- 1Phenomics Laboratory, Department of Plant Resources and Environment, Jeju National University, Jeju, Republic of Korea
- 2Department of Electronic Engineering, Faculty of Applied Energy System, Jeju National University, Jeju, Republic of Korea
- 3Department of Biology and Environmental Engineering, University of Craiova, Craiova, Romania
- 4National Institute of Agricultural Sciences, Rural Development Administration (RDA), Jeonju, Republic of Korea
Traditional farming methods, effective for generations, struggle to meet rising global food demands due to limitations in productivity, efficiency, and sustainability amid climate change and resource scarcity. Precision agriculture presents a viable solution by optimizing resource use, enhancing efficiency, and fostering sustainable practices through data-driven decision-making supported by advanced sensors and Internet of Things (IoT) technologies. This review examines various smart sensors used in precision agriculture, including soil sensors for moisture, pH, and plant stress sensors etc. These sensors deliver real-time data that enables informed decision-making, facilitating targeted interventions like optimized irrigation, fertilization, and pest management. Additionally, the review highlights the transformative role of IoT in precision agriculture. The integration of sensor networks with IoT platforms allows for remote monitoring, data analysis via artificial intelligence (AI) and machine learning (ML), and automated control systems, enabling predictive analytics to address challenges such as disease outbreaks and yield forecasting. However, while precision agriculture offers significant benefits, it faces challenges including high initial investment costs, complexities in data management, needs for technical expertise, data security and privacy concerns, and issues with connectivity in remote agricultural areas. Addressing these technological and economic challenges is essential for maximizing the potential of precision agriculture in enhancing global food security and sustainability. Therefore, in this review we explore the latest trends, challenges, and opportunities associated with IoT enabled smart sensors in precision agriculture.
1 Introduction
Traditional agricultural practices have been utilized for generations, leveraging local knowledge and techniques passed down through families. This small-scale farming often includes strategies such as crop rotation (Lundborg, 1990; Vasilescu et al., 2023). However, a significant drawback of traditional agriculture is its reliance on outdated methods, which can limit productivity and efficiency. Farmers may experience challenges such as lower crop yields stemming from rigid crop rotations and inadequate pest management techniques (Jain, 2012). Moreover, traditional practices are typically more vulnerable to climate variability, making crops susceptible to extreme weather events and pest outbreaks. As a result, these methods struggle to meet the growing demands of a global population, prompting a need for more sustainable and productive farming approaches (Janc et al., 2019). The shift to precision agriculture represents a viable solution, enhancing farming efficiency through technology and data analytics. This modern approach optimizes resource use, increases yields, and promotes sustainability (Charania and Li, 2020; Karunathilake et al., 2023) Precision agriculture enables real-time monitoring and targeted interventions, allowing farmers to better adapt to climate change and improve economic viability while minimizing environmental impacts compared to traditional practices (Lipper et al., 2014; Bogoviz et al., 2023; Gemtou et al., 2024).
Precision agriculture represents a significant advancement in the modern agricultural sector, highlighting the increasing need to enhance food production efficiency while simultaneously reducing environmental impacts (Işık et al., 2017; Evett et al., 2020; Wu et al., 2022). As global populations rise and the demand for food escalates, the agricultural landscape must adapt—this is where precision agriculture comes into play, utilizing advanced technologies to empower farmers and optimize operations (Afzaal et al., 2020; Wanyama et al., 2024). At its core, precision agriculture focuses on using data-driven approaches to inform agricultural practices. By harnessing technologies like the Internet of Things (IoT), artificial intelligence (AI), big data analytics, and cloud computing (Rodríguez et al., 2017; Abioye et al., 2020; Mansoor and Chung, 2024; Mansoor et al., 2024b; Sheikh et al., 2024), farmers can make informed decisions that lead to better resource utilization and improved crop yields. The benefits of precision agriculture extend beyond simple yield enhancements; they also encompass significant reductions in resource wastage, particularly water and fertilizers. This not only conserves water but also ensures that crops receive the precise amounts of water they need for optimal growth (Uztürk and Büyüközkan, 2024).
Agriculture 1.0 was defined using basic tools, manual and animal labor, and a heavy dependence on natural factors such as sunlight and rainfall. Farmers depended on their understanding of the land, weather patterns, and traditional farming techniques passed down through generations, typically from fathers to sons. This period was characterized by subsistence farming, where families grew just enough food to meet their own needs. The Industrial Revolution marked a significant transformation in agriculture, leading to what is known as the “Green Revolution” due to substantial increases in crop yield and productivity (Gagliardi et al., 2022; Liu et al., 2021; Zhai et al., 2020; Aggarwal and Verma, 2022). Agriculture 2.0 introduced machinery like tractors and harvesters, replacing manual labor and enhancing efficiency. This period also saw the rise of chemical fertilizers and pesticides, which boosted crop yields but negatively impacted the environment. Many innovations stemmed from the re-adaptation of mechanical and chemical industries that had previously catered to military needs during World War II. Additionally, breeding programs expanded through public universities, research institutes, and private companies (Aggarwal and Verma, 2022; Gagliardi et al., 2022).
Agriculture 3.0, often referred to as precision agriculture, utilized technology to enhance farming methods. It integrated GPS technology, remote sensing, and Geographic Information Systems (GIS) to gather data on soil conditions, crop health, and weather patterns. This information was used to create detailed maps, allowing for the targeted application of inputs like fertilizers, pesticides, and irrigation, which reduced waste and lessened environmental harm. During this period, public awareness grew regarding the environmental consequences of excessive fertilizer and chemical usage (Aggarwal and Verma, 2022; Gagliardi et al., 2022; Casavola and Gagliardi, 2012; Nargotra and Khurjekar, 2020).
The evolution of precision agriculture is often framed within the contexts of Agriculture 4.0 and Agriculture 5.0. Agriculture 4.0, described as the “Digital Revolution in Agriculture,” focuses heavily on the incorporation of sophisticated technologies to facilitate efficient agricultural practices (Maffezzoli et al., 2024). Agriculture 4.0 significantly enhances precision agriculture through a variety of technological advancements that improve efficiency, accuracy, and sustainability in farming practices. By utilizing IoT sensors, farmers can collect real-time data on conditions such as soil moisture, temperature, and crop health, allowing for informed decision-making (Zhai et al., 2020; Javaid et al., 2022). Additionally, big data analytics helps identify trends and make predictive assessments that optimize resource allocation. Drones equipped with multispectral cameras provide high-resolution aerial imagery, enabling remote monitoring of large fields and identifying areas that require attention. AI and machine learning (ML) further aid precision agriculture by analyzing data to predict outcomes and automate decision-making processes related to irrigation, fertilization, and pest control (Latino et al., 2022). Variable rate technology (VRT) allows for tailored applications of inputs like fertilizers and pesticides based on specific field characteristics, reducing waste and environmental impact. GPS technology enhances precision mapping and guides autonomous machinery, ensuring accurate operation in planting, harvesting, and resource application (Latino et al., 2022; Tenreiro et al., 2023). Moreover, Agriculture 4.0 fosters collaboration and connectivity among farmers through shared data, promoting integrated farming systems that improve overall management. By optimizing resource use and reducing carbon footprints, these advancements contribute to sustainable agricultural practices, demonstrating how Agriculture 4.0 is revolutionizing the efficiency and effectiveness of farming (Zambon et al., 2019; Zhai et al., 2020; Javaid et al., 2022).
Transitioning into Agriculture 5.0, we observe a paradigm shift towards a more human-centric approach in agricultural innovation. While Agriculture 4.0 emphasizes data and automation, Agriculture 5.0 combines technology with human ingenuity and sustainable practices (Islam et al., 2024). It accommodates the use of advanced IoTs, robotics, AI, and collaborative efforts between humans and machines, promoting resilience within agricultural systems (Hurst and Spiegal, 2023; Kazakis and Tsirliganis, 2023). Agriculture 5.0 seeks to foster a deeper collaboration among human expertise, machine efficiency, and sustainable methodologies, creating a synergistic effect where both humans and machines can contribute to overcoming agricultural challenges (da Silveira and Amaral, 2022; Ross and Maynard, 2021). For example, autonomous agricultural equipment equipped with AI can work alongside farmers, enhancing their capabilities in tasks such as planting, harvesting, and pest control, while ensuring that these processes are executed with minimal environmental impact. Moreover, this evolution also accentuates the importance of sustainable practices in agriculture (Pham et al., 2013; de la Parte et al., 2024; Popescu et al., 2024). As awareness of environmental concerns grows, there is an increasing emphasis on practices that not only increase yield but also maintain ecological balance. By incorporating sustainable techniques within the precision agriculture framework, farmers can reduce their carbon footprint, enhance biodiversity, and maintain healthier soils (Fraser and Campbell, 2019; Juwono et al., 2023; Ku et al., 2023; Mansoor et al., 2024a). Precision agriculture is transforming agriculture by integrating advanced technologies to meet food production demands. This shift from a data-driven model to a collaborative approach, incorporating multi-omics data analysis, emphasizes the synergy between technology and human expertise, fostering resilient agricultural systems (Abioye et al., 2023). As stakeholders adopt these innovations, including the integration of multi-omics insights, the agricultural sector is increasingly poised to ensure food security and sustainability for the growing global population.
As environmental concerns grow, there is an increasing emphasis on agricultural practices that enhance yield while maintaining ecological balance. By integrating sustainable techniques within the precision agriculture framework, farmers can effectively reduce their carbon footprint, bolster biodiversity, and promote healthier soils (Konfo et al., 2024). Precision agriculture optimizes resource use and minimizes waste, leading to significant environmental benefits. For instance, reductions in carbon emissions result from decreased fertilizer and pesticide application, which not only lowers greenhouse gas emissions but also enhances soil health, measurable through a soil health index that reflects improved organic matter and nutrient availability (Ahmad and Dar, 2020; Farooqui et al., 2024). Moreover, precision irrigation enhances water use efficiency, conserving vital resources and protecting local ecosystems. Collectively, these metrics illustrate how precision agriculture fosters sustainable farming methods and enhances overall environmental stewardship.
IoT is a global network that enables devices to operate, identify, and monitor objects across the globe via the internet, connecting virtual and physical entities through integrated information and communication technologies (Pham et al., 2013; Abioye et al., 2023) The main objective of smart farming is to enhance real-time information sharing across autonomous networks using smart sensors and internet connectivity. Various communication solutions, such as wireless sensors and Radio-Frequency Identification (RFID) technologies, support interconnectivity among networks and devices (Juwono et al., 2023; Ku et al., 2023). In smart farming, critical parameters are monitored to improve yield, optimize environmental conditions, manage irrigation, control pests and fertilizers, oversee soil health, and enhance greenhouse production, all while reducing operational costs (Evans, 2011; Gómez Romero et al., 2016; Nukala et al., 2016; Fraser and Campbell, 2019; Mansoor et al., 2024a). These technologies play a vital role within IoT platforms and are classified into data acquisition, investigation, and evaluation categories (Zecha et al., 2013; Freeman and Freeland, 2015; Balafoutis et al., 2017). Countries such as those in Europe, Australia, and the USA have embraced smart farming, alongside individual nations like Italy (Borgogno Mondino and Gajetti, 2017), Brazil (Pivoto et al., 2018), Ireland (Das et al., 2019), and India (Mogili and Deepak, 2018).
We analyzed the existing literature on the application of IoT platforms and wireless communication technologies across various agricultural activities. At the outset of this review, relevant articles were sourced from the Web of Science using keywords such as “smart agriculture,” Agriculture 4.0, IoT, smart farming, digital agriculture. This collection included 6000 recent articles comprehensive bibliometric information, which was subsequently used as input for VOS viewer (Software) analysis. This analysis assesses the frequency of keyword usage and citation metrics in the selected articles. Additionally, it visualizes the co-occurrence of keywords and the co-citation of references (Figure 1).
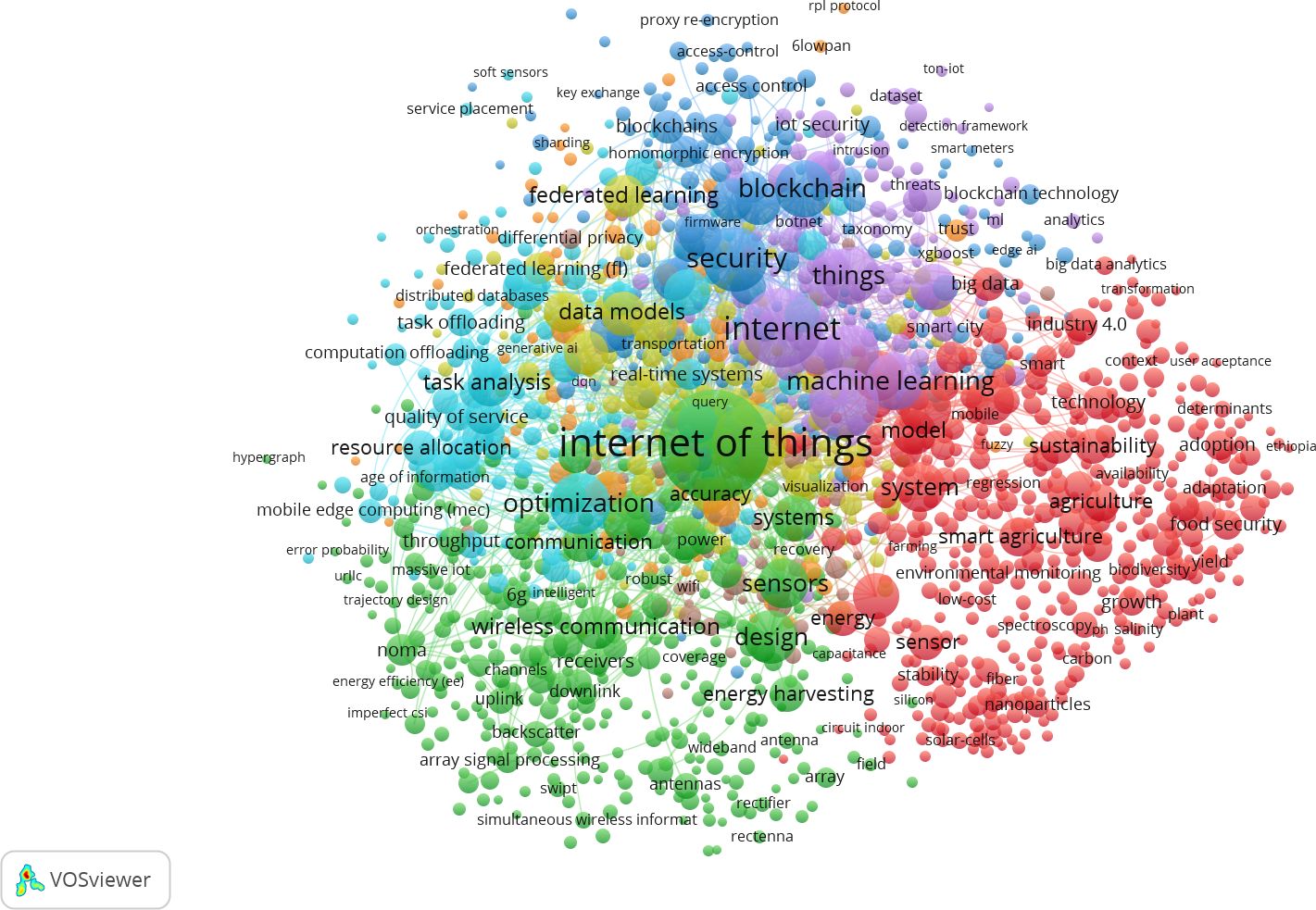
Figure 1. VOS viewer keyword co-occurrence network visualizes associations and clusters related to the “Internet of Things” (IoT). It features various color-coded clusters representing interconnected concepts. The central term, “Internet of Things,” connects to multiple branches, highlighting its relevance. Key clusters include the green cluster with “optimization” and “analytics,” focusing on data processing; the blue cluster with “security” and “blockchain,” emphasizing secure data transactions; the yellow cluster with “machine learning,” showcasing AI advancements; and the red cluster with “energy” and “sustainability,” addressing environmental concerns. Lines between keywords illustrate co-occurrence, helping identify trending topics and common research areas in IoT. This image was created with VOSviewer.
To create the visual map, specific criteria were established, with a minimum threshold of five co-occurrences for keywords. Out of a total of 20767 keywords, only 1440 met this threshold criterion. The keyword with greatest total link strength is selected. The size of each node represents the frequency of co-occurrences of terms, while the thickness of the connecting lines indicates how often these keywords appear together (Figure 1). The connections illustrate the relationships between items, and each node reflects the strength of a particular item. Similarly, co-citation analysis evaluates how frequently an article has been cited across the selected documents. The visualization of the co-occurrence network of citations is depicted in Figure 2.
