant role in the immune response of plants to various biological stresses (Chen et al., 2017). Two WRKY TF genes (Nitab4.5_0000929g0030 and Nitab4.5_0000274g0070) were identified in this study (Supplementary Table 5). Numerous research findings have shown that WRKY22 and WRKY40 TF genes have positive regulatory effects on the resistance of Solanaceae crops to bacterial wilt (Dang et al., 2014; Cai et al., 2015; Hussain et al., 2018). These results suggest that WRKY genes could be important positive regulators of tobacco plant resistance against bacterial wilt (Hussain et al., 2018). The relationship between these candidate genes and TBW resistance needs to be verified.
The fundamental task is to find excellent genes or QTNs related to the target trait to achieve the precise breeding, design, and breed aggregate of excellent genes/alleles. Most researchers have only used linkage analysis or association analysis to identify QTLs or SNPs/QTNs. Previous studies only contain basic theoretical results (Nishi et al., 2003; Lan et al., 2014), with few researchers using these results to screen material. However, pleiotropic genes regulate quantitative traits, their genetic laws are complex (Yang et al., 2012), and it is difficult for a single QTL to reflect the advantages of traits. Here, 38 stable QTNs and 52 candidate genes were detected by GWAS, filling a gap and laying a theoretical foundation for subsequent design and breeding. Using research results to evaluate material phenotypes will assist in selecting material containing multiple superior alleles to increase the probability of selecting material with desired traits, which has important implications for molecular marker-assisted screening. Therefore, this study screened tobacco varieties that carry the target QTN alleles and candidate genes that could be used as resistant parents for gene pyramiding to improve TBW resistance.
Conclusion
In this study, we used GBS technology for the first time to conduct GWAS for TBW resistance to identify QTNs, superior alleles, and candidate genes for breeding highly resistant tobacco varieties. We demonstrated that TBW resistance is genetically complex. We identified 38 novel stable QTNs with significantly different alleles in the association panel. We predicted the five best parental cross combinations based on superior allele information for developing tobacco varieties that are highly resistant to R. solanacearum. Moreover, 52 candidate genes were associated with TBW resistance. The results from this study serve as the basis for resistance gene cloning and further understanding of the molecular mechanisms of tobacco resistance to R. solanacearum.
Data Availability Statement
The sequence read data from the genotyping-by-sequencing (GBS) for the 94 sequenced tobacco accession are available in NCBI Sequence Read Archive (SRA) under the accession number PRJNA759331 (https://www.ncbi.nlm.nih.gov/sra/PRJNA759331).
Author Contributions
PG conceived and designed the experiments. RLa, RLi, YX, MI, and WZ performed the experiments and analyzed data. YX, MI, RLi, QY, ZZ, and WZ contributed to reagents, materials, and analysis tools. RLa, MI, RLi, YX, KS, and PG wrote the manuscript. All authors have read and agreed to the published version of the manuscript.
Funding
The authors declare that this study received funding from the Scientific and Technological Projects of Guangdong Tobacco Corporation (201403 and 201702). The funder was not involved in the study design, data collection, analysis, interpretation of data, the writing of this article, or the decision to submit it for publication.
Conflict of Interest
WZ is employed by Nanxiong Research Institute of Guangdong Tobacco Co., Ltd., Nanxiong, China.
The remaining authors declare that the research was conducted in the absence of any commercial or financial relationships that could be construed as a potential conflict of interest.
Publisher’s Note
All claims expressed in this article are solely those of the authors and do not necessarily represent those of their affiliated organizations, or those of the publisher, the editors and the reviewers. Any product that may be evaluated in this article, or claim that may be made by its manufacturer, is not guaranteed or endorsed by the publisher.