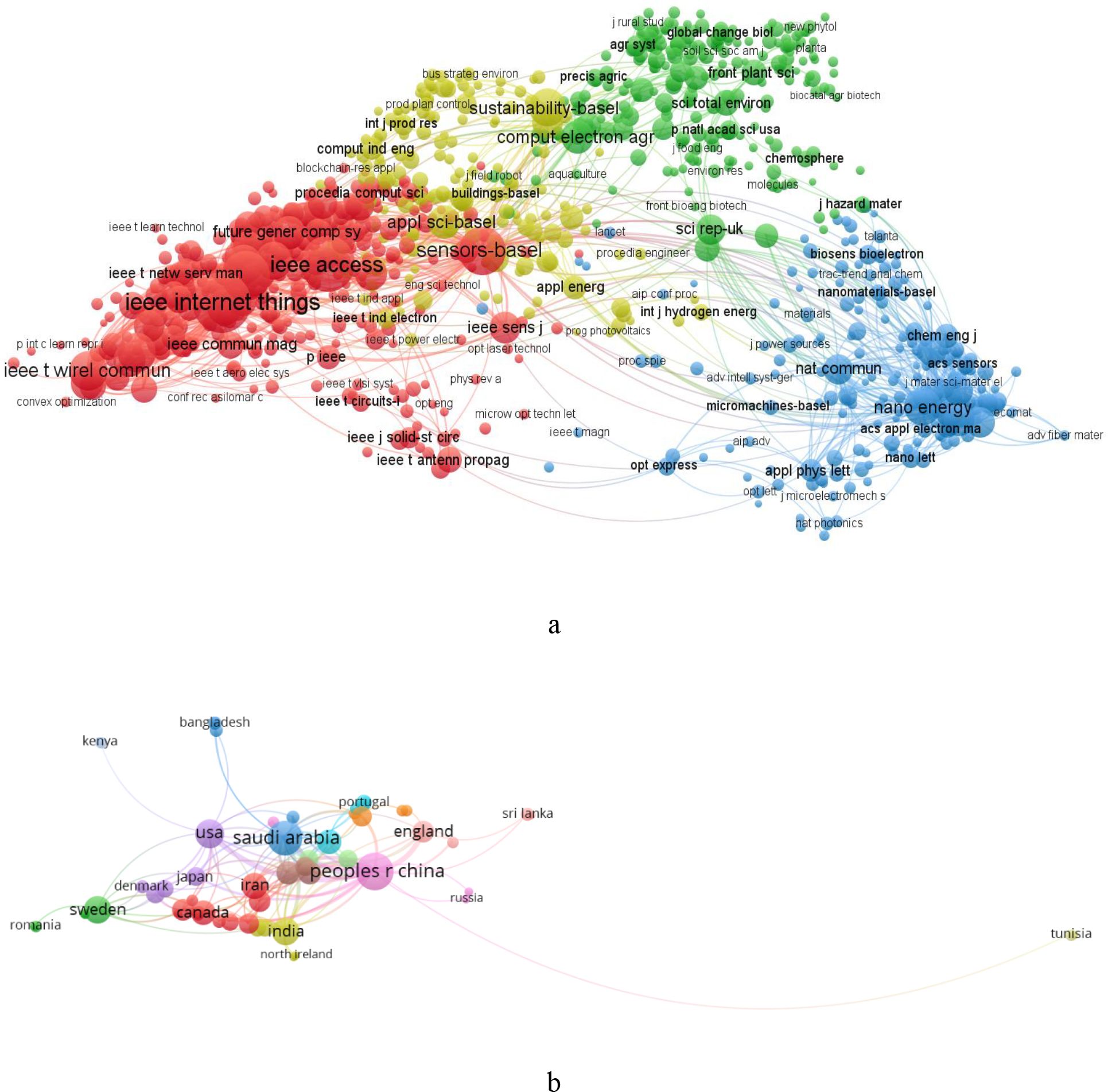
Figure 2. Network visualization of keyword usage and co-citation metrics in the journals. (a) Network visualization of co-citation of references across different journals, highlighting the interconnectedness of cited works. (b) Network visualization map illustrating international research in precision agriculture, showcasing the global distribution and collaboration in this field.
2 Smart sensor trends
The modern agricultural industry has significant challenges that need the use of innovative technologies. Agriculture, a vital economic sector (Gollin, 2010) is being impacted by inflation, rising labor costs (Ray et al., 2023), and climate change (Outhwaite et al., 2022; Verma et al., 2022), resulting in diminished agricultural yields. In this scenario, Precision Agriculture emerges as a crucial solution. This sophisticated agricultural technique employs state-of-the-art technology to enhance the amount and timing of inputs necessary for cultivation, aiming to increase output and efficiency.
Sensors play a crucial role in agriculture, detecting environmental changes and transmitting information to processors (Ullo and Sinha, 2021; Liu et al., 2022). In precision agriculture, smart sensors integrate onboard computing capabilities, allowing them to process and analyze data independently (Rahman et al., 2023; Rajak et al., 2023; Saqib et al., 2024). These sensors are equipped with microprocessors that enable local data processing, autonomous decision-making, and direct communication with other devices via Wi-Fi, Bluetooth, or cellular networks (Yin et al., 2021). This autonomy is particularly useful in large-scale farming, where real-time data on soil conditions, plant health, and climate can significantly influence management decisions. Precision agriculture utilizes various sensor technologies to improve the efficiency and productivity of agricultural practices. Soil sensors deliver critical data on moisture levels, pH, temperature, nutrient content, and electrical conductivity, guiding decisions related to irrigation, fertilization, and planting (Roper et al., 2021). Plant health monitoring employs sensors like leaf sensors, chlorophyll fluorescence sensors, Normalized difference vegetation index (NDVI) sensors, and hyperspectral sensors (Li et al., 2021), while environmental sensors monitor atmospheric conditions that impact agricultural decisions (Rahman et al., 2024; Yin et al., 2021; Han, 2024). Specialized sensors, like yield monitors and water potential sensors, offer precise data on crop yield and soil water availability, enhancing farming operations (Table 1).
The cost of sensors is a crucial factor because it directly affects farmers’ ability to invest in these technologies within their budget constraints. The cost-effectiveness of agriculture sensors depends on their complexity and function (Saqib et al., 2020). Soil moisture sensors range from $50 to $300, while weather stations can cost $100 to several thousand dollars. Nutrient sensors typically range from $500 to $2000. Pest and disease sensors, part of advanced systems, also cost a few hundred to several thousand dollars. Agricultural drones vary from $1,000 to $25,000 or more, depending on features. Basic moisture sensors are inexpensive and provide essential data for irrigation management, while advanced sensors like electrical conductivity and nutrient sensors require higher initial investments but enable precise fertilization and soil health monitoring (Panagopoulos et al., 2014; Kiropoulos et al., 2021; Guerrero et al., 2021). Specialized sensors, like yield monitors and water potential sensors, help farmers assess crop performance and optimize water use, leading to improved crop quality and higher revenues. The return on investment of precision agriculture sensors depends on the scale of the farming operation, the type of sensor, and the specific needs of the farm. Low-cost sensors offer immediate benefits, while high-end technologies provide long-term savings through resource efficiency and yield optimization. Farmers should make informed decisions about which sensors align with their financial capabilities and operational goals.
2.1 Soil sensors
A soil sensor is a device used to measure various physical and chemical properties of soil, such as moisture content, temperature, pH level, electrical conductivity, and nutrient concentration (Yin et al., 2021). Traditional assessment methods, such as soil sampling and laboratory analyses, often lack the necessary spatial and temporal resolution. Therefore, there is an increasing demand for innovative technologies capable of providing precise soil data to enhance smart or precision agriculture systems (Zhang et al., 2024). Recent developments in soil sensors for precision agriculture focus on essential factors for monitoring plant growth cycles. Key elements affecting crop productivity include soil moisture, temperature, pH, nutrient levels, pests, and pollutants. Site-specific management practices, like irrigation and fertilizer usage, rely on data collected from various soil sensors (Kalita et al., 2017; de Jong et al., 2020). A review of six types of soil sensors highlights their technologies, designs, performance, advantages, and disadvantages as well as also discusses research trends and challenges in soil sensors and smart agriculture to guide future studies.
2.2.1 Soil moisture sensors
Soil moisture is vital for assessing soil health and is key to plant growth. It affects the soil’s physical and chemical properties, which in turn impacts salt dissolution, the uptake of water and nutrients by plants, and the activity of microorganisms in the soil (Zhang et al., 2022). Keeping track of soil moisture levels is essential to ensure the right conditions for agricultural production. Soil moisture sensors help farmers measure the water content in the soil, allowing them to determine the optimal timing and amount of irrigation needed for healthy plant growth. Soil moisture measurement is crucial for various applications, including agriculture and hydrological studies. Techniques have been developed to measure soil moisture based on accuracy, cost, and complexity (Zhang et al., 2017, 2024).
Soil moisture sensors are categorized based on the technology they use to detect the moisture levels in the soil, and each type serves specific purposes. Volumetric sensors, particularly capacitive soil dielectric permittivity sensors, are common and appropriate for low-cost, wireless applications. Capacitive sensors measure the capacitance between two plates, which changes with the soil’s dielectric constant, influenced by its moisture content. These sensors are preferred for their low power requirements and minimal interference from soil salinity. Anindita Kalita’s study on polymethyl methacrylate (PMMA) coated capacitive sensors for soil moisture sensing suggests they could be a cost-effective and easy-to-fabricate solution for real-time soil moisture monitoring in agriculture. Despite initial sensitivity limitations, they could optimize irrigation practices and crop productivity, highlighting the need for further research (de la Parte et al., 2024).
Resistive sensors measure the electrical resistance between electrodes inserted into the soil, but their accuracy can be compromised by soil composition variations. Steven M. de Jong’s study on Electrical Resistivity Tomography (ERT) showed it can effectively monitor soil moisture dynamics under controlled field conditions, but challenges remain due to environmental variables and ERT’s limitations (de Jong et al., 2020).
Time Domain Reflectometry (TDR) and Time Domain Transmissometer (TDT) sensors use electromagnetic waves to measure moisture, however they are costly and complicated, suitable for research and precision agricultural activities. Time-Domain Reflectometry (TDR) is known for its precision and is widely used in scientific research. Zhongdian and his research group developed a TDR-based method for measuring soil erosion and soil moisture content, demonstrating high accuracy and automation potential. Further refinement and testing are suggested for wider applicability (Zhang et al., 2022) Time Domain Transmissometer (TDT) measures the transmission time of electromagnetic waves through the soil, providing excellent accuracy and usefulness for depth-specific moisture profiling. Raphaël Pederiva and colleagues developed an on-chip terahertz (THz) characterization technique for low-volume or thin-film materials. The method uses time-domain transmissometer to determine the complex refractive index of materials over a frequency range of hundreds of gigahertz (GHz). The device uses ultrafast photoconductive switches driven by a femtosecond laser, allowing for high precision and minimal sample volume (Krzeminska et al., 2022).
Frequency Domain Reflectometry (FDR) uses the frequency change of an electromagnetic wave to determine soil moisture, making it effective for continuous monitoring across various soil conditions. The study in the Gryteland catchment in Norway used frequency domain reflectometry (FDR) and electrical resistivity tomography (ERT) to monitor soil moisture and temperature patterns. Key findings showed different patterns on north-facing and south-facing slopes, impacting freezing and thawing cycles. The study suggests that local terrain features, particularly slope aspects, are crucial in hydrological processes and should be considered in environmental and agricultural management strategies (Ma et al., 2022).
Optical methods, such as visible and near-infrared spectrophotometry, leverage the soil’s light absorption and scattering properties, which change with moisture content. These methods are advantageous for non-contact measurements and are particularly useful in remote sensing applications (Abdulraheem et al., 2023). The choice of a suitable technique depends on the specific requirements of the application, including accuracy, cost considerations, and environmental conditions. The use of Sentinel-2 imagery and the optical trapezoid model (OPTRAM) to monitor soil moisture variability in agricultural production stages. The method, which uses Sentinel-2 imagery, is used to explore high-resolution spatial heterogeneity of soil moisture and monitor various stages of agricultural production (Hassanpour et al., 2020; Stańczyk et al., 2023). The results show that the OPTRAM model can produce accurate soil moisture estimates, improving irrigation management and crop growth understanding, ultimately leading to better water resource management in agriculture (Crioni et al., 2025).
Precision irrigation uses soil moisture sensors to monitor real-time water levels and optimize crop decisions. Sensors are deployed at multiple depths to capture moisture variations across the root zone (Bwambale et al., 2022). IoT-based systems analyze this data, combining weather forecasts and crop models to determine precise irrigation schedules. Automated systems adjust water application based on the data, ensuring efficient water distribution and preventing over- or under-irrigation (Kanimozhi and Vadivel, 2024). This dynamic approach refines irrigation strategies, conserving water, enhancing plant health, and improving agricultural yields. For example, in Zhang et al. (2017), an IoT-based soil monitoring system was implemented in a citrus orchard, where real-time soil moisture data helped optimize fertilization and irrigation strategies, leading to reduced water waste, improved efficiency, and enhanced crop productivity (Zhang et al., 2017).
2.2.2 pH sensors
Soil pH is crucial for plant growth and fertilizer application efficiency. Real-time soil pH sensors, integrated with precision agriculture technologies, provide real-time feedback on soil conditions. These sensors communicate with cloud-based systems, enabling automated pH adjustments (Table 1) (Lavanaya and Parameswari, 2018; Yin et al., 2021; Fauziah et al., 2024).
In addition to improving nutrient absorption, real-time pH monitoring enhances soil microbial activity, which is vital for organic matter decomposition and natural nitrogen fixation (Xu et al., 2024). Studies have shown that microbial communities thrive best within a neutral to slightly acidic pH range (5.5–7.5), where beneficial bacteria such as nitrogen-fixing Rhizobia and phosphate-solubilizing Pseudomonas species actively contribute to soil fertility (Saeed et al., 2021). Savich investigated the application of soil amendments combined with pH-responsive sensors in saline soils, demonstrating that integrating real-time monitoring with phosphogypsum and organic fertilizers significantly enhanced CO2 assimilation and crop biomass (Savich et al., 2021). The study emphasized the role of real-time pH adjustments in improving photosynthetic activity, ultimately leading to higher productivity in challenging soil conditions.
Furthermore, Zhao analyzed the long-term impact of no-till (NT) agriculture on soil pH stability, revealing that real-time pH monitoring could help mitigate soil acidification by optimizing nitrogen application rates and periodic liming schedules. By integrating pH sensors into conservation tillage practices, farmers can maintain soil health while minimizing the negative effects of prolonged nitrogen fertilization (Zhao et al., 2022).
In addition to chemical amendments, biological strategies for pH optimization are gaining traction. Yaghoubi Khanghahi highlighted the potential of plant growth-promoting bacteria (PGPB) in modifying soil pH and improving nutrient availability. Their research demonstrated that combining bio-inoculants with real-time pH sensors allowed farmers to adjust pH levels in response to microbial activity, reducing dependence on synthetic fertilizers. The findings suggest that a holistic approach integrating biological, chemical, and technological solutions can maximize soil fertility and improve overall crop resilience (Yaghoubi Khanghahi et al., 2021). A typical conductometric pH sensor consists of conductivity electrodes and a thin layer of pH-responsive sensing material.
The study presents a 3D macroporous graphene-functionalized soil pH microsensor, fabricated on Si/SiO2 substrates with Au-interdigitated electrodes. The sensor increases conductance with pH increase, exhibiting a sensitivity of 97 μS/pH and a response of 650%. It detects soil pH variations in different soil samples, with sensitivity varying with gravimetric moisture contents (Penn and Camberato, 2019; Siddiqui and Aslam, 2023). A potentiometric soil pH sensor measures soil pH by detecting voltage difference between reference and pH-sensitive electrodes. Accurate pH readings are crucial for agriculture and research, requiring regular calibration and calibration with known solutions (Siddiqui and Aslam, 2023). Matthew McCole, presents a potentiometric measurement system for on-site soil pH and potassium levels detection (McCole et al., 2023). The system uses 3D printed ion-selective electrodes, a PSoC4 microcontroller, and a reference electrode for ion activity. The system is portable, user-friendly, and efficient, enabling real-time soil analysis and precise management of soil nutrients, potentially leading to better crop yields and reduced environmental impact (Childs et al., 2000). Ion-selective pH sensors (ISE) measure hydrogen ions in solutions or soil, providing accurate real-time data through a glass electrode and reference electrode, requiring regular calibration. An alternative method is the ion-selective field-effect transistor (ISFET), which includes a drain, source, and gate electrode. pH-sensitive materials like silicon oxide, silicon nitride, and aluminum oxide are coated on the gate electrode. When in contact with the solution, these materials induce changes in gate voltage, affecting the current between the source and drain electrodes based on pH variations. Due to the complexity of soil, ISFETs must be well-protected to avoid damage during insertion (Shylendra et al., 2025).
2.2.3 Temperature sensors
Soil temperature, which varies between -10 and 50°C, is a significant determinant in agriculture, affecting germination, flowering, decomposition, and multiple phases of plant development (Bollero et al., 1996; Onwuka and Mang, 2018). It profoundly affects the physical, chemical, and microbiological processes in soil that are critical for plant growth. Soil temperature is influenced by factors such as specific heat capacity, thermal conductivity, bulk density, texture, water content, and surface coverings (Passioura, 2002; Hatfield and Prueger, 2015).