Supplementary Material
The Supplementary Material for this article can be found online at: https://www.frontiersin.org/articles/10.3389/fpls.2021.744175/full#supplementary-material
Footnotes
- ^ http://www.R-project.org/
- ^ https://cran.r-project.org/web/packages/mrMLM/index.html
- ^ https://solgenomics.net/organism/Nicotiana_tabacum/genome
- ^ http://kobas.cbi.pku.edu.cn/kobas3
References
Avinash, P., Umesha, S., and Marahel, S. (2017). Role of hydrogen peroxide and ascorbate-glutathione pathway in host resistance to bacterial wilt of eggplant. Russ. J. Plant Physiol. 64, 375–385. doi: 10.1134/S1021443717030049
Bates, D., Mächler, M., Bolker, B., and Walker, S. (2014). Fitting linear mixed-effects models using lme4. J. Stat. Softw. 67, 1–48. doi: 10.18637/jss.v067.i01
Bradbury, P. J., Zhang, Z., Kroon, D. E., Casstevens, T. M., Ramdoss, Y., and Buckler, E. S. (2007). TASSEL: software for association mapping of complex traits in diverse samples. Bioinformatics 23, 2633–2635. doi: 10.1093/bioinformatics/btm308
Buckler, E. S., and Thornsberry, J. M. (2002). Plant molecular diversity and applications to genomics. Curr. Opin. Plant Biol. 5, 107–111. doi: 10.1016/S1369-5266(02)00238-8
Cai, H., Yang, S., Yan, Y., Xiao, Z., Cheng, J., Wu, J., et al. (2015). CaWRKY6 transcriptionally activates CaWRKY40, regulates Ralstonia solanacearum resistance, and confers high temperature and high-humidity tolerance in pepper. J. Exp. Bot. 66, 3163–3174. doi: 10.1093/jxb/erv125
Chaurasia, S., Singh, A. K., Songachan, L. S., Sharma, A. D., Bhardwaj, R., and Singh, K. (2020). Multi-locus genome-wide association studies reveal novel genomic regions associated with vegetative stage salt tolerance in bread wheat (Triticum aestivum L.). Genomics 112, 4608–4621. doi: 10.1016/j.ygeno.2020.08.006
Chen, F., Hu, Y., Vannozzi, A., Wu, K., Cai, H., Qin, Y., et al. (2017). The WRKY transcription factor family in model plants and crops. CRC. Crit. Rev. Plant Sci. 36, 311–335. doi: 10.1080/07352689.2018.1441103
Cui, Y., Zhang, F., and Zhou, Y. (2018). The application of multi-locus GWAS for the detection of salt-tolerance loci in rice. Front. Plant Sci. 9:1464. doi: 10.3389/fpls.2018.01464
Dadras, A. R., Sabouri, H., Nejad, G. M., Sabouri, A., and Shoai-Deylami, M. (2014). Association analysis, genetic diversity and structure analysis of tobacco based on AFLP markers. Mol. Biol. Rep. 41, 3317–3329. doi: 10.1007/s11033-014-3194-6
Danakumara, T., Kumari, J., Singh, A. K., Sinha, S. K., Pradhan, A. K., Sharma, S., et al. (2021). Genetic dissection of seedling root system architectural traits in a diverse panel of hexaploid wheat through multi-locus genome-wide association mapping for improving drought tolerance. Int. J. Mol. Sci. 22:7188. doi: 10.3390/ijms22137188
Dang, F., Wang, Y., She, J., Lei, Y., Liu, Z., Eulgem, T., et al. (2014). Overexpression of CaWRKY27, a subgroup IIe WRKY transcription factor of Capsicum annuum, positively regulates tobacco resistance to Ralstonia solanacearum infection. Physiol. Plant. 150, 397–411. doi: 10.1111/ppl.12093
Denny, T. (2006). “Plant pathogenic Ralstonia species,” in Plant-Associated Bacteria, ed. S. S. Gnanamanickam (Dordrecht: Springer Netherlands), 573–644.