A soil temperature sensor operates by converting temperature fluctuations into an electrical signal, which is then processed into digital data. Various types of electronic temperature sensors suitable for this application include thermocouples, resistance temperature detectors (RTDs), thermistors, and semiconductor-based sensors (Davaji et al., 2017). Thermocouples function by generating a voltage due to the temperature difference at the junction of two dissimilar metals, typically iron and constantan. They are recognized for their rapid response and automation capabilities, making them appropriate for monitoring soil temperature. Specially calibrated cables are essential for long-distance measurements. Resistance temperature detectors (RTDs) are composed of a conductive metal wire coiled around a non-conductive core, offering high accuracy and stability. They display increased delicacy relative to thermocouples and show a reduced response time to temperature changes due to their protective housing (Kool et al., 2016).
Thermistors, made from ceramic or polymer materials, demonstrate a change in resistance when subjected to temperature fluctuations. They provide high resolution due to significant thermal coefficients; however, they require complex calibration because of their non-linear response. The resolution of this issue can be achieved (Xu et al., 2023). Kool and colleagues’ study highlights the importance of accurately measuring soil temperature gradients to determine soil heat and latent heat fluxes. They used thermistors to monitor soil temperature, but found discrepancies of 0.2°C under uniform conditions. To improve accuracy, they developed an in-situ calibration technique that minimized uncertainty to 0.05°C. This allowed for more precise measurements in a vineyard under arid conditions and showed stable thermistor offsets over a five-year period (Kool et al., 2016).
2.2.4 Nutrient sensors
Nutrient sensors for soil are advanced tools critical for precision agriculture, designed to identify and measure essential soil nutrients, including nitrogen, phosphorus, and potassium. These sensors operate on various principles, including ion-selective electrodes, optical sensors, electrochemical sensors, and spectroscopy, each tailored for specific nutrient types (Burton et al., 2020a). They enable real-time monitoring and mapping of soil nutrient levels, thus facilitating precise and efficient fertilizer application. This approach improves crop yields and optimizes fertilizer use, reducing costs and minimizing environmental impacts by preventing nutrient runoff, thus preserving soil health and protecting water quality. Nutrient sensors represent a significant advancement in agricultural technology, enhancing sustainable farming practices by optimizing plant growth and resource management (Horváth et al., 2024).
Various nutrient sensors have been developed for agricultural applications, each demonstrating unique capabilities and stages of development (Burton et al., 2020b). The Visible-Near Infrared (Vis-NIR) sensor is currently in use in both laboratory and field settings, effectively measuring soil pH and nutrient levels. Similarly, the Visible-Mid Infrared (Vis-MIR) sensor has shown promise in laboratory settings for assessing soil mineral nitrogen content (Ehsani et al., 1999, 2001). The Attenuated Total Reflectance (ATR) spectroscopy sensor operates in both laboratory and field environments, focusing on soil nutrient analysis (Christy et al., 2003).
Raman spectroscopy, used in laboratory and field applications, is effective for evaluating various soil nutrients (Jahn et al., 2006). Additionally, Ion Selective Electrodes (ISE) and Ion-Selective Field Effect Transistors (ISFET) are utilized in laboratory and field settings for measuring soil pH and nutrients (Sudduth et al., 1997; Adamchuk et al., 2005; Aravamudhan and Bhansali, 2008; van Staden et al., 2018). Each sensor type contributes vital insights into soil health and nutrient management, enhancing precision agriculture practices (Burton et al., 2020b).
2.2.5 Electrical conductivity sensors
Soil electrical conductivity (EC) is a crucial indicator in agriculture, as high soil salinity can negatively impact crop growth and reduce agricultural productivity. Soil EC is directly related to the types and concentrations of ions in soil moisture, such as sodium, chloride, calcium, and magnesium, which enhance the soil’s electrical conductivity. Higher moisture levels generally increase soil EC because they facilitate the movement of soluble salts (Liu et al., 2024). The physical composition of the soil, including its clay, sand, and organic matter content, can also influence EC readings. Clay soils typically have higher EC values due to their finer texture and greater cation exchange capacity.
Techniques for measuring soil EC include laboratory measurements, in-situ sensors, and remote sensing techniques. High levels of soil salinity can lead to osmotic stress, which can cause dehydration and stunted growth, and ion toxicity, which can accumulate in plant tissues to toxic levels. To mitigate these effects, various soil amendments and management practices may be employed, such as leaching, which involves applying ample irrigation water to flush out excess salts from the root zone, soil amendments, and crop selection and rotation (Wang et al., 2025).
2.2.6 Soil pollutant sensors
Soil pollutant sensors are essential tools in modern agriculture and environmental management, designed to detect and measure harmful substances in the soil, such as heavy metals, pesticides, herbicides, and industrial pollutants. Excessive application of agrochemicals, industrial activities, and household waste contribute significantly to soil health degradation, crop safety concerns, and environmental quality deterioration. Advanced technologies, including electrochemical detection, optical sensing, and biosensing, enable precise and real-time monitoring of soil pollutants (Garlando et al., 2020). Electrochemical sensors quantify changes in soil conductivity due to specific contaminants, while optical sensors utilize light interactions to identify pollutants, such as organic chemicals. Biosensors employ biological components, including enzymes or microbes, for the precise detection of toxic substances. These sensors are employed in multiple applications, such as monitoring soil health in agriculture, optimizing fertilizer use, ensuring environmental compliance, and aiding soil remediation efforts. Soil pollutant sensors enable the early identification of contaminants, which mitigates risks to human health, protects ecosystems, and promotes sustainable agricultural practices. Their role is essential in addressing soil contamination problems (Garnaik and Nayak, 2024).
2.3 Insect/pest sensors
Plant diseases and pests can significantly compromise the quality and yield of agricultural products by inflicting damage on plant roots, bulbs, and aerial parts through their feeding behaviors. Common soil-dwelling pests that contribute to agricultural loss include various species such as beetles, moths, butterflies, and flies. To facilitate the detection of these soil pests, a range of advanced methodologies has been developed. Optoelectronic sensors harness light-based technologies to identify changes in environmental conditions attributable to pest activity. Acoustic sensors capture the sounds generated by pests while they interact with plants or soil, thereby enabling their identification. Impedance sensors assess variations in electrical resistance that may indicate the presence of pests or their feeding behavior (Stańczyk et al., 2023).
Furthermore, nanostructured biosensors provide a highly sensitive detection mechanism by utilizing nanomaterials to enhance their capabilities, facilitating the identification of specific pests or biological markers associated with pest-induced damage. These innovative detection methodologies furnish agricultural practitioners with essential tools to monitor and manage pest populations more effectively, ultimately contributing to the protection of crop health and the enhancement of agricultural productivity.
Fazeel Ahmed Khan and his team have developed an IoT-based system for environmental monitoring and disease detection in smart greenhouses. The system monitors the greenhouse’s environment, manages water irrigation, collects images, and predicts plant diseases using leaf datasets. The research validates the proposed system design and architecture for IoT-based monitoring and water irrigation management. The system also enhances greenhouse management and supports agribusinesses and farmers by transitioning traditional greenhouses into smart greenhouses, thereby automating and improving agricultural practices using advanced technologies (Khan et al., 2020).
2.4 Plant stress sensors
Plant stress refers to the negative impact on plant growth and development due to biotic and abiotic factors, such as pests, diseases, drought, salinity, and extreme temperatures (Bashir et al., 2021). Understanding and managing plant stress is crucial for improving crop yield and sustainability, especially in the face of global challenges like climate change and food security issues (Galieni et al., 2021; Mansoor et al., 2022). Plant stress mechanisms involve complex physiological and biochemical processes, triggering a cascade of molecular and cellular responses. Techniques for detecting plant stress include remote sensing, thermal imaging, fluorescence imaging, and spectroscopy and hyperspectral imaging. Types of stress sensors include moisture sensors, nutrient sensors, soil salinity sensors, gas exchange sensors, and chlorophyll fluorescence sensors. Moisture sensors monitor soil and plant water status, while nutrient sensors detect deficiencies or toxicities of nutrients. Soil salinity sensors measure soil salinity, which can adversely affect plant growth due to osmotic stress and nutrient imbalance (Yin et al., 2021). Gas exchange sensors measure photosynthesis and respiration rates, indicating plant health and stress levels. Future directions in plant stress detection include Positron Emission Tomography (PET) and advanced metabolomics, which offer deeper insights into physiological and metabolic changes occurring in plants under stress (Galieni et al., 2021). These technologies provide more detailed data and non-destructive ways to monitor plants, facilitating timely interventions and better management practices to enhance plant health and crop yields (Yin et al., 2021; Galieni et al., 2021).
2.5 Positional and motion sensors
Motion and positional sensors are critical tools in precision agriculture, enhancing the implementation of farming practices. These sensors enable precise navigation and guidance of agricultural machinery, ensuring the accurate execution of tasks such as planting, fertilizing, and harvesting. The integration of GPS and IoT technologies facilitates the creation of comprehensive field maps, the tracking of machinery movement, and the enhancement of automated systems, including tractors and drones (Pandey et al., 2021; Getahun et al., 2024). The application of variable rate technology (VRT) through these sensors allows farmers to apply precise amounts of seeds, fertilizers, or water in designated areas, thus reducing waste and improving efficiency (He, 2023). They enhance sustainability by reducing fuel consumption, limiting chemical overuse, and decreasing environmental impact. Motion and positional sensors are critical for tracking livestock movement and protecting valuable agricultural assets (Tenreiro et al., 2023). The ability to provide real-time feedback and data-driven insights allows farmers to make informed decisions, enhancing productivity, lowering costs, and fostering sustainable agricultural practices. With the advancement of precision agriculture, these sensors play a crucial role in improving accuracy and efficiency in modern farming practices (Nackley et al., 2021; Rajak et al., 2023; Naidu et al., 2024).
3 IoT and sensor integration in precision agriculture
The IoT is revolutionizing agriculture by enabling smarter resource management and enhancing productivity. IoT-based systems use intelligent sensors to monitor field conditions in real-time, transmitting data via wireless networks to cloud platforms for precise irrigation adjustments (Rajak et al., 2023). IoT integration with mobile internet allows farmers to remotely monitor and control agricultural systems using mobile applications. Combining IoT with agricultural robotics advances intelligent farming practices, with robots autonomously performing tasks like seeding, fertilization, and pesticide application (Botta et al., 2022).
IoT platforms also facilitate environmental monitoring and early pest detection, with tools like video surveillance and pest-monitoring lamps enabling remote observation of pest activity. Meteorological data collected by IoT sensors aids in forecasting agricultural disasters. However, challenges like high costs, inconsistent standards, and limited compatibility across platforms hinder widespread IoT adoption in agriculture. Addressing these barriers by developing unified data standards and cost-effective IoT products can enhance agricultural productivity, expand benefits to more farmers, and promote sustainable agricultural development (Xu et al., 2022).
The integration of IoT with diverse sensor technologies allows for continuous, real-time monitoring of various agricultural parameters such as soil moisture, pH levels, temperature, nutrient status, and plant health (Marios and Georgiou, 2017; Khanal et al., 2020). These sensors collect data at high temporal and spatial resolutions, providing a detailed view of the field conditions. The real-time data transmission enabled by IoT technologies ensures immediate availability of information, which is crucial for timely decision-making and intervention (Wang et al., 2011). IoT-enabled sensor networks offer the capability for remote monitoring of agricultural fields, reducing the necessity for physical presence (Srbinovska et al., 2015).
Through web interfaces and mobile applications, farmers can access data collected by sensors from anywhere, enabling them to monitor and manage their fields more effectively. This remote accessibility is particularly beneficial for large-scale operations or farms located in hard-to-reach areas (Hashim et al., 2015). Integrating sensors with IoT in agriculture offers significant benefits, including process automation driven by real-time data. For instance, irrigation systems can adjust automatically based on soil moisture levels, while fertilizer and pesticide applications can be tailored to the specific needs of different crop zones, reducing waste and environmental impact (Liang and Shah, 2023). IoT platforms aggregate data from various sensors, enabling advanced analytics and predictive insights that help farmers anticipate issues like pest outbreaks and plant diseases. Furthermore, ML algorithms optimize resource allocation and crop management by leveraging historical and real-time data (Mowla et al., 2023).
The scalable design of IoT platform allows for the integration of diverse sensors and data sources, ensuring adaptability to changing farm conditions and facilitating a flexible management approach in precision agriculture (Sharma and Shivandu, 2024). The use of IoT technology in precision agriculture is markedly reducing human involvement while improving operability and system stability. IoT-driven smart irrigation systems, autonomous machinery, and real-time soil and crop monitoring facilitate efficient resource utilization and enhanced productivity. Soil moisture sensors and automated irrigation optimize water consumption, while GPS-guided tractors, drones, and robotic seeders execute field operations autonomously (Shantaram et al., 2005; Pratama et al., 2021).
AI-driven predictive maintenance guarantees equipment dependability by identifying problems before to failure (McCole et al., 2023). Furthermore, cloud-based farm management systems provide farmers with remote monitoring and decision-making capabilities, including real-time sensor data, weather predictions, and AI analytics. The use of 5G, LoRaWAN, and edge computing enhances system connectivity and reactivity, allowing fully autonomous, data-driven agriculture. These developments result in reduced labor costs, optimal resource use, and improved sustainability, guaranteeing that the future of precision agriculture is more efficient, resilient, and ecologically sustainable (Liu et al., 2024).
4 Smart sensors and IoT in precision agriculture
Modern technology is important in maintaining agricultural productivity even with limited resources. It helps farmers monitor climate changes, track soil nutrient levels, manage water usage, and streamline data handling in farming operations. Various sensors and computing tools are now available to collect and manage data from cropping systems to make timely and informed decisions (Ali et al., 2023). Various digital platforms and camera-based monitoring systems empower farmers to observe their fields remotely. IoT applications and smart farming techniques enhance decision-making by simulating and forecasting crop yields under anticipated climatic conditions (Akbar et al., 2024). Moreover, advanced neural networks and simulation models have reliable decision support for farming activities (Figure 3). By integrating these technologies, farmers can optimize resource use, minimize waste, and enhance crop health and productivity, contributing to more sustainable and efficient farming systems (Mana et al., 2024).
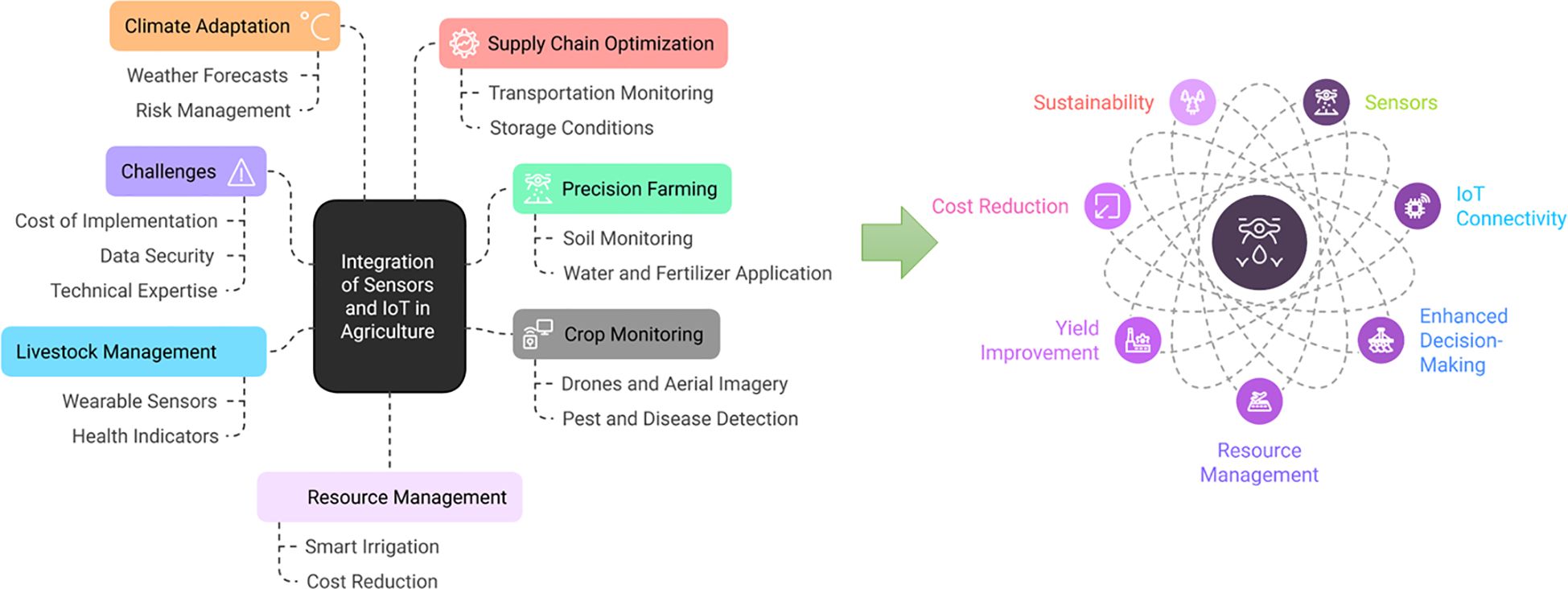
Figure 3. Figure highlights key applications including resource efficacy, data-driven decision-making, livestock monitoring, precision farming, pest and disease management, smart irrigation, greenhouse automation, and supply chain optimization. Sustainability, cost reduction, yield improvement and resource management can enhance productivity, sustainably manage re-sources, and improve agricultural efficiency.