Drake-Stowe, K., Bakaher, N., Goepfert, S., Philippon, B., Mark, R., Peterson, P., et al. (2017). Multiple disease resistance loci affect soilborne disease resistance in tobacco (Nicotiana tabacum). Phytopathology 107, 1055–1061. doi: 10.1094/PHYTO-03-17-0118-R
Earl, D. A., and vonHoldt, B. M. (2012). STRUCTURE HARVESTER: a website and program for visualizing STRUCTURE output and implementing the Evanno method. Conserv. Genet. Resour. 4, 359–361. doi: 10.1007/s12686-011-9548-7
Edwards, K. D., Fernandez-Pozo, N., Drake-Stowe, K., Humphry, M., Evans, A. D., Bombarely, A., et al. (2017). A reference genome for Nicotiana tabacum enables map-based cloning of homeologous loci implicated in nitrogen utilization efficiency. BMC Genomics 18:448. doi: 10.1186/s12864-017-3791-6
Elshire, R. J., Glaubitz, J. C., Sun, Q., Poland, J. A., Kawamoto, K., Buckler, E. S., et al. (2011). A robust, simple genotyping-by-sequencing (GBS) approach for high diversity species. PLoS One 6:e19379. doi: 10.1371/journal.pone.0019379
Eshraghi, L., Anderson, J. P., Aryamanesh, N., McComb, J. A., Shearer, B., and Giles, G. E. (2014). Defence signalling pathways involved in plant resistance and phosphite-mediated control of Phytophthora Cinnamomi. Plant Mol. Biol. Rep. 32, 342–356. doi: 10.1007/s11105-013-0645-5
Evanno, G., Regnaut, S., and Goudet, J. (2005). Detecting the number of clusters of individuals using the software STRUCTURE: a simulation study. Mol. Ecol. 14, 2611–2620. doi: 10.1111/j.1365-294X.2005.02553.x
Feys, B. J., and Parker, J. E. (2000). Interplay of signaling pathways in plant disease resistance. Trends Genet. 16, 449–455. doi: 10.1016/S0168-9525(00)02107-7
Flint-Garcia, S. A., Thornsberry, J. M., and Edward, S. B. (2003). Structure of linkage disequilibrium in plants. Annu. Rev. Plant Biol. 54, 357–374. doi: 10.1146/annurev.arplant.54.031902.134907
Freeman, J. L., Persans, M. W., Nieman, K., Albrecht, C., Peer, W., Pickering, I. J., et al. (2004). Increased glutathione biosynthesis plays a role in nickel tolerance in Thlaspi nickel hyperaccumulators W inside box sign. Plant Cell 16, 2176–2191. doi: 10.1105/tpc.104.023036
Fricano, A., Bakaher, N., Corvo, M. D., Piffanelli, P., Donini, P., Stella, A., et al. (2012). Molecular diversity, population structure, and linkage disequilibrium in a worldwide collection of tobacco (Nicotiana tabacum L.) germplasm. BMC Genet. 13:18. doi: 10.1186/1471-2156-13-18
Gao, J. M., Wang, Z. D., and Zhang, W. X. (2010). Genetic analysis on resistance to bacterial wilt in oriental tobacco. Chin. Tob. Sci 31, 1–4.
Gutterson, N., and Reuber, T. L. (2004). Regulation of disease resistance pathways by AP2/ERF transcription factors. Curr. Opin. Plant Biol. 7, 465–471. doi: 10.1016/j.pbi.2004.04.007
Hamdoun, S., Zhang, C., Gill, M., Kumar, N., Churchman, M., Larkin, J. C., et al. (2016). Differential roles of two homologous cyclin-dependent kinase inhibitor genes in regulating cell cycle and innate immunity in arabidopsis1. Plant Physiol. 170, 515–527. doi: 10.1104/pp.15.01466
Hou, S., Zhu, G., Li, Y., Li, W., Fu, J., Niu, E., et al. (2018). Genome-wide association studies reveal genetic variation and candidate genes of drought stress related traits in cotton (Gossypium hirsutum l.). Front. Plant Sci. 9:1276. doi: 10.3389/fpls.2018.01276
Hussain, A., Li, X., Weng, Y., Liu, Z., Ashraf, M., Noman, A., et al. (2018). CaWRKY22 acts as a positive regulator in pepper response to Ralstonia Solanacearum by constituting networks with CaWRKY6, CaWRKY27, CaWRKY40, and CaWRKY58. Int. J. Mol. Sci. 19:1426. doi: 10.3390/ijms19051426
Hyun, D. Y., Sebastin, R., Lee, G., Lee, K. J., Kim, S., Yoo, E., et al. (2021). Genome-wide SNP markers for genotypic and phenotypic differentiation of melon (Cucumis melo L.) Varieties Using Genotyping-by-Sequencing. Int. J. Mol. Sci. 22:6722.