Precision farming uses IoT-enabled sensors to monitor and manage agricultural operations with the highest accuracy. Various sensors embedded in fields collect data on soil moisture, pH, temperature, and nutrient levels. These sensors provide farmers with real-time data for the health and needs of various crops. The biggest advantage of this granular data includes the optimization of irrigation, fertilization, and pest control resulting in increased yields and reduced resource wastage (Sishodia et al., 2020). For example, soil moisture sensors can help farmers determine the optimal watering schedule, preventing over-irrigation and conserving water (Figure 4). Similarly, nutrient sensors analyse soil composition to recommend precise fertilizer applications, reducing costs and minimizing environmental runoff. Precision farming technologies also give variable-rate application of inputs which helps to treat specific areas of a field differently based on their unique conditions. This level of customization improves productivity while promoting environmental sustainability (Sishodia et al., 2020).
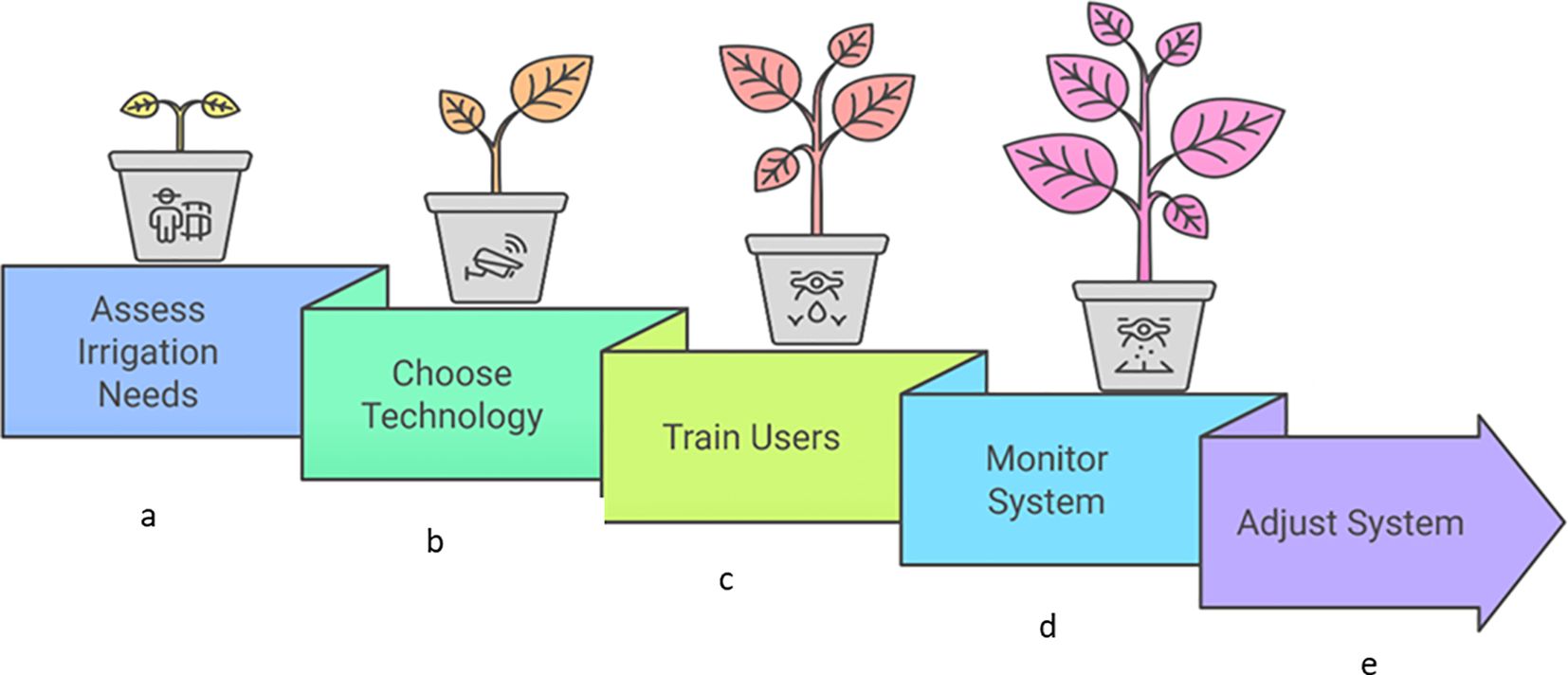
Figure 4. It explains a five-stage process for implementing smart irrigation systems. The figure depicts a sequential workflow for establishing effective smart irrigation. This involves: (a) Evaluating site-specific irrigation requirements. (b) Selecting appropriate sensors and controllers. (c) Providing training on system operation. (d) Continuously checking system performance. (e) Making necessary adjustments for efficiency. Each stage is visually represented by a potted plant progressing in growth, illustrating the impact of effective irrigation management.
IoT and sensors have transformed livestock management by continuous monitoring of animal health, behavior, and the environment. Some wearable sensors on livestock collect data on body temperature, movement, heart rate, and feeding habits. This information is then transmitted to centralized platforms where farmers can identify health issues or various irregularities (Isaac, 2021; Monteiro et al., 2021). For example, sensors can detect signs of illness, such as reduced movement or abnormal temperature. Additionally, sensors monitor reproductive cycles showing timely breeding and increasing reproduction rates. IoT platforms can also track the location and activity of grazing animals, reducing the risk of theft and improving pasture management. This combination of real-time monitoring and predictive analytics helps increase animal welfare, boost productivity, and reduce economic losses (Davaji et al., 2017).
4.1 Smart irrigation systems
Water is one of the most critical resources in agriculture and its efficient use is important in growing scarcity (Mansoor and Chung, 2024). IoT-enabled smart irrigation systems provide a solution by automating water distribution based on real-time data. Sensors placed in fields monitor soil moisture, weather conditions, and crop water requirements. These systems then analyze the data to activate irrigation systems only when needed. Smart irrigation reduces water consumption by preventing overwatering and ensuring even distribution (Figure 4). For example, drip irrigation systems equipped with IoT sensors deliver water directly to plant roots. This minimizes evaporation and runoff (Math et al., 2018).
Water scarcity is a significant challenge for global agriculture, necessitating the adoption of smart irrigation systems that utilize real-time data from soil moisture sensors, weather conditions, and crop water requirements to optimize water use efficiency (Ingrao et al., 2023) Traditional irrigation methods often lead to water wastage, uneven distribution, and reduced crop yields due to inefficient scheduling and overwatering. IoT-enabled smart irrigation systems integrate wireless sensors, cloud computing, and AI to automate water distribution based on real-time environmental conditions, ensuring crops receive the optimal amount of water while minimizing losses due to evaporation and runoff (Koul et al., 2022).
Soil moisture sensors provide continuous feedback on volumetric water content (VWC) and soil matric potential. Weather monitoring systems allow dynamic adjustments to irrigation schedules, while evapotranspiration models predict water loss through transpiration and soil evaporation. The optimization of smart irrigation is achieved through various adaptive techniques that integrate sensor data with AI-based decision models (Veeramanju, 2024).
Real-time irrigation optimization has been shown to yield significant water savings while improving crop productivity. Studies indicate that smart irrigation can reduce agricultural water consumption by 30-50% compared to conventional practices (Mallareddy et al., 2023). AI-based irrigation scheduling improves water-use efficiency (WUE) by up to 60%, ensuring that each unit of water applied contributes maximally to crop growth and yield. Smart irrigation also prevents soil erosion and nutrient leaching, preserving soil fertility and long-term sustainability (Alharbi et al., 2024).
As technology continues to evolve, the future of smart irrigation systems looks promising. Innovations such as AI and ML are expected to enhance the predictive capabilities of these systems, allowing for even more precise irrigation management (Figure 4). Additionally, the increasing focus on sustainable agriculture and water conservation will likely drive the adoption of smart irrigation technologies worldwide (Zhang et al., 2021; Sharma and Shivandu, 2024). Smart irrigation systems are transforming the way we manage water resources in agriculture. By leveraging technology to optimize irrigation practices, these systems not only conserve water but also promote sustainable farming and enhance crop productivity. As we move towards a future where water scarcity is a growing concern, the importance of smart irrigation systems will only continue to rise. The effectiveness of these systems hinges on various sensor types, each with a specific function, and application. As water scarcity, drought, climate changes intensify, the importance of smart sensor systems will continue to grow.
4.2 Pest and disease management
Crop losses due to pests and diseases are a significant challenge for farmers. Various sensors installed in fields can detect environmental conditions that favour pest infestations or disease outbreaks, such as high humidity or temperature fluctuations (Wang et al., 2024). Additionally, advanced imaging sensors can identify early signs of plant stress or damage caused by pests. IoT networks connect these sensors to central platforms which analyze the data and send alerts to farmers. This early warning system leads to targeted interventions, such as applying pesticides or introducing biological control agents (Wang et al., 2024).
Crop health sensors and smart pest traps provide real-time data on plant stress and pest populations, allowing for early detection of pests and diseases. This data is analyzed using advanced algorithms and farm management software, enabling predictive analytics and threshold alerts for timely intervention. By targeting specific problem areas, farmers can implement focused control measures, thereby minimizing the use of chemical pesticides. This approach supports Integrated Pest Management (IPM) and enhances sustainable farming practices, ultimately promoting healthier crops and reducing environmental impact. IPM emphasizes the use of monitoring and assessment to identify pest species and their life cycles, enabling targeted and timely interventions. IPM utilizes a mix of biological control, cultural practices, habitat manipulation, and, when necessary, chemical methods in a way that minimizes risks to human health, beneficial organisms, and the environment. By fostering ecological balance and promoting natural pest resistance, IPM seeks to reduce reliance on chemical pesticides and improve the long-term health of ecosystems. This approach is particularly relevant in agriculture but is also applicable in urban settings and natural resource management, making it a valuable framework for sustainable pest management (Khan et al., 2020; Chin et al., 2023).
4.3 Supply chain optimization
The benefits of IoT in agriculture extend beyond the farm, revolutionizing the agricultural supply chain. Smart sensors track the journey of produce from the field to the market. This ensures quality control and traceability. For example, temperature and humidity sensors in storage and transport facilities monitor conditions for perishable goods that reduce spoilage and ensure freshness. IoT platforms also provide real-time updates on the location and status of shipments thereby improving delivery times. This transparency is best for consumer confidence in the safety and authenticity of agricultural products (Shiyale et al., 2020). Supply chain optimization is a critical aspect of modern business strategy, aiming to enhance efficiency and effectiveness from raw material procurement to product delivery to the end customer. The initial step in this process involves comprehensive data collection and analysis, which provides a foundation for understanding current performance. By gathering data from procurement, production, inventory, and distribution, businesses can identify bottlenecks and areas for improvement. Utilizing advanced analytics tools aids in diagnosing issues and setting the stage for continuous improvement. Following data analysis, accurate demand forecasting becomes imperative. Leveraging historical data alongside market trends allows organizations to predict market demand with greater precision, thereby reducing the likelihood of overproduction or shortages. Advanced forecasting methods enable dynamic adjustments, informed by real-time data, ensuring that businesses remain agile and responsive to market changes.
Inventory management plays a crucial role in supply chain optimization by balancing stock levels to avoid both overstock and stockouts. Techniques such as just-in-time (JIT) inventory or safety stock calculations help maintain this balance. Efficient inventory management reduces holding costs and improves cash flow while ensuring product availability. In optimizing supplier relationships, businesses need to focus on collaboration and effective communication. Building strong relationships with suppliers is essential for negotiation and reliability. Developing supplier scorecards can help evaluate performance, fostering a culture of continuous improvement and innovation. These relationships are pivotal in creating a resilient supply chain. Process and workflow improvements are another critical component. Streamlining operations by eliminating waste and redundancies can significantly enhance productivity.
Methodologies such as Lean, Six Sigma, or Total Quality Management (TQM) are valuable in identifying inefficiencies and driving process enhancements, ultimately leading to increased operational efficiency. Technology integration offers transformative potential in supply chain optimization. Implementing supply chain management software and automation tools can increase visibility and coordination across the supply chain. Emerging technologies like the Internet of Things (IoT), Artificial Intelligence (AI), and Blockchain provide opportunities for real-time tracking and secure transactions, further enhancing supply chain efficiency.
Logistics and distribution optimization are vital for minimizing costs and delivery times. By planning optimal transportation routes and utilizing distribution centers strategically, businesses can ensure rapid delivery and reduced logistics costs. This logistical agility is crucial in today’s fast-paced business environment, where customer expectations for quick delivery are high (Figure 5).
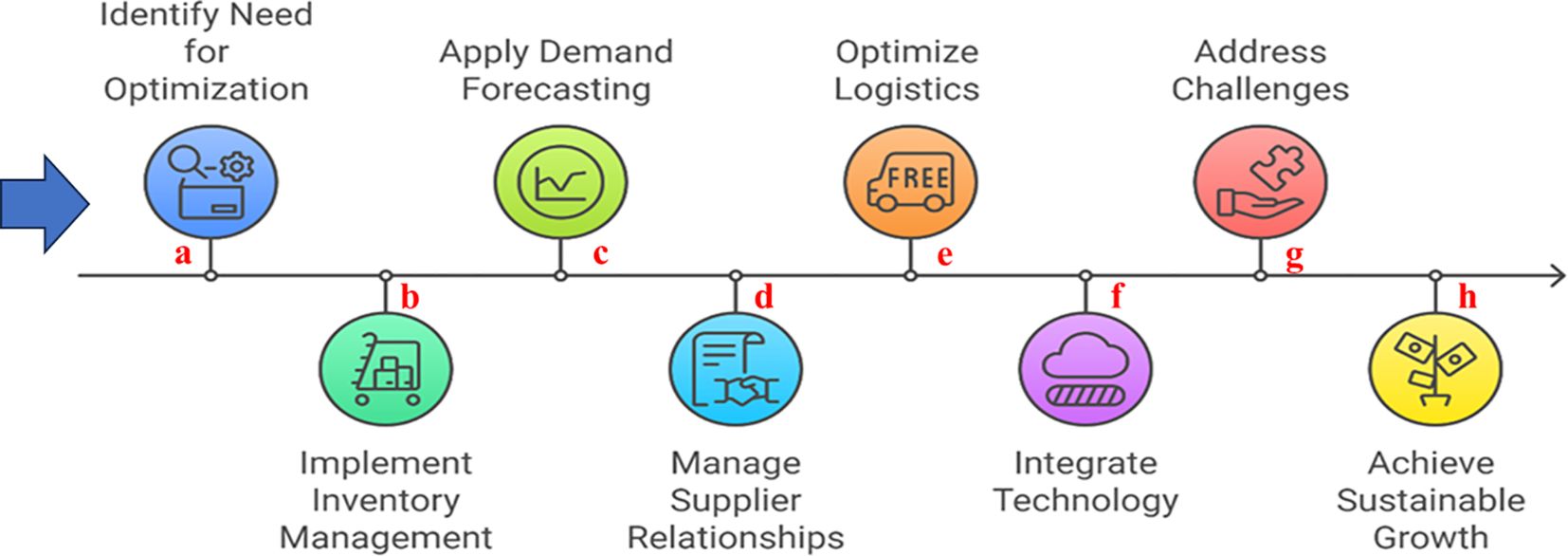
Figure 5. This figure illustrates key strategies for optimizing supply chains, which include (a), Identification of need (b) Inventory Management, (c) Demand Forecasting, (d) Supplier Relationship Management, (e) Logistics Optimization, (f) Technology Integration, (g) addressing challenges and (h) achieve growth by enhance overall supply chain performance. Each strategy plays a critical role in enhancing efficiency, reducing costs, and improving customer satisfaction within the supply chain framework.
Risk management and contingency planning are essential for safeguarding against potential disruptions such as supplier failures or demand fluctuations. Identifying these risks and developing comprehensive contingency plans ensures that businesses can maintain operations even in adverse conditions, thereby increasing supply chain resilience. Finally, performance measurement and continuous improvement are necessary to sustain supply chain optimization. Establishing key performance indicators (KPIs) provides a benchmark for evaluating success. Regularly reviewing and refining strategies based on KPI performance ensures that the supply chain remains efficient and aligned with organizational goals. Engaging in cross-functional collaboration and enhancing communication channels within the organization further supports this ongoing optimization process. Supply chain optimization is essential for businesses aiming to enhance their operational efficiency and customer satisfaction. By implementing effective strategies and leveraging technology, organizations can navigate the complexities of supply chains and achieve sustainable growth. Continuous assessment and adaptation to market changes will further ensure that supply chains remain resilient and competitive.
4.4 Greenhouse automation
IoT-enabled sensors and actuators automate greenhouse operations for the optimal conditions for plant growth. Sensors monitor variables such as temperature, humidity, light intensity, and carbon dioxide levels, while actuators adjust ventilation, heating, and lighting systems accordingly. For example, if sensors detect high temperatures, IoT platforms can automatically activate cooling fans or open vents to regulate the environment (Mishra et al., 2025). This level of automation reduces labour costs, maintains consistent growing conditions, and maximizes yields. Greenhouse automation is an innovative approach in modern agriculture that significantly enhances productivity and sustainability by integrating various technologies. Greenhouse automation offers significant advantages for modern agriculture, leading to enhanced environmental control, increased labor efficiency, data-driven decision-making, optimized resource utilization, and ultimately, higher crop yields (Shiyale et al., 2020; Acharya et al., 2022). This technology leverages several key components, including climate control systems for precise management of temperature, humidity, and ventilation, automated irrigation systems for efficient water distribution, sophisticated lighting systems to optimize light exposure, and automated nutrient delivery systems for precise feeding (Kumar et al., 2022a). Additionally, a network of monitoring sensors collects real-time data on various environmental parameters and plant health.
4.4.1 Climate control systems
i. Temperature Management: These systems employ heaters, coolers, and fans to maintain optimal temperature ranges for plant growth.
ii. Humidity Control: By utilizing dehumidifiers and humidifiers, these systems help maintain appropriate moisture levels, which is vital for plant health.
iii. Ventilation: Automated vents and exhaust systems regulate airflow, preventing overheating and ensuring fresh air circulation.
4.4.2 Automated irrigation systems
i. Drip or Sprinkler Irrigation: These systems allow for precise water delivery directly to plant roots or over crop surfaces, minimizing water waste.
ii. Soil Moisture Sensors: These sensors monitor soil moisture levels, enabling irrigation to be scheduled based on real-time data rather than a fixed schedule.
4.4.3 Sophisticated lighting systems
i. LEDs and Grow Lights: These specialized lights provide optimal wavelengths for photosynthesis and can be controlled to simulate natural light cycles, promoting healthy plant growth.
ii. Light Intensity and Duration Control: Automated systems adjust light intensity and exposure duration depending on plant needs and growth stages.
4.4.4 Nutrient delivery systems
i. Fertigation Systems: These automatically mix fertilizers with irrigation water, ensuring plants receive nutrients in the right proportions at the right times.
ii. Monitoring Nutrient Levels: Sensors can measure nutrient concentrations in the substrate, allowing for adjustments in real-time.
4.4.5 Monitoring sensors and data collection
i. Environmental Sensors: These devices collect data on temperature, humidity, light intensity, CO2 levels, and more, providing a comprehensive view of the greenhouse environment.
ii. Plant Health Monitoring: Sensors can track plant growth metrics and detect stress indicators, allowing for timely interventions.
4.4.6 Implementation strategy
i. Needs Assessment: Conduct a thorough evaluation of the greenhouse’s current capabilities and limitations to determine which automation features are most beneficial.
ii. Technology Selection: Choose technologies and systems that align with the greenhouse’s specific requirements while ensuring compatibility with existing infrastructures.
Successful greenhouse automation hinges on an iterative process of evaluation and adaptation. Regular data analysis allows growers to identify areas for improvement, streamline processes, and incorporate new technologies as they become available (Kumar et al., 2022b). This ongoing commitment to enhancement not only maximizes immediate benefits but also prepares the greenhouse for future challenges and opportunities. By understanding and implementing these components and strategies, growers can fully realize the advantages of greenhouse automation, ensuring more efficient and sustainable agricultural practices (Acharya et al., 2022). Greenhouse automation presents numerous opportunities for innovation and growth in the agricultural sector. By embracing these technologies, farmers can enhance productivity, reduce costs, and contribute to a more sustainable food system. As the industry continues to evolve, those who invest in automation will likely lead the way in meeting the challenges of modern agriculture.
4.5 Decision-making, resource efficiency and sustainability
The vast amounts of data generated by sensors and IoT devices enable advanced analytics and AI applications in agriculture. Farmers can use this to make informed decisions about planting schedules, crop rotation, and resource allocation. Predictive analytics can forecast weather patterns, pest outbreaks, and market trends. AI-driven systems analyze historical and real-time data to recommend optimal farming practices (Qazi et al., 2022). For example, ML algorithms can identify patterns in soil and weather data to suggest the best times for planting and harvesting. Precision agriculture leverages technology and data analysis to improve decision-making, optimize resource efficiency, and enhance sustainability. By using various tools, such as satellite imagery, drones, sensors, and weather stations, farmers can collect vast amounts of data regarding crop health, soil conditions, and environmental factors. This data-driven insight allows for informed decisions that can lead to more effective management practices, reducing uncertainty and risk in agricultural operations (Xu et al., 2022; Hoque and Padhiary, 2024).
One of the key aspects of decision-making in precision agriculture is predictive analytics. Advanced algorithms and machine learning techniques can analyze historical and real-time data to forecast crop yields, pest outbreaks, and potential disease occurrences. This predictive capability enables farmers to take proactive management steps, thereby enhancing crop resilience. Additionally, precision agriculture promotes customized farming practices by employing variable rate technology (VRT), which allow farmers to tailor inputs—such as seeds, fertilizers, and pesticides—to specific areas in their fields based on need rather than applying uniform treatments across the entire area (Bwambale et al., 2022; Kanimozhi and Vadivel, 2024). Resource efficiency is another significant advantage of precision agriculture. By facilitating precise application of fertilizers, pesticides, and water, farmers can optimize input costs while maximizing effectiveness. For example, by using soil moisture sensors and automated irrigation systems, water can be applied only where and when necessary, which is crucial especially in regions facing water scarcity. Moreover, GPS-guided machinery ensures accurate planting, cultivation, and harvesting, thereby reducing fuel consumption and wear on equipment. This attention to resource management not only saves costs but also minimizes environmental impacts, particularly regarding chemical runoff and waste (Pandey et al., 2021; Xu et al., 2022; Naidu et al., 2024). Sustainability is a fundamental principle underlying precision agriculture. The approach encourages practices that enhance soil health, such as cover cropping, reduced tillage, and crop rotation, all while monitoring soil conditions over time (Ullo and Sinha, 2021; Liu et al., 2022). This focus on maintaining soil quality is crucial for long-term agricultural productivity. Moreover, by precisely targeting resource applications, farmers can significantly decrease the use of pesticides and herbicides, helping preserve local ecosystems and promote biodiversity within farming landscapes. This sustainable approach can effectively lower the carbon footprint of agricultural operations, as efficient resource use translates to reduced greenhouse gas emissions (Ehsani et al., 1999).
5 Integration of IoT sensors with AI and ML
The integration of IoT sensors with AI and ML is revolutionizing precision agriculture by enabling real-time data collection, predictive analytics, and automated decision-making (Zhang et al., 2021; Hoque and Padhiary, 2024). IoT sensors deployed across agricultural fields continuously monitor and transmit real-time environmental and crop data, collecting information on key parameters such as soil moisture and pH, temperature and humidity, light intensity, leaf chlorophyll content, and NDVI. These sensors communicate data wirelessly via long range wide area network (LoRaWAN), 5G, or satellite networks, feeding it into AI-powered cloud platforms for further processing (Xu et al., 2022).
AI algorithms process the collected data to identify trends, detect anomalies, and make predictions. Key applications include ML for crop yield prediction, pest and disease detection, smart irrigation and water management, VRT, and decision tree algorithms for resource allocation and precision farming (He, 2023). Real-time decision-making and automated control are possible through AI and ML models continuously analyzing IoT data, allowing automated farming systems to activate smart irrigation systems, adjust greenhouse ventilation and temperature for ideal plant growth conditions, deploy autonomous drones or robotic sprayers for targeted pesticide and nutrient application, and send alerts and recommendations to farmers through mobile applications (Bwambale et al., 2022; Kanimozhi and Vadivel, 2024).
The benefits of IoT-AI integration in precision agriculture include higher crop yields, reduced costs, sustainability, labor efficiency, and climate adaptation. Accurate yield predictions and early disease detection lead to improved productivity, while precision application of resources lowers input costs. Data-driven decisions reduce waste and promote environmentally friendly farming. Automated AI-powered systems minimize manual intervention, and predictive models help farmers adjust to changing weather patterns proactively (Veeramanju, 2024).
6 Challenges associated with precision agriculture
The integration of advanced technologies such as AI, sensors and the IoT in agriculture presents transformative opportunities to enhance productivity and sustainability. However, the adoption of smart farming faces significant barriers that affect its successful implementation. A major obstacle in smart farming is the unclear ownership of data generated by precision agricultural technologies. Farmers produce substantial volumes of data, yet uncertainties regarding data rights, sharing practices, and usage often lead to conflicts and reluctance in adopting new technologies. Additionally, the heterogeneous nature of agricultural data necessitates proprietary software platforms for storage and transfer, further complicating ownership disputes (Wiseman et al., 2019; Demestichas et al., 2020; Saiz-Rubio and Rovira-Más, 2020).
Increased connectivity in smart farming makes systems vulnerable to cyberattacks, including data breaches and unauthorized control of autonomous machinery. Hijacking autonomous systems such as drones, robotic weeders, or tractors can result in severe disruptions, financial losses, and crop damage. The need for robust cybersecurity frameworks to safeguard data privacy and system integrity is critical to building trust and resilience in smart farming (Barreto and Amaral, 2018; Liu et al., 2020). The implementation of smart sensors also comes with a range of challenges that can hinder their effectiveness (Liu et al., 2020).
In soil monitoring, the use of soil moisture sensors and NPK sensors offers critical insights into soil health and fertility. Yet, the high initial costs of these sensors can be a significant barrier for farmers, especially small-scale operators. Additionally, NPK sensors often face challenges related to data accuracy, which can be compromised by issues like improper calibration. This often necessitates additional time and resources for regular calibration and maintenance to ensure reliable readings (Gupta et al., 2020). When it comes to crop health monitoring, multispectral cameras and drone sensors provide powerful tools for assessing crop conditions. However, the complexities associated with data processing and interpretation can overwhelm users without adequate training or resources. Moreover, consistent data management is essential to derive actionable insights; the lack of systematic approaches can lead to missed opportunities for improving crop yields. Weather tracking relies on weather stations and atmospheric sensors, yet this area faces its own set of challenges. These systems often rely on external data sources which can introduce errors into the data if the sources are unreliable. Furthermore, establishing infrastructure for atmospheric sensors in remote areas can be logistically difficult, potentially limiting the coverage and reliability of weather monitoring in sparsely populated agricultural regions (Koduru and Koduru, 2022; Otieno, 2023).
In livestock management, GPS collars and RFID tags enhance tracking and monitoring of animals, contributing to better herd management. However, concerns around privacy and data security can pose significant hurdles for adoption (Akhigbe et al., 2021). Additionally, the reliance on battery-operated devices like RFID tags presents challenges, as the effectiveness of these systems is contingent upon maintaining adequate battery life to ensure uninterrupted functionality. Supply chain efficiency through IoT-enabled GPS devices and environmental sensors contributes to streamlined operations, yet the complexity of data integration can complicate the process (Tan and Sidhu, 2022). Farmers and supply chain managers must navigate various data formats and platforms, which can lead to inconsistencies and inefficiencies. The potential for system failures in environmental sensors is another concern, as these interruptions can adversely affect operational continuity and productivity (Kleinschmidt et al., 2019; Sarma and Barbhuiya, 2019; de Araujo Zanella et al., 2020; Van Der Linden et al., 2020; Yazdinejad et al., 2021; McCaig et al., 2023).
Lastly, in the realm of sustainability, smart irrigation sensors and pH sensors represent vital innovations for resource management. However, they require ongoing maintenance and calibration to operate effectively, which can be a significant commitment for farmers. Additionally, there can be resistance from traditional farming practices, where growers may be hesitant to adopt new technologies without clear demonstrable benefits (Klerkx et al., 2019; Koduru and Koduru, 2022; Tiwari et al., 2024). Implementing IoT platforms in agriculture demands adaptability to local conditions and often requires substantial customization, making it resource-intensive. Farmers with limited technical expertise may find these systems difficult to manage, adding to implementation challenges (Gupta et al., 2020). Farmers must navigate varying regional regulations on data protection, environmental standards, and agricultural practices. Meeting these complex legal requirements can be burdensome, especially in the absence of clear guidelines (Ali et al., 2024). The absence of uniform standards for smart farming technologies creates compatibility issues among devices and platforms, hindering seamless integration (Koduru and Koduru, 2022; Otieno, 2023).
The initial investment and operational expenses for advanced technologies can be prohibitive, particularly for small-scale farmers. Limited access to affordable options exacerbates the digital divide, leaving smaller operators at a disadvantage compared to large-scale enterprises [129]. Many farmers hesitate to adopt smart technologies due to uncertain profitability. The lack of concrete evidence demonstrating financial benefits further delays widespread adoption (Tiwari et al., 2024). Limited power availability and poor connectivity in rural regions pose additional barriers to using smart farming tools. Advances in wireless power transfer and on-site energy generation are needed to mitigate these limitations. A significant knowledge gap exists between farmers and the technologies they are expected to use. While farmers possess practical expertise, many lack the specialized training required to operate sophisticated tools driven by AI and big data (Liu et al., 2020).
Ongoing education and skill development are essential for the effective use of smart farming systems. However, limited access to training programs in rural areas, along with a growing demand for skilled labor, may displace traditional agricultural workers (McCaig et al., 2023). A significant challenge with IoT devices used in smart farming is their outdoor installation, which exposes them to harsh environmental conditions such as heavy rain, dust, wind, and extreme temperatures. These adverse conditions can lead to unforeseen mechanical failures in sophisticated devices. To address this issue, manufacturers of IoT devices should utilize materials that can endure these environmental stresses, thereby enhancing the durability and reliability of their products for consistent performance over time (Rajak et al., 2023).Environmental exposure causes gradual sensor deterioration due to factors like corrosion and dust, reducing data accuracy. The limited computational capacity of agricultural sensors also makes implementing robust security measures challenging, increasing the system’s vulnerability to attacks such as sleep deprivation, which depletes battery life and disrupts data collection (Sarma and Barbhuiya, 2019).
Many farmers resist adopting new technologies due to adherence to traditional practices and skepticism about their benefits. Demonstrating tangible, long-term advantages is key to overcoming this reluctance (Van Der Linden et al., 2020) about data privacy, security, and the reliability of AI-driven decisions discourage full engagement with smart farming systems. Building trust through transparency and reliable performance is essential for widespread adoption (Kleinschmidt et al., 2019; de Araujo Zanella et al., 2020; Yazdinejad et al., 2021). Enhancing transparency and reliable performance is crucial for building trust in smart farming systems, particularly regarding data privacy, security, and AI-driven decision-making. Transparency involves clearly communicating how data is collected, stored, and utilized, allowing farmers to understand what information is being gathered and how it benefits their operations. By openly sharing details about algorithms used to make decisions, alongside robust data privacy policies that comply with regulations, stakeholders can alleviate concerns about data misuse and unauthorized access. This clarity ensures that farmers feel more secure in adopting smart farming technologies, knowing that their data is managed responsibly (Supplementary Table S1). Reliable performance, on the other hand, relates to the consistent accuracy and effectiveness of AI-driven technologies in improving agricultural outcomes. When farmers observe tangible benefits, such as increased yields or resource optimization, they are more likely to trust these systems (Koduru and Koduru, 2022). Providing access to performance metrics and establishing feedback mechanisms helps demonstrate the technology’s effectiveness and fosters a sense of partnership. Furthermore, offering training and support empowers farmers to use these systems competently, while creating community platforms for sharing experiences enhances mutual trust. Together, these elements form a foundation for widespread adoption of smart farming technologies, ultimately leading to more sustainable agricultural practices (Klerkx et al., 2019). While smart farming offers immense potential to revolutionize agriculture, its success depends on addressing a diverse array of technical, regulatory, economic, educational, and social barriers. Collaborative efforts among stakeholders—including farmers, technology developers, policymakers, and researchers—are vital to creating sustainable and inclusive smart farming ecosystems.
7 Conclusion and future
Precision agriculture, driven by the integration of sensors and the IoT, presents a transformative opportunity to enhance agricultural productivity and sustainability. In this review we explored the diverse array of sensor technologies currently employed in precision agriculture. While offering significant benefits, such as optimized resource utilization, increased yields, and improved decision-making, the widespread adoption of these technologies faces considerable challenges. High initial investment costs, the need for specialized expertise, data security concerns, and infrastructural limitations in rural areas pose significant barriers. Furthermore, the lack of clear data ownership guidelines and compatibility issues among various sensor systems and platforms hinder seamless integration.