Ikram, M., Han, X., Zuo, J. F., Song, J., Han, C. Y., Zhang, Y. W., et al. (2020). Identification of QTNs and their candidate genes for 100-seed weight in soybean (Glycine max L.) using multi-locus genome-wide association studies. Genes (Basel) 11, 1–22. doi: 10.3390/genes11070714
Jiang, G., Wei, Z., Xu, J., Chen, H., Zhang, Y., She, X., et al. (2017). Bacterial wilt in China: History, current status, and future perspectives. Front. Plant Sci. 8:1549. doi: 10.3389/fpls.2017.01549
Jing, Y., Teng, W., Qiu, L., Zheng, H., Li, W., Han, Y., et al. (2021). Genetic dissection of soybean partial resistance to sclerotinia stem rot through genome wide association study and high throughput single nucleotide polymorphisms. Genomics 113, 1262–1271. doi: 10.1016/j.ygeno.2020.10.042
Kuchel, H., Ye, G., Fox, R., and Jefferies, S. (2005). Genetic and economic analysis of a targeted marker-assisted wheat breeding strategy. Mol. Breed. 16, 67–78. doi: 10.1007/s11032-005-4785-7
Kuhn, H., Lorek, J., Kwaaitaal, M., Consonni, C., Becker, K., Micali, C., et al. (2017). Key components of different plant defense pathways are dispensable for powdery mildew resistance of the arabidopsis mlo2 mlo6 mlo12 triple mutant. Front. Plant Sci. 8:1006. doi: 10.3389/fpls.2017.01006
Lan, T., Zheng, S., Yang, L., Wu, S., Wang, B., Zhang, S., et al. (2014). Mapping of quantitative trait loci conferring resistance to bacterial wilt in tobacco (Nicotiana tabacum L.). Plant Breed. 133, 672–677. doi: 10.1111/pbr.12202
Lee, S. J., Ban, S. H., Kim, G. H., Kwon, S. 2nd, Kim, J. H., and Choi, C. (2017). Identification of potential gene-associated major traits using GBS-GWAS for Korean apple germplasm collections. Plant Breed. 136, 977–986. doi: 10.1111/pbr.12544
Leng, X., Xiao, B., Wang, S., Gui, Y., Wang, Y., Lu, X., et al. (2010). Identification of NBS-type resistance gene homologs in tobacco genome. Plant Mol. Biol. Rep. 28, 152–161. doi: 10.1007/s11105-009-0134-z
Li, C., Fu, Y., Sun, R., Wang, Y., and Wang, Q. (2018). Single-locus and multi-locus genome-wide association studies in the genetic dissection of fiber quality traits in upland cotton (Gossypium hirsutum L.). Front. Plant Sci. 9:1083. doi: 10.3389/fpls.2018.01083
Li, H., and Durbin, R. (2009). Fast and accurate short read alignment with Burrows-Wheeler transform. Bioinformatics 25, 1754–1760. doi: 10.1093/bioinformatics/btp324
Li, T., Ma, X., Li, N., Zhou, L., Liu, Z., Han, H., et al. (2017). Genome-wide association study discovered candidate genes of Verticillium wilt resistance in upland cotton (Gossypium hirsutum L.). Plant Biotechnol. J. 15, 1520–1532. doi: 10.1111/pbi.12734
Li, Y., Feng, J., Liu, H., Wang, L., Hsiang, T., Li, X., et al. (2016). Genetic diversity and pathogenicity of ralstonia solanacearum causing tobacco bacterial wilt in China. Plant Dis. 100, 1288–1296. doi: 10.1094/PDIS-04-15-0384-RE
Li, Y. Y., Wang, L., Sun, G. W., Li, X. H., Chen, Z. G., Feng, J., et al. (2021). Digital gene expression analysis of the response to Ralstonia solanacearum between resistant and susceptible tobacco varieties. Sci. Rep. 11:3887. doi: 10.1038/s41598-021-82576-8
Lipka, A. E., Tian, F., Wang, Q., Peiffer, J., Li, M., Bradbury, P. J., et al. (2012). GAPIT: genome association and prediction integrated tool. Bioinformatics 28, 2397–2399. doi: 10.1093/bioinformatics/bts444