Future for precision agriculture are promising, particularly given the ongoing advancements in AI, machine learning, and sensor technologies. Digital twins, virtual farm replicas powered by IoT data, will enable farmers to test strategies and optimize operations in simulated environments. Sustainability will be a central focus, with IoT technologies promoting carbon sequestration, minimizing resource waste, and leveraging renewable energy-powered devices. Improved connectivity through 5G networks and low power wide area network (LPWANs) will bridge gaps in rural and remote farming areas, enabling real-time monitoring and control of agricultural systems. Customizable and scalable IoT platforms will enhance affordability and usability, expanding accessibility. The development of more affordable and user-friendly systems, coupled with targeted training and education programs for farmers, will be crucial in expanding the reach and impact of precision agriculture. Addressing the data security concerns through robust cybersecurity frameworks and establishing clear data ownership protocols will foster trust and encourage wider adoption.
Practical applications in real production include automated smart irrigation, AI-powered crop health monitoring and pest control, autonomous farming equipment, supply chain optimization through blockchain and IoT, and greenhouse automation for controlled environments. These technologies provide significant economic advantages by increasing productivity, reducing input costs, and enhancing efficiency. Farmers can maximize yields while minimizing operational expenses, resulting in higher profitability and greater resilience to market fluctuations. Precision farming techniques reduce waste, conserve natural resources, and lower the carbon footprint, making agriculture more sustainable and environmentally friendly. Collaborative efforts among technology developers, policymakers, and farmers are essential to scaling up adoption of precision agriculture. Investment in affordable, user-friendly IoT solutions, standardization of sensor technologies, and education initiatives will ensure broader accessibility to smart farming practices. Establishing robust cybersecurity frameworks and clear data ownership policies will foster trust and encourage more widespread implementation.
Author contributions
SM: Conceptualization, Data curation, Formal analysis, Investigation, Software, Validation, Visualization, Writing – original draft, Writing – review & editing. SI: Data curation, Formal analysis, Software, Validation, Writing – original draft, Writing – review & editing. SP: Formal analysis, Investigation, Validation, Visualization, Writing – review & editing. SK: Conceptualization, Formal analysis, Investigation, Supervision, Validation, Visualization, Writing – review & editing. YC: Conceptualization, Formal analysis, Funding acquisition, Project administration, Visualization, Writing – review & editing. JB: Conceptualization, Data curation, Formal analysis, Funding acquisition, Investigation, Project administration, Resources, Software, Supervision, Validation, Visualization, Writing – review & editing.
Funding
The author(s) declare that financial support was received for the research and/or publication of this article. This work was carried out with the support of “Cooperative Research Program for Agriculture Science and Technology Development (Project No. PJ01723801),” Rural Development Administration, Republic of Korea. This research also was supported by the Brain Pool Plus program funded by the Ministry of Science and ICT through the National Research Foundation of Korea (Grant No. RS-2024-00403759) and the KAIST-UC Berkeley-VNU Climate Change Research Center (Grant No. 2021K1A4A8A01079356).
Conflict of interest
The authors declare that the research was conducted in the absence of any commercial or financial relationships that could be constructed as a potential conflict of interest.
Generative AI statement
The author(s) declare that no Generative AI was used in the creation of this manuscript.
Publisher’s note
All claims expressed in this article are solely those of the authors and do not necessarily represent those of their affiliated organizations, or those of the publisher, the editors and the reviewers. Any product that may be evaluated in this article, or claim that may be made by its manufacturer, is not guaranteed or endorsed by the publisher.
Supplementary material
The Supplementary Material for this article can be found online at: https://www.frontiersin.org/articles/10.3389/fpls.2025.1587869/full#supplementary-material
References
Abdulraheem, M. I., Zhang, W., Li, S., Moshayedi, A. J., Farooque, A. A., Hu, J. (2023). Advancement of remote sensing for soil measurements and applications: A comprehensive review. Sustainability 15, 15444. doi: 10.3390/su152115444
Abioye, E. A., Abidin, M. S. Z., Mahmud, M. S. A., Buyamin, S., Ishak, M. H. I., Abd Rahman, M. K. I., et al. (2020). A review on monitoring and advanced control strategies for precision irrigation. Comput. Electron. Agric. 173, 105441. doi: 10.1016/j.compag.2020.105441
Abioye, A. E., Abidin, M. S. Z., Mahmud, M. S. A., Buyamin, S., Mohammed, O. O., Otuoze, A. O., et al. (2023). Model based predictive control strategy for water saving drip irrigation. Smart. Agric. Technol. 4, 100179. doi: 10.1016/j.atech.2023.100179
Acharya, B., Garikapati, K., Yarlagadda, A., Dash, S. (2022). “Internet of things (IoT) and data analytics in smart agriculture: Benefits and challenges,” in AI, Edge and IoT-based Smart Agriculture (USA: Elsevier), 3–16.
Adamchuk, V. I., Lund, E. D., Sethuramasamyraja, B., Morgan, M. T., Dobermann, A., Marx, D. B. (2005). Direct measurement of soil chemical properties on-the-go using ion-selective electrodes. Comput. Electron. Agric. 48, 272–294. doi: 10.1016/j.compag.2005.05.001
Afzaal, H., Farooque, A. A., Abbas, F., Acharya, B., Esau, T. (2020). Precision irrigation strategies for sustainable water budgeting of potato crop in Prince Edward Island. Sustainability 12, 2419. doi: 10.3390/su12062419
Aggarwal, S., Verma, A. (2022). “Transformations in the ways of improving from agriculture 1.0 to 4.0,” in 2022 5th International Conference on Contemporary Computing and Informatics (IC3I). 170–174 (IEEE).
Ahmad, S. F., Dar, A. H. (2020). Precision Farming for Resource Use Efficiency. In: Kumar, S., Meena, R. S., Jhariya, M. K. (eds) Resources Use Efficiency in Agriculture. Springer, Singapore. doi: 10.1007/978-981-15-6953-1_4
Akbar, J. U. M., Kamarulzaman, S. F., Muzahid, A. J. M., Rahman, M. A., Uddin, M. (2024). A comprehensive review on deep learning assisted computer vision techniques for smart greenhouse agriculture. IEEE Access. doi: 10.1109/ACCESS.2024.3349418
Akhigbe, B. I., Munir, K., Akinade, O., Akanbi, L., Oyedele, L. O. (2021). IoT technologies for livestock management: a review of present status, opportunities, and future trends. Big. Data Cogn. Comput. 5, 10. doi: 10.3390/bdcc5010010
Alharbi, S., Felemban, A., Abdelrahim, A., Al-Dakhil, M. (2024). Agricultural and Technology-based strategies to improve water-use efficiency in Arid and Semiarid areas. Water (Basel). 16, 1842. doi: 10.3390/w16131842
Ali, A., Hussain, T., Tantashutikun, N., Hussain, N., Cocetta, G. (2023). Application of smart techniques, internet of things and data mining for resource use efficient and sustainable crop production. Agriculture 13, 397. doi: 10.3390/agriculture13020397
Ali, G., Mijwil, M. M., Buruga, B. A., Abotaleb, M., Adamopoulos, I. (2024). A survey on artificial intelligence in cybersecurity for smart agriculture: state-of-the-art, cyber threats, artificial intelligence applications, and ethical concerns. Mesopotamian. J. Comput. Sci. 2024, 53–103. doi: 10.58496/MJCSC/2024/007
Aravamudhan, S., Bhansali, S. (2008). Development of micro-fluidic nitrate-selective sensor based on doped-polypyrrole nanowires. Sens. Actuators. B. Chem. 132, 623–630. doi: 10.1016/j.snb.2008.01.046
Balafoutis, A. T., Beck, B., Fountas, S., Tsiropoulos, Z., Vangeyte, J., van der Wal, T., et al. (2017). Smart Farming Technologies – Description, Taxonomy and Economic Impact. In: Pedersen, S., Lind, K. (eds) Precision Agriculture: Technology and Economic Perspectives. Progress in Precision Agriculture. Springer, Cham. doi: 10.1007/978-3-319-68715-5_2
Barreto, L., Amaral, A. (2018). “Smart farming: Cyber security challenges,” in 2018 International Conference on Intelligent Systems (IS). 870–876 (IEEE).
Bashir, S. S., Hussain, A., Hussain, S. J., Wani, O. A., Zahid Nabi, S., Dar, N. A., et al. (2021). Plant drought stress tolerance: Understanding its physiological, biochemical and molecular mechanisms. Biotechnol. Biotechnol. Equip. 35, 1912–1925. doi: 10.1080/13102818.2021.2020161
Bogoviz, A. V., Osipov, V. S., Vorozheykina, T. M., Yankovskaya, V. V., Sklyarov, I. Y. (2023). “Food security in the digital economy: traditional agriculture vs. Smart agriculture based on artificial intelligence,” in Food Security in the Economy of the Future: Transition from Digital Agriculture to Agriculture 4.0 Based on Deep Learning (Switzerland: Springer), 59–74.
Bollero, G. A., Bullock, D. G., Hollinger, S. E. (1996). Soil temperature and planting date effects on corn yield, leaf area, and plant development. Agron. J. 88, 385–390. doi: 10.2134/agronj1996.00021962008800030005x
Borgogno Mondino, E., Gajetti, M. (2017). Preliminary considerations about costs and potential market of remote sensing from UAV in the Italian viticulture context. Eur. J. Remote Sens. 50, 310–319. doi: 10.1080/22797254.2017.1328269
Botta, A., Cavallone, P., Baglieri, L., Colucci, G., Tagliavini, L., Quaglia, G. (2022). A review of robots, perception, and tasks in precision agriculture. Appl. Mech. 3, 830–854. doi: 10.3390/applmech3030049
Burton, L., Jayachandran, K., Bhansali, S. (2020a). Review—The “Real-time” Revolution for in situ soil nutrient sensing. J. Electrochem. Soc. 167, 37569. doi: 10.1149/1945-7111/ab6f5d
Burton, L., Jayachandran, K., Bhansali, S. (2020b). The “Real-Time” revolution for in situ soil nutrient sensing. J. Electrochem. Soc. 167, 037569. doi: 10.1149/1945-7111/ab6f5d
Bwambale, E., Abagale, F. K., Anornu, G. K. (2022). Smart irrigation monitoring and control strategies for improving water use efficiency in precision agriculture: A review. Agric. Water Manag. 260, 107324. doi: 10.1016/j.agwat.2021.107324
Casavola, A., Gagliardi, G. (2012). Fault detection and isolation of electrical induction motors via LPV fault observers. IFAC. Proc. 45, 800–805. doi: 10.3182/20120829-3-MX-2028.00112
Charania, I., Li, X. (2020). Smart farming: Agriculture’s shift from a labor intensive to technology native industry. Internet Things. 9, 100142. doi: 10.1016/j.iot.2019.100142
Childs, P. R. N., Greenwood, J. R., Long, C. A. (2000). Review of temperature measurement. Rev. Sci. Instruments. 71, 2959–2978. doi: 10.1063/1.1305516
Chin, R., Catal, C., Kassahun, A. (2023). Plant disease detection using drones in precision agriculture. Precis. Agric. 24, 1663–1682. doi: 10.1007/s11119-023-10014-y
Christy, C. D., Drummond, P., Laird, D. A. (2003). “An on-the-go spectral reflectance sensor for soil,” in 2003 ASAE Annual Meeting (USA: American Society of Agricultural and Biological Engineers), 1.
Crioni, P. L. B., Teramoto, E. H., da Cunha, C. F., Kiang, C. H. (2025). Evaluation of the OPTRAM using sentinel-2 imagery to estimate soil moisture in urban environments. Rev. Bras. Geografia. Física. 18, 605–621. doi: 10.26848/rbgf.v18.1.p605-621
Das, V. J., Sharma, S., Kaushik, A. (2019). Views of Irish farmers on smart farming technologies: An observational study. AgriEngineering 1, 164–187. doi: 10.3390/agriengineering1020013
da Silveira, F. D., Amaral, F. G. (2022). Agriculture 4.0. In: Zhang, Q. (eds) Encyclopedia of Smart Agriculture Technologies. Springer, Cham. doi: 10.1007/978-3-030-89123-7_207-1
Davaji, B., Cho, H. D., Malakoutian, M., Lee, J.-K., Panin, G., Kang, T. W., et al. (2017). A patterned single layer graphene resistance temperature sensor. Sci. Rep. 7, 8811. doi: 10.1038/s41598-017-08967-y
de Araujo Zanella, A. R., da Silva, E., Albini, L. C. P. (2020). Security challenges to smart agriculture: Current state, key issues, and future directions. Array 8, 100048. doi: 10.1016/j.array.2020.100048
de Jong, S. M., Heijenk, R. A., Nijland, W., van der Meijde, M. (2020). Monitoring soil moisture dynamics using electrical resistivity tomography under homogeneous field conditions. Sensors 20, 5313. doi: 10.3390/s20185313
de la Parte, M. S. E., Martínez-Ortega, J.-F., Castillejo, P., Lucas-Martínez, N. (2024). Spatio-temporal semantic data management systems for IoT in agriculture 5.0: Challenges and future directions. Internet Things. 25, 101030.
Demestichas, K., Peppes, N., Alexakis, T. (2020). Survey on security threats in agricultural IoT and smart farming. Sensors 20, 6458. doi: 10.3390/s20226458
Ehsani, M. R., Upadhyaya, S. K., Fawcett, W. R., Protsailo, L. V., Slaughter, D. (2001). Feasibility of detecting soil nitrate content using a mid–infrared technique. Transactions of the ASAE 44 (6), 1931.
Ehsani, M. R., Upadhyaya, S. K., Slaughter, D., Shafii, S., Pelletier, M. (1999). A NIR technique for rapid determination of soil mineral nitrogen. Precis. Agric. 1, 219–236. doi: 10.1023/A:1009916108990
Evans, D. (2011). The internet of things. How the Next Evolution of the Internet is Changing Everything, Whitepaper, Cisco Internet Business Solutions Group (IBSG), Vol. 1. 1–12.
Evett, S. R., O’Shaughnessy, S. A., Andrade, M. A., Kustas, W. P., Anderson, M. C., Schomberg, H. H., et al. (2020). Precision agriculture and irrigation: Current US perspectives. Trans. ASABE. 63, 57–67. doi: 10.13031/trans.13355
Farooqui, N. A., Haleem, M., Khan, W., Ishrat, M. (2024). “Precision agriculture and predictive analytics: enhancing agricultural efficiency and yield,” in Intelligent techniques for Predictive Data Analytics, 171–188. New Jersey, US.
Fauziah, N. O., Fitriatin, B. N., Fakhrurroja, H., Simarmata, T. (2024). Enhancing soil nutritional status in smart farming: the role of IoT-based management for meeting plant requirements. Int. J. Agron. 2024, 8874325. doi: 10.1155/2024/8874325
Fraser, E. D. G., Campbell, M. (2019). Agriculture 5.0: reconciling production with planetary health. One Earth 1, 278–280. doi: 10.1016/j.oneear.2019.10.022
Freeman, P. K., Freeland, R. S. (2015). Agricultural UAVs in the US: potential, policy, and hype. Remote Sens. Appl. 2, 35–43.
Gagliardi, G., Cosma, A. I. M., Marasco, F. (2022). A decision support system for sustainable agriculture: The case study of coconut oil extraction process. Agronomy 12, 177. doi: 10.3390/agronomy12010177
Galieni, A., D’Ascenzo, N., Stagnari, F., Pagnani, G., Xie, Q., Pisante, M. (2021). Past and future of plant stress detection: an overview from remote sensing to positron emission tomography. Front. Plant Sci. 11. doi: 10.3389/fpls.2020.609155
Garlando, U., Bar-On, L., Avni, A., Shacham-Diamand, Y., Demarchi, D. (2020). “Plants and environmental sensors for smart agriculture, an overview,” in 2020 IEEE SENSORS, 1–4.
Garnaik, S., Nayak, J. (2024). “Biosensor: A tool for assessment of soil pollutants,” in Applied Biotechnology and Bioinformatics: Agriculture, Pharmaceutical Research and Environment, 395–406. New Jersey USA.
Gemtou, M., Kakkavou, K., Anastasiou, E., Fountas, S., Pedersen, S. M., Isakhanyan, G., et al. (2024). Farmers’ Transition to climate-smart agriculture: A systematic review of the decision-making factors affecting adoption. Sustainability 16, 2828. doi: 10.3390/su16072828
Getahun, S., Kefale, H., Gelaye, Y. (2024). Application of precision agriculture technologies for sustainable crop production and environmental sustainability: A systematic review. Sci. World J. 2024, 2126734. doi: 10.1155/2024/2126734
Gollin, D. (2010). Agricultural productivity and economic growth. Handb. Agric. Econ. 4, 3825–3866. doi: 10.1016/S1574-0072(09)04073-0
Gómez Romero, C. D., Díaz Barriga, J. K., Rodríguez Molano, J. I. (2016). “Big data meaning in the architecture of IoT for smart cities,” in Data Mining and Big Data: First International Conference, DMBD 2016, Bali, Indonesia, June 25-30, 2016. 457–465 (Springer), Proceedings 1.
Guerrero, A., De Neve, S., Mouazen, A. M. (2021). Current sensor technologies for in situ and on-line measurement of soil nitrogen for variable rate fertilization: A review. Adv. Agron. 168, 1–38. doi: 10.1016/bs.agron.2021.02.001
Gupta, M., Abdelsalam, M., Khorsandroo, S., Mittal, S. (2020). Security and privacy in smart farming: Challenges and opportunities. IEEE Access 8, 34564–34584. doi: 10.1109/Access.6287639
Han, R. (2024). Application of inertial navigation high precision positioning system based on SVM optimization. Syst. Soft. Comput. 6, 200105. doi: 10.1016/j.sasc.2024.200105
Hashim, N. M. Z., Mazlan, S. R., Abd Aziz, M. Z. A., Salleh, A., Jaâ, A. S., Mohamad, N. R. (2015). Agriculture monitoring system: a study. J. Teknol. 77. doi: 10.11113/jt.v77.4099
Hassanpour, R., Zarehaghi, D., Neyshabouri, M. R., Feizizadeh, B., Rahmati, M. (2020). Modification on optical trapezoid model for accurate estimation of soil moisture content in a maize growing field. J. Appl. Remote Sens. 14, 34519. doi: 10.1117/1.JRS.14.034519
Hatfield, J. L., Prueger, J. H. (2015). Temperature extremes: Effect on plant growth and development. Weather. Clim. Extrem. 10, 4–10. doi: 10.1016/j.wace.2015.08.001
He, L. (2023). “Variable rate technologies for precision agriculture,” in Encyclopedia of Digital Agricultural Technologies (Switzerland: Springer), 1533–1542.
Hoque, A., Padhiary, M. (2024). Automation and AI in precision agriculture: innovations for enhanced crop management and sustainability. Asian J. Res. Comput. Sci. 17, 95–109. doi: 10.9734/ajrcos/2024/v17i10512
Horváth, J., Kátai, L., Szabó, I., Korzenszky, P. (2024). An electrical conductivity sensor for the selective determination of soil salinity. Sensors 24, 3296. doi: 10.3390/s24113296
Hurst, Z. M., Spiegal, S. (2023). Design thinking for responsible agriculture 4.0 innovations in rangelands. Rangelands 45, 68–78. doi: 10.1016/j.rala.2023.03.003
Ingrao, C., Strippoli, R., Lagioia, G., Huisingh, D. (2023). Water scarcity in agriculture: An overview of causes, impacts and approaches for reducing the risks. Heliyon. doi: 10.1016/j.heliyon.2023.e18507
Işık, M. F., Sönmez, Y., Yılmaz, C., Özdemir, V., Yılmaz, E. N. (2017). Precision irrigation system (PIS) using sensor network technology integrated with IOS/Android application. Appl. Sci. 7, 891. doi: 10.3390/app7090891
Isaac, J. O. (2021). IOT-Livestock monitoring and management system. Int. J. Eng. Appl. Sci. Technol. 5, 254–257.
Islam, M. H., Anam, M. Z., Hoque, M. R., Nishat, M., Bari, A. B. M. M. (2024). Agriculture 4.0 adoption challenges in the emerging economies: Implications for smart farming and sustainability. J. Econ. Technol. 2, 278–295. doi: 10.1016/j.ject.2024.09.002
Jahn, B. R., Linker, R., Upadhyaya, S. K., Shaviv, A., Slaughter, D. C., Shmulevich, I. (2006). Mid-infrared spectroscopic determination of soil nitrate content. Biosyst. Eng. 94, 505–515. doi: 10.1016/j.biosystemseng.2006.05.011
Jain, H. K. (2012). Transition to twenty-first century agriculture: change of direction. Agric. Res. 1, 12–17. doi: 10.1007/s40003-011-0008-0
Janc, K., Czapiewski, K., Wójcik, M. (2019). In the starting blocks for smart agriculture: The internet as a source of knowledge in transitional agriculture. NJAS-Wageningen. J. Life Sci. 90, 100309. doi: 10.1016/j.njas.2019.100309
Javaid, M., Haleem, A., Singh, R. P., Suman, R. (2022). Enhancing smart farming through the applications of Agriculture 4.0 technologies. Int. J. Intelligent. Networks 3, 150–164. doi: 10.1016/j.ijin.2022.09.004
Juwono, F. H., Wong, W. K., Verma, S., Shekhawat, N., Lease, B. A., Apriono, C. (2023). Machine learning for weed–plant discrimination in agriculture 5.0: An in-depth review. Artif. Intell. Agricult.
Kalita, A., Chakraborty, M., Biswas, K. (2017). “A comparative study of polymer coated capacitive sensors for soil moisture sensing,” in 2017 IEEE International Instrumentation and Measurement Technology Conference (I2MTC). 1–6 (IEEE).
Kanimozhi, A., Vadivel, R. (2024). Optimized water management for precision agriculture using IoT-based smart irrigation system. World J. Adv. Res. Rev. 21, 802–811. doi: 10.30574/wjarr.2024.21.3.0682
Karunathilake, E., Le, A. T., Heo, S., Chung, Y. S., Mansoor, S. (2023). The path to smart farming: Innovations and opportunities in precision agriculture. Agriculture 13, 1593. doi: 10.3390/agriculture13081593
Kazakis, N. A., Tsirliganis, N. C. (2023). Holistic approach to Agriculture 4.0 for new farmers. Soc. Impacts. 1, 100007.
Khan, F. A., Ibrahim, A. A., Zeki, A. M. (2020). Environmental monitoring and disease detection of plants in smart greenhouse using internet of things. J. Phys. Commun. 4, 055008. doi: 10.1088/2399-6528/ab90c1
Khanal, S., Kc, K., Fulton, J. P., Shearer, S., Ozkan, E. (2020). Remote sensing in agriculture—accomplishments, limitations, and opportunities. Remote Sens. (Basel). 12, 3783. doi: 10.3390/rs12223783
Kiropoulos, K., Bibi, S., Vakouftsi, F., Pantzios, V. (2021). “Precision agriculture investment return calculation tool,” in 2021 17th International Conference on Distributed Computing in Sensor Systems (DCOSS). 267–271 (IEEE).
Kleinschmidt, J. H., Kamienski, C., Prati, R. C., Kolehmainen, K., Aguzzi, C. (2019). “End-to-end security in the IoT computing continuum: Perspectives in the SWAMP project,” in 2019 9th Latin-American Symposium on Dependable Computing (LADC). 1–2 (IEEE).
Klerkx, L., Jakku, E., Labarthe, P. (2019). A review of social science on digital agriculture, smart farming and agriculture 4.0: New contributions and a future research agenda. NJAS-Wageningen. J. Life Sci. 90, 100315.
Koduru, T., Koduru, N. P. (2022). An overview of vulnerabilities in smart farming systems. J. Student. Res. 11. doi: 10.47611/jsrhs.v11i1.2303
Konfo, T. R. C., Chabi, A. B. P., Gero, A. A., Lagnika, C., Avlessi, F., Biaou, G., et al. (2024). Recent climate-smart innovations in agrifood to enhance producer incomes through sustainable solutions. J. Agric. Food Res. 15, 100985. doi: 10.1016/j.jafr.2024.100985
Kool, D., Heitman, J. L., Lazarovitch, N., Agam, N., Sauer, T. J., Ben-Gal, A. (2016). In situ thermistor calibration for improved measurement of soil temperature gradients. Soil Sci. Soc. America J. 80, 1514–1519. doi: 10.2136/sssaj2016.05.0134
Koul, B., Yakoob, M., Shah, M. P. (2022). Agricultural waste management strategies for environmental sustainability. Environ. Res. 206, 112285. doi: 10.1016/j.envres.2021.112285
Krzeminska, D., Bloem, E., Starkloff, T., Stolte, J. (2022). Combining FDR and ERT for monitoring soil moisture and temperature patterns in undulating terrain in south-eastern Norway. Catena. (Amst). 212, 106100. doi: 10.1016/j.catena.2022.106100
Ku, K.-B., Mansoor, S., Han, G. D., Chung, Y. S., Tuan, T. T. (2023). Identification of new cold tolerant Zoysia grass species using high-resolution RGB and multi-spectral imaging. Sci. Rep. 13, 13209. doi: 10.1038/s41598-023-40128-2
Kumar, S., Meena, R. S., Sheoran, S., Jangir, C. K., Jhariya, M. K., Banerjee, A., et al. (2022a). “Remote sensing for agriculture and resource management,” in Natural Resources Conservation and Advances for Sustainability (He (Switzerland): Elsevier), 91–135.
Kumar, A., Singh, V., Kumar, S., Jaiswal, S. P., Bhadoria, V. S. (2022b). IoT enabled system to monitor and control greenhouse. Mater. Today.: Proc. 49, 3137–3141. doi: 10.1016/j.matpr.2020.11.040
Latino, M. E., Menegoli, M., Lazoi, M., Corallo, A. (2022). Voluntary traceability in food supply chain: a framework leading its implementation in Agriculture 4.0. Technol. Forecast. Soc. Change 178, 121564.
Lavanaya, M., Parameswari, R. (2018). “Soil nutrients monitoring for greenhouse yield enhancement using pH value with IOT and wireless sensor network,” in 2018 Second International Conference on Green Computing and Internet of Things (ICGCIoT). 547–552 (IEEE).
Li, S., Zhang, M., Ji, Y., Zhang, Z., Cao, R., Chen, B., et al. (2021). Agricultural machinery GNSS/IMU-integrated navigation based on fuzzy adaptive finite impulse response Kalman filtering algorithm. Comput. Electron. Agric. 191, 106524. doi: 10.1016/j.compag.2021.106524
Liang, C., Shah, T. (2023). IoT in agriculture: The future of precision monitoring and data-driven farming. Eigenpub. Rev. Sci. Technol. 7, 85–104.
Lipper, L., Thornton, P., Campbell, B. M., Baedeker, T., Braimoh, A., Bwalya, M., et al. (2014). Climate-smart agriculture for food security. Nat. Clim. Chang. 4, 1068–1072. doi: 10.1038/nclimate2437
Liu, C., Jiang, X., Yuan, Z. (2024). Plant Responses and adaptations to salt stress: A review. Horticulturae 10, 1221. doi: 10.3390/horticulturae10111221
Liu, Y., Ma, X., Shu, L., Hancke, G. P., Abu-Mahfouz, A. M. (2020). From industry 4.0 to agriculture 4.0: Current status, enabling technologies, and research challenges. IEEE Trans. Industr. Inform. 17, 4322–4334. doi: 10.1109/TII.2020.3003910
Liu, Y., Ma, X., Shu, L., Hancke, G. P., Abu-Mahfouz, A. M. (2021). From industry 4.0 to agriculture 4.0: current status, enabling technologies, and research challenges. IEEE Trans. Ind. Inform. 17, 4322–4334. doi: 10.1109/TII.2020.3003910
Liu, C., Xu, D., Dong, X., Huang, Q. (2022). A review: Research progress of SERS-based sensors for agricultural applications. Trends Food Sci. Technol. 128, 90–101. doi: 10.1016/j.tifs.2022.07.012
Lundborg, P. (1990). Rural-urban migration and the transition from traditional to modern agriculture. J. Dev. Econ. 33, 287–307. doi: 10.1016/0304-3878(90)90025-7
Ma, C., Johansen, K., McCabe, M. F. (2022). Combining Sentinel-2 data with an optical-trapezoid approach to infer within-field soil moisture variability and monitor agricultural production stages. Agric. Water Manag. 274, 107942. doi: 10.1016/j.agwat.2022.107942
Maffezzoli, F., Ardolino, M., Bacchetti, A. (2024). Maturity level and Effects of the 4.0 Paradigm on the Italian Agricultural Industry: A preliminary study. Proc. Comput. Sci. 232, 1819–1828. doi: 10.1016/j.procs.2024.02.004
Mallareddy, M., Thirumalaikumar, R., Balasubramanian, P., Naseeruddin, R., Nithya, N., Mariadoss, A., et al. (2023). Maximizing water use efficiency in rice farming: A comprehensive review of innovative irrigation management technologies. Water (Basel). 15, 1802. doi: 10.3390/w15101802
Mana, A. A., Allouhi, A., Hamrani, A., Rahman, S., el Jamaoui, I., Jayachandran, K. (2024). Sustainable AI-based production agriculture: exploring AI applications and implications in agricultural practices. Smart. Agric. Technol., 100416. doi: 10.1016/j.atech.2024.100416
Mansoor, S., Ali Wani, O., Lone, J. K., Manhas, S., Kour, N., Alam, P., et al. (2022). Reactive oxygen species in plants: from source to sink. Antioxidants 11, 225. doi: 10.3390/antiox11020225
Mansoor, S., Chung, Y. S. (2024). Functional phenotyping: understanding the dynamic response of plants to drought stress. Curr. Plant Biol., 100331. doi: 10.1016/j.cpb.2024.100331
Mansoor, S., Hamid, S., Tuan, T. T., Park, J. E., Chung, Y. S. (2024a). Advance computational tools for multiomics data learning. Biotechnol. Adv., 108447. doi: 10.1016/j.biotechadv.2024.108447
Mansoor, S., Karunathilake, E. M. B. M., Tuan, T. T., Chung, Y. S. (2024b). Genomics, phenomics, and machine learning in transforming plant research: advancements and challenges. Hortic. Plant J. doi: 10.1016/j.hpj.2023.09.005
Marios, S., Georgiou, J. (2017). “Precision agriculture: Challenges in sensors and electronics for real-time soil and plant monitoring,” in 2017 IEEE Biomedical Circuits and Systems Conference (BioCAS). 1–4 (IEEE).
Math, A., Ali, L., Pruthviraj, U. (2018). “Development of smart drip irrigation system using IoT,” in 2018 IEEE Distributed Computing, VLSI, Electrical Circuits and Robotics (DISCOVER). 126–130 (IEEE).
McCaig, M., Dara, R., Rezania, D. (2023). Farmer-centric design thinking principles for smart farming technologies. Internet Things. 23, 100898. doi: 10.1016/j.iot.2023.100898
McCole, M., Bradley, M., McCaul, M., McCrudden, D. (2023). A low-cost portable system for on-site detection of soil pH and potassium levels using 3D printed sensors. Results. Eng. 20, 101564. doi: 10.1016/j.rineng.2023.101564
Mishra, R. K., Dash, A. R., Panda, A. K. (2025). IoT-enabled smart farming: A cloud-based approach for polyhouse automation. Expert Syst. Appl., 127358. doi: 10.1016/j.eswa.2025.127358
Mogili, U. M. R., Deepak, B. (2018). Review on application of drone systems in precision agriculture. Proc. Comput. Sci. 133, 502–509. doi: 10.1016/j.procs.2018.07.063
Monteiro, A., Santos, S., Gonçalves, P. (2021). Precision agriculture for crop and livestock farming—Brief review. Animals 11, 2345. doi: 10.3390/ani11082345
Mowla, M. N., Mowla, N., Shah, A. F. M. S., Rabie, K. M., Shongwe, T. (2023). Internet of Things and wireless sensor networks for smart agriculture applications: A survey. IEEE Access 11, 145813–145852. doi: 10.1109/ACCESS.2023.3346299
Nackley, L. L., Warneke, B., Fessler, L., Pscheidt, J. W., Lockwood, D., Wright, W. C., et al. (2021). Variable-rate spray technology optimizes pesticide application by adjusting for seasonal shifts in deciduous perennial crops. Horttechnology 31, 479–489. doi: 10.21273/HORTTECH04794-21
Naidu, K. J., Babu, K. V., Sai, C. R. C., Ganesh, P., Sai, T. G., Naidu, N. S. (2024). Precision agriculture monitoring system. Biosci. Biotechnol. Res. Asia. 21, 1543–1551. doi: 10.13005/bbra/
Nargotra, M., Khurjekar, M. J. (2020). “Greenhouse based on IoT and AI for societal benefit,” in Proceedings of the 2020 International Conference on Emerging Smart Computing and Informatics (ESCI), Pune, India, 12–14 March 2020.
Nukala, R., Panduru, K., Shields, A., Riordan, D., Doody, P., Walsh, J. (2016). “Internet of things: A review from ‘Farm to fork,” in 2016 27th Irish signals and systems conference (ISSC). 1–6 (IEEE).