Liu, J. Z., and Lam, H. M. (2019). Signal transduction pathways in plants for resistance against pathogens. Int. J. Mol. Sci. 20:2335. doi: 10.3390/ijms20092335
Ma, L., Liu, M., Yan, Y., Qing, C., Zhang, X., Zhang, Y., et al. (2018). Genetic dissection of maize embryonic callus regenerative capacity using multi-locus genome-wide association studies. Front. Plant Sci. 9:561. doi: 10.3389/fpls.2018.00561
Mackay, I., and Powell, W. (2007). Methods for linkage disequilibrium mapping in crops. Trends Plant Sci. 12, 57–63. doi: 10.1016/j.tplants.2006.12.001
McKenna, A., Hanna, M., Banks, E., Sivachenko, A., Cibulskis, K., Kernytsky, A., et al. (2010). The genome analysis toolkit: a MapReduce framework for analyzing next-generation DNA sequencing data. Genome Res. 20, 1297–1303. doi: 10.1101/gr.107524.110
Nakaya, A., and Isobe, S. N. (2012). Will genomic selection be a practical method for plant breeding? Ann. Bot. 110, 1303–1316. doi: 10.1093/aob/mcs109
Ni, C., Xu, X. H., and Zhang, X. W. (2011). Genetic analysis of easy curing potential in flue-cured tobacco with the mixed major-gene plus polygene inheritance model. Chin. Tob. Sci 32, 1–4.
Nishi, T., Tajima, T., Noguchi, S., Ajisaka, H., and Negishi, H. (2003). Identification of DNA markers of tobacco linked to bacterial wilt resistance. Theor. Appl. Genet. 106, 765–770. doi: 10.1007/s00122-002-1096-9
Peleman, J. D., and Van Der Voort, J. R. (2003). Breeding by design. Trends Plant Sci. 8, 330–334. doi: 10.1016/S1360-1385(03)00134-1
Peng, Y., Liu, H., Chen, J., Shi, T., Zhang, C., Sun, D., et al. (2018). Genome-wide association studies of free amino acid levels by six multi-locus models in bread wheat. Front. Plant Sci. 9:1196. doi: 10.3389/fpls.2018.01196
Pritchard, J. K., Stephens, M., and Donnelly, P. (2000). Inference of population structure using multi-locus genotype data. Genetics 155, 945–959. doi: 10.1093/genetics/155.2.945
Purcell, S., Neale, B., Todd-Brown, K., Thomas, L., Ferreira, M. A. R., Bender, D., et al. (2007). PLINK: a tool set for whole-genome association and population-based linkage analyses. Am. J. Hum. Genet. 81, 559–575. doi: 10.1086/519795
Qian, Y., Wang, X., Wang, D., Zhang, L., Zu, C., Gao, Z., et al. (2013). The detection of QTLs controlling bacterial wilt resistance in tobacco (N. tabacum L.). Euphytica 192, 259–266. doi: 10.1007/s10681-012-0846-2
Ribaut, J. M., de Vicente, M. C., and Delannay, X. (2010). Molecular breeding in developing countries: challenges and perspectives. Curr. Opin. Plant Biol. 13, 213–218. doi: 10.1016/j.pbi.2009.12.011
Sakiroglu, M., and Brummer, E. C. (2017). Identification of loci controlling forage yield and nutritive value in diploid alfalfa using GBS-GWAS. Theor. Appl. Genet. 130, 261–268. doi: 10.1007/s00122-016-2782-3
Sharma, S., Katoch, V., and Banyal, D. K. (2021). Review on harnessing biotechnological tools for the development of stable bacterial wilt resistant solanaceous vegetable crops. Sci. Hortic. (Amsterdam). 285:110158. doi: 10.1016/j.scienta.2021.110158
Shi, H., Yan, H., Li, J., and Tang, D. (2013). BSK1, a receptor-like cytoplasmic kinase, involved in both BR signaling and innate immunity in Arabidopsis. Plant Signal. Behav. 8:e24996. doi: 10.4161/psb.24996
Smith, T. E., and Clayton, E. E. (1948). Inheritance of resistance to bacterial wilt in tobacco. J. Agric. Res. 76, 27–32.
Su, J., Ma, Q., Li, M., Hao, F., and Wang, C. (2018). Multi-locus genome-wide association studies of fiber-quality related traits in Chinese early-maturity upland cotton. Front. Plant Sci. 9:1169. doi: 10.3389/fpls.2018.01169
Sun, C., and Li, J. (2017). Biosynthesis, catabolism, and signal transduction of brassinosteroids. Zhiwu Shengli Xuebao/Plant Physiol. J. 53, 291–307. doi: 10.13592/j.cnki.ppj.2017.1002
Tamba, C. L., Ni, Y.-L., and Zhang, Y.-M. (2017). Iterative sure independence screening EM-Bayesian LASSO algorithm for multi-locus genome-wide association studies. PLoS Comput. Biol. 13:e1005357. doi: 10.1371/journal.pcbi.1005357
Tamba, C. L., and Zhang, Y.-M. (2018). A fast mrMLM algorithm for multi-locus genome-wide association studies. bioRxiv [preprint] 341784. doi: 10.1101/341784
Tang, W., Kim, T. W., Oses-Prieto, J. A., Sun, Y., Deng, Z., Zhu, S., et al. (2008). BSKs mediate signal transduction from the receptor kinase BRI1 in Arabidopsis. Science (80-.). 321, 557–560. doi: 10.1126/science.1156973
Thapa, R., Singh, J., Gutierrez, B., Arro, J., and Khan, A. (2021). Genome-wide association mapping identifies novel loci underlying fire blight resistance in apple. Plant Genome 14:e20087. doi: 10.1002/tpg2.20087
Tian, Z., Qian, Q., Liu, Q., Yan, M., Liu, X., Yan, C., et al. (2009). Allelic diversities in rice starch biosynthesis lead to a diverse array of rice eating and cooking qualities. Proc. Natl. Acad. Sci. U.S.A. 106, 21760–21765. doi: 10.1073/pnas.0912396106
Verma, R. K., Chetia, S. K., Dey, P. C., Rahman, A., Saikia, S., Sharma, V., et al. (2021). Genome-wide association studies for agronomical traits in winter rice accessions of Assam. Genomics 113, 1037–1047. doi: 10.1016/j.ygeno.2020.11.033
Wang, J., Wan, X., Crossa, J., Crouch, J., Weng, J., Zhai, H., et al. (2006). QTL mapping of grain length in rice (Oryza sativa L.) using chromosome segment substitution lines. Genet. Res. 88, 93–104. doi: 10.1017/S0016672306008408
Wang, K., Li, M., and Hakonarson, H. (2010). ANNOVAR: functional annotation of genetic variants from high-throughput sequencing data. Nucleic Acids Res. 38, e164–e164. doi: 10.1093/nar/gkq603
Wang, M. L., Zhu, C., Barkley, N. A., Chen, Z., Erpelding, J. E., Murray, S. C., et al. (2009). Genetic diversity and population structure analysis of accessions in the US historic sweet sorghum collection. Theor. Appl. Genet. 120, 13–23. doi: 10.1007/s00122-009-1155-6
Wang, S.-B., Feng, J.-Y., Ren, W.-L., Huang, B., Zhou, L., Wen, Y.-J., et al. (2016). Improving power and accuracy of genome-wide association studies via a multi-locus mixed linear model methodology. Sci. Rep. 6:19444. doi: 10.1038/srep19444
Wang, Y., Lv, H., Xiang, X., Yang, A., Feng, Q., Dai, P., et al. (2021). Construction of a SNP fingerprinting database and population genetic analysis of cigar tobacco germplasm resources in China. Front. Plant Sci. 12:618133. doi: 10.3389/fpls.2021.618133
Wernsman, E. A. (1999). An overview of tobacco breeding-past, present, and future. TSRC Sept. 25, 5–35.