Onwuka, B., Mang, B. (2018). Effects of soil temperature on some soil properties and plant growth. Adv. Plants Agric. Res. 8, 34–37. doi: 10.15406/apar.2018.08.00288
Otieno, M. (2023). An extensive survey of smart agriculture technologies: Current security posture. World J. Adv. Res. Rev. 18, 1207–1231. doi: 10.30574/wjarr.2023.18.3.1241
Outhwaite, C. L., McCann, P., Newbold, T. (2022). Agriculture and climate change are reshaping insect biodiversity worldwide. Nature 605, 97–102. doi: 10.1038/s41586-022-04644-x
Panagopoulos, Y., Makropoulos, C., Gkiokas, A., Kossida, M., Evangelou, L., Lourmas, G., et al. (2014). Assessing the cost-effectiveness of irrigation water management practices in water stressed agricultural catchments: The case of Pinios. Agric. Water Manag. 139, 31–42. doi: 10.1016/j.agwat.2014.03.010
Pandey, P. C., Tripathi, A. K., Sharma, J. K. (2021). “An evaluation of GPS opportunity in market for precision agriculture,” in GPS and GNSS Technology in Geosciences (USA: Elsevier), 337–349.
Passioura, J. B. (2002). Soil conditions and plant growth. Plant Cell Environ. 25, 311–318. doi: 10.1046/j.0016-8025.2001.00802.x
Penn, C. J., Camberato, J. J. (2019). A critical review on soil chemical processes that control how soil pH affects phosphorus availability to plants. Agriculture 9, 120. doi: 10.3390/agriculture9060120
Pham, M. H., Saleem, S. K., Okello, N. (2013). “Real-time optimization of irrigation scheduling in agriculture,” in 2013 25th Chinese Control and Decision Conference (CCDC). 4435–4439 (IEEE).
Pivoto, D., Waquil, P. D., Talamini, E., Finocchio, C. P. S., Dalla Corte, V. F., de Vargas Mores, G. (2018). Scientific development of smart farming technologies and their application in Brazil. Inf. Process. Agric. 5, 21–32. doi: 10.1016/j.inpa.2017.12.002
Popescu, S. M., Mansoor, S., Wani, O. A., Kumar, S. S., Sharma, V., Sharma, A., et al. (2024). Artificial intelligence and IoT driven technologies for environmental pollution monitoring and management. Front. Environ. Sci. 12, 1336088. doi: 10.3389/fenvs.2024.1336088
Pratama, H., Yunan, A., Candra, R. A. (2021). Design and build a soil nutrient measurement tool for citrus plants using NPK soil sensors based on the internet of things. Brilliance.: Res. Artif. Intell. 1, 67–74. doi: 10.47709/brilliance.v1i2.1300
Qazi, S., Khawaja, B. A., Farooq, Q. U. (2022). IoT-equipped and AI-enabled next generation smart agriculture: A critical review, current challenges and future trends. IEEE Access 10, 21219–21235. doi: 10.1109/ACCESS.2022.3152544
Rahman, S. A., Khan, S. A., Iqbal, S., Khadka, I. B., Rehman, M. M., Jang, J., et al. (2024). Hierarchical porous biowaste-based dual humidity/pressure sensor for robotic tactile sensing, sustainable health, and environmental monitoring. Adv. Energy Sustainabil. Res. 5, 2400144. doi: 10.1002/aesr.202400144
Rahman, S. A., Khan, S. A., Iqbal, S., Rehman, M. M., Kim, W. Y. (2023). Eco-friendly, high-performance humidity sensor using purple sweet-potato peel for multipurpose applications. Chemosensors 11, 457. doi: 10.3390/chemosensors11080457
Rajak, P., Ganguly, A., Adhikary, S., Bhattacharya, S. (2023). Internet of Things and smart sensors in agriculture: Scopes and challenges. J. Agric. Food Res. 14, 100776. doi: 10.1016/j.jafr.2023.100776
Ray, S., Haqiqi, I., Hill, A. E., Taylor, J. E., Hertel, T. W. (2023). Labor markets: A critical link between global-local shocks and their impact on agriculture. Environ. Res. Lett. 18, 035007. doi: 10.1088/1748-9326/acb1c9
Rodríguez, F. L., Horváth, K., Martín, J. G., Maestre, J. M. (2017). Mobile model predictive control for the évora irrigation test canal. IFAC-PapersOnLine 50, 6570–6575.
Roper, J. M., Garcia, J. F., Tsutsui, H. (2021). Emerging technologies for monitoring plant health in vivo. ACS Omega. 6, 5101–5107. doi: 10.1021/acsomega.0c05850
Ross, P., Maynard, K. (2021). Towards a 4th industrial revolution. Intelligent. Buildings. Int. 13, 159–161. doi: 10.1080/17508975.2021.1873625
Saeed, Q., Xiukang, W., Haider, F. U., Kučerik, J., Mumtaz, M. Z., Holatko, J., et al. (2021). Rhizosphere bacteria in plant growth promotion, biocontrol, and bioremediation of contaminated sites: a comprehensive review of effects and mechanisms. Int. J. Mol. Sci. 22, 10529. doi: 10.3390/ijms221910529
Saiz-Rubio, V., Rovira-Más, F. (2020). From smart farming towards agriculture 5.0: A review on crop data management. Agronomy 10, 207.
Saqib, M., Almohamad, T. A., Mehmood, R. M. (2020). A low-cost information monitoring system for smart farming applications. Sensors 20, 2367. doi: 10.3390/s20082367
Saqib, M., Khan, S. A., Khan, M., Iqbal, S., Rehman, M. M., Kim, W. Y. (2024). Self-powered humidity sensor driven by triboelectric nanogenerator composed of bio-wasted peanut skin powder. Polymers. (Basel). 16, 790. doi: 10.3390/polym16060790
Sarma, R., Barbhuiya, F. A. (2019). “Internet of Things: attacks and defences,” in 2019 7th International Conference on Smart Computing & Communications (ICSCC). 1–5 (IEEE).
Savich, V. I., Artikova, H. T., Nafetdinov, S. S., Salimova, K. H. (2021). Optimization of plant development in case of soil salinization. Am. J. Agric. Biomed. Eng. 3, 24–29. doi: 10.37547/tajabe/Volume03Issue02-05
Shantaram, A., Beyenal, H., Veluchamy, R. R. A., Lewandowski, Z. (2005). Wireless sensors powered by microbial fuel cells. Environ. Sci. Technol. 39, 5037–5042. doi: 10.1021/es0480668
Sharma, K., Shivandu, S. K. (2024). Integrating artificial intelligence and Internet of Things (IoT) for enhanced crop monitoring and management in precision agriculture. Sensors. Int., 100292. doi: 10.1016/j.sintl.2024.100292
Sheikh, M., Iqra, F., Ambreen, H., Pravin, K. A., Ikra, M., Chung, Y. S. (2024). Integrating artificial intelligence and high-throughput phenotyping for crop improvement. J. Integr. Agric. 23, 1787–1802. doi: 10.1016/j.jia.2023.10.019
Shiyale, P. D., Thombre, H., Tyagi, G., Chauhan, A. (2020). IoT-based smart greenhouse automation. Int. J. Creat. Res. Thoughts. (IJCRT). 8.
Shylendra, S., Wajrak, M., Kang, J. J. (2025). Advancements in solid-state metal pH sensors: A comprehensive review of metal oxides and nitrides for enhanced chemical sensing: A review. IEEE Sens. J. doi: 10.1109/JSEN.2024.3510858
Siddiqui, M. S., Aslam, M. (2023). Three-dimensional conductometric network based on reduced graphene oxide for soil pH sensors. ACS Appl. Nano. Mater. 6, 17376–17386. doi: 10.1021/acsanm.3c02033
Sishodia, R. P., Ray, R. L., Singh, S. K. (2020). Applications of remote sensing in precision agriculture: A review. Remote Sens. (Basel). 12, 3136. doi: 10.3390/rs12193136
Srbinovska, M., Gavrovski, C., Dimcev, V., Krkoleva, A., Borozan, V. (2015). Environmental parameters monitoring in precision agriculture using wireless sensor networks. J. Clean. Prod. 88, 297–307. doi: 10.1016/j.jclepro.2014.04.036
Stańczyk, T., Kasperska-Wołowicz, W., Szatyłowicz, J., Gnatowski, T., Papierowska, E. (2023). Surface soil moisture determination of irrigated and drained agricultural lands with the OPTRAM Method and Sentinel-2 observations. Remote Sens. (Basel). 15, 5576. doi: 10.3390/rs15235576
Sudduth, K. A., Hummel, J. W., Birrell, S. J. (1997). “Sensors for site-specific management,” in The state of site specific management for agriculture. American Society of America, (USA), 183–210.
Tan, W. C., Sidhu, M. S. (2022). Review of RFID and IoT integration in supply chain management. Operations. Res. Perspect. 9, 100229. doi: 10.1016/j.orp.2022.100229
Tenreiro, T. R., Avillez, F., Gómez, J. A., Penteado, M., Coelho, J. C., Fereres, E. (2023). Opportunities for variable rate application of nitrogen under spatial water variations in rainfed wheat systems—an economic analysis. Precis. Agric. 24, 853–878. doi: 10.1007/s11119-022-09977-1
Tiwari, S., Bhardwaj, B., Arora, D., Khatri, S. (2024). “Challenges and barriers to smart farming adaptation: A technical, economic, and social perspective,” in Smart Agritech: Robotics, AI, and Internet of Things (IoT) in Agriculture. American Society of America, (USA), 75–111.
Ullo, S. L., Sinha, G. R. (2021). Advances in IoT and smart sensors for remote sensing and agriculture applications. Remote Sens. (Basel). 13, 2585. doi: 10.3390/rs13132585
Uztürk, D., Büyüközkan, G. (2024). Industry 4.0 technologies in Smart Agriculture: A review and a Technology Assessment Model proposition. Technol. Forecast. Soc. Change 208, 123640.
Van Der Linden, D., Michalec, O. A., Zamansky, A. (2020). Cybersecurity for smart farming: socio-cultural context matters. IEEE Technol. Soc. Magazine. 39, 28–35. doi: 10.1109/MTAS.44
van Staden, J. K. F., Nuta, R.-G., Tatu, G.-L. (2018). Determination of nitrite from water catchment areas using graphite based electrodes. J. Electrochem. Soc. 165, B565. doi: 10.1149/2.0311813jes
Vasilescu, A. G., Pleşoianu, A.-I., Pătru-Stupariu, I. (2023). Aspects of traditional agricultural landscapes: potential alternative development paths for sustainable agriculture—A review. Biodivers. Conserv. 32, 3703–3730. doi: 10.1007/s10531-023-02658-z
Veeramanju, K. T. (2024). Predictive Models for Optimal Irrigation Scheduling and water management: A Review of AI and ML Approaches. Int. J. Manag. Technol. Soc. Sci. (IJMTS). 9, 94–110.
Verma, K. K., Song, X.-P., Joshi, A., Tian, D.-D., Rajput, V. D., Singh, M., et al. (2022). Recent trends in nano-fertilizers for sustainable agriculture under climate change for global food security. Nanomaterials 12, 173. doi: 10.3390/nano12010173
Wang, Z., Qiao, X., Wang, Y., Yu, H., Mu, C. (2024). IoT-based system of prevention and control for crop diseases and insect pests. Front. Plant Sci. 15, 1323074. doi: 10.3389/fpls.2024.1323074
Wang, Z., Zhao, C., Zhang, H., Fan, H. (2011). “Real-time remote monitoring and warning system in general agriculture environment,” in 2011 international conference of information technology, computer engineering and management sciences. 160–163 (IEEE).
Wang, L., Zhou, F., He, M., Liu, H. (2025). Contribution of osmotic suction to suction stress for unsaturated saline clay and its suction stress characteristic curve. J. Geotech. Geoenviron. Eng. 151, 04024143. doi: 10.1061/JGGEFK.GTENG-12813
Wanyama, J., Bwambale, E., Kiraga, S., Katimbo, A., Nakawuka, P., Kabenge, I., et al. (2024). A systematic review of fourth industrial revolution technologies in smart irrigation: constraints, opportunities, and future prospects for sub-Saharan Africa. Smart. Agric. Technol., 100412. doi: 10.1016/j.atech.2024.100412
Wiseman, L., Sanderson, J., Zhang, A., Jakku, E. (2019). Farmers and their data: An examination of farmers’ reluctance to share their data through the lens of the laws impacting smart farming. NJAS-Wageningen. J. Life Sci. 90, 100301. doi: 10.1016/j.njas.2019.04.007
Wu, Y.-D., Chen, Y.-G., Wang, W.-T., Zhang, K.-L., Luo, L.-P., Cao, Y.-C., et al. (2022). Precision fertilizer and irrigation control system using open-source software and loose communication architecture. J. Irrigat. Drainage. Eng. 148, 04022012. doi: 10.1061/(ASCE)IR.1943-4774.0001669
Xu, J., Gu, B., Tian, G. (2022). Review of agricultural IoT technology. Artif. Intell. Agric. 6, 10–22. doi: 10.1016/j.aiia.2022.01.001
Xu, L., Li, L., Tang, L., Zeng, Y., Chen, G., Shao, C., et al. (2023). Rapid printing of high-temperature polymer-derived ceramic composite thin-film thermistor with laser pyrolysis. ACS Appl. Mater. Interfac. 15, 9996–10005. doi: 10.1021/acsami.2c20927
Xu, L.-X., Wang, F., Yao, Y., Yao, M., Kuzyakov, Y., Yu, G.-H., et al. (2024). Key role of microbial necromass and iron minerals in retaining micronutrients and facilitating biological nitrogen fixation in paddy soils. Fundam. Res. doi: 10.1016/j.fmre.2024.02.007
Yaghoubi Khanghahi, M., Strafella, S., Allegretta, I., Crecchio, C. (2021). Isolation of bacteria with potential plant-promoting traits and optimization of their growth conditions. Curr. Microbiol. 78, 464–478. doi: 10.1007/s00284-020-02303-w
Yazdinejad, A., Zolfaghari, B., Azmoodeh, A., Dehghantanha, A., Karimipour, H., Fraser, E., et al. (2021). A review on security of smart farming and precision agriculture: Security aspects, attacks, threats and countermeasures. Appl. Sci. 11, 7518. doi: 10.3390/app11167518
Yin, H., Cao, Y., Marelli, B., Zeng, X., Mason, A. J., Cao, C. (2021). Soil sensors and plant wearables for smart and precision agriculture. Adv. Mater. 33, 2007764. doi: 10.1002/adma.202007764
Zambon, I., Cecchini, M., Egidi, G., Saporito, M. G., Colantoni, A. (2019). Revolution 4.0: Industry vs. agriculture in a future development for SMEs. Processes 7, 36.
Zecha, C. W., Link, J., Claupein, W. (2013). Mobile sensor platforms: categorisation and research applications in precision farming. J. Sensors. Sensor. Syst. 2, 51–72. doi: 10.5194/jsss-2-51-2013
Zhai, Z., Martínez, J. F., Beltran, V., Martínez, N. L. (2020). Decision support systems for agriculture 4.0: Survey and challenges. Comput. Electron. Agric. 170, 105256.
Zhang, X., Feng, G., Sun, X. R. (2024). Advanced technologies of soil moisture monitoring in precision agriculture. J. Agric. Food Res., 101473. doi: 10.1016/j.jafr.2024.101473
Zhang, P., Guo, Z., Ullah, S., Melagraki, G., Afantitis, A., Lynch, I. (2021). Nanotechnology and artificial intelligence to enable sustainable and precision agriculture. Nat. Plants 7, 864–876. doi: 10.1038/s41477-021-00946-6
Zhang, Z., Liu, L., Huang, M., Chen, F., Niu, J., Liu, M. (2022). Feasibility of soil erosion measurement using time domain reflectometry. Catena. (Amst). 218, 106571. doi: 10.1016/j.catena.2022.106571
Zhang, X., Zhang, J., Li, L., Zhang, Y., Yang, G. (2017). Monitoring citrus soil moisture and nutrients using an IoT based system. Sensors 17, 447. doi: 10.3390/s17030447
Keywords: precision agriculture, smart sensors, IoT, big data, automation, challenges
Citation: Mansoor S, Iqbal S, Popescu SM, Kim SL, Chung YS and Baek J-H (2025) Integration of smart sensors and IOT in precision agriculture: trends, challenges and future prospectives. Front. Plant Sci. 16:1587869. doi: 10.3389/fpls.2025.1587869
Received: 06 March 2025; Accepted: 11 April 2025;
Published: 14 May 2025.
Edited by:
Karansher Singh Sandhu, Bayer Crop Science, United StatesReviewed by:
Gurjeet Singh, Texas A and M University, United StatesVinay Kumar Reddy Nannuru, Norwegian University of Life Sciences, Norway
Srikanth Panthulugiri, Iowa State University, United States
Copyright © 2025 Mansoor, Iqbal, Popescu, Kim, Chung and Baek. This is an open-access article distributed under the terms of the Creative Commons Attribution License (CC BY). The use, distribution or reproduction in other forums is permitted, provided the original author(s) and the copyright owner(s) are credited and that the original publication in this journal is cited, in accordance with accepted academic practice. No use, distribution or reproduction is permitted which does not comply with these terms.
*Correspondence: Yong Suk Chung, eXNjaHVuZ0BqZWp1bnUuYWMua3I=; Jeong-Ho Baek, Zmlyc3RsZW9uQGtvcmVhLmty
†These authors have contributed equally to this work