Xie, C., Mao, X., Huang, J., Ding, Y., Wu, J., Dong, S., et al. (2011). KOBAS 2.0: a web server for annotation and identification of enriched pathways and diseases. Nucleic Acids Res. 39, W316–W322. doi: 10.1093/nar/gkr483
Xu, S. (2013). Mapping quantitative trait loci by controlling polygenic background effects. Genetics 195, 1209–1222. doi: 10.1534/genetics.113.157032
Yang, G., Li, Y., Wang, Q., Zhou, Y., Zhou, Q., Shen, B., et al. (2012). Detection and integration of quantitative trait loci for grain yield components and oil content in two connected recombinant inbred line populations of high-oil maize. Mol. Breed. 29, 313–333. doi: 10.1007/s11032-011-9548-z
Yang, Q., He, Y., Kabahuma, M., Chaya, T., Kelly, A., Borrego, E., et al. (2017). A gene encoding maize caffeoyl-CoA O-methyltransferase confers quantitative resistance to multiple pathogens. Nat. Genet. 49, 1364–1372. doi: 10.1038/ng.3919
Yu, J., Pressoir, G., Briggs, W. H., Bi, I. V., Yamasaki, M., Doebley, J. F., et al. (2006). A unified mixed-model method for association mapping that accounts for multiple levels of relatedness. Nat. Genet. 38, 203–208. doi: 10.1038/ng1702
Zeng, D., Tian, Z., Rao, Y., Dong, G., Yang, Y., Huang, L., et al. (2017). Rational design of high-yield and superior-quality rice. Nat. Plants 3:17031. doi: 10.1038/nplants.2017.31
Zhang, J., Feng, J. Y., Ni, Y. L., Wen, Y. J., Niu, Y., Tamba, C. L., et al. (2017). PLARmEB: Integration of least angle regression with empirical Bayes for multi-locus genome-wide association studies. Heredity 118, 517–524. doi: 10.1038/hdy.2017.8
Keywords: tobacco germplasm, bacterial wilt resistance, SNP, genome-wide association analysis, quantitative trait nucleotide, superior alleles
Citation: Lai R, Ikram M, Li R, Xia Y, Yuan Q, Zhao W, Zhang Z, Siddique KHM and Guo P (2021) Identification of Novel Quantitative Trait Nucleotides and Candidate Genes for Bacterial Wilt Resistance in Tobacco (Nicotiana tabacum L.) Using Genotyping-by-Sequencing and Multi-Locus Genome-Wide Association Studies. Front. Plant Sci. 12:744175. doi: 10.3389/fpls.2021.744175
Received: 19 July 2021; Accepted: 22 September 2021;
Published: 21 October 2021.
Edited by:
Ezio Portis, University of Turin, ItalyReviewed by:
Shengping Zhang, Institute of Vegetables and Flowers, Chinese Academy of Agricultural Sciences (CAAS), ChinaWen Yao, Henan Agricultural University, China
Copyright © 2021 Lai, Ikram, Li, Xia, Yuan, Zhao, Zhang, Siddique and Guo. This is an open-access article distributed under the terms of the Creative Commons Attribution License (CC BY). The use, distribution or reproduction in other forums is permitted, provided the original author(s) and the copyright owner(s) are credited and that the original publication in this journal is cited, in accordance with accepted academic practice. No use, distribution or reproduction is permitted which does not comply with these terms.
*Correspondence: Peiguo Guo, Z3VvcGdAZ3podS5lZHUuY24=
†These authors have contributed equally to this work